Abstract
Comparison between accelerometric and macroseismic observations is made for three M w = 4.5 earthquakes, which occurred in north-eastern France and south-western Germany in 2003 and 2004. Scalar and spectral instrumental parameters are processed from the accelerometric data recorded by nine accelerometric stations located between 29 and 180 km from the epicentres. Macroseismic data are based on French Internet reports. In addition to the single questionnaire intensity, analysis of the internal correlation between the encoded answers highlights four predominant fields of questions bearing different physical meanings: (1) “vibratory motions of small objects”, (2) “displacement and fall of objects”, (3) “acoustic noise” and (4) “personal feelings”. Best correlations between macroseismic and instrumental observations are obtained when the macroseismic parameters are averaged over 10-km-radius circles around each station. Macroseismic intensities predicted by published peak ground velocity (PGV)–intensity relationships agree with our observed intensities, contrary to those based on peak ground acceleration (PGA). Correlation between the macroseismic and instrumental data for intensities between II and V (EMS-98) is better for PGV than for PGA. Correlation with the response spectra exhibits clear frequency dependence for all macroseismic parameters. Horizontal and vertical components are significantly correlated with the macroseismic parameters between 1 and 10 Hz, a range corresponding to both natural frequencies of most buildings and high energy content in the seismic ground motion. Between 10 and 25 Hz, a clear lack of correlation between macroseismic and instrumental observations exists. It could be due to a combination of the decrease in the energy signal above 10 Hz, a high level of anthropogenic noise and an increase in variability in soil conditions. Above 25 Hz, the correlation coefficients between the acceleration response spectra and the macroseismic parameters are close to the PGA correlation level.
Similar content being viewed by others
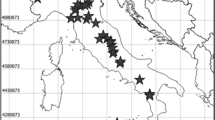
Avoid common mistakes on your manuscript.
1 Introduction
Numerous relationships have been established between macroseismic intensities and ground motion parameters. Before 1999, most of them were based on macroseismic intensities related to a city or a zip code area (e.g. Gutenberg and Richter 1956; Trifunac and Brady 1975; Murphy and O’Brien 1977; Wald et al. 1999a). Since the early 2000, intensities based on individual testimonies are more and more used, thanks to the numerous earthquake witnesses answering internet questionnaires. Wald et al. (1999a, b, c) promoted this new approach based on the internet questionnaire “Did you feel it?”. Similar procedures are now implemented in several countries (Musson and Henni 1999; De Rubeis et al. 2009; Atkinson and Wald 2007; Cecic and Musson 2004).
The way macroseismic intensities are commonly determined depends on the kind of data: collective intensities (Souriau 2006; Trifunac and Brady 1975; Wald et al. 1999a), tagged intensities (Boatwright et al. 2001) and intensities from individuals (Atkinson and Kaka 2007). It also depends on the data processing method: isoseismal interpolation (Souriau 2006; Boatwright et al. 2001; Dewey et al. 1995), averaging intensity over a zip code area (Wald et al. 1999a; Atkinson and Kaka 2006). In the present paper, we analyse a large set of macroseismic data collected by the Bureau Central Sismologique Français (BCSF)Footnote 1 and explore different processing methods. Instrumental data are taken from the French accelerometric network [Réseau Accélérometrique Permanent (RAP), Pequegnat et al. 2008].Footnote 2
One goal of this paper is to determine the most appropriate method for processing internet intensities and associating them with instrumental ground motion data. We also propose a more in-depth comparison between macroseismic observations and ground motion parameters by examining groups of answers in the macroseismic reports (named hereafter macroseismic parameters). The aim of this comparison is twofold: (1) check if macroseismic parameters are better correlated with instrumental ground motion parameters than intensity and (2) analyse the frequency range where the correlation is maximum.
In the following, we first analyse the content of the BCSF macroseismic reports and we review the way accelerometric records are processed to estimate the engineering parameters of the ground motion, namely, “peak ground acceleration” (PGA), “peak ground velocity” (PGV), “cumulative absolute velocity” (CAV), “arias intensity” (AI), “pseudo-spectral acceleration” (PSA) and “pseudo-spectral velocity” (PSV). In the second part of the paper, we test different ways of associating ground motion observations and macroseismic parameters and compute correlation coefficients for each of them.
2 Data analysis and pre-processing
Nine RAP stations located at distances <180 km from the epicentres recorded three earthquakes, which occurred in the northeast of France and southwest of Germany in 2003 and 2004 (Fig. 1): Rambervillers, 22/02/2003, M w = 4.8; Roulans 23/02/2004, M w = 4.4; and Waldkirch 05/12/2004, M w = 4.6. A large number of testimonies, either collective (i.e. by the city officers) or individual (i.e. by inhabitants), were collected by BCSF within this distance range (Table 1 and Fig. 1).
Map of the study area. Red stars show the epicentres of Rambervillers (22/02/2003) (top left), Roulans (23/02/2004) (top right) and Waldkirch (05/12/2004) (bottom left) earthquakes. Dots show the location of the individual testimonies for each earthquake, and their colours indicate the averaged values of Single Questionnaire Intensities (SQI) for each locality. The nine accelerometric stations are shown by red triangles with their associated 10-km-radius circles
2.1 Macroseismic parameters
Two types of observations, based on individual and collective macroseismic reports, are available in France. Collective reports are collected by BCSF and processed independently by both BCSF and the “Bureau de Recherches Géologiques et Minières” (BRGM) for Sisfrance.Footnote 3 EMS-98 intensities are given by BCSF (Grünthal 1998; Cara et al. 2005), while BRGM makes use of the MSK macroseismic scale (Medvedev and Sponheuer 1969). The collective reports filled in by the city officers provide a statistical description of the observed effects over the entire city. Individual reports are collected by BCSF since 2000 through an online questionnaire. They are filled in by individuals. When damage to buildings occurs, a field survey is conducted to complete the information collected on the website and by the city officers. An individual macroseismic report reflects what a single person feels or sees in his/her close environment. For intensities less than V, the only difference between the individual and collective questionnaires lies in the description of personal feelings. Otherwise, there is no difference for the most objective observations related to the effects on objects and furniture. The intensity value estimated for each individual report is hereafter referred to as a Single Questionnaire Intensity (SQI).
Because the three earthquakes investigated here have been broadly felt in Germany and Switzerland, it would have been worthwhile to extend our analysis of macroseismic reports to the German and Swiss data. Unfortunately, the questions asked in those reports concern more the statistical macroseismic effect observed in a district than the local effects (e.g. Cara et al. 2005). Moreover, at the time these earthquakes occurred, the German macroseismic questionnaire was not yet particularly designed for the use of EMS-98. These two factors prevented us from mixing French data based on a specific EMS-98 individual questionnaire form, with the German and Swiss data.
For assessing one SQI value per questionnaire form, answers to each question are first converted into an intensity value or to a range of most likely intensities according to the description of the EMS-98 degree. For example, SQI = III for a weak vibration or oscillation of an object and SQI ≥ V for a report on “fall of objects”. Inspection of all the SQI values belonging to a single macroseismic report then allows the analyst to issue his/her final SQI value by taking the median of the SQIs related to each filled-in question on the report. When the person reporting macroseismic effects is located on the third or fourth floor, the final SQI is reduced by one degree, according to the EMS-98 recommendations (Grünthal 1998). Only SQI fulfilling minimum quality criteria are eventually kept in the BCSF data basis. These SQI quality criteria are mainly based on the coherency of answers to the different questions. Furthermore, with the exception of intensity level II, SQI values are considered unreliable when based on the answer to one question only. Note that the answers related to a field of questions may cover overlapping intensity ranges. For example, questions related to personal feelings cover intensities between II and VIII, effects on objects cover the range [III–VII], effects on furniture cover the range [III–VIII] and effects on buildings are restricted to intensities larger than or equal to V according to the EMS-98 rules.
Individual questionnaires have slightly changed between 2003 and 2004. Thus, reports based on two versions of the individual questionnaire are analysed here (generations 7 and 9, hereafter named G7 and G9). Individual BCSF questionnaires include 50 questions covering effects felt by people, effects on objects, damages on structures and acoustic noises. The questions related to damages on structures are not considered here because they were negligible for the three earthquakes investigated in the present paper.
When a witness gives his/her postal address, an accurate localization of his/her report is made. If not, the testimony is located at the centre of the city (church or city hall). Unfortunately, most of the witnesses do not give their complete address. Only 31 % of the Rambervillers testimonies, 43 % for Roulans and 38 % for Waldkirch are accurately located. More precisely, for these three earthquakes, 4.8, 12.8 and 11.3 % of witnesses gave the street number of their house, while 26.3, 30.6 and 27 % of them, respectively, only indicate the street name.
To facilitate the analysis of the macroseismic reports, the 30 questions, which are relevant for evaluating intensities lower than V, have been grouped into a smaller number of question fields. Our first idea was to gather the questions into groups having similar physical meanings, such as the different kinds of vibration of small objects for example. In order to gather the questions in a more objective way than from an a priori hypothesis, we have computed the internal correlation coefficient between answers to the different questions after encoding them between 1 and −1. Binary answers such as “yes/no” were encoded “1/−1”. Ternary answers such as “strong/ medium/weak” were encoded “0.99/0.66/0.33”, respectively. When a question calls for more than three possible answers, the effects are regularly encoded between 0.99 and 0.33. “No answers” is encoded 0, and the SQI values [I–VI] are encoded 1–6.
Internal correlation between the encoded answers to a pair of question X and Y has been evaluated by computing the correlation matrix:
where X i and Y i are the answers of the witness “i”, and N XY is the number of testimonies related to the pair (X, Y). Questions not related to the ground motion, such as those related to the location of the witnesses for example, have been removed from the computation of the correlation matrix. In the end, 24 out of 30 questions were kept for computing the correlation coefficients R XY . Note that any linear transformation applied to the series of answers related to one question does not change the values of the correlation coefficients R XY .
Some items of the questionnaire can be put aside for several reasons. We deal with three moderate earthquakes, for which some questions are not relevant (see G7 items in Table 2): P14, rush out of the building; P15, lost balance; O8, opening and closing doors and windows; and O9, breakage of object and windows.
Moreover, two out of the three earthquakes occurred during day time making question P12-G7 (people awaken by the quake) inappropriate. Furthermore, some questions are related to visual observations such as presence of liquids (O6 and O7), but it is not obvious that people reporting were in presence of the observables. In such situations, they may answer “no” to the question and the negative answer (quoted −1) is anticorrelated with the positive answer (quoted >0) related to strong shaking.
Correlation coefficients R XY are similar for witnesses located on the ground floor, the first to second floor or the third to fourth floor. As these floor-specific coefficients do not show significant differences, we did not further correct the observations for floor-level influences. However, for reasons previously mentioned, the testimonies from witnesses located above the fourth floor where not taken into account in SQI computations.
The correlation matrix R XY is shown in Fig. 2 for the Rambervillers earthquake, which presents the largest number of individual reports. Very similar matrices are obtained for the two other earthquakes. Based on these correlation matrices, it is possible to identify groups of questions for which the answers are highly intercorrelated and are also well correlated with the SQI. The final grouping of questions was made when pairs of questions are both mutually correlated and related to some possible common source of shaking (e.g. effects on small and heavy objects are not in the same groups). We thus identify four groups of questions having distinct physical meanings and presenting high internal correlation coefficients between the reported answers (Fig. 2):
-
Q_VM: vibratory motions (0.7 < R < 0.9)
-
Q_D&F: displacement and fall of objects (0.8 < R < 0.9)
-
Q_N : acoustic noises (0.6 < R < 0.8)
-
Q_PF: personal feeling (0.7 < R < 0.85, correlation between P13 and questions of the other groups).
Internal correlation matrix for the 10,662 individual reports collected after the Rambervillers 2003 earthquake. Each square represents the correlation coefficient between the answers to a pair of questions (generation code G7). Three groups exhibit high internal correlation: Q_VM (vibratory motions), Q_D&F (displacement and fall of objects) and Q_N (acoustic noise). Answers to Q_PF (personal feeling) are correlated with the three groups. Note that the answers within the three groups and Q_N are all correlated with the Single Questionnaire Intensity SQI. The correlation matrices for the Waldkirch 2004 and Roulans 2004 earthquakes present very similar patterns
The fourth “group” (Q_PF), restricted to a single question, has been kept isolated due to its very different nature, dealing with the subjective manner a witness feels the earthquake. Note also that the answers to the questions belonging to these four groups present a high correlation with the SQI values, not surprisingly since macroseismic intensity is an overall figure of all the reported effects. Thereafter, these four question fields, together with SQI, are referred to as the five “macroseismic parameters”. The questions P1-G7 (Was the quake felt?) and P2-G7, (Did you personally feel it?) are also well correlated with these five groups, but they are not associated with a question field since they do not add any information on the ground shaking itself. Note furthermore that there are very few “no” answers to question P1, and P2 bears more information on the quality of the report than on the shaking itself.
2.2 Ground motion parameters
Records from the nine RAP stations located in the northeast of France are the basis of our instrumental observations. The Rambervillers and Roulans earthquakes are recorded by the nine stations, while only seven stations recorded the Waldkirch 2004 earthquake. In order to remove the low-frequency noise that could cause spurious signal in velocity estimates, the records were filtered with a two-order Butterworth high-pass filter with a 0.5 Hz cut-off frequency. An example of three-component RAP accelerograms is displayed in Fig. 3, together with the acceleration and velocity Fourier spectra. In this study, horizontal PGA, PGV, PSA, PSV, CAV, and AI are estimated on the basis of the Euclidian norm of the two horizontal components of the ground motion:
Example of instrumental three-component ground motion recording (Rambervillers earthquake at station STHE): a accelerometric records, b acceleration Fourier Spectra, c velocity spectra and d acceleration response spectra (damping factor 5 %). In d, the three-component response spectra are shown together with the Horizontal resultant (Euclidian Norm of the horizontal components)
Although CAV, CAVSTD and AI are mainly used by structural engineers to characterize the destructive potential of an earthquake, an attempt is made here to explore their correlation with macroseismic parameters for low levels of intensity. The following definitions of CAV (Campbell and Bozorgnia 2010) and AI, both in centimetres per second units, are computed from acceleration records a(t):
g being the standard acceleration of gravity.
3 Associating macroseismic and ground motion parameters
3.1 Single or statistically averaged macroseismic observations?
Associating macroseismic and instrumental observations at sites where instrumental and high-quality macroseismic data are both available is rarely possible. Indeed, the density of accelerometric stations is often much lower than the density of testimonies in a populated area, and the reverse may be true in less-inhabited areas. In order to address the sensitivity of macroseismic and instrumental parameter association, first we compared the closest collective and individual macroseismic observation within a distance <4 km from the accelerometric station. In order to get statistically more robust estimates of the macroseismic parameters, we tested a second approach that considered averages of the macroseismic parameters taken inside circular surfaces around each station.
In an ideal case, the first approach should only consider accelerometric stations and testimonies located on sites with identical soil conditions. Witnesses should also be located on the ground floor in order to minimize the possible effect of oscillation of the building. Unfortunately, such a situation is not frequent, and we had to extend our search of closest testimonies by relaxing some of the above conditions. In decreasing order of priorities, we kept the macroseismic reports: (1) located on the same geological units as the accelerometric station (compulsory); (2) as completely filled in as possible; (3) as close as possible to the station; (4) located on the ground floor, if not first to second floor, but cautiously excluding those located on the third to fourth floor even if the overall R XY are not floor dependent; and (5) located at the street address, and if not, at the city centre. A similar approach with collective intensities was followed by Wald et al. (1999a, b, c) who decided, for example, to compare instrumental parameters with the nearest intensity observation within 3 km of the station but without precise consideration of soil condition.
In our study, a simple classification of site conditions is used, based on a sediment/rock distinction and taking into account both Regnier et al. (2010) site conditions fixed according to the Eurocode 8 and the geological information from the BRGM 1:50,000 map (Table 3). Except for station STSM, reports are mostly located on the same soil as the nearby station; this makes the above condition (1) fulfilled for most sites.
In the second approach, we have considered circular surfaces that contain a statistically significant number of at least 15 testimonies. This guarantees a reasonably small standard deviation of the mean as discussed below. By visual inspection of histograms of the number of testimonies versus SQI in each circle (e.g. STMU and 10-km radius in Fig. 4) and, more quantitatively, by applying a chi-square test to the distribution, we conclude that a unimodal, Gaussian hypothesis is a reasonable representation of the distribution of SQIs. In order to be sure that the arithmetic mean is the best estimator, we have compared the arithmetic mean of the SQIs, quoted <SQI> hereafter, with their weighted mean, the mode, and the median. Comparing <SQI> and the weighted mean for the Rambervillers earthquake (Table 4), it appears that, when rounded to the half intensity unit, their values are very close, except for the median. We will furthermore see that the correlation coefficients involving <SQI> are smaller than those involving the median and present very little differences with the SQI weighted mean. Thus, we only present here the results for <SQI> as indicator of intensity within each circle.
An important choice in this second approach is the size of the circles surrounding each station. A radius of 10 km guarantees:
-
A minimum number of 15 macroseismic reports, with the exception of stations STSM for the Roulans earthquake (seven reports) and STFL for Waldkirch earthquake (one report).
-
A low (<0.2) standard deviation of the Mean SQI values:
$$ {\text{SDOM}} = \frac{\sigma }{{\sqrt {N} }} $$(5)where N is the number of SQI data in each circle, and σ is their standard deviation within the circle.
-
Independence of the data sets (for a radius > 10 km several circles overlap, making some reports attached to two or more stations).
The same 10-km radius circle was used for averaging the other four macroseismic parameters.
In order to know which of the two approaches is the most appropriate for associating macroseismic and ground motion parameters, we have computed linear regressions between the horizontal resultant of ground motion parameters (recorded at nine, nine and seven stations for Rambervillers, Roulans and Waldkirch earthquakes, respectively) and the values of the different averaged SQIs, as illustrated in Fig. 5 for the arithmetic means (<SQI>). Figure 6 displays the value of the correlation coefficient for: (1) the nearest SQI, (2) the nearest collective intensity, (3) the arithmetic mean (<SQI>), (4) the weighted mean and (5) the mode or the median. With a 23 degrees of freedom series, parameters are considered as significantly correlated when R = 0.5, and highly correlated when R ≥ 0.6 (e.g. Taylor 1997). Figure 6 shows that correlations are much better for the 10-km radius averaging approach than for the closest-report approach. Note also that for this latter approach the correlation coefficients are higher with the BCSF collective intensities based on some statistics relying on city officers than with the closest SQI based on single but closest macroseismic reports. Finally, correlations with the arithmetic means (<SQI>) are better than for the other averaging processes. This reinforces our choice for the arithmetic mean proposed above.
Illustration of the significance of the correlation between an instrumental parameter (PGV) and the averaged macroseismic parameter (<SQI>). The top grey curve represents <SQI> averaged within 10-km-radius circles around each accelerometric station for each of the three earthquakes. The bottom dark curve represents the horizontal resultant PGV at the centre of each circle. The correlation coefficient between both curves is R = 0.79 in this case
Correlation between the instrumental scalar parameters PGA, PGV, AI and CAV computed on the horizontal resultant, and the different types of SQI averages: arithmetic mean, weighted mean, mode, and median, taken within 10-km-radius circles centred on the accelerometric stations. The best correlation is obtained between PGV and the arithmetic average <SQI> (blue symbols). Black symbols represent correlations between the scalar instrumental parameters and the closest collective intensity. The SQI values closest to the station (orange symbols) and the median (purple symbols) are poorly correlated with all instrumental parameters
3.2 Correlations between the macroseismic and ground motion parameters
In order to determine which macroseismic parameter best represents the ground motion, correlation coefficients have been computed between, on the one hand, the scalar (PGA, PGV, CAV and AI) and frequency-dependent (PSA and PSV) instrumental parameters and, on the other hand, the five macroseismic parameters (SQI, Q_VM “vibratory motions of small objects”, Q_D&F “displacement and fall of objects”, Q_N “acoustic noises” and Q_PF “personal feelings”). Because low-intensity macroseismic effects are likely to mainly reflect the natural frequencies of the witnesses environment, the damped oscillator response spectra seems more appropriate than the Fourier spectra when looking at the frequency-dependence of macroseismic parameters. Correlations computed for PSA and PSV are similar since these two response spectra only differ by a multiplicative frequency factor common to all stations. For this reason, only correlations with PSA are displayed here.
As shown in Fig. 7a for the horizontal resultant, PGV clearly exhibits the highest correlation among the scalar parameters. With a value above R = 0.6 for the five macroseismic parameters, the largest correlation is obtained with the averaged macroseismic intensity <SQI> (R ~ 0.8). The correlation between PGA and <SQI> is smaller (R = 0.6); however, it is close to the correlation with “vibratory motion of small objects” (group Q_VM). The correlations for AI and CAV are very low (R < 0.3).
Correlation coefficient R between the instrumental parameters and the averaged macroseismic parameters within 10-km-radius circles around each station for the three earthquakes taken together. a Correlations with the horizontal resultant. b Correlations with the vertical component. The left part of the figures show the correlation between the macroseismic parameters and the pseudo-spectral acceleration (PSA) representing the maximum acceleration of oscillators with different eigenfrequencies and 5 % damping. The right part of the figures show the correlation with the scalar instrumental parameters as in Fig. 6. The red line R = 0.5 represents the threshold for a significant correlation
Looking at the variation of correlation with the acceleration response spectra in Fig. 7a, the most striking effect is a clear loss of correlation between 10 and 25 Hz, while at higher frequency, correlations increase again. They reach R = 0.5 for <SQI> and personal feelings, and they are near R = 0.6 for the “vibratory motions of small objects”, which is close to the PGA correlation value. At frequencies below 7 Hz, correlation is higher than 0.5 for all macroseismic parameters. However, a drop of correlation is observed at 1 Hz, particularly for <SQI>. At this frequency, the highest correlation is obtained for the “acoustic noise” (R = 0.7), the second highest correlation being obtained for the “displacement and fall of objects”.
The same type of correlation computation has also been performed for the horizontal signal component bearing the maximum PGA. The correlation coefficients are smaller than when horizontal resultants are used, but they present the same general pattern, except the slight drop of correlation below 1 Hz for <SQI>. The main difference between these two ways of looking at the horizontal motions lies in the high-frequency behaviour. Only <SQI> and the “vibratory motion of small objects” reach a correlation R = 0.5 above 25 Hz when working with the component bearing the maximum PGA, while there are four out of the five macroseismic parameters above R = 0.5 when using the horizontal resultant as in Fig. 7a.
For the vertical component (Fig. 7b), the highest correlations are found below 10 Hz and above 25 Hz, as for the horizontal case, but the loss of correlation between 10 and 25 Hz is less pronounced than for the horizontal resultant, except for the “acoustic noise” and the “displacement and fall of objects”. As for the horizontal case, PGV is the scalar parameter presenting the best correlation with all macroseismic parameters. The highest correlation with the scalar instrumental parameters on Fig. 7b is between the “vibratory motions of small objects” and PGV (R = 0.69). The second and third highest correlations are obtained for <SQI> (R = 0.64) and the “acoustic noise” (R = 0.61), respectively. The other scalar macroseismic parameters exhibit poor correlations with PGA, CAV and AI.
Concerning the frequency dependence of the correlations with the vertical component (Fig. 7b left), the drop of correlation for “vibratory motions of small objects” in the frequency band 10–25 Hz is less severe than for the horizontal resultant, and it reaches a value slightly above R = 0.6 at 31–33 Hz, a value close to the correlation with PGA. The drop of correlation is also less severe for the personal feelings and <SQI>. Note that <SQI> is well correlated with the response spectra between 3 and 9 Hz and beyond 30 Hz only. The other macroseismic parameters are correlated with the response spectra at 0.5 Hz and around 5 Hz. The frequency range where the macroseismic and ground motion parameters are correlated is thus narrower for the vertical than for the horizontal motion below 10 Hz.
3.3 Relationships between PGV and <SQI>
PGV and PGA are the most often used parameters in the relationships between macroseismic intensity and ground motion indicators. In the literature, the intensity-ground motion relationships are most of the time derived from the horizontal resultant (Atkinson and Kaka 2006). As we have seen from our data, the correlation coefficients are higher with the horizontal resultant than with the vertical component.
Several relationships can be extracted from the linear regression equations. Taking the pair of parameters (<SQI>, horizontal resultant PGV) having the highest correlation (R = 0.79; see Fig. 6), we propose the following linear equation relating BCSF < SQI > to PGV, valid for the east of France in the narrow intensity range [II–V], EMS-98:
The linear Eq. (6) is based on the BCSF SQI values, averaged over 10-km radius circle around each of the nine accelerometric stations shown in Fig. 1. It corresponds to distances ranging between 30 and 180 km from the epicentre.
4 Discussion
Relationships between macroseismic intensity and PGA or PGV published by different authors are compared with our averaged intensities <SQI> in Fig. 8 (Table 5). These relationships have been established either with magnitudes similar to those considered here (Atkinson and Kaka 2006; Atkinson and Kaka 2007; Kaka and Atkinson 2004) or are based on larger sets of magnitudes but include intensities between II and VI (Souriau 2006; Atkinson and Wald 2007; Wald et al. 1999c). To check if the RAP signals are similar to lower frequency accelerograms often used in published studies, we ran a stability test by applying a low-pass filter to the signals with a 25-Hz cut-off frequency. Figure 9 shows that the unfiltered and low-pass filtered PGA and PGV are both spread along the bisector. One can thus compare the PGA and PGV computed on the RAP signal with older values published in the literature.
Intensities predicted for PGA (a) and PGV (b) according to the intensity prediction equations displayed in (Table 5), compared to the averaged local Intensities <SQI> (red triangles). Vertical bars show the standard deviations of SQI within 10-km-radius circles around each accelerometric stations and the three earthquakes taken together. Note that focal distance is one of the parameters of the relationships of Souriau 2006 and Kaka and Atkinson 2004
Figure 8a shows that <SQI> are below most of the intensities predicted from PGA when using published intensity–PGA relationships, except that of Wald et al. (1999b). Furthermore, the slope of the intensity versus PGA trend is smaller in our case than for the other authors. This could be due to the differences in the data sets and data processing: Souriau (2006) analysed a larger set of observations composed of 20 earthquakes located over the whole French metropolitan territory; intensities used are either BCSF EMS-98 or SisFrance MSK collective intensities and isoseismal smoothing was applied to make the intensity data less erratic. As shown in Fig. 10, even if they are larger than our <SQI> and closer to the intensities predicted by the equation of Souriau (2006), SisFrance and BCSF collective intensities are still smaller than those predicted by Souriau (2006). Neither the PGA values predicted from intensities by different authors nor the slope of the published relationships are thus in agreement with our <SQI> versus PGA observations in the east of France.
Comparison between different kind of intensities (collective EMS-98 BCSF, MSK SisFrance, and <SQI>) and the intensities predicted from Souriau 2006’ relationship for the PGA determined at the accelerometric station and the three earthquakes investigated in this paper
Agreement is much better when comparing intensities with PGV (Fig. 8b). Indeed, equations for PGV of Atkinson and Kaka (2006), Atkinson and Wald (2007), Kaka and Atkinson (2004) and Wald et al. (1999b) fit quite well with our data within their standard deviations, in particular Atkinson and Wald (2007). This reinforces our conclusion made from inspection of correlations between the instrumental and macroseismic parameters that PGV is better connected to <SQI> than PGA is at the low-intensity level we are looking at. Note, however, that our <SQI> versus PGV slope is slightly smaller than the published ones.
Concerning the CAV and AI, published prediction equations poorly fit with our data confirming thus our conclusion made from the correlation analysis (Fig. 7).
Finally, concerning our rather small slope in the PGV–intensity plots, it is interesting to look at the compilation of relationships made by Cua et al. (2010) (Fig. 11). Despite a large variability of intensity values published in the literature, this figure clearly shows that there is a change in the trend of data around I = V in this intensity/LogPGV plot. At lower intensities, the slope is smaller than above intensity V. Our intensity values <SQI> related to nine circular areas of 10-km radius and limited to the range II–V are compatible with the apparent small slope in the figure of Cua et al. 2010. It is also compatible with the relationship of Atkinson and Wald 2007 reported in Fig. 11. The other important conclusion possibly drawn from Fig. 11 is that a linear intensity versus log PGV relationship fitting our data cannot be extrapolated to intensities larger than V and may be valid only at a low level of seismic shaking.
Intensities versus log(PGV) observations compiled by Cua et al. (2010) compared with our horizontal resultant PGV data (red triangle). The red line shows relationship of Atkinson and Wald 2007 presenting a low intensity versus log(PGV) trend, as our data, for intensity between II and IV (continuous line). The dotted line shows its extrapolation toward higher and lower values
Looking at the frequency dependence of the correlation between macroseismic parameters and the ground acceleration response spectra displayed in Fig. 7, it is important to note that 98.2 % of the witnesses reporting macroseismic effects were located inside buildings. Because acceleration response spectra reflect the building acceleration at different frequencies, the overall high correlations observed at frequencies <10 Hz on Fig. 7a and b are likely to simply reflect the natural frequencies of the buildings. Correlation of macroseismic intensity with response spectra has been noticed by several authors. Spence et al. (1992) revealed that higher correlations between MMI and ground motion parameters are obtained when the 5 % damping spectral acceleration (mean amplitudes in the short-period range of 0.1–0.3 s) is used instead of peak acceleration. Boatwright et al. (2001) showed that for the 1994 Northridge, California, earthquake correlations between the intensity and 5 % damped pseudovelocity–response spectra are strongest at 1.5 s and weakest at 0.2 s.
Indeed, a compilation of natural frequencies of buildings in Grenoble, France, shows for example that the fundamental mode of vibration of typical masonry buildings corresponds to frequencies lower than 8 Hz (Michel 2007). At frequencies lower than 1 Hz, it is also interesting to notice that the answers to the question field “displacement and fall of objects” present a rather high correlation with the horizontal motion, while it is lower than the “vibratory motions of small objects” at higher frequencies. The increase in correlation at high frequencies is particularly clear for the “vibratory motions of small objects”. This could be related to higher frequencies of vibration when small objects, like glasses and plates stored on shelves, are shaken. This could also be related to any effects induced by high frequency peaks of the ground acceleration on the witnesses’ environment, like a shock wave hitting the building.
The drastic loss of correlation for all macroseismic parameters between 10 and 25 Hz is more difficult to explain. The loss of correlation is larger for the horizontal motion (Fig 7.a) than for the vertical one (Fig. 7.b), in particular for the vibration of small objects that are a priori more sensitive to high frequency vibrations. The loss of correlation may be due to the combined effect of increased anthropogenic noise in an urban environment in this frequency range (Hunaidi and Tremblay 1997) and to the low level of energy in the ground motion above 10 Hz (e.g. Fig. 3b at STHE) making the “signal-to-noise” ratio too low at some stations to be coherently correlated with the macroseismic observations. A good site to explore this possibility is to look at the anthropogenic noise at STMU station at the centre of the city of Strasbourg, which presents a strong anthropogenic noise around 15 Hz. Applying a 10- to 20-Hz pass-band filter to the STMU records of the three earthquakes, we have estimated the signal/noise ratio on the filtered signal by comparing the amplitude before and after the first P-arrival. The signal/noise ratio varies from 100 for the Waldkirch earthquake, which occurred during the night, at 2:52 a.m. local time, when the anthropogenic noise was at its minimum, to a factor 2 in the worst case of the Roulans earthquake, which occurred at 6:31 p.m. local time, when the anthropogenic noise was high. The low signal/noise ratio at STMU only concerns the Roulans event, one out of the 25 data points in the computation of the correlation coefficients. It is thus very unlikely that such a strong loss of correlation on the horizontal resultant around 15 Hz could only be due to the strong increase of anthropogenic noise at the noisiest stations.
Another possibility for this loss of correlation between 10 and 25 Hz could be an increase in variability in soil conditions between the nearby accelerometric station, mostly located on sediments (Table 3), and the places where individuals are reporting the macroseismic effects. If so, this would mean that macroseismic effects typical of the frequency band of 10–25 Hz are not spatially coherent within the 10-km radius circles. The averaging process of the macroseismic parameters could then produce values not correlated with the response spectra computed for the accelerometric stations located at the centres of the circles. Note that a soil with low shear velocity V S and thickness h presents a resonance frequency f r = V S/4h, which is typically in the band of 10–25 Hz (e.g. for h = 5 m and V S = 300 m/s, f r = 15 Hz). Variability in the surficial soil layers might thus explain this loss of correlation. Further investigation with check of the soil conditions beneath accelerometic stations, however, would be necessary to check if the variability of soil conditions explains the observed loss of correlation.
Concerning the frequency behaviour of each macroseismic parameter, except for the slight difference of the “vibratory motions of small objects” as compared with the ”displacement and fall of objects”, none of them is representative of a single frequency range. The macroseismic intensity <SQI> follows the same overall frequency dependence as the four more objective macroseismic “questions field” parameters. This fact strengthens the idea that macroseismic intensity provides robust means of estimating some averaged PSA below 10 Hz, together with an averaged peak ground velocity PGV and, to a lesser extent, peak ground acceleration PGA, at least for the data set considered in this study.
The reasons why the “acoustic noise” parameter presents a frequency dependence quite similar to the other macroseismic parameters, <SQI> in particular, remains an open question. “Acoustic noise” should be heard only above 20 Hz, and the correlation with the response spectra should thus grow at high frequency. This is not the case since the correlation remains below R = 0.3 for both the horizontal and the vertical components above 30 Hz (Fig. 7). The maximum correlation is observed at low frequency, below 10 Hz, and its maximum R > 0.7 is observed with the horizontal components below 1 Hz. What is the origin of the noise? The only possibility we foresee is that non-linear processes convert the low-frequency seismic motion into audible noise in the local environment of the witnesses at the low macroseismic intensity level we are dealing with.
5 Conclusion
The relationship between macroseismic and ground motion parameters (scalar and spectral) is presented for three earthquakes of magnitude 4.4 ≤ M w ≤ 4.8 felt in the north-east of France. We have used an encoding scheme to analyse the BCSF individual reports. Internal correlations of each encoded question led us to define four macroseismic parameters: “vibratory motions of small objects”, “displacement and fall of objects”, “acoustic noises” and “personal feelings”. Not surprisingly, these macroseismic parameters are also well correlated with the intensity estimates SQI attached to each testimony.
Correlation between these five macroseismic and several ground motion parameters depends on the strategy used. Individual macroseismic parameters show a systematically stronger correlation with the ground motion parameters when they are averaged over circles centred at the accelerometric station than when only the closest testimony is considered. Smoothing of the macroseismic parameters by averaging, i.e. removing the subjectivity inherent to a single report, seems thus a good way to guarantee more robust values of the macroseismic parameters for comparison with ground motion parameters.
Concerning the scalar ground motion parameters, PGV is that presenting the highest correlation with the macroseismic parameters. Horizontal PGA is well correlated only with <SQI> and the “vibratory motion of small objects” but not with the other macroseismic parameters. The vertical PGA exhibits a significant correlation only with the “vibratory motions of small objects”. CAV and Arias intensity, commonly used by structural engineers are not pertinent indicators for such low levels of ground motion (intensity values used in this study range between II and V EMS-98).
Concerning the PSA determined from the response spectra computed at the accelerometric stations, the horizontal PSA shows better correlation with the macroseismic parameters than the vertical PSA. PSA exhibits a high correlation in particular for the intensity <SQI> (R = 0.7 for 0.5 Hz and between 1 and 10 Hz). The “acoustic noise” and “fall and displacement of objects” macroseismic parameter also shows a high correlation in this low frequency range. A likely explanation for these high correlations may be that this frequency range corresponds to typical natural frequencies of buildings, which could amplify the seismic ground motion. Around 15 Hz, a loss of correlation is observed for all the macroseismic parameters. It is more pronounced for the horizontal PSA than for the vertical one. Absence of correlation around 15 Hz remains an open question. It could be due to a combination of the decrease in the energy signal above 10 Hz, a high level of anthropogenic noise and an increase in variability in soil conditions. Above 25 Hz, the correlation of PSA with the “vibratory motion of small object” and the intensity <SQI> rises and reaches a level close to the correlation with PGA.
References
Atkinson GM, Kaka SI (2006) Relationships between felt intensity and instrumental ground motion for New Madrid ShakeMaps. Technical Report, Department of Sciences, Carleton University, Ottawa, Canada K1S5B6
Atkinson GM, Kaka SI (2007) Relationships between felt intensity and instrumental ground motion in the central United States and California. Bull Seismol Soc Am 90(2):497–510
Atkinson GM, Wald DJ (2007) "Did you feel it?" intensity data: a surprisingly good measure of earthquake ground motion. Seismol Res Lett 78(3):362–368
Baer M, Deichmann N, Braunmiller J, Husen S, Fäh D, Giardini D, Kästli P, Kradolfer U, Wiemer S (2005) Earthquakes in Switzerland and surrounding regions during 2004. Eclogae Geol Helv 98:407–418
Boatwright J, Thywissen K, Seekins LC (2001) Correlation of ground motion and intensity for the 17 January 1994 Northridge, California, earthquake. Bull Seismol Soc Am 91(4):739–752
Brenn N, Stange S, Brüstle W, Henk A, Stribrny B (2006) Das Beben von Waldkirch am 5.12.2004, poster, Albert Ludwigs Universität, Freiburg, Germany
Campbell K, Bozorgnia Y (2010) Analysis of cumulative absolute velocity (CAV) and JMA instrumental seismic intensity (IJMA) using the PEER-NGA strong motion database, PEER report 2010/102 Pacific Earthquake Engineering Research Center, College of Engineering, University of California, Berkeley
Cara M, Brüstle W, Gisler M, Kästl P, Sira C, Weihermüller C, Lambert J (2005) Transfrontier macroseismic observations of the Ml = 5.4 earthquake of February 22, 2003 at Rambervillers, France. J Seismol 9:317–328
Cara M, Schlupp A, Sira C (2007) Observations sismologiques: Sismicité de la France en 2003, 2004, 2005, Bureau Central Sismologique Français. ULP/EOST-CNRS/INSU, Strasbourg, p 199
Cecic I, Musson R (2004) Macroseismic surveys in theory and practice. Nat Hazards 31(1):39–61
Cua G, Wald D J, Allen TI, Garcia D, Worden CB, Gerstenberger M, Lin K, Marano K (2010) “Best practices” for using macroseismic intensity and ground motion-intensity. GEM Technical Report 2010-4
Delouis B, Charlet J, Vallee M (2009) A method for rapid determination of moment magnitude Mw for moderate to large earthquakes from the near-field spectra of strong-motion records (MWSYNTH). Bull Seismol Soc Am 99:1827–1840
De Rubeis V, Sbarra P, Sorrentino D, Tosi P (2009) Web based macroseismic survey: Fast information exchange and elaboration of seismic intensity effects in Italy. In: Proceedings of the 6th International ISCRAM Conference, Gothenburg, Sweden
Dewey JW, Glen Reagor B, Dengler L, Moley K (1995) Intensity distribution and isoseismal maps for the Northridge, California earthquake of January 17, 1994. Open-File report 95-92, US Geological Survey, p 5
Grünthal G (eds) (1998) European Macroseismic Scale 1998 EMS-98. Cahiers du Centre Européen de Géodynamique et de Séismologie vol.15, Luxembourg, 99 pp
Gutenberg B, Richter CF (1956) Earthquake magnitude, intensity, energy and acceleration. Bull Seismol Soc Am 46:105–145
Hanned A (2007) La séquence de répliques du séisme de Rambervillers du 22 Février 2003. Master thesis, Ecole et Observatoire des Sciences de la Terre de Strasbourg, 28 pp
Hunaidi O, Tremblay N (1997) Traffic-induced building vibration in Montréal. Can J Civ Eng 24:736–753
Kaka SI, Atkinson GM (2004) Relationships between instrumental ground-motion parameters and modified Mercalli intensity in eastern North America. Bull Seismol Soc Am 94(5):1728–1736
Lesueur C (2011) Relations entre les mesures de mouvements du sol et les observations macrosismiques en France : Etude basée sur les données accélérométriques du RAP et les données macrosismiques du BCSF, Ph-D thesis, Université de Strasbourg, 161 pp
Medvedev SV, Sponheuer W (1969) Scale of seismic intensity. In: Proc. World Conf. Earthquake Engr. A-2, 4th, Santiago, Chile, pp 143–153
Michel C (2007) Vulnérabilité Sismique de l’échelle du bâtiment à celle de la ville. Apport des techniques expérimentales in situ. Application à Grenoble. PhD Thesis, Université Joseph Fourier - Grenoble I, 211 pp.
Murphy JR, O’Brien LJ (1977) The correlation of peak ground acceleration amplitude with seismic intensity and other physical parameters. Bull Seismol Soc Am 67(3):877–915
Musson RMW, Henni PHO (1999) From questionnaires to intensities—assessing free-form macroseismic data in the UK. Phys Chem Earth Pt A 24(6):511–515
Péquegnat C, Guéguen P, Hatzfeld D, Langlais M (2008) The French Accelerometric Network (RAP) and National Data Centre (RAP-NDC). Seismol Res Lett 79(1):79–89
Regnier J, Laurendau A, Duval AM, Gueguen P (2010) From heterogeneous set of soil data to VS profile—application on the French permanent accelerometric network (RAP) sites. In: Proceeding 14EECE Ohrid, Macedonia Republic, August 30th–September 3rd 2010
Souriau A (2006) Quantifying felt events: a joint analysis of intensities, accelerations and dominant frequencies. J Seismol 10(1):23–38
Spence R, Coburn A, Pomonis A (1992) Correlation of ground motion with building damage: The definition of a new damage-based seismic intensity scale. In: Proceedings of Tenth World Conf. on Earthq. Eng., Madrid, vol. 1, pp 551–556
Taylor JR (1997) Error analysis: the study of uncertainties in physical, 2nd edn. University Science Book, Sausalito, p 327
Trifunac MD, Brady AG (1975) On the correlation of seismic intensity scales with the peaks of recorded strong ground motion. Bull Seismol Soc Am 65(1):139–162
Wald DJ, Quitoriano V, Heaton TH, Kanamori H, Scrivner CW, Worden CB (1999a) TriNet "Shakemaps": rapid generation of peak ground motion and intensity maps for earthquakes in Southern California. Earthquake Spectra 15(3):537–555
Wald DJ, Quitoriano V, Heaton TH, Kanamori H (1999b) Relationships between peak ground acceleration, peak ground velocity, and modified mercalli intensity in California. Earthquake Spectra 15(3):557–564
Wald DJ, Quitoriano V, Dengler LA, Dewey JW (1999c) Utilization of the internet for rapid community intensity maps. Seismol Res Lett 70(6):680–697
Acknowledgement
We are thankful to Fabian Bonilla, from IRSN-IFSTTAR, who helped us with the instrumental data processing. Maria Lancieri, from IRSN, provided invaluable assistance in clarifying the statistical concepts applied to macroseismic data. Two anonymous reviewers greatly helped us improve a former version of the manuscript. Within the framework of the RAP consortium, Chloé Lesueur benefited from a grant of the French Ministry in charge of Environment and Natural Hazards (MEDDTL) and Institut de Radioprotection et de Sureté Nucléaire (IRSN).
Author information
Authors and Affiliations
Corresponding author
Rights and permissions
About this article
Cite this article
Lesueur, C., Cara, M., Scotti, O. et al. Linking ground motion measurements and macroseismic observations in France: a case study based on accelerometric and macroseismic databases. J Seismol 17, 313–333 (2013). https://doi.org/10.1007/s10950-012-9319-2
Received:
Accepted:
Published:
Issue Date:
DOI: https://doi.org/10.1007/s10950-012-9319-2