Abstract
The present study compared the infant’s tendency in the first year of life to produce clusters of particular vocal types (squeals, vocants, and growls) in typically developing (TD) and autistic infants. Vocal clustering provides evidence of vocal category formation and may establish a foundation for speech development. Specifically, we compared the extent of vocal clustering across outcome groups and age groups. We also examined the associations between the extent of vocal clustering and later outcomes at 2 years within the autistic group. Randomly selected 5-min segments (27,153 5-min segments total) from 1293 all-day home recordings from 103 TD infants and 44 autistic infants across the first year were humancoded (about 9.75 h of data coded per infant on average) to derive vocal clustering patterns. Fisher’s exact tests were used to compare the occurrence of squeals versus vocants, as well as growls versus vocants, across coded segments. Infants in both groups demonstrated clear clustering patterns of squeals and growls across all age groups. The extent of vocal clustering in the autistic group did not correlate significantly with later language, repetitive behavior, or autism severity outcomes. These findings highlight the robustness of the systematic production of vocal categories across the first year of life. The similarity of the clustering patterns in the TD and autistic groups suggests that vocal category formation through active infant vocal exploration is a robust feature of early speech development.
Similar content being viewed by others
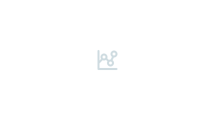
Avoid common mistakes on your manuscript.
Introduction
Research on precursors to speech has revealed vocal capabilities and inclinations within the first year of human life not seen in any other primate at any age (Griebel et al., 2016; Griebel & Oller, 2014; Oller et al., 2019b). From the first months of life, the human infant produces prelinguistic vocalizations (termed “protophones”) that lay foundations for speech, even though they do not yet constitute speech. Infants go through stages of protophone development before they utter their first word: 1) phonation without systematic supraglottal articulation, yielding primarily vowel-like sounds or “vocants” (0–2 months); 2) primitive articulation or “gooing” where vocants are combined with tongue movements that often involve contact with structures in the back of the mouth (1–4 months); 3) vocal play or expansion, where playful vocalization produces a variety of sounds such as squealing, growling, and raspberries (3–8 months); and 4) canonical babbling with well-formed syllables, such as [ba], [da], or [na] beginning as early as 5 months but not later than 10 months in typical development (Kent, 2022; Stark, 1980).
Recent work involving home day-long recordings of both typically developing (TD) and prematurely born infants collected using Language Environment Analysis (LENA) recorders has revealed that infants produce protophones far more often than previously expected, ranging from four to five per waking minute in the first months after full-term birth and continuing throughout the first year (Oller et al., 2019a, 2019b). Oller et al. (2019a) reported that even the prematurely born infants still in neonatal intensive care began protophone production at high rates soon after extubation, which allowed them to breathe on their own. As many as 90% of protophones are spontaneously produced, not directed to or elicited by any caregiver (Long et al., 2020; Oller et al., 2021). Babies seem to be building a vocal capability actively, not merely responding to caregiver elicitation, but rather exploring vocalizations and finding ways to incorporate new sounds into a repertoire, a pattern reminiscent of the proposed agent-like activity of infants and children as proposed in “construction grammar” (Croft, 2001; Elbers, 1997; Tomasello, 2003).
The present paper focuses on vocal play, an understudied yet potentially crucial phenomenon, defined as a vocal pattern in which infants engage in bouts of practice-like protophone production (Stark, 1980, 1981). Vocal play is often focused on a small number of recognizable vocal categories that manifest different phonatory patterns: vocants (or vowel-like sounds, usually with normal or “modal” phonation), squeals (with high pitch and often falsetto or “loft” phonation), and growls (often with low pitch and fry or harsh phonation) (Buder et al., 2013, 2018). Anecdotally, parents often report that vocal play across these categories does not appear to be randomly sequenced but rather tends to occur in clusters of particular types (Oller, 2000). For example, an infant may produce vocants (the most common phonatory category) for a period of several minutes and then abruptly produce several growls within a similar time period, returning a few minutes later to producing vocants, or switching to squeals.
The pattern of non-random occurrence of these protophone types in vocal play suggests endogenously generated practice, unlike any vocal pattern that has ever been reported to occur in our ape relatives. Infant protophone production appears to constitute endogenous development of categories. While they do not constitute speech sounds, protophones reveal an ability that all speech requires, namely the ability to create vocal categories that are not in the human innate repertoire of vocal signals, such as crying, shrieking, or laughing (Oller et al., 2016).
Prior Research on Vocal Play in Typical Development
The notion of vocal play in human infants began to attain modern currency with longitudinal research by Stark (Stark, 1981; Stark et al., 1975). She suggested that infants from 3–5 months of age tend to engage in long bouts of vocal activity and coined the term “vocal play” to refer to it. Other researchers conducting longitudinal research on vocal development also observed that infants produced playful, repetitive protophones and that parents confirmed vocal play occurred at home (Laufer & Horii, 1977; Oller, 1978, 1980). Infants appeared to expand and consolidate their repertoires of vocal types during the vocal play stage, practicing the most salient sounds, namely vocants, squeals, and growls. Notably, there was very little quantification of the rate of occurrence or the pattern of repetition or clustering in infant production of the vocal types that were recognized by the investigators. Instead, research tended to focus on acoustic and articulatory descriptions of infant vocalizations (Bauer & Kent, 1987; Holmgren et al., 1986; Kent, 1981; Robb & Saxman, 1988), the phonetic and acoustic definition of canonical babbling (Oller, 1980, 1981, 1986), and parent-infant vocal interaction (Beebe et al., 1988; Bloom, 1988; Kaye & Fogel, 1980; Stern et al., 1975).
More recent research on this topic has quantified that small samples of infants have evidenced the production of particular vocal types in concentrated periods or in non-random repetitions of individual vocal types (Oller et al., 2007; also see Supporting Information in Oller, 2013). By far the most extensive empirical study of vocal play clustering was reported in a recent study, where 130 TD infants were tracked longitudinally with all-day recordings across their first year of life (Yoo et al., in press). Human coding of randomly selected five-minute segments from home recordings showed that TD infants demonstrated a strong tendency to engage in non-random clustering of squeals and growls with regard to the most frequently occurring category of protophones, the vocants (Yoo et al., in press). Specifically, more than 60% of TD infants in this study showed significant clustering patterns of squeals or growls on average across the first year, suggesting that TD infants engage in systematic production of the three vocal categories from their first months.
Early Vocal Production in Autism
The study of early vocal production in both TD and clinical populations may supply an empirical basis for recognizing clinically meaningful differences. Infant vocalizations have been widely studied in infant siblings of autistic childrenFootnote 1 given that differences in vocal development may be leveraged as early emerging behavioral markers that could facilitate early detection of autism. However, within this body of literature, more attention has been focused on the frequency or rate of vocalizations (volubility) than on how vocalizations of different types are sequenced or distributed when infants produce them. Findings have been mixed with regard to whether infants with elevated likelihood for autism (EL) show different volubility than infants with typical likelihood for autism (TL). No difference was reported in the frequency of vocalization during a five-minute free play between EL and TL infants (Northrup & Iverson, 2015) or in percentage of time engaged in vocalizations in videotaped first birthday parties in autistic infants compared to TD infants (Osterling et al., 2002). Other studies have reported fewer or lower rates of speech-like vocalizationsFootnote 2 in EL infants compared to TL infants (Chenausky et al., 2017; Patten et al., 2014; Paul et al., 2011; Warlaumont et al., 2014). Swanson et al. (2018) used automated processing developed by the LENA Foundation to identify infant vocalizations and found a greater number of vocalizations in EL infants compared to TL infants, driven by a subgroup of “hypervocal” infants. A related finding from Plate et al. (2021) revealed that the majority of the “hypervocal” EL infants from the Swanson et al. (2018) paper did not develop autism at 24 months, and no significant difference was found in overall vocalization rate between EL infants with a confirmed diagnosis of autism and TD infants at 6, 12, or 24 months. Most studies on this topic have involved manual coding of vocalizations from brief (< 30-min samples) audio or video recordings (Chenausky et al., 2017; Northrup & Iverson, 2015; Patten et al., 2014; Paul et al., 2011; Plate et al., 2021) or automated detection of vocalizations from day-long recordings (Swanson et al., 2018; Warlaumont et al., 2014).
To our knowledge, no study has examined patterns of infant vocal play as we define it here in autistic infants using either laboratory or naturalistic day-long home recordings. Theoretically, given the empirical evidence of clustering in TD infants (Yoo et al., in press), a finding that autistic infants also show a similar pattern of non-random occurrence of protophone types would hint at robustness of self-organization in vocal category development (Oller et al., 2016) and would highlight infants’ endogenous inclinations to engage in vocal exploration. Clinically, describing the pattern of vocal practice in autistic children may help us understand how autism interacts with early vocal development and could open doors to identifying additional early vocal markers of autism.
The Present Study
The present study represents the first effort to compare patterns of vocal clustering in TD and autistic infants. Our study addresses the first year of life using human coding of randomly sampled segments from day-long recordings. The study has the following objectives. First, we test the extent to which infants tended to produce vocal categories (squeals, vocants, and growls) in clusters rather than randomly distributing them across day-long recordings, focusing on possible differences in the extent of clustering across the TD and autistic groups. Second, we report developmental patterns of vocal clustering across six age categories in the first year in both groups. Third, we explore the extent to which clustering in vocal play correlated with later language outcomes and/or clinical features of autism from diagnostic evaluations at 2 years. The relationship between the extent of vocal clustering and later outcomes may unfold in two ways. On the one hand, evidence of vocal clustering in autistic infants may be positively correlated with later language outcomes, suggesting that vocal practice may support language development generally. On the other hand, given that restrictive and repetitive patterns of behaviors (RRBs) are a core feature of autism (American Psychiatric Association, 2013), a high degree of vocal clustering in early infancy may reflect a high degree of repetitive vocalization, be a marker for early repetitive behaviors and stereotypies, and correlate with the extent of RRBs at 2 years.
Methods
The institutional review boards of Emory University (Emory IRB00059383 and IRB00097674) and the University of Memphis (IRB #2143) approved the procedures used in this study. All families provided written consent prior to their participation in the longitudinal project from which the data in the present study were derived.
Participants
A total of 147 infants (103 TD infants and 44 autistic infants) were selected based on confirmed outcomes at 3 years from a larger database of > 300 infants who participated in a longitudinal sibling study of speech and language development across the first three years of life at the Marcus Autism Center (MAC) in Atlanta, Georgia. Newborn infants were recruited as having either elevated likelihood (EL) for autism (having at least one older biological sibling with a confirmed autism diagnosis) or typical likelihood (TL) for autism (no familial history of autism in 1st, 2nd, or 3rd degree relatives). Exclusion criteria included birth complications (e.g., born preterm < 34 weeks, prenatal or perinatal complications), evidence of a disorder influencing speech perception or production (e.g., hearing loss, cleft palate), genetic conditions including those associated with autism (e.g., Fragile X syndrome, tuberous sclerosis), and other medical conditions such as non-febrile seizure disorders or conditions requiring tube feeding or ventilation.
Recruitment was conducted blind to sex or socioeconomic status (SES). Home language recordings from infants were selected for human coding to balance sex and SES distributions to the extent possible given male-to-female ratios of autism and the demographics of the Atlanta metropolitan area. The proportion of male vs. female participants was not statistically significant between groups (χ2 = 1.42, p = 0.23). However, a significant difference was detected for SES backgrounds between groups: the TD group included more infants from high SES backgrounds than the autistic group (χ2 = 21.71, p < 0.001). Thus, we conducted between-group analyses in stratified groups of SES to evaluate the potential effect of SES on vocal clustering patterns. No significant differences emerged between the outcome groups in either the low-SES or high-SES groups. The pattern in both SES groups mirrored the overall results (See Supplementary Material S3 for details). Demographic information for all participants is presented in Table 1.
All infants received a full diagnostic characterization at 2 and 3 years of age, including administration of the Autism Diagnostic Observation Schedule, 2nd Edition (ADOS-2; Lord et al., 2012) and assessment of cognitive and language outcomes using the Mullen Scales of Early Learning (MSEL; Mullen, 1995). At each assessment, diagnostic impressions were assigned by two different senior-level clinicians, blind to prior autism likelihood status and diagnoses. Confirmatory diagnoses were reached through consensus using clinicians’ diagnostic impressions and ADOS-2 diagnostic classifications. Only infants who were initially recruited as TL and later confirmed as having no clinical features were included in the TD group for this study. All infants later diagnosed with autism regardless of autism likelihood status were included in the autistic group. Language, cognitive, and autism severity characteristics for all participants are presented in Table 2.
Day-Long Recording Procedures
Caregivers completed day-long recordings approximately once a month between approximately 0 to 36 months. The present study involved human coding of recording data between 0 and 13 months. Audio recordings were completed using LENA recorders. On average, families of TD infants completed 9.1 recordings (range: 4–13) across the ages studied and families of autistic infants completed 8.1 recordings (range: 3–12), with an average recording time of approximately 11 hours per day.
Data included 1293 all-day recordings (357 for autistic infants, 936 for TD infants). Human coding on 21 randomly selected five-minute segments from each recording yielded 27,153 segments for data analysis. For age-related analyses, recording age was rounded and data were grouped as follows: 0–2 months, 3–4 months, 5–6 months, 7–8 months, 9–10 months, and 11–13 months, with cutoffs at 2.5, 4.5, 6.5, 8.5, and 10.5 months respectively.
Coding Procedures and Categories
Thirty-six English-speaking graduate students in the University of Memphis Origin of Language Laboratories (OLL) were trained for human coding of infant vocalizations. Coding was conducted using Action Analysis, Coding, and Training (AACT, Delgado et al., 2010), which allows the use of keystroke and mouse-selected coding for vocal categories in real time. Coders were blind to diagnostic and demographic information. Coding was conducted at the utterance level based on a “breath-group criterion” in accord with which phonation begins an utterance and inhalation ends it (Lynch et al., 1995). Vegetative sounds (e.g., coughing, sneezing, burping, and effort grunts) were not coded. The three primary protophones (vocants, squeals, and growls) accounted for approximately ~ 85% of all utterances across the first year and are the focus of the analyses for this study. Cries, whimpers, and laughs (about 13% of coded utterances) were excluded from analysis.
In the present study, the clustering of squeals and growls is referenced to vocants, the apparent default category for protophones, since they constituted about 75% of utterances, while growls were about 7% and squeals about 5%. The real-time method may limit coding of the non-default phonatory categories (squeal and growl) to particularly salient instances of squeal and growl, a limitation that we think corresponds to perceptions of parents, who (like real-time coders) have only one opportunity to judge each infant utterance.
Detailed definitions for squeals, vocants, and growls are provided in the Supplementary Material S1. The three categories are often easy to recognize as sharply distinct, but many infant utterances involve considerable ambiguity; they may shift from one vocal regime to another within utterance, presumably because infants are exploring vocalization. Thus, coders were required to make a forced choice among the three categories in real time based on the most auditorily salient feature of the entire vocalization. The key point here is that ambiguity is an inherent aspect of the judgments of protophones because they are exploratory and often yield fuzzy or uncertain impressions, an important issue to consider in assessment of coder agreement, which we address below.
Analyses of Clustering Patterns
We conducted Fisher’s exact tests (Fisher, 1934; Freeman & Halton, 1951) to evaluate the null hypothesis that the proportion of squeals with respect to vocants was the same, or growls with respect to vocants was the same, across the five-minute segments in each recording that were included for analysis, at the 95% confidence level. Fisher’s exact test is an appropriate test of independence in the analysis of contingency tables. It is robust with regard to small or uneven n’s (as often occurred in individual five-minute segments in our data) and is consequently particularly suitable compared to other non-parametric tests of independence of categorical variables, such as chi-square. In Supplementary Material S2 we tabulate raw data from example recordings to help readers understand the Fisher’s exact test results intuitively.
Segments with high rates of crying or whimpering and segments where the infant was asleep were deemed incompatible with vocal play. Consequently, we excluded segments in which 1) the infant was judged by the coder to be asleep throughout the five minutes (as indicated in a short questionnaire completed after coding each segment; n = 7235 segments), and 2) segments where ≥ 5 cry or whimper utterances occurred (n = 2795 segments). After applying the exclusion criteria, we analyzed the remaining total of 17,137 segments. The proportion of excluded segments due to cry and sleep did not differ significantly between the TD and autistic groups.
Fisher’s exact tests were conducted on all available recordings (n = 1293 recordings), one for squeals vs. vocants and again for growls vs. vocants. A significant Fisher’s exact test for the available comparisons within a recording would signify that the protophone types were distributed in a non-random way, indicating clustering. In other words, either squeals or growls or both were being produced significantly more often in particular segments than in other segments of the same recording. Prior to the analyses, we evaluated all recordings for the possibility that there were no squeals or growls throughout the recording. If a recording did not contain any coded squeals, it was designated as “not analyzable” by the Fisher’s exact test for the squeals vs vocants comparison. Likewise, the growls vs vocants comparison was not feasible if a recording did not contain any coded growls. There were 141 recordings with no coded squeals, 140 with no coded growls, and 47 with neither squeals nor growls. It is important to note that the recordings that did not contain squeals or growls were not excluded from the final data but rather were included in the denominator when calculating the percentage of recordings that showed significant clustering patterns of squeals or growls with respect to vocants.
To answer the main research question regarding the extent of clustering of squeals and growls with respect to vocants in autistic infants as compared to TD infants, the proportion of recordings that showed significant clustering patterns of squeals and growls (with all available recordings represented in the denominator) was first calculated for each infant and then summarized for the TD and the autistic groups. We also calculated the proportion of recordings that showed significant clustering patterns of either squeals or growls, the numerator being the number of recordings where the Fisher’s exact test for either squeals vs vocants or growls vs vocants was significant. Given that the distribution of proportion data was not normal, we report group means and bootstrapped 95% confidence intervals calculated across 5000 resampling iterations. Between-group differences were examined using permutation testing with 5000 resamples. To examine the developmental trend of the extent of clustering of squeals and growls, data were averaged for all the recordings of each infant within each age interval prior to computing means and bootstrapped confidence intervals for each age interval and group.
Finally, correlation analyses were conducted in the autistic group using the proportion of recordings that showed significant clustering patterns of squeals, growls, or either squeal or growls, and outcome measures at 2 years. The outcome measures include (1) ADOS Overall Total scores, (2) ADOS Social Affect scores, (3) ADOS Restricted and Repetitive Behavior (RRB) scores, (4) Mullen Scales of Early Learning (MSEL; Mullen, 1995) Receptive Language t-scores, and (5) MSEL Expressive Language t-scores.
Intercoder Agreement
We examined coder agreement across 289 five-minute segments, including 21 segments randomly selected from nine agreement recordings of both TD and autistic infants. The agreement recordings came from the same longitudinal studies but were not included in the actual data reported under Results. All 21 segments of some recordings were coded independently by the 36 coders who provided data for the results reported below. Additional coders who had been trained the same way also coded all 21 segments of some or all of the nine recordings. The number of individuals who coded each of the 9 recordings varied from 20–48 (mean = 32.1 coders per recording).
As expected, coders varied in their counts of the three protophone types, indicating predictable disagreement among coders about categorizations of squeals, growls, and vocants. The coefficient of variation (CoV, ratio of standard deviation to mean) across coders provides a measure of the degree of coder variation. The mean CoV across the nine recordings for vocants was 0.21, while squeals and growls showed notably more variation (CoV = 0.59 and 0.96 respectively). The data thus show substantial differences among coders in their judgments about vocants, squeals, and growls. Acoustically and auditorily analyzed examples offer perspective on why such disagreement is to be expected, namely that many utterances of infants involve a mixture of the phonatory regimes that characterize the three protophone types. These mixtures of regimes put forced-choice coders in the position of having to decide which among the phonatory regimes within any utterance is most salient, and often there is no absolute correct answer.
We conducted a permutation test (Good, 2005) on all of the nine agreement recordings to determine whether coder variation had any significant effect on the dependent variables of interest (percentage of recordings that showed significantly clustering pattern of squeals, growls, or either squeals or growls). We resampled the 48 coders without replacement 5000 times and calculated the proportion of recordings that showed significant clustering patterns of squeals or growls with respect to vocants to determine the empirical distribution of our dependent variables under the null hypothesis that coder identity had no effect. The test indicated that the null hypothesis could not be rejected at the 95% confidence interval, and there was therefore no evidence that coder differences significantly skewed the results. Detailed results and elaboration of the permutation tests are provided in Supplementary Material S4.
Results
Clustering of Protophones in TD and Autistic Groups
Results indicated a considerable tendency for infants in both outcome groups to show significant clustering patterns. For squeals, 39% of recordings from TD infants showed significant clustering with respect to vocants (95% CI = [0.36, 0.44]), and 46% of recordings from autistic infants showed significant clustering (95% CI = [0.40, 0.53]). The percentage of recordings that showed significant clustering did not differ significantly across the TD and autistic groups (p = 0.14). With respect to growls, 39% of recordings from TD infants showed significant clustering (95% CI = [0.34, 0.44]) and 43% of recordings from autistic infants showed significant clustering (95% CI = [0.35,51]. Again, no significant difference was found in the percentage of recordings that showed significant clustering of growls across the TD and autistic groups (p = 0.41).
When calculating the percentages, the number of excluded recordings that were classified as non-analyzable based on the absence of coded squeals or growls was included in the denominators. Consequently, the percentages reflect clustering of squeals and growls across all recordings available for all the infants. Since only 21 segments (105 min) were selected from each all-day recording for coding (about 1/6 of the available recording time), these values necessarily underestimate the percentage of days the infants actually engaged in some clustering of squeals and/or growls. The data thus supply a conservative estimate of the amount of clustering of squeals and growls.
Evaluation of whether individual infants showed either significant clustering of squeals or growls in each recording revealed that 66% of recordings from autistic infants and 61% recordings from TD infants showed a significant result for one or the other. Thus, the findings suggest infants usually showed some discernible clustering patterns for protophones.
Clustering Patterns of Protophones Across Ages
The data by age for each vocal type comparison showed clear evidence of clustering of squeals and growls with respect to vocants across all age groups in both the TD and autistic groups. Figure 1 provides a summary of the developmental patterns, indicating that clustering of squeals and growls occurred from the first months of life and at all the age intervals. For TD infants, the percentage of recordings that showed significant evidence of clustering ranged from 26 to 49% for squeals and from 33 to 43% for growls. For autistic infants, the percentage of recordings that showed significant evidence of clustering of squeals was slightly higher (although not statistically significantly) than for TD infants, ranging from 37 to 57%. The percentage of recordings that showed significant evidence of clustering of growls ranged from 33 to 43% for autistic infants.
Developmental Patterns of Vocal Clustering across Six Age Intervals in the First Year. a Values were averaged for all the recordings of each infant within each age interval prior to computing means. b Error bars represent bootstrapped 95% confidence intervals calculated across 5000 resampling iterations
Interestingly, for both groups, the greatest amount of clustering did not fall within the 3–4, 5–6, or 7–8 month intervals, the ages that were thought to constitute the vocal play stage in the early stage models of vocal development (Kent, 2022; Nathani et al., 2006; Oller, 1978, 2000; Stark, 1980). The clustering of squeals showed a tendency to increase toward the middle of the year and peaked at around 9–10 months for both groups. The clustering for growls appeared to be more stable across age than for squeals, showing the highest values at the first and the final intervals of the year. The last panel of Fig. 1 displays the percentage of recordings that showed significant clustering of either squeals or growls at each of the age intervals for both groups. Significant clustering of either squeals or growls occurred in from 49 to 68% of the recordings from TD infants and from 57 to 69% of the recordings from autistic infants. A slight increasing trend for clustering patterns was observed for both groups. Notably, all the age intervals beyond 5 months demonstrated significant clustering for at least 60% of the recordings. And there was no age interval, even the 0–2 month interval, where significant clustering was absent.
Correlation Between the Extent of Vocal Clustering and Outcomes at 2 Years
Correlation analyses revealed that the proportion of recordings with significant clustering of squeals, growls, or either squeal or growls did not correlate significantly with any outcome measures at 2 years. The very low correlation coefficients for the extent of vocal clustering and MSEL expressive or receptive language ranged between − 0.13 to 0.1 (all p values > 0.5). Likewise, the correlation coefficients for the extent of vocal clustering and ADOS-2 scores ranged between − 0.2 and 0.09 (all p values > 0.3). Detailed results are presented in Table 3.
Discussion
To our knowledge, this study represents the first quantitative assessment of the tendency of autistic infants to produce vocal categories in clusters across the first year of life. We compared infants’ vocal play patterns for squeals, vocants, and growls across 1293 longitudinal day-long recordings from 103 TD infants and 44 autistic infants. On average, for each infant, 117 five-minute segments (~ 9.75 hours) across their first year of life were human-coded to examine these vocal play patterns. Given that vocants dominate infants’ early vocalizations, constituting about 80% of all protophones (Oller et al., 2021) and demonstrate primarily modal phonation in the usual pitch range of each infant, the present work focused on the clustering of protophones with non-modal phonation (squeals and growls) in relation to vocants.
Every infant in the study, regardless of diagnostic outcome, showed significant clustering of either squeals or growls in at least one of their recordings. For both TD and autistic infants, more than 60% of recordings on average showed significant clustering for squeals or growls. These findings highlight the robustness of the systematic production of vocal categories across the first year of life. The similarity of the clustering patterns in the TD and autistic groups suggests that vocal category formation through active infant vocal exploration is a deep human tendency.
It has long been speculated that vocal exploration is critically important in establishing foundations for language (Oller, 1980; Stark, 1980;), but now there is a basis to speculate further: it appears that vocal category formation as manifested in clustering may be critically important to the development of foundations for language. The high volubility of exploratory vocalization may form a circumstance in which category formation emerges simply because infants listen to themselves and notice the differences in phonatory patterns they are able to produce. The apparent practice that accompanies this recognition would seem to fit with modern theories emphasizing the inherent nature of curiosity and active “seeking” for information about the world, certainly in humans, but presumably in many species (Gottlieb & Oudeyer, 2018; Panksepp, 2009;). Curiosity and the exploration that is motivated by it are thought by some to be the primary drivers of learning (Panksepp & Biven, 2012).
Because significant clustering of both squeals and growls was evident at the very first age interval for both TD and autistic infants, it seems clear that active vocal exploration occurred from very early in life. Not only did protophones show the tendency to cluster from the first months from the present study, but another study revealed that protophones also demonstrate functional flexibility from at least the first three months (Jhang & Oller, 2017). The cited work showed that each of the three vocal categories can express both neutral and negative emotional states on different occasions of use. Additional work showed that such flexibility of usage extends to include positive as well as neutral and negative affective states once smiling is established (by about 3 months), after which the full range of affectively flexible expression occurs for all three phonatory categories at all subsequent infant ages throughout the first year (Oller et al., 2013). The infants’ tendency to produce protophones in clusters and to use them flexibly in terms of affective expression suggests infants achieve both stable form and flexible function in the first months. Taken together, these findings reiterate the special role protophones serve as precursors to oral language.
It may be thought surprising that no strong relation was revealed between vocal clustering in the autistic group and autism severity or language outcomes. We initially speculated that vocal clustering might be a manifestation of RRBs in some autistic children. The lack of a significant correlation should not, however, be taken as the final word on the topic, because again, the coding system allowed only three categories of phonation to be indicated, a limitation that may have obscured possible relations between vocal clustering and later outcomes. It is also important to note that our study’s focus on the first year of life and our focus only on human coding of three particular vocal categories may have caused us to miss other features of vocalization that may differ across autistic individuals compared with TD individuals. Prior work has suggested that prosodic differences may indeed accompany speech in autistic children and adults. For example, both variability of pitch and long-term spectra of speech have been found different across autistic and TD children 4–6 years of age (Bonneh et al., 2011). A recent meta-analysis involving 39 studies also concluded that autistic individuals had higher mean pitch, larger range and variability of pitch, and greater voice duration than TD controls (Asghari et al., 2021). Sheinkopf et al. (2000) reported that autistic preschoolers produced significantly more vocalizations with atypical vocal quality (defined as vocalizations produced as squeals, growls, or yells) than children with developmental delay. Interestingly, atypical vocal quality ratio in autistic children did not correlate significantly with joint attention measures in Sheinkopf et al. (2000), a finding that may correspond to the lack of a significant correlation between vocal clustering and social communication measured by the ADOS-2 Social Affect score in the present study. Future longitudinal studies are warranted to further illuminate the relationship between early vocalization and autism outcomes.
Limitations
While real-time coding is an efficient way to code a large number of day-long recordings, it provides only a simplified sketch of infant vocalization. Individual utterances of infants at any age can include characteristics of any one or all the phonatory categories, in addition to involving multiple vocal regimes not generally addressed in defining the three primary categories. To simplify the task, coders were trained to make each judgment based on the most auditorily salient characteristic of each utterance. Real-time coding may be less precise or reliable than repeat-listening coding (but see Willadsen et al., 2020), yet there is an important sense in which real-time coding may be preferable, because it resembles the recognition of infant vocalizations by caregivers, who interact with infants in real time and, like our coders, only have one opportunity to interpret each vocalization. The findings of the study seem to confirm common observations from caregivers that infants produce various vocal types in clusters (Stark, 1978; Oller, 2000), a pattern that suggests infants may be practicing these vocal categories. The very fact that parents express the opinion that infants are practicing vocally when they repeat sounds adds weight to the biological argument that infant vocal activity must provide reliable evidence to caregivers of infants’ growing capacity for vocal communication (Oller, 2000).
Another limitation is that all EL infants recruited to our sample had an older autistic sibling, but we did not control for the number of older siblings in either diagnostic group. Given the endogenous nature of infant vocalizations, it could be that having an older sibling would have little or no influence on infant vocal practice patterns, but findings from this study may not generalize well to autistic infants from single-incidence families or families with multiple older children.
Conclusions
This study is, as far as we know, the first to examine patterns of clustering in vocal production in autistic infants quantitatively. Three main findings emerged. First, both TD and autistic infants showed clustering patterns of protophones in their first year, suggesting that all the infants in this study appeared to be practicing vocal categories. This main finding suggests that vocal clustering practice is a robust feature of and an important precursor for language development. Second, the clustering of vocal categories occurred in both groups from the first month and showed an increasing trend of clustering of squeals and a stable trend of clustering of growls. Lastly, the extent of clustering of vocal categories in autistic infants in the first year showed extremely low and non-significant correlations with autism severity or language outcomes at 2 years.
Overall, the results provide support for the idea that vocal play and clustering of vocal types may be a fundamental property of human development, laying a deep foundation for later speech and language development. It is hard to imagine how one could learn to talk without the ability to form vocal categories. The fact that our results did not reveal significant reduction in vocal play clustering among the autistic infants suggests further that the clustering tendency may be so important to human development that it has been evolved to resist developmental differences associated with emerging autism, at least in the first year of life. We caution, however, that our study is focused on phonatory development only. There is much room remaining for comparative evaluation of vocal capacities and inclinations across autism and typical development.
Notes
We use “autistic infants” to refer to infants who later received a confirmed diagnosis of autism. We also draw a distinction between “EL (elevated likelihood) infants” and “autistic infants”, with the latter referring to infants with a confirmed diagnosis of autism regardless of their likelihood status.
Speech-like or speech-related vocalizations are defined variously across studies. Some studies only include vocalizations that can be reasonably represented by phonetic symbols (excluding squeals and growls) in the speech-like category (Paul et al., 2011; Plate et al., 2021). Other studies include all three phonatory protophones (squeals, growls, and vocants) and others in addition to canonical babbling as speech-like vocalizations (Patten et al., 2014; Yoo et al., 2018; Oller, 2000; Oller et al., 2021).
References
American Psychiatric Association. (2013). DSM-V. American Journal of Psychiatry. https://doi.org/10.1176/appi.books.9780890425596.744053
Asghari, S. Z., Farashi, S., Bashirian, S., & Jenabi, E. (2021). Distinctive prosodic features of people with autism spectrum disorder: A systematic review and meta-analysis study. Scientific Reports. https://doi.org/10.1038/s41598-021-02487-6
Bauer, H. R., & Kent, R. D. (1987). Acoustic analyses of infant fricative and trill vocalizations. The Journal of the Acoustical Society of America, 81(2), 505–511.
Beebe, B., Alson, D., Jaffe, J., Feldstein, S., & Crown, C. (1988). Vocal congruence in mother-infant play. Journal of Psycholinguistic Research, 17(3), 245–259. https://doi.org/10.1007/BF01686358
Bloom, K. (1988). Quality of adult vocalizations affects the quality of infant vocalizations. Journal of Child Language, 15(3), 469–480. https://doi.org/10.1017/S0305000900012502
Bonneh, Y., Levanon, Y., Dean-Pardo, O., Lossos, L., & Adini, Y. (2011). Abnormal speech spectrum and increased pitch variability in young autistic children. Frontiers in Human Neuroscience. https://doi.org/10.3389/fnhum.2010.00237
Buder, E., McDaniel, V. F., Bene, E. R., Ladmirault, J., & Oller, D. K. (2018). Registers in infant phonation. Journal of Voice, 33(3), 382.e21-382.e32. https://doi.org/10.1016/j.jvoice.2017.12.013
Buder, E., Warlaumont, A. S., & Oller, D. K. (2013). An acoustic phonetic catalog of prespeech vocalizations. In B. Peter & A. A. N. MacLeod (Eds.), Comprehensive Perspectives on Child Speech Development and Disorders: Pathways from Linguistic Theory to Clinical Practice. Nova Publishers.
Chenausky, K., Nelson, C., & Tager-Flusberg, H. (2017). Pre-linguistic vocal trajectories at 6–18 months of age as early markers of autism. Journal of Speech, Language, and Hearing Research, 60(4), 865–876. https://doi.org/10.1044/2016_JSLHR-S-15-0400
Croft, W. (2001). Radical Construction Grammar Syntactic Theory in Typological Perspective. Oxford University Press.
Delgado, R., Buder, E., & Oller, D. (2010). Action Analysis Coding and Training (AACT). Intelligent Hearing Systems.
Elbers, L. (1997). Output as input: A constructivist hypothesis in language acquisition. Archives De Psychologie, 65(253), 131–140.
Fisher, R. A. (1934). Statistical Methods for Research Workers. Springer.
Freeman, G., & Halton, J. H. (1951). Note on an exact treatment of contingency, goodness of fit and other problems of significance. Biometrika, 38, 141–149.
Gilkerson, J., & Richards, J. A. (2008). The LENA natural language study. LENA Foundation Technical Report, 1–26.
Good, P. I. (2005). Permutation, Parametric, and Bootstrap Tests of Hypotheses. Springer.
Gottlieb, J., & Oudeyer, P.-Y. (2018). Towards a neuroscience of active sampling and curiosity. Nature Reviews Neuroscience, 19(12), 758–770. https://doi.org/10.1038/s41583-018-0078-0
Griebel, U., & Oller, D. K. (2014). Origins of language in a comparative perspective. Evolutionary Science of Human Behavior: An Interdisciplinary Approach, 257–280.
Griebel, U., Pepperberg, I. M., & Oller, D. K. (2016). Developmental plasticity and language: A comparative perspective. Topics in Cognitive Science, 8(2), 435–445. https://doi.org/10.1111/tops.12200
Holmgren, K., Lindblom, B., Aurelius, G., Jailing, B., & Zetterström, R. (1986). On the phonetics of infant vocalization. In B. Lindblom & R. Zetterström (Eds.), Precursors of Early Speech: Proceedings of an International Symposium Held at the Wenner-Gren Center Stockholm. Palgrave Macmillan.
Jhang, Y., & Oller, D. K. (2017). Emergence of functional flexibility in infant vocalizations of the first 3 months. Frontiers in Psychology. https://doi.org/10.3389/fpsyg.2017.00300
Kaye, K., & Fogel, A. (1980). The temporal structure of face-to-face communication between mothers and infants. Developmental Psychology, 16(5), 454–464. https://doi.org/10.1037/0012-1649.16.5.454
Kent, R. (1981). Articulatory-acoustic perspectives on speech development. Language Behavior in Infancy and Early Childhood, 105–126
Kent, R. (2022). The maturational gradient of infant vocalizations: Developmental stages and functional modules. Infant Behavior and Development, 66, 101682. https://doi.org/10.1016/j.infbeh.2021.101682
Laufer, M. Z., & Horii, Y. (1977). Fundamental frequency characteristics of infant non-distress vocalization during the first twenty-four weeks. Journal of Child Language, 4(2), 171–184. https://doi.org/10.1017/S0305000900001586
Long, H. L., Bowman, D. D., Yoo, H., Burkhardt-Reed, M. M., Bene, E. R., & Kimbrough Oller, D. (2020). Social and endogenous infant vocalizations. PLoS ONE. https://doi.org/10.1371/journal.pone.0224956
Lord, C., Rutter, M., DiLavore, P., Risi, S., Gotham, K., & Bishop, S. (2012). Autism Diagnostic Observation Schedule—Second Edition (ADOS-2). Los Angeles, CA: Western Psychological Services.
Lynch, M. P., Oller, D. K., Steffens, M. L., & Buder, E. H. (1995). Phrasing in prelinguistic vocalizations. Developmental Psychobiology, 28(1), 3–25. https://doi.org/10.1002/dev.420280103
Mullen, E. M. (1995). Mullen Scales of Early Learning: Manual and Item Administrative Books. American Guidance Services Inc.
Nathani, S., Ertmer, D. J., & Stark, R. E. (2006). Assessing vocal development in infants and toddlers. Clinical Linguistics & Phonetics, 20(5), 351–369. https://doi.org/10.1080/02699200500211451
Northrup, J. B., & Iverson, J. M. (2015). Vocal coordination during early parent-infant interactions predicts language outcome in infant siblings of children with autism spectrum disorder. Infancy, 20(5), 523–547.
Oller, D. K. (1978). Infant vocalization and the development of speech. Allied Health and Behavioral Sciences, 1(4), 523–549.
Oller, D. K. (1980). The emergence of the sounds of speech in infancy. Child Phonology. https://scholar.google.com/citations?view_op=view_citation&hl=en&user=oCLGfM4AAAAJ&cstart=200&pagesize=100&sortby=pubdate&citation_for_view=oCLGfM4AAAAJ%3Au5HHmVD_uO8C&inst=1027955225067792439
Oller, D. K. (1981). Infant vocalizations: Exploration and reflexivity. Language Behavior in Infancy and Early Childhood, 85–103.
Oller, D. K. (1986). Metaphonology and infant vocalizations. Precursors of Early Speech: Proceedings of an International Symposium Held at The Wenner-Gren Center, Stockholm, September 19–22, 1984, 21–35.
Oller, D. K. (2000). The emergence of the speech capacity. Psychology Press. https://doi.org/10.4324/9781410602565
Oller, D. K., Buder, E. H., Ramsdell, H. L., Warlaumont, A. S., Chorna, L., & Bakeman, R. (2013). Functional flexibility of infant vocalization and the emergence of language. Proceedings of the National Academy of Sciences of the United States of America, 110(16), 6318–6323. https://doi.org/10.1073/pnas.1300337110
Oller, D. K., Caskey, M., Yoo, H., Bene, E. R., Jhang, Y., Lee, C. C., Bowman, D. D., Long, H. L., Buder, E. H., & Vohr, B. (2019a). Preterm and full term infant vocalization and the origin of language. Scientific Reports, 9(1), 1–10. https://doi.org/10.1038/s41598-019-51352-0
Oller, D. K., Griebel, U., Iyer, S. N., Jhang, Y., Warlaumont, A. S., Dale, R., & Call, J. (2019b). Language origins viewed in spontaneous and interactive vocal rates of human and bonobo infants. Frontiers in Psychology. https://doi.org/10.3389/fpsyg.2019.00729
Oller, D. K., Griebel, U., & Warlaumont, A. S. (2016). Vocal development as a guide to modeling the evolution of language. Topics in Cognitive Science, 8(2), 382–392. https://doi.org/10.1111/tops.12198
Oller, D. K., Iyer, S., Buder, E., Kwon, K., Chorna, L., & Conway, K. (2007). Diversity and contrastivity in prosodic and syllabic development. In J. Trouvain & W. J. Berry (Eds.), Proceedings of ICPhS (Saarbrücken) (pp. 303–308).
Oller, D. K., Ramsay, G., Bene, E., Long, H. L., & Griebel, U. (2021). Protophones, the precursors to speech, dominate the human infant vocal landscape. Philosophical Transactions of the Royal Society B: Biological Sciences, 376(1836), 20200255. https://doi.org/10.1098/rstb.2020.0255
Osterling, J. A., Dawson, G., & Munson, J. A. (2002). Early recognition of 1-year-old infants with autism spectrum disorder versus mental retardation. Development and Psychopathology, 14(2), 239–251.
Panksepp, J. (2009). The emotional antecedents to the evolution of music and language. Musicae Scientiae. https://doi.org/10.1177/1029864909013002111
Panksepp, J., & Biven, L. (2012). A meditation on the affective neuroscientific view of human and animalian MindBrains. In From the Couch to the Lab: Trends in Psychodynamic Neuroscience (pp. 145–175).
Patten, E., Belardi, K., Baranek, G. T., Watson, L. R., Labban, J. D., & Oller, D. K. (2014). Vocal patterns in infants with autism spectrum disorder: Canonical babbling status and vocalization frequency. Journal of Autism and Developmental Disorders, 44(10), 2413–2428. https://doi.org/10.1007/s10803-014-2047-4
Paul, R., Fuerst, Y., Ramsay, G., Chawarska, K., & Klin, A. (2011). Out of the mouths of babes: Vocal production in infant siblings of children with ASD. Journal of Child Psychology and Psychiatry, 52(5), 588–598. https://doi.org/10.1111/j.1469-7610.2010.02332.x
Plate, S., Yankowitz, L., Resorla, L., Swanson, M. R., Meera, S. S., Estes, A., Marrus, N., Cola, M., Petrulla, V., Faggen, A., Pandey, J., Paterson, S., Pruett, J. R., Hazlett, H., Dager, S., John, T., Botteron, K., Zwaigenbaum, L., Piven, J., IBIS Network. (2021). Infant vocalizing and phenotypic outcomes in autism: Evidence from the first 2 years. Child Development, 93(2), 468–483. https://doi.org/10.1111/cdev.13697
Robb, M., & Saxman, J. (1988). Acoustic observations in young children’s non-cry vocalizations. Journal of the Acoustical Society of America, 83(5), 1876–1882.
Sheinkopf, S. J., Mundy, P., Oller, D. K., & Steffens, M. (2000). Vocal atypicalities of preverbal autistic children. Journal of Autism and Developmental Disorders. https://doi.org/10.1023/A:1005531501155
Stark, R. E. (1978). Features of infant sounds: The emergence of cooing. Journal of Child Language, 5(3), 379–390. https://doi.org/10.1017/S0305000900002051
Stark, R. E. (1980). Stages of speech development in the first year of life. In G. H. Yeni-Komshian, J. F. Kavanagh, & C. A. Ferguson (Eds.), Child Phonology. Academic Press.
Stark, R. E. (1981). Infant vocalization: A comprehensive view. Infant Mental Health Journal, 2(2), 118–128.
Stark, R. E., Rose, S. N., & McLagen, M. (1975). Features of infant sounds: The first eight weeks of life. Journal of Child Language, 2(2), 205–221. https://doi.org/10.1017/S0305000900001045
Stern, D., Jaffe, J., Beebe, B., & Bennett, S. L. (1975). Vocalizing in unison and in alternation: Two modes of communication within the mother-infant dyad. In D. Aaronson & R. Rieber (Eds.), Developmental Psycholinguistics and Communication Disorders. New York Academy of Sciences.
Swanson, M. R., Shen, M. D., Wolff, J. J., Boyd, B., Clements, M., Rehg, J., Elison, J. T., Paterson, S., Parish-Morris, J., Chappell, J. C., Hazlett, H. C., Emerson, R. W., Botteron, K., Pandey, J., Schultz, R. T., Dager, S. R., Zwaigenbaum, L., Estes, A. M., Piven, J., & Gu, H. (2018). Naturalistic language recordings reveal “Hypervocal” infants at high familial risk for autism. Child Development. https://doi.org/10.1111/cdev.12777
Tomasello, M. (2003). Constructing a Language: A Usage-Based Theory of Language Acquisition. Harvard University Press.
Warlaumont, A. S., Richards, J. A., Gilkerson, J., & Oller, D. K. (2014). A social feedback loop for speech development and its reduction in autism. Psychological Science, 25(7), 1314–1324. https://doi.org/10.1177/0956797614531023
Willadsen, E., Persson, C., Patrick, K., Lohmander, A., & Oller, D. K. (2020). Assessment of prelinguistic vocalizations in real time: A comparison with phonetic transcription and assessment of inter-coder-reliability. Clinical Linguistics & Phonetics, 34(7), 593–616. https://doi.org/10.1080/02699206.2019.1681516
Yoo, H., Bowman, D. A., & Oller, D. K. (2018). The origin of protoconversation: An examination of caregiver responses to cry and speech-like vocalizations. Frontiers in Psychology, 9, 1510. https://doi.org/10.3389/fpsyg.2018.01510
Yoo, H., Su, P. L., Ramsay, G., Long, H. L., Bene, E. R., & Oller, D. K. (in press). Infant vocal category exploration as a foundation for speech development. PLOS One.
Acknowledgments
The authors wish to thank the participating families for their time and dedication to the longitudinal component of this project. We also thank OLL lab graduate research assistant coders.
Funding
Research reported in this publication was supported by NIDCD Grant R01DC015108 (PI: Oller, Subaward PI: Ramsay), and NIMH Grant P50MH100029 (Co-PI: Ramsay). Additional support was provided by the Plough Foundation, the Holly Lane Foundation, the Marcus Foundation, the Woodruff-Whitehead Foundation, and the Georgia Research Alliance.
Author information
Authors and Affiliations
Contributions
The authors confirm their contribution to the paper as follows: study conception and design: PLS, HY, GR, ERB, and DKO; data collection and clinical assessment: GR, CK, SPW, SR, MLP, NB; data coding, analysis, and interpretation of results: PLS, HY, GR, ERB, HLL, DKO; Writing—Original draft: PLS, DKO; Writing—Review and Editing: PLS, HY, HLL, GR, ERB, CK, SPW, SR, MLP, NB, DKO. All authors reviewed the results and approved the final version of the manuscript.
Corresponding author
Ethics declarations
Conflict of interest
DKO is an unpaid member of the LENA Scientific Advisory Board. No other authors have any competing interests to report. The authors have no conflicts of interest to disclose.
Additional information
Publisher's Note
Springer nature remains neutral with regard to jurisdictional claims in published maps and institutional affiliations.
Supplementary Information
Below is the link to the electronic supplementary material.
Rights and permissions
Springer Nature or its licensor (e.g. a society or other partner) holds exclusive rights to this article under a publishing agreement with the author(s) or other rightsholder(s); author self-archiving of the accepted manuscript version of this article is solely governed by the terms of such publishing agreement and applicable law.
About this article
Cite this article
Su, P.L., Yoo, H., Ramsay, G. et al. Foundations of Vocal Category Development in Autistic Infants. J Autism Dev Disord (2024). https://doi.org/10.1007/s10803-024-06267-9
Accepted:
Published:
DOI: https://doi.org/10.1007/s10803-024-06267-9