Abstract
The lack of stringent policies to avert climate change has increased the importance of effective and timely adaptation. Adequate adaptation is particularly important for agricultural communities in developing countries, which may most suffer the consequences of climate change. Evidence is still scarce on how people in the most vulnerable areas form climate change beliefs and whether such beliefs exhibit cognitive biases. Using survey data from rural households in Bangladesh together with a meteorological measure of excess dryness relative to historical averages, I study the effect of long-term average drought exposure and short-term deviations on beliefs about drought frequency and the interpretation of drought events. To explore how individuals interpret past droughts, I use an instrumental variable approach and investigate whether individual beliefs lead to asymmetric distortion of objective information. The results show that individuals recollect and overweight evidence tilted towards their prior beliefs, providing evidence of confirmation bias as a directional motivated reasoning mechanism. The findings highlight the need for models that account for behavioral factors and cognitive biases in the study of climate change beliefs for effective communication and adaptation policies.
Similar content being viewed by others
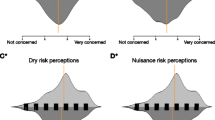
Avoid common mistakes on your manuscript.
1 Introduction
Climate change threatens to alter the frequency, timing, duration, intensity and spatial distribution of extreme weather events, including droughts (IPCC 2021). Despite broad scientific consensus that human activities are causing climate change (Oreskes 2004), there is ample disagreement among the general public in the beliefs about climate change and its causes (Lee et al. 2015). The inertia of policies to avert significant climate change has increased the importance of adaptation. Effective adaptation is particularly important in developing countries and rural areas (Mertz et al. 2009). The relationship between meteorological conditions and agricultural yields has been extensively empirically documented (Auffhammer and Schlenker 2014; Carleton and Hsiang 2016; Hultgren et al. 2022) with implicit models of adaptation that assume agents react to objectively interpreted new information conditional on prior beliefs, fully accounted for by meteorological conditions. Understanding the determinants of beliefs and the existence of cognitive biases among the most vulnerable communities, whose activities heavily rely on natural resources and climate, is of paramount importance since it may have direct implications for adaptive behavioral responses (Zappalà 2023).
This paper studies the effect of drought exposure on beliefs about climate change and investigates whether individuals adopt directional motivated reasoning, according to which they tend to overweight evidence that confirms their prior beliefs. I combine a two-wave survey of rural households in Bangladesh with a meteorological measure of dryness at the union-levelFootnote 1, the Standardized Precipitation Evapotranspiration Index (SPEI) (Vicente-Serrano et al. 2010). First, I document how long-term average exposure to dryness and short-term deviations affect individuals’ beliefs about drought frequency and their accuracy in interpreting these events. To define accuracy, I compute the deviation between the self-reported number of droughts and meteorological events measured using climatological cut-offs (McKee et al. 1993; Paulo et al. 2012). A positive difference indicates overestimation in the recollection of droughts. Second, I examine the potential cognitive heuristics adopted in the interpretation of droughts. I test whether individuals asymmetrically distort objective information overweighting evidence that confirms their prior beliefs, showing evidence of confirmation bias (Rabin and Schrag 1999; Kahneman and Tversky 1982). To identify the causal effect of prior beliefs on how information from drought events is distorted, I adopt an instrumental variable approach using as instrument the twenty-year long-term average exposure to dryness, which exploits quasi-random variation in the SPEI realizations within unions over time. The exogeneity of the instrument relies on the assumption that accounting for time- and individual-specific unobserved heterogeneity, deviations in meteorological conditions of dryness do not affect the accuracy of recollecting drought events via other channels than beliefs. To assess the validity of this assumption, I perform several checks ruling out other channels such as adaptation, recent deviations in terms of dryness, and information.
The analysis yields two main findings. First, twenty-year long-term average exposure to dryness predicts beliefs of increase in droughts and the interpretation of drought events, whereas short-term deviations in exposure do not matter. Individuals form beliefs based on exposure to their average climatic conditions and beliefs about slow-onset environmental changes are inelastic to short-term deviations. Second, I document that individuals overestimate the number of drought events when they believe that droughts have increased. This result shows that individuals adopt directional motivated reasoning, with the interpretation of droughts biased towards their priors. This finding differs from objective processing of information in a Bayesian setting, where individual prior beliefs do not affect the interpretation of information (Druckman and McGrath 2019).
The paper makes several contributions to the literature. First, it relates to the branch analysing the determinants of climate change beliefs, widely investigated in developed countries and identified in political orientation, education, and personal experience of weather shocks (e.g., Carlsson et al. 2021; Czarnek et al. 2021; Poortinga et al. 2019; Hoffmann et al. 2022.Footnote 2 A growing attention has been devoted to individuals whose economic livelihood depends on climate, including farmers or fishers. Most evidence is based on US data (Gramig et al. 2013; Rejesus et al. 2013; Arbuckle et al. 2013a, b), whereas it is yet understudied the formation process in developing countries. Understanding climate change awareness in Bangladesh is of paramount importance, where, according to the 2007–2008 Gallup World Poll representative survey, more than 65% of respondents had never heard of climate change, in contrast with the low levels (below 10%) of climate change skepticism in high-income countries (Lee et al. 2015). More than a decade later, in the 2019 Gallup World Risk Poll, more than one-third of the population in Bangladesh was still unable to provide an answer to the potential effects of climate change (Rzepa and Ray 2020). The paper provides empirical evidence of the determinants of beliefs on the consequences of climate change in a developing country, focusing on slow-onset environmental changes. Importantly, I exploit the unique longitudinal dimension of the survey to account for individual-specific unobserved heterogeneity and study within-individual changes in beliefs.
Second, this paper relates to the strand of literature that investigates cognitive heuristics associated with climate change beliefs, including anchoring, availability, representativeness or motivated reasoning (Joireman et al. 2010; Li et al. 2011; Zaval et al. 2014). This paper contributes to this literature testing the confirmation bias hypothesis. Individuals exhibit confirmation bias as a form of directional motivated reasoning if they misread the new evidence as supportive of existing hypotheses, interpreting information and overweighting evidence that confirms their beliefs (Fryer et al. 2019; Agnew et al. 2018b; Faia et al. 2021). Notwithstanding previous theoretical discussions of directional motivated reasoning mechanisms (Druckman and McGrath 2019) and other cognitive biases (Zhao and Luo 2021) in climate change beliefs, former empirical studies have focused on other types of cognitive biases, including availability bias (Gallagher 2014), representativeness and spreading activation (Deryugina 2013) in the US. The sole exception in rural communities in developing countries finds recency bias among Indian farmers (Kala 2017).
The literature on motivated reasoning has concluded that prior climate beliefs influence the interpretation of environmental changes (Goebbert et al. 2012; Zanocco et al. 2018). Previous empirical studies testing motivated reasoning neglect potential endogeneity concerns between the interpretation of evidence and beliefs (Howe and Leiserowitz 2013; Myers et al. 2013; Shao 2016). I build on studies of motivated reasoning in climate change beliefs (Weber 1997; Osberghaus and Fugger 2022; Stahlmann-Brown and Walsh 2022) to estimate the effect of beliefs on the interpretation of weather events in a developing country. In Bangladesh, where climate change awareness is particularly low (Lee et al. 2015; Rzepa and Ray 2020) and drought vulnerability extremely high (Shahid 2011), examining the drivers of the interpretation of droughts and the presence of cognitive biases is fundamental. This is, to the best of my knowledge, the first study that tests whether individuals display directional motivated reasoning in a developing country, identifying the causal effect of beliefs on how information from weather events is distorted in a quasi-experimental setting.
The remainder of the paper is organized as follows. Section 2 describes the data used in the empirical analysis. Section 3 defines the conceptual framework for the propositions that I test empirically. Section 4 presents the empirical approach. Section 5 discusses the results and their robustness. Section 6 concludes.
2 Data
I combine data from two main sources to measure beliefs and self-reported incidence of drought events at the individual level on the one hand, and meteorological measures of exposure to dryness and occurrence of drought events computed at the union level, on the other.
Beliefs about droughts and self-reported drought events. I measure individual beliefs and self-reported frequency of drought events from the Bangladesh Climate Change Adaptation Survey (BCCAS). The data consist of a two-wave survey by the International Food Policy Research Institute (2014), collecting information from 800 agricultural households in 40 randomly selected unions in Bangladesh (Table A1). The first wave was conducted in January 2011 and previously analysed in Delaporte and Maurel (2018). A follow-up wave (International Food Policy Research Institute 2014) was conducted in September 2012. More than 97%, i.e., 766 out of 800 households, were reinterviewed in the second wave.Footnote 3 I construct a binary variable, Belief of Increase in Droughts, or simply Belief, equal to one if the respondent answers “Longer periods of droughts” to the question “Have you noticed any changes in climate over the last 20 years? If yes, please specify what changes you have noticed.”
Prior to being asked about their beliefs, individuals are asked a series of questions about their memories of weather events in recent years, as in Weber (1997). I construct the variable self-reported # droughts using the question in the first wave “In the last five years, have the household’s properties and productivity been affected by droughts? How many times did it occur?”. The same question in the second wave asks respondents to report the number of droughts since the last interview. This variable is then used to measure the accuracy of recollection of drought events as explained below. Table A2 reports the exact wording and formulation of each question in the two waves.Footnote 4 Although the survey does not provide a formal definition of droughts and does not record differently the intensity of perceived weather events, different interpretation of droughts by different respondents does not pose a challenge to the validity of the empirical analysis that exploits within-individual variation in beliefs over time.Footnote 5
Dryness exposure. To construct a measure of exposure to dryness, I use a climatological measure, the Standardized Precipitation Evapotranspiration Index (SPEI) (Vicente-Serrano et al. 2010), which provides information about drought conditions at the global scale, with a 0.5\(^\circ \) spatial resolution (\(\approx \) 55 km at the Equator) and a monthly time resolution. The SPEI-1 compares the amount of precipitation and potential evapotranspiration to obtain a measure of drought based on water balance accumulated over one month and is constructed using data from the Climatic Research Unit of the University of East Anglia (CRU TS version 4.03). The index is a standardized probability measuring the deviation in dryness relative to the average observed during the available 1901–2018 time period in each grid cell. A value of zero indicates the median amount (half of the historical amounts are below the median, and half are above the median), and the index is negative for dry, and positive for wet conditions. For instance, a value equal to – 1 indicates that the difference between precipitation and potential evapotranspiration is one standard deviation lower than the historical average for a given grid cell.
I build two measures of exposure to dryness at the union level to account for long-term average and short-term deviation (Guiteras et al. 2015; Hsiang and Jina 2014; Bento et al. 2020).Footnote 6 I construct union-level SPEI monthly realizations as a weighted average of the union surface over each grid cell. Figure A1 displays the spatial relationship between the union boundaries and the SPEI gridded cells. The long-term exposure is the average of the monthly SPEI across the previous twenty years, indicating whether this period was relatively drier or wetter than the historical average for each union. This measure is constructed as the “objective counterfactual” of the individual beliefs that droughts have increased in the previous twenty years. Beliefs are assumed to be formed from the long-term average exposure in the union of residence.Footnote 7
I construct a short-term deviation measure from the long-term average, as the difference between the average SPEI monthly realizations over the previous five years and the twenty-year long-term average, for the first wave, and the difference between the average SPEI monthly realizations between the two waves and the twenty-year long-term average, for the second wave.Footnote 8
Drought events. To have a measure of individual accuracy of recollection of droughts, I compare the self-reported number with the objectively recorded number of drought events. The climatology literature defines a drought event as the period of consecutive time points in which the SPEI is below certain thresholds (Spinoni et al. 2014). Specifically, there are five classes of droughts: (1) non-drought (\(\text {SPEI}>-0.5\)); (2) mild droughts (\(-1<\text {SPEI}\le -0.5\)); (3) moderate droughts (\(-1.5<\text {SPEI}\le -1\)); (4) severe droughts (\(-2<\text {SPEI}\le -1.5\)); (5) extreme droughts (\(\text {SPEI}\le - 2\)) (Paulo et al. 2012; McKee et al. 1993). Since the SPEI is normally distributed, each of the five classes respectively accounts for about 69.1%, 15%, 9.2%, 4.4% and 2.3% of the set of historical values for each grid cell.
Based on this classification, I compute for each union the number of extreme drought events that have occurred in the five years before the first wave of the survey and between the first and the second wave.Footnote 9 To test the robustness of the results, I employ other cut-offs to define the objective number of droughts, including moderate (\(\text {SPEI}\le -1\)) and severe (\(\text {SPEI}\le -1.5\)) droughts. Figure A2 shows the timeline of the survey compared to the construction of the measures of dryness and drought events.
Following this approach, I create a measure of accuracy of recollection of past drought events:
where \(\Delta ^{type}_{it}\) (\(type \in \{\text {moderate; severe; extreme}\}\)) measures the deviation between the self-reported number of droughts by individual i in survey wave t with the number of droughts recorded using the SPEI in union u over the same time period. These wave-specific measures of interpretation infer whether respondents overestimate or underestimate the number of drought events that they have experienced. For instance, a positive value shows that individuals overestimated the number of drought events. By matching households with objectively recorded drought events at the union level, I measure asymmetric changes in the recollection of drought events for individuals that faced the same course of events and have been exposed to the same set of objective information.Footnote 10
Descriptive statistics. The final sample is composed of 714 individuals. Since the focus is on personal experience, the sample includes only households who have been surveyed in both waves and did not move, and for which the respondent was the same. This setting accounts for individual-level unobserved heterogeneity (including different interpretation of the questions) and allays concerns about the biasedness of the coefficients associated with self-reported subjective measures. Table A3 tests for differences in means for the main variables between the sample of attritors and non-attritors in the first wave and finds no statistically significant differences.
Tables A4 and A5 provide, respectively, summary statistics on self-reported variables and objective measures of drought exposure. On average, half of the sample believes that droughts have increased over the past twenty years. All unions have experienced at least one moderate drought event in both time periods considered in the first and second waves. Although between the two waves only one extreme drought event is recorded in Chaklarhat, in the northwest region of Bangladesh, an area historically prone to drought events (Alamgir et al. 2015), the share of individuals believing that droughts have increased is 46 percentage points higher in the second wave.
Table A4 shows that respondents on average underestimate the number of droughts when the accuracy measure \(\Delta \) includes moderate and severe droughts. On the contrary, \(\Delta \) is on average positive, but close to zero, using only meteorological extreme drought events. Figure A3 displays the frequency distribution of \(\Delta \) with the three cut-offs for the objective measure. A large share of respondents underestimates droughts with moderate (98.6%) and severe cut-offs (68.6%). There may thus be a systematic upward bias when including these two types of drought events as objective counterfactual of the self-reported number of droughts to construct \(\Delta \). This would translate into a downward bias in \(\Delta \). Therefore, I construct \(\Delta \) only including extreme droughts. In this case, most of the respondents (65%) are accurate (\(\Delta = 0\)) and the distribution is right-skewed with more than 25% of the respondents overestimating. Generally, droughts are shown to have substantial impacts on agriculture when the SPEI is below – 1.5, i.e., if the drought is at least severe (Zargar et al. 2011). Hence, extreme drought events may be a valid objective counterfactual for the self-reported droughts, although I test for the robustness of the results including moderate and severe droughts.
3 Conceptual Framework
This section describes a conceptual framework, whose objective is twofold. First, it models the relationship between objective exposure to dryness and self-reported individual beliefs and the way individuals recollect drought events. Second, it sets as a benchmark the Bayesian updating framework in the context of drought events, defining how a Bayesian updater would interpret new information as independent from her prior belief and use both available evidence and prior belief to form a posterior. This is used in comparison to an agent who adopts directional motivated reasoning and interprets evidence as tilted towards her prior beliefs.
3.1 Objective Exposure, Beliefs and Accuracy
In the climate impact literature, an outcome of interest y is related to the environmental exposure E, whose functional form f is ex-ante unknown and requires accurate data in order to be unbiased and precisely estimated. The use of accurate data is even more relevant for extreme weather events, where self-reported survey data have been predominantly used in the literature, despite potentially subject to endogeneity concerns (Guiteras et al. 2015). The baseline equation is
where y represents the outcome of interest, in this case, the belief of increase in droughts and the interpretation of drought events, and E represents dryness exposure. The use of objectively measured right-hand side variables allays the concern about the presence of correlated measurement error between the explanatory and the outcome variable. Self-reported environmental exposure E would provide little information about the relationship of interest between beliefs and exposure to dryness. For example, poorer households may be more exposed to droughts but less able to assess damages accurately.
Individuals may form their beliefs of increase in droughts using their long-term average exposure to dryness as a reference point to judge deviations from the average. In this case, a household frequently exposed to larger droughts and one not frequently exposed would consider a drought of the same magnitude differently. For this reason, a priori, it is uncertain whether beliefs and the recollection of drought events depend on the average conditions of exposure to dryness, deviations from the average, or both. Low-exposure households may be more likely to change their beliefs if they experience a larger drought, whereas households with a larger long-term average exposure to excess dryness may have a more inelastic reaction to deviations from the mean. The following proposition formulates a first initial prediction about the relationship between drought exposure and beliefs.
Proposition 1
Exposure to excess dryness positively affects the belief of increase in droughts and the recollection of drought events, i.e. \(\partial y / \partial E = \partial f(E) / \partial E \ge 0\).
3.2 Bayesian Framework
Bayes’ rule is commonly used for modeling the belief updating process. In a Bayesian updating framework, new information is embodied into prior beliefs to reach an updated posterior belief. Using the standard law of large numbers, a Bayesian updater who forms beliefs conditional on the full sequence of signals would form with probability equal to one a posterior belief of the correct state of nature.
Consider an agent with a prior belief \(\pi (\mu )\), where \(\pi \) denotes the function of belief \(\mu \) as the probability distribution regarding the true state \(\pi (\mu ) \thicksim \mathcal {N}(\widehat{\mu _0}, \widehat{\sigma _0}^2)\), with \(\widehat{\mu _0}\), the agent’s best guess about the true state of the world, and \(\widehat{\sigma _0}^2\), the individual’s uncertainty around her guess, where a \(\widehat{}\) denotes anything related to perceptions (Druckman and McGrath 2019). In this study, the individual belief about an increase in droughts in the past twenty years \(\pi (\mu )\) includes her estimate of the increase in droughts \(\widehat{\mu _0}\) and the confidence in that estimate \(\widehat{\sigma _0}^2\).
Bayesian updating occurs when new information, x, is provided to the individual as a draw from the distribution \(\mathcal {N}(\mu , \widehat{\sigma _x}^2)\), centered at the true state of the world \(\mu \) and with variance in the individual perception of the credibility of the new information, \(\widehat{\sigma _x}^2\). Agents embody the new information and form an updated posterior belief, \(\pi (\mu |x)\). Here, new information x corresponds to the number of drought events in the union of residence of households.
Druckman and McGrath (2019) discuss the accuracy-driven motivated reasoning in climate change preference formation in the Bayesian framework. Individuals aim at arriving at a correct conclusion, evaluating new information x to maximize the likelihood that the posterior belief is an accurate estimate of the true state of nature. Therefore, the evaluation of x is independent of the individual’s prior belief \(\pi (\mu )\). The individual’s prior belief \(\pi (\mu )\) does not affect the interpretation of the new information \(\widehat{x}\), here the self-reported number of drought events.
Estimating every component of Bayes’ formula and the posterior belief is not feasible in this empirical setting due to the lack of available data. Nevertheless, this theoretical result is used to compare how an accuracy-motivated Bayesian agent would differ from an agent that displays directional motivated reasoning. In the latter case, the individual belief would distort the interpretation of new evidence and bias it towards it.
3.3 Directional Motivated Reasoning
In psychology, a “heuristic” is a simplified model for making inferences. Individuals who apply cognitive heuristics may not use all available information or may oversimplify such information when processing it. These cognitive biases are departures from Bayesian updating and some of them have already been documented in the context of climate change belief formation (Gallagher 2014; Deryugina 2013; Fryer et al. 2019). Druckman and McGrath (2019) summarize three mechanisms of directional motivated reasoning in climate change preference formation. Under motivated reasoning, the interpretation of personal experience of climatic changes stems from prior beliefs rather than from impartially detecting changes in their local environment (Palm et al. 2017). The first and foremost mechanism is the confirmation bias (Lodge and Taber 2013).
Individuals subject to confirmation bias are motivated to maintain their prior belief \(\pi (\mu )\) after elaborating new information and thus they seek out information that confirms their prior belief. The distribution from which the individual draws the new information x is no longer \(\mathcal {N}(\mu , \widehat{\sigma _x}^2)\) but \(\mathcal {N}(\widehat{\mu _0}, \widehat{\sigma '_{x}}^{2})\), centered at the mean of the individual’s prior beliefs and not at the true state of the world. The individual belief \(\pi (\mu )\) thus affects the perceived new information \(\widehat{x}\), the self-reported number of drought events.
The interpretation of the information is accurate if \(\widehat{x} - x=0\). Using Eq. (1), individuals are accurate if self-reported and objective number of drought events coincide, i.e. \(\Delta = 0\). Following Fryer et al. (2019), the functional form of the confirmation bias and distortion of information relates the interpretation of objective information x compared to the perceived information \(\widehat{x}\), as a function of the prior belief \(\mu \). Under confirmation bias, the interpretation of information is distorted in the direction of individual beliefs for a given objective information x. This implication is formulated in the form of the following proposition.
Proposition 2
Individuals display directional motivated reasoning and are subject to confirmation bias if the prior belief \(\mu \) affects and distorts the interpretation of the information x. Under directional motivated reasoning, the interpretation of drought events measured as the deviation between the self-reported and recorded number of droughts is a function of individual beliefs of increase in droughts \(\mu \):
4 Empirical Approach
4.1 Objective Exposure, Beliefs and Accuracy
I first examine the effect of exposure to dryness on beliefs of increase in droughts and on how individuals self-report drought events compared to the objectively recorded number. The probability of overestimating the number of droughts is defined as a dummy equal to one if the self-reported number is greater than the number of objectively recorded extreme drought events with the SPEI (i.e., \(\Delta >0\)), and zero otherwise. Afterward, I shift the focus to the extent of overestimation, using the \(\Delta \) measure that takes negative values if individuals underestimate, null if they are accurate and positive if they overestimate the number of drought events.
I employ an OLS regression in a panel setting using individual- and year-specific fixed effects. I estimate beliefs of increase in droughts over the previous twenty years and interpretation of droughts as a function of the long-term average exposure, the deviation from long-term average, and their interaction to account for the heterogeneous effect of deviations. This functional form f(E) is adopted since individuals perceive exposure relative to their average environment and use it as a reference point to judge deviations from that average. The full specification is written as:
where \(y_{it}\) is the belief of increase in drought or the interpretation of drought events for individual i in survey wave t. The coefficients on all weather variables can be interpreted causally since within-union realizations of weather are plausibly exogenous (Carleton and Hsiang 2016; Auffhammer and Carleton 2018). I exploit within-individual variation by accounting for time-invariant individual-specific and year-specific characteristics to identify the effect of drought exposure. Individual-specific fixed effects absorb the effect of all time-invariant factors that differ between individuals, including unobservable characteristics that could not be accounted for in a cross-sectional empirical design, such as personality traits, gender, location, education level, interpretation of droughts (Hsiang 2016). Similarly, \(\lambda _t\) controls for unobserved shocks common to all individuals in a given year.
In five cases out of the 40 sampled unions, the 0.5\(^\circ \) grid cells of the SPEI data embed more than one union. Standard errors clustered at the union-level would be underestimated. For this reason, I cluster standard errors at the grid cell level to account for correlation and heteroskedasticity across unions, and a fortiori individuals, within the same cell.Footnote 11
4.2 Directional Motivated Reasoning
To examine whether individuals exhibit confirmation bias, I formulate a new specification that relates beliefs of increase in droughts to their interpretation. This approach empirically tests Eq. (3): individuals who display directional motivated reasoning distort the interpretation of new information as a function of their beliefs. Figure A4 provides stylized evidence of this mechanism. The frequency distribution of the measure \(\Delta \) for individuals holding beliefs that droughts have increased is more left-skewed than for individuals who do not hold such beliefs. The t test of a difference in means between the two samples is – 11.26: \(\Delta \) has an average of – 0.12 among the non-believers, and an average of 0.47 among the believers, implying a statistical difference between the two samples (p-value < 0.001). This is confirmed by a Kolmogorov-Smirnov test conducted under the null hypothesis of equal distribution of \(\Delta \) by beliefs, which I fail to accept (p-value < 0.001).
I design an econometric specification that uses as outcome both the probability and the extent to which individuals overestimate. The baseline equation writes
where \(\text {Belief}_{it}\) is the binary variable indicating whether individual i in survey wave t believes that droughts have increased over the past twenty years. Deviation\(_{ut}\) refers to the short-term deviation in dryness from the LT Exposure and \(\alpha _i\) and \(\lambda _t\) are individual and year fixed effects. Standard errors are clustered at the grid cell level.
Even when accounting for the fixed effects, the OLS regression may yield biased estimates of the effect of beliefs on accuracy for several reasons. First, individuals may alter their long-lasting beliefs after receiving new information and therefore beliefs could change as a consequence of the interpretation of drought events. Equation (5) may be subject to simultaneity bias and the estimates of the effect of beliefs on the interpretation of drought events would be biased downwards. Second, the estimate of the coefficient may also be biased because of classical measurement error. This would lead to an attenuation bias and thus \(\hat{\gamma }\) would again be biased towards zero. The errors in measurement of the belief may be correlated with the noise \(u_{it}\), which represents other unobservable determinants of outcomes, for example, poorer households might be more exposed to droughts but less able to assess damages accurately. Finally, other omitted time-varying individual-specific characteristics such as risk perceptions may be simultaneously correlated with changes in individuals’ beliefs about droughts and in the recollection of drought events.
To address the concerns on endogeneity, I adopt an instrumental variable approach using as an instrument the average long-term exposure to dryness over the previous twenty years. This variable complies with the two restrictions for a valid instrument. The variable is relevant as shown from the estimation of Eq. (4) (Table 1, column 3). A household frequently exposed to large extreme weather events and one not frequently exposed may differently interpret an event of the same magnitude (Guiteras et al. 2015). Average long-term exposure is expected to satisfy the exclusion restriction, by determining individuals’ interpretation of past drought events only through their beliefs about these events. The validity of the instrument and the identifying assumption is discussed below. Testing whether the interpretation of new information is tilted towards the beliefs provides evidence of confirmation bias if the estimated coefficient \(\hat{\gamma }\) is positive and statistically significant.
4.2.1 Identifying Assumption and Instrument Validity
In an OLS regression, the identification of the effect of beliefs on individuals’ interpretation of drought events is threatened by reverse causality, omitted variable bias and classical measurement error. To address these and similar concerns, I adopt an instrumental variable approach and use the twenty-year long-term average exposure to dryness as an instrument for beliefs of increase in droughts. The instrumental variable approach strengthens the causality argument under the exclusion restriction that exposure to dryness does not affect the accuracy of recollecting drought events via other channels besides beliefs.
The variation underlying the instrument, relative changes in long-term exposure to excess dryness, is plausibly as good as random and hence likely exogenous to within-individual variation over time. By retaining only variation in beliefs generated by the quasi-experimental variation in long-term dryness, this approach exploits the rational component of changes in beliefs estimated from variation in dryness exposure. If individuals did not exhibit confirmation bias, using an instrumental variable approach, beliefs should have a null effect on the interpretation of weather events.
There are three major concerns that may violate the exclusion restriction. In what follows, I describe additional tests that assuage concerns on its validity. First, variation in the instrument may have an indirect effect on self-reported evidence through the omitted variable of subjective well-being and mood (Mellon 2021). According to the empirical evidence on self-reported life satisfaction (Maddison and Rehdanz 2011), the estimates of beliefs on recollection of droughts may be downward biased. Droughts have a negative effect on happiness (Sekulova and van den Bergh 2013; Keshavarz and Karami 2012) and life satisfaction (Carroll et al. 2009), which could positively affect the overestimation of past weather events (Forgas et al. 2009) and thus threaten the exclusion restriction. Nevertheless, studies using an individual fixed-effect empirical setting (Feddersen et al. 2016) do not detect a relationship between climate and self-reported life satisfaction and find an effect close to zero. These findings allay potential concerns about the validity of the instrument.
Second, objective drought exposure may affect the individual’s recollection of past drought events through past adaptation. This concern would arise if past environmental conditions affected past actions, which would in turn impose “historical restraints” on current actions (Lemoine 2021). Households that adapted due to changes in dryness might experience fewer droughts, and thus underestimate them, than if they had not adapted. For this reason, this potential channel would bias downwards the 2SLS estimates. In order to allay the potential concern about the validity of the instrument, the econometric specification includes a history of transient shocks proxied by the short-term deviation from the long-term exposure to dryness. This should reduce the bias introduced by historical restraints. In Sect. 5, additional robustness checks show that the adaptation channel does not threaten the identification of the effect of beliefs.
Finally, the instrument may be positively correlated with the propensity of individuals to seek weather information and listen to weather forecasts. The literature exploring this channel uses internet search activity data to examine if local short-run weather fluctuations cause people to seek information about climate change, finding that they have an effect on search behavior (Choi et al. 2020), but not always consistent with the projected impacts of climate change (Lang 2014). The main difference between the previous findings and my design stands in the use of a long-term average in place of short-term fluctuations. The use of long-term exposure to dryness should allay the concern on its potential correlation with seeking information on climate change. Furthermore, if this channel existed, seeking and receiving more weather information would be negatively correlated with the recollection of drought events. A more informed individual would be able to reduce the distance between the self-reported and objective number of droughts. Therefore, this channel would underestimate the effect of beliefs on the overestimation of drought events. The survey does not contain explicit information on the individual use of weather information, however, in Sect. 5, I discuss additional robustness checks that assuage concerns about the validity of the instrument. Table A6 shows the correlations between the instrument and the additional controls included in the robustness exercises. Out of the ten estimates, I find that only one is statistically significant at the 10% level, which is consistent with sampling variation given the multiple tests carried out, thus strengthening the exogeneity hypothesis of the instrument.
5 Results
5.1 Drought Exposure, Beliefs and Accuracy
Table 1 displays the results for the effect of objective exposure to dryness on beliefs and recollection of drought events. Columns (1) and (2) separately investigate whether short-term deviations and long-term exposure predict self-reported beliefs and the measure of accuracy. Column (3) includes them both, and in column (4) I include their interaction, as in Eq. (4).
When considering the belief of increase in droughts, both the long-term average exposure (column 1) and the short-term deviation from the reference environment (column 2) have a positive statistically significant effect. The effect of long-term average exposure is more than ten times larger than the effect of short-term deviations. A one standard deviation (SD) increase in long-term exposure is associated with approximately a 1.2 SD increase in the probability of believing that droughts have increased over the previous twenty years (15.13\(\times \)0.04/0.5).Footnote 12 In contrast, a one SD increase in deviations from the average drought exposure increases the probability of believing in an increase in droughts by around 0.14 SD (0.729\(\times \)0.10/0.5). When considering the effect of both LT Exposure and Deviation in column (3) and including their interaction (column 4), only the coefficient associated with long-term exposure is statistically significant.
When regressing the probability of overestimating the number of drought events and the extent of overestimation on the full specification, the effect of short-term deviation and of the interaction term are not statistically different from zero (columns 8 and 12). Long-term exposure continues to have a sizeable positive and statistically significant effect on individuals’ overestimation of drought events across all specifications.
The findings suggest that objective exposure matters for climate change belief formation in Bangladesh. Long-term average exposure to dryness predicts beliefs about increases in droughts and overestimation of past droughts, whereas deviations from local average conditions do not matter. On the one hand, these results differ from previous findings that show that, although in a different geographical context, recent, local weather anomalies matter for the formation of climate change beliefs in the United States (Konisky et al. 2016; Kaufmann et al. 2017). On the other hand, these findings add empirical evidence to the result that the experience of a single drought event may not be enough to alter climate change beliefs and what matters is the average dryness condition in the long-term (Carlton et al. 2016). To corroborate this hypothesis, I regress beliefs on long-term average exposure and the average number of drought events experienced over the five years before the first wave and between the two waves including different types of droughts (moderate, severe, extreme). Table A7 shows that beliefs are only explained by long-term average exposure.
The results are robust to different estimation methods, using a logit method for the belief of increase in droughts and the probability of overestimating drought events (Table A8) and a Poisson method for the extent of overestimation (Table A9). The results are not specific to the cut-off used to compute the objective number of drought events. I re-estimate Eq. (4) including moderate and severe drought events (Tables A10 and A11). Long-term exposure to dryness has a positive and statistically significant effect on the extent of overestimating drought events (column 8). The coefficient is larger in magnitude when including also moderate droughts, smaller when considering severe droughts, but still larger than in the baseline specification in Table 1 that only records extreme droughts. An increase in long-term exposure makes the environment more drought-prone, affecting in primis the probability of a moderate drought event and thus increasing the probability of overestimating droughts.
Three additional tests check the robustness of the results to other measures of drought exposure. First, results are robust to measuring dryness and meteorological droughts using different time scales of the SPEI. Different time scales define the period considered over which water deficits accumulate. I replicate the baseline results using the SPEI-4, SPEI-6 and SPEI-12 that, respectively, account for water deficits accumulated in the previous four, six and twelve months (Table A12). I also rescale the SPEI monthly realizations relative to each respondent’s lifetime exposure to dryness conditions. To do so, I compute the individual-specific lifetime mean and standard deviation of dryness conditions using the SPEI and then normalize the SPEI monthly realizations used to construct long-term exposure and short-term deviations. This approach allows for the same SPEI realization in a given union to have different standardized probabilities with respect to each individual’s lifetime exposure. Table A13 shows that results for different SPEI time scales are robust, providing suggestive evidence that individuals perceive exposure and form beliefs relative to their average environment based on their lifetime exposure. Figure A5 shows that individual beliefs are relatively inelastic to short-term deviations and depend on the relative long-term average exposure compared to their lifetime experience. Finally, instead of obtaining union-specific values of SPEI based on zonal statistics, I interpolate gridded data based on the inverse squared distance from the union centroids using as distance cut-offs 40, 80 and 120 km. Baseline results hold constructing dryness measures based on this approach (Table A14).
I also restrict the focus to the respondents who (weakly) overestimate the number of past drought events (Table A15). The sample size drops down to around 100 observations when considering those who overestimate severe drought events, therefore while the precision of the estimates deteriorates, the coefficients on deviations are never statistically significant and the coefficients on long-term exposure remain fairly stable across the estimations.
5.2 Directional Motivated Reasoning
Next, I shift the focus to the relationship between beliefs and interpretation of drought events. I test the hypothesis that individuals adopt directional motivated reasoning and are subject to confirmation bias. Under this hypothesis, individuals’ interpretation of drought events would be biased towards their prior beliefs, such that holding beliefs that droughts have increased has a positive effect on the probability and extent of overestimating the number of droughts.
Since only beliefs of increase in droughts are recorded in the survey, I only focus on the overestimation of the number of past drought events. Further research should explore whether directional motivated reasoning is displayed also by individuals who hold beliefs about a decrease in droughts, leading to a biased underestimation of drought events.
Panel A of Table 2 reports the OLS (columns 1 and 2) and 2SLS (columns 3 and 4) estimates of Eq. (5) using as outcome the indicator that individuals overestimated the number of droughts (\(\Delta > 0\)) (columns 1 and 3), and the extent to which individuals overestimated droughts (\(\Delta \)) (columns 2 and 4). Panel B reports the first stage estimates of the instrumental variable approach. The coefficient associated with Belief is positive and strongly statistically significant in both the OLS and 2SLS specifications. Consistent with Proposition 2, the belief of an increase in droughts increases the likelihood of overestimating drought occurrence by about 80 p.p. (column 3). When exploiting the extent of overestimation, beliefs have a positive and statistically significant effect, increasing the overestimation by four (column 4).
The magnitude of the 2SLS coefficient associated with Belief is significantly larger than the OLS estimate both in the probability and extent of overestimation. One potential explanation is that the OLS estimates suffer from reverse causality and attenuation bias due to measurement error.Footnote 13 A second possibility is that the 2SLS estimation identifies a local average treatment effect (LATE) for individuals that were more exposed to variation in excess dryness and thus more likely to update their beliefs about increases in droughts and overestimate their number.
These results provide suggestive evidence that individuals adopt directional motivated reasoning when interpreting drought events. The information is distorted, and changes in the perception of information for a given objective information set are driven by individual beliefs. Figure 1 plots the cumulative distribution functions of the predicted values of the extent of overestimation from Eq. (5) for the two belief types. The gap between the two distributions shows that individuals with prior beliefs that droughts have increased exhibit confirmation bias and overestimate the number of drought events.

Notes: The figure shows the cumulative distribution function (CDF) for the extent of overestimation predicted from Eq. (5) using a 2SLS approach. The dashed green line shows the CDF for individuals in the estimation sample that did not report that droughts have increased. The solid red line shows the CDF for individuals in the estimation sample with a belief that droughts have increased. The extent of overestimation \(\Delta \) is computed as explained in Eq. (1): I use the cut-off for extreme drought events (SPEI \(\le \) – 2) to compute the number of objective drought events in a given union and subtract it from the number of self-reported drought events over the same time period
Differential cumulative distribution functions of predicted overestimation of droughts.
Same number of recorded droughts. Using objectively recorded droughts, all individuals within the same grid cell are exposed to the same set of objective information (the households in the sample do not change place of residence across the two waves). Any variation in the interpretation of droughts stems from asymmetric changes in beliefs. To corroborate this hypothesis, I restrict the sample to the unions that experienced the same objective number of drought events. This setting is similar to an experiment in which all participants are given the same objective evidence (with the clear difference that in this setting the evidence cannot be controlled by the experimenter).
Since there is substantial heterogeneity across unions in the number of extreme droughts experienced, this analysis can only be performed for the unions that have not experienced any extreme drought event.Footnote 14 With this restriction, all households in the sub-sample did not face any drought in the years before each survey wave, and any residual asymmetric variation in interpretation is explained by changes in individual beliefs (accounting for short-term deviation in excess dryness and individual-specific and year-specific fixed effects). The marginal effect of believing in an increase in drought increases the probability of overestimating droughts by 95 p.p. (Table A16). This result demonstrates that individuals with prior beliefs of increases in droughts, although not recently exposed to extreme droughts, will distort their interpretation and overestimate them.
Measures of drought exposure. I also test for the robustness of the results using different SPEI time scales to construct drought exposure based on different periods over which water deficits accumulate. Table A17 shows similar 2SLS estimates using the SPEI-4, SPEI-6 and SPEI-12. I also normalize the SPEI to individual-specific lifetime exposure to dryness conditions and obtain a robust positive effect of beliefs on the probability and the extent of overestimating droughts across different SPEI temporal scales (Table A18). Finally, the results are robust to the construction of drought exposure based on the interpolation of gridded data weighted by the inverse squared distance from the union centroids using as distance cut-offs 40, 80 and 120 km (Table A19).
Drought cut-offs. The findings are robust to different drought cut-offs to construct \(\Delta \) (Table A20). The effect of beliefs is larger including moderate droughts (14.03, column 3) and severe droughts (6.92, column 4), compared to the effect on recollection of extreme droughts (4.05, column 4 in Table 2). This result suggests that the more ambiguous the signal, the more the evidence is open to interpretation. This situation creates room for the learner to adopt directional motivated reasoning and interpret ambiguous new information as a reinforcement of prior beliefs (Agnew et al. 2018b, a). I also limit the analysis to the sub-sample of individuals who overestimate droughts using the cut-offs of severe and extreme droughts (Table A21). Using extreme drought events, the OLS and 2SLS coefficients are positive, suggesting that individuals distort their interpretation of information due to their beliefs and exhibit directional motivated reasoning.
Historical restraints. As discussed in Sect. 4.2.1, a potential threat to the validity of the instrument concerns individuals exposed to more harmful conditions of dryness who may be more prone to adapt. Past weather affects past actions (i.e., adaptation), imposing historical restraints on current actions (i.e., interpreting drought events). The inclusion of short-term deviations from long-term average exposure in the baseline specification as a measure of transient shock is a first way to allay the concerns about this potential threat (Lemoine 2021). Below, I discuss two additional robustness checks to deal with this concern.
Short-term deviations. First, I vary the definition of short-term deviations and include one-year and two-year lagged annual deviation measures from the long-term average exposure to dryness and I also extend the time horizon up to five years. Using a longer history of transient shocks reduces the bias introduced by “historical restraints” (Lemoine 2021). The coefficient associated with belief is consistently statistically significant across all specifications and larger in magnitude than in the baseline estimates (Table A22).
Adaptation. Second, I account for different measures at the union-level that proxy for variations in the cost of adaptation (Tables A23 and A24).Footnote 15 I include measures of the presence of different types of banks (state-owned Krishi bank, Commercial bank, Grameen bank, or any of the three) that could affect adaptation by relaxing households’ financial constraints (columns 1–4). Similarly, I include an indicator of the presence of agricultural extension or a block officer (column 5), which may alter the weather information set of households. I also account for access to electricity (column 6), which could facilitate the use of electricity-dependent irrigation techniques, and for the presence of a shop for fertilizers or pesticides (column 7), which may influence the input use in agricultural production. The coefficients on beliefs are consistently positive, with no considerable variations in magnitude. When including all controls in column (8), the estimates are larger in magnitude, suggesting that the baseline estimates could be underestimating the effect of beliefs.
Weather information. As explained in Sect. 4.2.1, the validity of the instrument may be threatened if individuals more exposed to dryness are more likely to seek weather information. This channel would downward bias the effect of beliefs since more informed individuals would be more accurate. Although the survey does not contain detailed information on the propensity of individuals to listen to weather forecasts, I use data on the type of information on agricultural practices that could relate to droughts received from extension agents and whether individuals receive information from other sources besides the extension worker, in particular TV, radio or newspapers.Footnote 16 When controlling for these variables, the 2SLS estimates of beliefs are positive, statistically significant and always larger in magnitude than the baseline estimates, suggesting that baseline estimates could be underestimating the true causal effect of beliefs (Table A25).
6 Discussion and Conclusion
Despite scientific consensus, beliefs about climate change and its causes vary widely across individuals, and awareness is still very low in the developing world (Lee et al. 2015). Understanding the determinants of beliefs and any potential biases that individuals may exhibit is essential for the design of more effective policies to help them adapt (Lemos et al. 2019). Particularly so for agricultural communities in developing countries, heavily exposed to the consequences of climate change and whose misinterpretation of weather signals may be considerably harmful. It is critical to understand if individuals misinterpret weather shocks because they lack information or because, instead of striving for accuracy, they pursue directional goals. Individuals may engage in motivated reasoning mechanisms when interpreting weather events and exhibit confirmation bias.
This paper studies the effect of dryness exposure on beliefs of increase in droughts and examines whether individuals adopt directional motivated reasoning in the interpretation of drought events. First, I investigate how long-term average exposure to dryness and short-term deviations affect beliefs and the accuracy of recollecting them, finding that only long-term average conditions matter. This result suggests that beliefs are longstanding, and hence shaped only by long-term conditions rather than short-term deviations and that one single drought event may not be enough to alter climate change beliefs. Second, I document that individuals engage in a form of directional motivated reasoning, adding the first empirical evidence in a developing country. Using an instrumental variable approach to tackle endogeneity concerns, I find that individuals distort the perception of information due to their beliefs. This result, robust to different specifications, provides suggestive evidence that individuals are subject to confirmation bias: they recollect and overweight evidence tilted towards their prior beliefs. From a normative perspective, individuals exhibiting motivated reasoning when it comes to slow-onset environmental changes suggest that policies should target individuals’ beliefs to avert ignoring information countering prior beliefs.
Despite recent advancements in accurate estimates of climate impacts accounting for adaptation benefits and costs across sectors (Carleton et al. 2022; Hultgren et al. 2022; Rode et al. 2021; Auffhammer 2022), the underlying conceptual framework still relies on perfectly informed and rational agents with unbiased beliefs measured by meteorological conditions (Deryugina and Hsiang 2017). This paper empirically shows for the first time that climate beliefs can exhibit directional motivated reasoning, in support of previous theoretical arguments diverging from Bayesian agents, with asymmetric distortion of objective information as a result of climate beliefs (Druckman and McGrath 2019). Integrating features of incomplete rationality of decision-makers and individual distortion of weather signals based on prior beliefs can have substantial consequences on climate impact estimates accounting for individual endogenous choices of adaptation.
These findings shed light on a cognitive bias that distorts the mental representation of climate change and may subsequently lead to erroneous interpretation of climate change consequences and prevent or facilitate behavioral responses (Zappalà 2023). Drought frequency in Bangladesh is projected to increase in the future, particularly in regions historically considered less prone to droughts (Mohsenipour et al. 2018). Since beliefs are formed on long-run exposure rather than short-term deviations and drive the interpretation of weather events as a result of motivated reasoning, if individuals do not update them, they may not put in place timely adaptation to avert climate damage. Understanding how household beliefs about climate impact and cognitive biases impact adaptive decisions remain interesting questions for future work.
Against this background, it is essential to identify the nature of the bias to propose adequate debiasing tools for effective policies. A solution proposed by Zhao and Luo (2021) involves forward-looking techniques generating arguments for forward-looking options. Accurate information on historical and projected changes in climate may shape individuals’ beliefs on climate change consequences and foster behavioral responses to put in place timely adaptation. Further work should focus on the role of information interventions exogenously varying the information set, and assessing how these affect beliefs and influence cognitive biases.
A limitation of this study opens avenues for future research. The data do not allow to test for the presence of directional motivated reasoning and confirmation bias among those who believe that droughts have decreased over time. Testing whether this prior belief, commonly associated with climate change deniers’ or climate skeptics’ position, leads to biased interpretation of weather events underestimating them, would be of particular interest.
Data Availability
All data used in this analysis are compiled from free, publicly available sources.
Notes
Unions are the smallest rural administrative and local government unit in Bangladesh. Administrative units are structured as follows: Division \(\supset \) District (Zila) \(\supset \) Sub-district (Upazila) \(\supset \) Union. There are 5158 unions, that have an average size of approximately 10–20 km\(^2\).
The remaining 34 households could not be interviewed because they migrated (15 households) or were not at home at the time of the survey.
From the BCCAS, I also collect individual and union characteristics from the community questionnaire that I use in subsequent robustness exercises.
The underlying assumption is that individuals do not differentially change their internal definition of droughts between the two survey waves.
For ease of interpretation of the coefficients in the empirical analysis, these measures are taken in their additive inverse form, meaning that higher values are associated with drier conditions.
Since the survey does not provide information on the place of residence of the respondents over the twenty years before the first wave, I assume they have not moved and have been exposed to the union-average dryness conditions.
This methodology is adopted in order to create a continuous measure of wave-specific variation in exposure to dryness that matches the time period covered by the self-reported number of drought events in the BCCAS.
The choice of the time periods mirrors the time period covered by the survey questions on the number of drought events experienced.
Despite the recurrent and devastating nature of droughts, previous studies in Bangladesh have more often focused on floods (Guiteras et al. 2015; Gray and Mueller 2012; Chen et al. 2017). In spite of data availability on individual beliefs and personal experience of floods in the survey, I focus on droughts since there exist meteorological measures both of exposure to dryness and drought events. Rainfall measures have been shown to be weak proxies for flood exposure, and flood extent is nowadays commonly measured using remote-sensing data from the NASA Moderate Resolution Imaging Spectroradiometer (MODIS) (Guiteras et al. 2015; Chen et al. 2017). Nevertheless, differently from drought event recording, to the best of my knowledge, there is no classification for the meteorological number of flood events.
Union, or grid-cell, fixed effects are superfluous since all individuals in the estimation sample never change place of residence and therefore union-specific unobserved heterogeneity is taken into account by individual-specific fixed effects.
Respondents’ median age is 45. Baseline results are robust if excluding individuals below 30 years old (around 7% of the sample).
In an OLS setting undermined by reverse causality, the coefficient associated with beliefs would be biased downwards, and under the classical error-in-variables assumption, OLS estimates would suffer from attenuation bias due to measurement error. As shown in Panel B of Table 2, long-term average exposure to dryness has a positive, significant effect on belief and the Kleibergen–Paap (K–P) Wald F-statistic for weak identification is 21.736, higher than any critical value reported by Stock and Yogo (2005).
Since the SPEI values are normally distributed, extreme drought events (SPEI \(\le -2\)) account for about 2.3% of all available historical values. On average, such SPEI values would then be recorded once every 44 months, explaining why no extreme droughts is the only case that brings together several unions.
I use the community questionnaire that asks questions regarding each village. Table A2 reports the exact wording and formulation of each question in the two waves.
I use the household questionnaire (module M) to construct different versions of a dummy variable of receiving information on soil and water conservation, crop protection, new crop varieties and crop utilization and a binary variable on the sources of information among which TV, radio and newspapers. Table A2 reports the exact wording and formulation of each question in the two waves.
References
Agnew JR, Bateman H, Eckert C, Iskhakov F, Louviere JJ, Thorp S (2018a) First impressions matter: an experimental investigation of online financial advice. Manag Sci 64(1):288–307
Agnew JR, Bateman H, Eckert C, Iskhakov F, Louviere JJ, Thorp S (2018b) Learning and confirmation bias: measuring the impact of first impressions and ambiguous signals. Wharton pension research council working paper, 15
Alamgir M, Shahid S, Hazarika MK, Nashrrullah S, Harun SB, Shamsudin S (2015) Analysis of meteorological drought pattern during different climatic and cropping seasons in Bangladesh. J Am Water Resour Assoc 51(3):794–806
Arbuckle JG, Morton LW, Hobbs J (2013a) Farmer beliefs and concerns about climate change and attitudes toward adaptation and mitigation: evidence from Iowa. Clim Change 118(3):551–563
Arbuckle JG, Prokopy LS, Haigh T, Hobbs J, Knoot T, Knutson C, Loy A, Mase AS, McGuire J, Morton LW, Tyndall J, Widhalm M (2013b) Climate change beliefs, concerns, and attitudes toward adaptation and mitigation among farmers in the Midwestern United States. Clim Change 117(4):943–950
Auffhammer M (2022) Climate adaptive response estimation: short and long run impacts of climate change on residential electricity and natural gas consumption. J Environ Econ Manag 114:102669
Auffhammer M, Carleton T (2018) Regional crop diversity and weather shocks in India. Asian Dev Rev 35(2):113–130
Auffhammer M, Schlenker W (2014) Empirical studies on agricultural impacts and adaptation. Energy Econ 46:555–561
Beattie G, Han Y, La Nauze A (2019) Conservation spillovers: the effect of rooftop solar on climate change beliefs. Environ Resource Econ 74(3):1425–1451
Bento A, Miller NS, Mookerjee M, Severnini ER (2020) A unifying approach to measuring climate change impacts and adaptation (NBER Working Paper No. 27247). National Bureau of Economic Research
Carleton T, Hsiang S (2016) Social and economic impacts of climate. Science 353:6304
Carleton T, Jina A, Delgado M, Greenstone M, Houser T, Hsiang S, Hultgren A, Kopp RE, McCusker KE, Nath I et al (2022) Valuing the global mortality consequences of climate change accounting for adaptation costs and benefits. Q J Econ 137(4):2037–2105
Carlsson F, Kataria M, Krupnick A, Lampi E, Löfgren Å, Qin P, Sterner T, Yang X (2021) The climate decade: changing attitudes on three continents. J Environ Econ Manag 107:102426
Carlton JS, Mase AS, Knutson CL, Lemos MC, Haigh T, Todey DP, Prokopy LS (2016) The effects of extreme drought on climate change beliefs, risk perceptions, and adaptation attitudes. Clim Change 135(2):211–226
Carroll N, Frijters P, Shields MA (2009) Quantifying the costs of drought: new evidence from life satisfaction data. J Popul Econ 22(2):445–461
Chen JJ, Mueller V, Jia Y, Tseng SK-H (2017) Validating migration responses to flooding using satellite and vital registration data. Am Econ Rev 107(5):441–45
Choi D, Gao Z, Jiang W (2020) Attention to global warming. Rev Financ Stud 33(3):1112–1145
Czarnek G, Kossowska M, Szwed P (2021) Right-wing ideology reduces the effects of education on climate change beliefs in more developed countries. Nat Clim Chang 11(1):9–13
Delaporte I, Maurel M (2018) Adaptation to climate change in Bangladesh. Clim Policy 18(1):49–62
Deryugina T (2013) How do people update? The effects of local weather fluctuations on beliefs about global warming. Clim Change 118(2):397–416
Deryugina T, Hsiang S (2017) The marginal product of climate (NBER working paper no. 24072). National Bureau of Economic Research
Druckman JN, McGrath MC (2019) The evidence for motivated reasoning in climate change preference formation. Nat Clim Chang 9(2):111–119
Faia E, Fuster A, Pezone V, Zafar B (2021). Biases in information selection and processing: Survey evidence from the pandemic (NBER working paper no. 28484). National Bureau of Economic Research
Feddersen J, Metcalfe R, Wooden M (2016) Subjective wellbeing: why weather matters. J R Stat Soc A Stat Soc 179(1):203–228
Forgas JP, Goldenberg L, Unkelbach C (2009) Can bad weather improve your memory? An unobtrusive field study of natural mood effects on real-life memory. J Exp Soc Psychol 45(1):254–257
Fryer RG, Harms P, Jackson MO (2019) Updating beliefs when evidence is open to interpretation: implications for bias and polarization. J Eur Econ Assoc 17(5):1470–1501
GADM (2021) GADM database of Global Administrative Areas, version 3.6. www.gadm.org
Gallagher J (2014) Learning about an infrequent event: evidence from flood insurance take-up in the United States. Appl Econ Am Econ J 206–233
Goebbert K, Jenkins-Smith HC, Klockow K, Nowlin MC, Silva CL (2012) Weather, climate, and worldviews: the sources and consequences of public perceptions of changes in local weather patterns. Weather Clim Soc 4(2):132–144
Gramig BM, Barnard JM, Prokopy LS (2013) Farmer beliefs about climate change and carbon sequestration incentives. Clim Res 56(2):157–167
Gray CL, Mueller V (2012) Natural disasters and population mobility in Bangladesh. Proc Natl Acad Sci 109(16):6000–6005
Guiteras R, Jina A, Mobarak AM (2015) Satellites, self-reports, and submersion: exposure to floods in Bangladesh. Am Econ Rev 105(5):232–36
Hansen J, Sato M, Ruedy R (2012) Perception of climate change. Proc Natl Acad Sci 109(37):E2415–E2423
Hoffmann R, Muttarak R, Peisker J, Stanig P (2022) Climate change experiences raise environmental concerns and promote green voting. Nat Clim Chang 12(2):148–155
Howe PD, Boudet H, Leiserowitz A, Maibach EW (2014) Mapping the shadow of experience of extreme weather events. Clim Change 127(2):381–389
Howe PD, Leiserowitz A (2013) Who remembers a hot summer or a cold winter? The asymmetric effect of beliefs about global warming on perceptions of local climate conditions in the US. Glob Environ Chang 23(6):1488–1500
Hsiang S (2016) Climate econometrics. Annu Rev Resour Econ 8(1):43–75
Hsiang S, Jina A (2014) The causal effect of environmental catastrophe on long-run economic growth: evidence from 6,700 cyclones (NBER working paper no. 20352). National Bureau of Economic Research
Hultgren A, Carleton T, Delgado M, Gergel DR, Greenstone M, Houser T, Hsiang S, Jina A, Kopp RE, Malevich SB, et al (2022) Estimating global impacts to agriculture from climate change accounting for adaptation. Available at SSRN https://ssrn.com/abstract=4222020
International Food Policy Research Institute (2014a) Bangladesh Climate Change Adaptation Survey (BCCAS). Round I. https://doi.org/10.7910/DVN/27704
International Food Policy Research Institute (2014b) Bangladesh Climate Change Adaptation Survey (BCCAS). Round II. https://doi.org/10.7910/DVN/27883
IPCC (2021) Climate Change 2021: the physical science basis. Contribution of working group i to the sixth assessment report of the intergovernmental panel on climate change. Cambridge University Press
Joireman J, Truelove HB, Duell B (2010) Effect of outdoor temperature, heat primes and anchoring on belief in global warming. J Environ Psychol 30(4):358–367
Kahneman D, Tversky A (1982) Judgment under uncertainty: heuristics and biases. Cambridge University Press, Cambridge
Kala N (2017) Learning, adaptation, and climate uncertainty: evidence from Indian agriculture. MIT Center for Energy and Environmental Policy Research working paper, 23
Kaufmann RK, Mann ML, Gopal S, Liederman JA, Howe PD, Pretis F, Tang X, Gilmore M (2017) Spatial heterogeneity of climate change as an experiential basis for skepticism. Proc Natl Acad Sci 114(1):67–71
Keshavarz M, Karami E (2012) Drought and happiness in rural Iran. J Soil Sci Environ Manag 3(3):66–76
Konisky DM, Hughes L, Kaylor CH (2016) Extreme weather events and climate change concern. Clim Change 134(4):533–547
Lang C (2014) Do weather fluctuations cause people to seek information about climate change? Clim Change 125(3):291–303
Lee TM, Markowitz EM, Howe PD, Ko C-Y, Leiserowitz AA (2015) Predictors of public climate change awareness and risk perception around the world. Nat Clim Chang 5(11):1014–1020
Lemoine D (2021) Estimating the consequences of climate change from variation in weather (NBER working paper no. 25008). National Bureau of Economic Research
Lemos MC, Eakin H, Dilling L, Worl J (2019) Social sciences, weather, and climate change. Meteorol Monogr 59:26–1
Li Y, Johnson EJ, Zaval L (2011) Local warming: daily temperature change influences belief in global warming. Psychol Sci 22(4):454–459
Lodge M, Taber CS (2013) The rationalizing voter. Cambridge University Press, Cambridge
Maddison D, Rehdanz K (2011) The impact of climate on life satisfaction. Ecol Econ 70(12):2437–2445
McCright AM, Dunlap RE, Xiao C (2014) The impacts of temperature anomalies and political orientation on perceived winter warming. Nat Clim Chang 4(12):1077–1081
McKee TB, Doesken NJ, Kleist J, et al (1993) The relationship of drought frequency and duration to time scales. In: Proceedings of the 8th conference on applied climatology, vol 17, No. 22, pp 179–183
Mellon J (2021) Rain, rain, go away: 176 potential exclusion-restriction violations for studies using weather as an instrumental variable. https://doi.org/10.2139/ssrn.3715610
Mertz O, Halsnæs K, Olesen JE, Rasmussen K (2009) Adaptation to climate change in developing countries. Environ Manag 43(5):743–752
Mohsenipour M, Shahid S, Chung E-S, Wang X-J (2018) Changing pattern of droughts during cropping seasons of Bangladesh. Water Resour Manag 32(5):1555–1568
Moore FC, Obradovich N, Lehner F, Baylis P (2019) Rapidly declining remarkability of temperature anomalies may obscure public perception of climate change. Proc Natl Acad Sci 116(11):4905–4910
Myers TA, Maibach EW, Roser-Renouf C, Akerlof K, Leiserowitz AA (2013) The relationship between personal experience and belief in the reality of global warming. Nat Clim Chang 3(4):343–347
Oreskes N (2004) The scientific consensus on climate change. Science 306(5702):1686–1686
Osberghaus D, Fugger C (2022) Natural disasters and climate change beliefs: the role of distance and prior beliefs. Glob Environ Chang 74:102515
Palm R, Lewis GB, Feng B (2017) What causes people to change their opinion about climate change? Ann Am Assoc Geogr 107(4):883–896
Paulo A, Rosa R, Pereira L (2012) Climate trends and behaviour of drought indices based on precipitation and evapotranspiration in Portugal. Nat Hazard 12(5):1481–1491
Poortinga W, Whitmarsh L, Steg L, Böhm G, Fisher S (2019) Climate change perceptions and their individual-level determinants: a cross-European analysis. Glob Environ Chang 55:25–35
Rabin M, Schrag JL (1999) First impressions matter: a model of confirmatory bias. Q J Econ 114(1):37–82
Rejesus RM, Mutuc-Hensley M, Mitchell PD, Coble KH, Knight TO (2013) US agricultural producer perceptions of climate change. J Agric Appl Econ 45(4):701–718
Rode A, Carleton T, Delgado M, Greenstone M, Houser T, Hsiang S, Hultgren A, Jina A, Kopp RE, McCusker KE et al (2021) Estimating a social cost of carbon for global energy consumption. Nature 598(7880):308–314
Rzepa A, Ray J (2020) World risk poll reveals global threat from climate change (Gallup Blog, Ed.) [News.Gallup.com [Online; posted 6-October-2020; Accessed on 1-March-2023]]
Sekulova F, van den Bergh JC (2013) Climate change, income and happiness: an empirical study for Barcelona. Glob Environ Chang 23(6):1467–1475
Shahid S (2011) Spatial assessment of drought hazard, vulnerability and risk in Bangladesh. In: Neves FD, Sanz JD (eds) Droughts: new research. Nova Science Pub Inc., New York, pp 215–241
Shao W (2016) Are actual weather and perceived weather the same? Understanding perceptions of local weather and their effects on risk perceptions of global warming. J Risk Res 19(6):722–742
Spinoni J, Naumann G, Carrao H, Barbosa P, Vogt J (2014) World drought frequency, duration, and severity for 1951–2010. Int J Climatol 34(8):2792–2804
Stahlmann-Brown P, Walsh P (2022) Soil moisture and expectations regarding future climate: evidence from panel data. Clim Change 171(1):1–20
Stock JH, Yogo M (2005) Testing for weak instruments in linear IV regression. In: Andrews DWK, Stock JH (eds) Identification and inference for econometric models: essays in Honor of Thomas Rothenberg. Cambridge University Press, Cambridge, pp 80–108
Vicente-Serrano SM, Beguerýa S, López-Moreno JI (2010) A multiscalar drought index sensitive to global warming: the standardized precipitation evapotranspiration Index. J Clim 23(7):1696–1718
Weber EU (1997) Perception and expectation of climate change: precondition for economic and technological adaptation. In: Bazerman M, Messick D, Tenbrunsel A, Wade-Benzoni K (eds) Psychological perspectives to environmental and ethical issues in management. Jossey-Bass, San Francisco, pp 314–341
Weber EU (2010) What shapes perceptions of climate change? Wiley Interdiscip Rev Clim Change 1(3):332–342
Zanocco C, Boudet H, Nilson R, Satein H, Whitley H, Flora J (2018) Place, proximity, and perceived harm: extreme weather events and views about climate change. Clim Change 149(3):349–365
Zappalà G (2023) Adapting to climate change accounting for individual beliefs. Mimeo
Zargar A, Sadiq R, Naser B, Khan FI (2011) A review of drought indices. Environ Rev 19:333–349
Zaval L, Keenan EA, Johnson EJ, Weber EU (2014) How warm days increase belief in global warming. Nat Clim Chang 4(2):143–147
Zhao J, Luo Y (2021) A framework to address cognitive biases of climate change. Neuron 109(22):3548–3551
Acknowledgements
I am extremely grateful to Katrin Millock for invaluable advice throughout this project. I am thankful to Tamma Carleton for her hospitality and guidance during my stay at UC Santa Barbara. I thank Andrew Clark, Tatyana Deryugina, Fabrice Etilé, Nicolas Jacquemet, Namrata Kala and an anonymous referee for the FAERE Working Paper Series for their useful comments, as well as seminar participants at the Paris School of Economics. I am also indebted to the Editor and two anonymous referees for their insightful feedback. All remaining errors are mine.
Funding
This work has been funded by a French government subsidy managed by the Agence Nationale de la Recherche under the framework of the Investissements d’avenir programme (ANR-17-EURE-001) and by the Université Paris 1 Panthéon-Sorbonne Economics Doctoral School (ED 465).
Author information
Authors and Affiliations
Corresponding author
Ethics declarations
Conflict of interest
The author has no competing interests to declare that are relevant to the content of this article.
Additional information
Publisher's Note
Springer Nature remains neutral with regard to jurisdictional claims in published maps and institutional affiliations.
Supplementary Information
Below is the link to the electronic supplementary material.
Rights and permissions
Springer Nature or its licensor (e.g. a society or other partner) holds exclusive rights to this article under a publishing agreement with the author(s) or other rightsholder(s); author self-archiving of the accepted manuscript version of this article is solely governed by the terms of such publishing agreement and applicable law.
About this article
Cite this article
Zappalà, G. Drought Exposure and Accuracy: Motivated Reasoning in Climate Change Beliefs. Environ Resource Econ 85, 649–672 (2023). https://doi.org/10.1007/s10640-023-00779-1
Accepted:
Published:
Issue Date:
DOI: https://doi.org/10.1007/s10640-023-00779-1