Abstract
Variations in continental water storage lead to loading deformation of the crust with typical peak-to-peak variations at very long baseline interferometry (VLBI) sites of 3–15 mm in the vertical component and 1–2 mm in the horizontal component. The hydrology signal at VLBI sites has annual and semi-annual components and clear interannual variations. We have calculated the hydrology loading series using mass loading distributions derived from the global land data assimilation system (GLDAS) hydrology model and alternatively from a global grid of equal-area gravity recovery and climate experiment (GRACE) mascons. In the analysis of the two weekly VLBI 24-h R1 and R4 network sessions from 2003 to 2010 the baseline length repeatabilities are reduced in 79 % (80 %) of baselines when GLDAS (GRACE) loading corrections are applied. Site vertical coordinate repeatabilities are reduced in about 80 % of the sites when either GLDAS or GRACE loading is used. In the horizontal components, reduction occurs in 70–80 % of the sites. Estimates of the annual site vertical amplitudes were reduced for 16 out of 18 sites if either loading series was applied. We estimated loading admittance factors for each site and found that the average admittances were 1.01 \(\pm \) 0.05 for GRACE and 1.39 \(\pm \) 0.07 for GLDAS. The standard deviations of the GRACE admittances and GLDAS admittances were 0.31 and 0.68, respectively. For sites that have been observed in a set of sufficiently temporally dense daily sessions, the average correlation between VLBI vertical monthly averaged series and GLDAS or GRACE loading series was 0.47 and 0.43, respectively.
Similar content being viewed by others
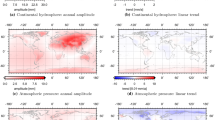
Avoid common mistakes on your manuscript.
1 Introduction
Very long baseline interferometry (VLBI) and global positioning system (GPS) measurements are precise enough to detect surface mass loading effects. Various geophysical problems, such as the determination of global sea-level rise or postglacial rebound, require uncertainties in station position determination at the 1 mm level. To be able to address these and other geophysical problems, we need to account for mass loading effects in geodetic analysis. Many investigators have demonstrated the presence of atmospheric pressure loading in geodetic measurements (see for instance, Dam and Herring 1994; van Dam et al. 1994; MacMillan and Gipson 1994; Petrov and Boy 2004). In this paper, we investigate the response of the surface of the Earth caused by variations in continental water storage. The variations in water storage are typically seasonal, but interannual variations are also evident in the site hydrology loading time series. These variations cause vertical displacements (see Sect. 3) of up to 2 cm depending on the Earth’s location and are large enough to be seen by space geodetic techniques.
Dam et al. (2001) investigated GPS site displacements caused by continental water loading. They convolved a loading Green’s function (Farrell 1972) with the mass loading field given by the Milly and Shmakin (2002) model and found that vertical displacements could be as large as 15 mm at some GPS sites. Subtracting these loading series from the observed GPS site position time series reduced the variance of the series. Over all sites, the average loading admittance squared (here, the response given by the site position series variance reduction divided by the variance of the loading signal) was close to unity, but there was a large admittance variation over the set of sites analyzed. Annual amplitudes of GPS site position series were reduced when the model series were applied. Schuh et al. (2003) investigated the effect of applying the sum of pressure loading, hydrology loading, and nontidal ocean loading in VLBI analysis; however, they did not consider the effect of the loading components separately. MacMillan and Boy (2004) used the Milly–Shmakin model to compute loading and found a reduction in baseline length scatter and site vertical annual amplitudes when the hydrology loading contribution was applied in VLBI analysis.
There have been a number of studies looking at the correlation between GPS position time series and gravity recovery and climate experiment (GRACE) measured continental hydrology signals. GRACE monitors the integrated effect of mass redistribution by measuring the temporal and spatial variations of Earth’s gravity field. Davis et al. (2004) determined the annual vertical deformation from the annual variation of the GRACE Stokes coefficients. They found good agreement in the region of the Amazon River basin, where the signal is very large, between annual vertical amplitudes for a set of GPS sites and the vertical deformation inferred from GRACE. vanDam et al. (2007) compared the vertical variation determined from European site GPS receivers with the variation inferred from GRACE and found that the annual signal in GPS heights was much less spatially coherent than heights inferred from GRACE. They concluded that disagreements between GPS and GRACE annual signals were caused by site or network-specific GPS technique errors, including possible troposphere mismodeling, bedrock thermal expansion, monument thermal expansion, phase modeling, and orbit errors. Tregoning et al. (2009) compared the variation from GPS topocentric site positions with the variation determined from GRACE and found good correlation in the vertical, especially for European sites, which they attributed to their improved GPS series compared with those used by vanDam et al. (2007). They applied pressure loading modeling for both GRACE and GPS to isolate the effect of the hydrology loading signal from GRACE. Tesmer et al. (2011) used completely reprocessed GPS data with state-of-the-art models and found good agreement between height coordinate time series and displacements derived from GRACE gravity coefficients. However, they did not remove the atmospheric pressure loading signal from either the GRACE or GPS displacements. They found that in 80 % of sites where the GRACE signal is larger than its precision, the vertical repeatability is reduced when monthly GRACE loading displacements are removed from the GPS vertical series.
In this paper we investigate the response of the surface of the Earth, measured by VLBI, caused by variations in continental water storage. In Sect. 2, we discuss the two hydrology data sets that we used to compute loading displacements. The first is the set of National Aeronautics and Space Administration (NASA) Goddard Space Flight Center (GSFC) GRACE mascons, described by Rowlands et al. (2005). The second dataset is the Noah hydrology model from the global land data assimilation system (GLDAS) (Rodell 2004). We computed vertical and horizontal hydrology loading series by convolving loading Green’s functions with the surface mass fields from these datasets. We describe the method for generating the site loading series and the characteristics of the derived series in Sect. 3. In Sect. 4, we discuss the results and improvements in VLBI analysis when the hydrology loading series are applied.
2 Hydrology datasets used
2.1 GRACE mascon solution
We computed the GRACE deformation series from the NASA GSFC mascons (Rowlands et al. 2005), which were computed using high-precision gravity field data measured by the GRACE satellite. Data are available for our investigation from 2003, near the beginning of the GRACE mission until 2010. GRACE mascon data are provided for \(2^{\circ }\) \(\times \) \(2^{\circ }\) equal area grid blocks with 10-day time resolution. It is assumed that the gravity change from each block is due to a thin uniform layer of mass, called a mascon, over the block. One estimates the surplus or deficit of mass of the mascon from inter-satellite K-band range-rate observations measured directly over the block of interest. The GRACE GSFC mascons were generated employing forward modeling for atmospheric pressure variation, non-barotropic ocean response and ocean tides. The mascon method should minimize the propagation of errors arising from mismodeling of other regions that are problematic for the alternative global spherical harmonic expansion approach (see Sabaka et al. 2010).
2.2 GLDAS hydrology model
The GLDAS Noah model, developed by Rodell (2004), uses data assimilation techniques and consists of land surface models that are constrained by observed meteorological fields. GLDAS Noah model output fields relevant to our investigation are soil moisture, snow water equivalent, and plant canopy surface water storage. The model is available at various resolutions, starting from a \(0.25^{\circ }\) \(\times \) \(0.25^{\circ }\) global angular grid with a monthly or 3-h time resolution. We did not find a significant difference between using the Noah model with a 3-h time resolution over using the monthly averaged model due to the fact that the series are very smooth. We therefore used the Noah model with monthly time resolution and a \(1^{\circ } \times 1^{\circ }\) spatial resolution since only this spatial resolution was available from 1980, the earliest date of the VLBI data that we analyze in standard solutions.
Since the GLDAS model does not account for ice sheet processes, we followed the recommendation of Rodell (2004) and masked out areas with permanent frost in our loading calculations. Most of the pixels that have permanent frost are located at high northern or southern latitudes, but there are also such pixels in regions with high mountains such as the Himalayas. We calculated the loading using a land–sea mask that removed ocean, sea, and lake pixels. Pixels with permanent frost were also removed for both GRACE and GLDAS to avoid any ice-loading contribution and allow a fair comparison.
2.3 Comparison of GLDAS and GRACE mass fields
The upper plot in Fig. 1 shows the standard deviation of hydrological mass expressed in centimeters of water. The GRACE mascons generally show the largest variation in the same areas. The lower plot shows for each pixel the difference between standard deviations of the variations of each dataset. The hydrologic variation is the largest within a 40\(^\circ \) latitude band about the equator in South America, South Asia, and Africa. Although areas in Europe and North America show smaller mass variation, the resulting hydrology loading signal is still significant. The differences between GRACE and GLDAS can reach 20–30 % in South America and Africa. Differences in North America and Europe are at the 10 % level. Groundwater and surface water are not included in the GLDAS model, but groundwater and surface water mass must be detected by GRACE since it is sensitive to the total mass change. This may explain some of the differences between the GRACE and GLDAS signals seen in Fig. 1. In one of many studies, Syed et al. (2008) have compared GLDAS and GRACE water storage change estimates and note the overall agreement in spatial and temporal variability. They attribute differences to the lack of surface water and groundwater modeling in GLDAS and to possible snow modeling deficiencies of the GLDAS model.
3 Site displacement series
In this section, we first summarize our calculation of loading using the usual Green’s function approach and then discuss error sources involved in the calculations. We then look at the characteristics of loading series at specific sites as well as the global variation of hydrology loading derived from GLDAS and GRACE data.
3.1 The Green’s function approach
The surface deformation of the Earth at a given VLBI site is the integrated effect of the deformation due to each source of mass change on the surface of the Earth. We calculated the site loading displacement by convolving an elastic loading Green’s function with the surface mass loading distribution (see Farrell 1972 for a discussion of the loading problem and the calculation of the Green’s function). We performed the convolution over the mass distributions from the GLDAS Noah model and from the GRACE mascon data gridblocks. Petrov and Boy (2004) provide convolution expressions for the vertical and horizontal loading at a site. We have used the same Green’s functions that were used by Petrov and Boy (2004) for atmospheric pressure loading. We used a point-mass representation to compute the convolution where we refined the grid more finely (to as small as \(0.001^{\circ } \times 0.001^{\circ }\)) the closer a mass load cell is to the station location in order to account for the singularity of the Green’s function at zero separation.
Depending on what geodetic technique is being used, loading displacements need to be in the appropriate reference frame. VLBI is a differential technique with a baseline observable and is therefore insensitive to the geocenter. We applied loading displacement series generated with a Green’s function such that the displacements are with respect to the center of the mass of the solid Earth plus the mass that causes the loading. Since VLBI is a differential technique and the displacements are applied at the observation level, we could alternatively have applied displacements with respect to the center of mass of the solid Earth and obtained the same results.
3.2 Loading series uncertainties
Since the GRACE mascons that are given at 10-day resolution are quite noisy, we smoothed our original GRACE 10-day loading series using a 10-day Gaussian filter. The noise in the 10-day mascons is due to sampling errors, the power in the spatial and temporal constraints, errors of the observation data, and errors in forward models (see Luthcke et al. 2013). For an estimate of the loading displacement uncertainty, we computed the root-mean-squared (RMS) difference between the original 10-day series and the smoothed hydrology loading series (recommended by D. Rowlands, personal communication, 2012). Based on these RMS differences, the error for points in the smoothed vertical loading series for most sites range from 0.8 to 1.2 mm. For comparison, we note that (Tesmer et al. 2011) estimated an error of 1.2 mm for their monthly GRACE loading series (computed from GRACE-derived Stokes coefficients) by using the difference between gravity solutions from two different analysis centers.
Kato et al. (2007) compared the output parameters of three models in the GLDAS family of land surface models to observed values at four sites. The standard deviation of soil moisture output from the three models was on average 25 % of the mean. This standard deviation was typically as large as or larger than the difference between a model and observed values of soil moisture, so that the standard deviation is a conservative estimate of the model error (M. Rodell, personal communication, 2013). Global averages of the terrestrial water storage change are divided nearly equally between soil moisture and snow water equivalent; soil moisture changes are clearly greater at low latitudes and snow water equivalent changes are greater at high latitudes (Syed et al. 2008). Since soil moisture is a large component of the loading, we take this as a crude measure of the error in GLDAS loading series. Based on the RMS GLDAS vertical variation for our sites in Table 1 and a model error of 25 %, the error in the GLDAS loading series ranges from 0.5 to 0.9 mm. As an alternative estimate of GLDAS error, we computed the loading series from an alternative GLDAS model, the variable infiltration capacity (Vic) model developed by Liang and Lettenmaier (1994). Assuming that the Noah and Vic models have the same RMS error relative to the truth and that their errors are uncorrelated, we estimated the error of each model from the differences between the models. The resulting vertical errors range from 0.6 to 1.2 mm depending on the site.
The main source of error that can affect the calculation of the hydrology loading series are the errors in the GRACE mascon fields or the GLDAS Noah hydrology model surface mass fields. We estimate that the sum of land–sea mask errors, errors in the Green’s function, and errors in the convolution calculation contribute no more than 4 % to the errors of the loading series, which is negligible compared with uncertainties in the mass fields. Based on the above estimates of the the uncertainties in the GLDAS and GRACE mass fields, the overall uncertainties in the vertical hydrology loading series are in the range of 0.6–1.2 mm depending on the site.
3.3 Characteristics of hydrology loading displacements
The three-dimensional loading displacements are largest in the vertical direction. Peak-to-peak loading displacements at VLBI sites, which have a characteristic annual period, are 3–15 mm in the vertical and 1–3 mm in the horizontal. Figure 2 shows two representative midlatitude site loading series from the GRACE period, 2003–2010. The loading series shown are smoothed 10-day series for two inland sites: Wettzell, Germany, and Hartebeesthoek, South Africa. Since both sites are inland sites, the loading series will have little sensitivity to errors in the land–sea mask. It can be seen in Fig. 2 that the hydrology loading series for both sites are clearly seasonal. The peak-to-peak variation of the loading series is about 8 mm for Hartrao and 10 mm for Wettzell and is therefore large enough to be seen in the VLBI analysis. The vertical loading series for Hartrao has interannual variations and an annual signal that is not as strong as it is for Wettzell. The amplitude is smaller before 2006 and the loading signal is missing a peak in 2007.
These loading series can be compared to Figure 1 and 2 in Petrov and Boy (2004), which show the displacements for these stations caused by atmospheric pressure loading. For these sites on an interannual time scale, the displacements caused by hydrology loading have about the same peak-to-peak variation as the displacements caused by atmospheric pressure loading. At the low-latitude Hartebeesthoek site, the annual vertical amplitudes of hydrology and atmosphere pressure loading are similar. At a midlatitude inland site like Wettzell, there is little annual signal in the pressure loading series, but the hydrology series has a clear annual variation. The subdaily variation is much larger for atmospheric pressure loading than it is for hydrology loading at all sites.
To get a better picture of where the loading signal is significant over the surface of the Earth, we computed the vertical GLDAS and GRACE loading series for each cell on a \(1^{\circ }\) x \(1^{\circ }\) global grid. We computed the annual amplitude and phase of the signal for each cell. The phase of the signal is the epoch during the year where the annual loading signal reaches a maximum. To estimate the amplitude and the phase, we fit hydrology loading series at each gridpoint with the function
where \(t\) is the decimal year, \(c\) is the annual amplitude, \(d\) is the phase in days and \(T=365.25\) days. By expanding the cosine term into cosine and sine terms, we can use linear least squares to estimate \(a, b, c>0\), and \(d\). The variations over the Earth’s surface of the estimated GLDAS amplitudes and phases and GLDAS–GRACE amplitudes and phases are shown in Fig. 3.
The distribution of the loading amplitude over the surface of the Earth is similar to the distribution of the standard deviation of the loading mass shown in Fig. 1. The loading series peaks at \(t=d/T\) when the cosine term reaches a maximum. At this epoch, the vertical loading displacement series is maximum, but the mass contributing to the loading reaches a minimum. Conversely, an increase in the mass loading produces a greater downward displacement of the loading series. From the plots of the loading series, it can be seen that the loading series peaks from July to August (days 250–300) for North America, Europe, South America, Southern Africa and Oceania and in January and February (days 50–100) for Northern Africa and Southern Asia. The phase values for the GLDAS Noah loading agree with the phases for the GRACE loading within 30 days for most continental regions.
As expected, the hydrology loading amplitudes are large in South America (Brazil), Southern Asia, and Southern Africa where the loading mass variability in Fig. 1 is large. In Fig. 3, the vertical loading signal from GRACE in Brazil is 30–40 % greater than from the GLDAS model. In general, the variation of the GLDAS and GRACE annual amplitudes agree well over the surface of the Earth, although there are differences in amplitude in some regions.
4 Detection of hydrology loading and the resulting improvement in VLBI analysis
We investigated whether hydrology loading can be detected in VLBI analysis and in the process evaluated the GLDAS and GRACE hydrology loading series. We considered four different tests to assess the presence of the hydrology signal in the VLBI time series, which we discuss in the next subsections: variance reduction of VLBI position and baseline length time series, site position annual amplitude reduction, estimation of the hydrology signal admittance in the VLBI data, and correlation between the hydrology loading series and the VLBI site position time series.
In the solutions discussed here, the hydrology loading series were applied at the observation level. The loading series are dominated by seasonal variations and have very little variation on subdaily time scale. As a test, we have applied 24-h observing session average hydrology loading corrections for each site in our VLBI analysis. The results were nearly identical to the analysis using the observation level model, where the loading models were evaluated at the epochs of each observation. Vertical site repeatabilities agree to better than 0.05 mm and horizontal repeatabities to better than 0.1 mm except for two sites with differences of 0.2 mm. The fact that observation level and daily average modeling yield nearly identical results implies that investigators interested in combining different solutions (for example, from different techniques) may apply the hydrology loading a posteriori. However, if this is done, no net translation and rotation constraints must be applied after including the loading contribution. Boehm et al. (2009) discusses the consequences of not performing this step.
We used the Calc/Solve system to perform the VLBI analysis reported in this paper. Ma et al. (1990) describes most of the theoretical models employed and the least squares estimation program Solve. In this analysis we applied several additional correction models, including antenna thermal deformation (Nothnagel 2009), VMF1 tropospheric mapping functions (Boehm et al. 2006), and atmospheric pressure loading (Petrov and Boy 2004). These are contributions that we have added to our standard operational geodetic solutions over the last 2–8 years.
The VLBI solutions reported in this paper yield estimated parameters that are global or session parameters depending on the solution type:
-
1.
Global parameters (based on the entire observing period of VLBI sessions included in the solution): site positions and velocities, source positions of all radio sources. Additional possible global parameters are site admittance factors and harmonic site position amplitudes.
-
2.
Session parameters (based on observations within each VLBI 24-h session): site positions when site position time series estimation is needed; pole coordinates and their rates (\(X\)p and \(X\)p rate, \(Y\)p and \(Y\)p rate); UT1 and UT1 rate, nutation; source positions of infrequently observed radio sources, wet zenith delay parameters (piecewise linear with 20-min intervals), and gradient troposphere parameters (piecewise linear with 6 h intervals) and clock parameters (with 60-min intervals).
4.1 WRMS reduction of baseline length and site position time series
We analyzed VLBI group delay data from the series of weekly operational International VLBI Service (IVS for Geodesy and Astrometry) R1 (Monday) and R4 (Thursday) 24-h sessions over the period 2003–2010, during which GRACE was observed. For each 24-h weekly session, there are generally 7–10 stations in the networks of R1 or R4 stations, which are chosen depending on the availability for each week. Over several years of weekly observation, a set of 18 stations were observed in the R1 and R4 series of sessions. A set of 4–5 core stations observed in all sessions in each of these networks remained relatively stable over time. Instead of estimating the positions and velocities as global parameters, we estimated the site positions for each session as session parameters constraining the estimation with a no-net translation constraint applied to the network of observing stations in the session. In the initial solution A1, no hydrology loading correction was applied.
We compared solution A1 with solution A2, where the GLDAS Noah hydrology loading correction was applied, and with solution A3, where the GRACE loading correction was applied.
We first looked at the improvement in the baseline length estimates when hydrology loading corrections were applied. The strength of the VLBI baseline length measurements is that the baseline length is independent of orientation, whereas the local station coordinates up–east–north (UEN) depend on orientation. The weighted root-mean-square (WRMS) repeatabilities were computed for all the baseline length series in solutions A1–A3. The WRMS repeatability of a baseline length series (or site position time series) is the WRMS of the residuals about a best-fit line through the values of the series. Figure 4a shows the baseline length WRMS reduction (in quadrature) as function of the baseline length for solutions A2 and A3 compared to solution A1. As a measure of improvement, the WRMS reduction is the square root of the difference between the squared WRMS of solution series A1 and the squared WRMS of solution series of A2 or A3. By applying GLDAS loading, 79 % of the baselines showed improvement and 80 % improved after applying the GRACE loading contribution. Improvement increases with baseline length, both because the projection of a site vertical displacement into the baseline direction increases with baseline length and because loading is largest in the site vertical direction, where the loading signal is usually two to three times the horizontal signal.
a WRMS reduction of the baseline length for GLDAS (black diamonds) and GRACE (open squares), b WRMS reduction of the vertical position estimates, c WRMS reduction of the north position estimates, and d WRMS reduction of the east position estimates. For the site position plots, the first pair of bars are for GLDAS and the second pair for GRACE. Within each pair, the first bar is the WRMS reduction with pressure loading applied, and in the second pressure loading is not applied
We also found that residual site motion estimates were reduced when hydrology loading was applied, resulting in improvement in the WRMS repeatabilities of the UEN site position series. The WRMS reduction for the R1/R4 sites are shown in Fig. 4b–d. Table 2 provides the observed UEN WRMS repeatabilities and the corresponding WRMS reduction appearing in these figures. The WRMS was reduced in the vertical component for 83 % (GLDAS) and 78 % (GRACE) of the R1 and R4 VLBI sites in solutions A2 and A3 relative to no-loading solution A1. We also found improvement in the horizontal components. In the east (north), we found WRMS reduction for 89 % (67 %) and 67 % (67 %) of sites for GLDAS and GRACE, respectively. On average, the WRMS (UEN) was reduced by (1.16, 0.64, 0.58 mm) with GLDAS and (0.94, 0.47, 0.55 mm) with GRACE.
It is possible that the hydrological WRMS reduction at a site is not as large as expected, because there may be temporal correlations between the applied hydrology and pressure loading contributions or because of errors in the pressure loading correction. To check this, we ran additional solutions where pressure loading was not applied. The results of this this test are also shown in Fig. 4 and for most sites the effect is not very significant in the vertical. The largest effect was for Seshan25 (China), where the WRMS reduction was larger by 0.8 (1.2) mm for GLDAS (GRACE) loading if pressure loading was also applied. There are significant differences of \(>\)1 mm in the east component for Matera, Wettzell, Hartrao, and Tsukuba.
We generally see WRMS vertical reduction for European VLBI sites (Matera, Medicina, Ny Alesund, Onsala, Wettzell). Similarly, Tregoning et al. (2009) obtained WRMS vertical reduction for 32 of 36 sites European GPS sites when GRACE loading was applied. For the horizontal components, the GPS WRMS reduction in the east is negative for most sites, but some sites show positive WRMS reduction in the north. The VLBI sites at Matera and Wettzell (two sites with colocated GPS receivers) also show a positive WRMS reduction in the north component when GRACE or GLDAS loading is applied, but a negative reduction for the east component. However, it is interesting that if pressure loading is not applied, the WRMS reduction shown in Fig. 4 for both horizontal components is positive for these two sites. For non-European sites, the GPS WRMS reduction is much less uniform than in Europe; Tregoning et al. (2009) found that the WRMS of GPS vertical residuals increased for 47 % of the GPS sites worldwide. There are a few sites (for example, Fortleza, Brazil) where the VLBI and GPS WRMS reduction appears to be similar, or as in Hobart (Tasmania) where both the VLBI and GPS suffer an increased vertical site variance. Cases where variance is increased for GLDAS or GRACE are mainly those on the coast or on small islands: Hobart (Tasmania, Australia), Kokee (Kauai, Hawaii), Ny-Alesund (Svalbard, Norway) and Tsukuba (Japan).
The vertical WRMS at Badary increases significantly with hydrlology loading applied. The Badary pressure admittance (see Table 4) given in Sect. 4.3 along with GLDAS (GRACE) hydrology admittance was only \( 0.51 \pm 0.16\,(0.40 \pm 0.06)\), which indicates that the pressure loading model may not be correct. However, the WRMS reduction for Badary changes by \(<\)0.2 mm in solutions that do not include pressure loading. Thus, pressure loading is not likely to be responsible for the anomalous Badary results. Unless the GRACE and GLDAS models are deficient for Badary, it appears that some other unknown unmodeled local motion, which is anticorrelated with hydrology loading, is responsible for this behavior. The GRACE hydrology admittance (see Sect. 4.3) is close to zero \((0.05 \pm 0.40)\), which means that if it is applied in a solution, the WRMS vertical repeatability should increase. The GLDAS admittance of \((-3.57 \pm 1.34)\) implies that the GLDAS model is anticorrelated with observed vertical motion, which is consistent with the increase in the vertical WRMS. The increase in WRMS vertical repeatability (negative WRMS reduction), \(-3.21\) mm (\(-1.21\) mm), for GRACE (GLDAS) is reasonably consistent with the vertical RMS 2.19 mm (1.50 mm) of the GRACE (GLDAS) model.
We also ran solutions similar to A1 and A2 in which we extended the period of analysis to 1980–2010, since GLDAS data are available over this longer period. Figure 5 shows the resulting reduction of WRMS for the most frequently observed VLBI sites when hydrology loading is applied. The level of WRMS reduction is similar to the reduction for the period 2003–2010. For the vertical (east and north), the WRMS was reduced for 82 % (72 and 82 %) of the 37 sites.
In general, the application of hydrology loading series improves most of the station position and baseline length estimates. These results are consistent with the hypothesis that there is a hydrology loading signal in the VLBI data.
4.2 Annual amplitude reduction of the site position variation
Given the size of the hydrology loading signal, which we discussed in Sect. 3, we expect a clear annual signal to be present for VLBI sites. It should be clearly present in the observed series unless there are any other unmodeled seasonal signals that are anticorrelated with hydrology loading.
Applying hydrology loading corrections in VLBI analysis should remove a portion of the observed seasonal signals. To investigate this, we have estimated the annual and semi-annual components of site position motion (along with the position offsets and velocities for all sites) as global parameters for frequently observing VLBI sites in three solutions: B1, B2, and B3 (see description of solution types at the beginning of Sect. 4). Solution B1 is a non-hydrology loading solution that includes contributions from antenna thermal deformation, the VMF mapping function, and atmospheric pressure loading. Solution B2 adds the contribution of GLDAS hydrology loading, and solution B3 alternatively applies GRACE hydrology loading. Applying the loading in the VLBI analysis is equivalent to applying a correction to the a priori model for the positions of the stations.
We compared the performance of GLDAS versus GRACE to account for the annual variations of the position estimates over the period 2003–2010. Figure 6 shows the site vertical amplitudes from the three solutions. Overall, the vertical amplitudes were reduced for 16 of 18 sites when either the GLDAS or GRACE loading series were applied. For several sites, the vertical amplitude is reduced to 1 mm or less. Horizontal amplitudes in the north component are reduced for 11 of 18 sites, whereas 8 of 18 sites had smaller east annual amplitudes, but most of the east amplitudes were not more than 0.5 mm. We also ran solutions similar to B1 and B2 for the period 1990–2010 and found a similar level of site vertical annual amplitude reduction when the GLDAS loading series was applied. The vertical amplitudes were reduced for 28 of 37 sites. Table 3 compares the WRMS of the estimated annual and semi-annual vertical amplitudes of all sites for solutions with and without hydrology loading applied. The amplitude reductions resulting from applying GLDAS for the period 2003–2010 are similar to the reduction for the longer period 1990–2010.
Estimated annual amplitude of the site UNE site position components over the time period 2003–2010 from three solutions: (1) no hydrology modeled in solution B1 (black bars), (2) GLDAS hydrology modeled in solution B2 (open bars), and (3) GRACE loading in solution B3 (grey bars). The error bars for each site are the same for all three solutions, but are only shown for B1
4.3 Hydrology and pressure loading admittance factors
A technique for estimating how much signal is present in the observables is to estimate the admittance of the signal. This approach was used in Petrov and Boy (2004) to investigate how much of the pressure loading signal is present in the observations. We represent the true hydrology loading series by the product \(A \cdot a_\mathrm{model}\) where \(A\) is an admittance factor and \(a_\mathrm{model}\) is the computed site vertical hydrology loading series. If a model is perfect, the admittance factor should be close to one, which would mean that the modeled signal is close to the true loading signal. The interpretation of admittance factors for hydrology loading is possibly problematic, since the signal is largely seasonal so that the admittance may include effects of other unmodeled correlated seasonal signals at a site.
We find the admittance factors by estimating them as additional global parameters (see description of solution types at the beginning of Sect. 4) for each site in Calc/Solve VLBI analysis. GLDAS and GRACE admittances were estimated for the period 2003–2010. To look for possible correlation between pressure loading and hydrology loading, we estimated both hydrological and pressure loading admittances in the solutions. We also performed the estimation for GLDAS loading over a longer time period (1990–2010) with a larger set of stations. Table 4 shows the estimated admittance factors and correlations. Overall, the mean hydrology admittance over the period 2003–2010 for GRACE (1.01 \(\pm \) 0.05) is closer to unity than for GLDAS (1.39 \(\pm \) 0.07) and the standard deviation of the estimates is smaller (0.27 versus 0.68). The mean pressure loading admittances for all cases in Table 4 are close to unity, consistent with Petrov and Boy (2004).
GLDAS admittances for several sites are significantly greater than unity indicating that other correlated unmodeled contributions are also present. Most of the correlations between pressure and hydrology loading admittances are not significant. However, in the most significant cases for GRACE, the admittances are anticorrelated: Fortleza (\(-0.33\)) and Tsukub32 (\(-0.34\)); and for GLDAS: Fortleza (\(-0.31\)) and Tigoconc (\(-0.36\)).
The admittances were estimated using the VMF1 mapping functions. As a test, when alternative solutions were made using the NMF mapping functions, the admittances for some of the sites changed significantly. For instance, the NMF GLDAS hydrology and pressure admittances at Tsukuba (0.85 and 1.21) were both much closer to unity. For Hobart26, the NMF GLDAS admittances were (1.04 and 0.85) again closer to unity than with VMF1. However, for Ny-Alesund, the NMF hydrology and pressure admittances were (0.58 and 0.42), which is much closer to unity for hydrology, but the pressure admittance decreased significantly from 0.98. To investigate further, we also ran solutions similar to A1 and A2 and found that the site vertical repeatabilities for these Tsukuba and Hobart26 were better with NMF than VMF1, which is an indication of a possible error in VMF1 for these sites. It is beyond the scope of this paper to fully investigate correlations between mapping function errors and the loading contributions.
For comparison with our results, we note that Tregoning et al. (2009) computed GLDAS vertical admittances for ten Canadian GPS sites by estimating a scale factor applied to the GLDAS displacements to fit the observed GPS height anomalies. They obtained factors ranging from 1 to 2.7. The larger-scale factors are either caused by deficiencies in the GLDAS model (for example, no modeling of groundwater) or other unmodeled errors in the GPS time series.
4.4 Correlation between the VLBI position time series and the hydrology loading series
Another way to investigate the presence of hydrology loading in the VLBI position time series is to calculate the correlation between the VLBI position time series and the hydrology loading series. First, we computed monthly VLBI series from the position time series (estimated in solution A1 where no hydrology loading was applied) by averaging all observations corresponding to the same month. This was done by weighting each observation using the errors of the position estimates. The GRACE mascon time series was smoothed with a Gaussian filter to obtain a monthly series. Since the hydrology loading series calculated from the GLDAS Noah model are already monthly, we did not process it further.
We chose a subset of stations observed in R1 and R4 sessions during the period 2003–2010, requiring that each station was observed in five or more session days during each month we considered. This was done to obtain good accuracy in the resampled series. In addition we required that a site satisfied the monthly sampling requirement for at least 10 months. The correlation between the position time series and the two hydrology loading series is given for these sites in Table 5.
The correlation depends on how dominant the hydrology loading signal is for a specific site and whether there are other unmodeled effects present that are correlated with the hydrology signal. The mean correlation is 0.47 for the GLDAS Noah model and 0.43 for the GRACE mascons, meaning that our hydrology loading series are on average correlated with the position time series. The worst correlations between hydrology loading series and VLBI time series are for island sites (Kokee, Ny Alesund) where signals are small or coastal sites (TIGO Concepcion, Matera).
The most frequently observed VLBI antenna during the GRACE period 2003–2010 is in Wettzell, Germany. Figure 7 shows the monthly vertical time series for Wettzell plotted against the monthly GLDAS Noah and GRACE mascon hydrology loading series. The correlation between the position time series and the GLDAS Noah model hydrology series is 57 % and for the GRACE mascon series 48 %. These correlations indicate that hydrology is a dominant signal for Wettzell and thus it is not surprising that the annual amplitude is reduced when hydrology loading is applied. The correlation is not as good at other sites, probably because some sites may have other remaining unmodeled effects that are of at least of the same magnitude as the hydrology signal.
Two sites that have a negative correlation with the hydrology loading series are Kokee (using GLDAS loading) and Ny Alesund (using GRACE loading), which is consistent with the increase in vertical variance (see Fig. 4b). These are both island sites so the hydrology signal is very small, as can be seen in Table 1. The negative correlation essentially means that hydrology loading is not the main effect that has to be modeled at those sites. For Gilcreek, Svetloe, Westford, Wettzell, and Zelenchukskaya, there was significant reduction of vertical variance (see Fig. 4b) and the correlations between the position time series and the hydrology loading series are all large enough to draw the conclusion that hydrology loading is a significant effect at these stations.
5 Conclusions and future work
We have verified the presence of a hydrology loading signal in VLBI measurements. Application of the hydrology loading series computed from either the GLDAS Noah model or from GRACE mascons improves the site position estimates. In analysis of R1+R4 VLBI sessions from 2003 to 2010, we found a baseline length WRMS reduction of 1–4 mm for 79 % (80 %) of the baselines when GLDAS (GRACE) hydrology loading is applied in VLBI analysis. There is also a reduction in the site position UEN repeatabilities for most sites; the vertical WRMS reduction was 1–3 mm for 83 % (78 %) of sites with GLDAS (GRACE) loading applied. In the east (north), there is a WRMS reduction of 0.5–2 mm for 89 % (67 %) and 67 % (67 %) of sites for GLDAS and GRACE, respectively. In the future, it would be illuminating to make a detailed comparison of loading results at colocated VLBI and GPS sites.
Global estimates of the annual site vertical amplitudes were reduced for 16 out of 18 sites when either the GLDAS or GRACE loading series were applied in analysis. We estimated loading vertical admittance factors for each site and found that the average admittances were 1.01 \(\pm \) 0.05 for GRACE and 1.39 \(\pm \) 0.07 for GLDAS. The standard deviation of the GRACE admittances and GLDAS admittances were 0.31 and 0.68, respectively. Based on this admittance result, we conclude that GRACE is better at correcting the hydrology signal in VLBI data. As a last test, we generated resampled VLBI monthly position time series and computed its correlation with the GLDAS series and monthly resampled loading series from GRACE. The average correlations between the VLBI vertical position time series and the GLDAS model series or the GRACE series were found to be 0.47 and 0.43, respectively, which is comparable to the level of correlations between GRACE loading series and GPS site postion vertical time series in Tregoning et al. (2009).
We have established a loading service available at http://lacerta.gsfc.nasa.gov/hydlo/ where we provide hydrology loading products. We produce site position loading series for a list of about 170 VLBI sites with respect to both the center of mass of the solid Earth and center of mass of the solid Earth + loading mass. These series are updated as soon as new GLDAS data become available. We also produce loading series computed on a \(1^{\circ } \times 1^{\circ }\) global grid which can be interpolated to any point of interest. These series are provisional products in the International Earth Rotation and Reference Systems Service Global Geophysical Fluids Center.
In the near future, we plan to compute series for the new GLDAS model, GLDAS-2, which is more self-consistent and should explain anomalies that we observed during the years 1995–1997. The main objective of GLDAS-2 is to create more climatologically consistent data sets using the same forcing data sets over the period of the model (Rodell, personal communication, 2013). We plan on making loading series computed from GLDAS-2 available on the loading service website as soon as they have been tested.
References
Boehm J, Werl B, Schuh H (2006) Troposphere mapping functions for GPS and very long baseline interferometry from European Center for Medium-Range Weather Forecasts operational analysis data. J Geophys Res 111:B02406. doi:10.1029/2005JB003629
Boehm J, Heinkelmann R, Mendes Cerveira PJ, Pany A, Schuh H (2009) Atmospheric loading corrections at the observation level. J Geod 83(11):1107–1113. doi:10.1007/s00190-009-0329-y
Davis JL, Elosegui P, Mitrovica JX, Tamisiea ME (2004) Climate-driven deformation of the solid Earth from GRACE and GPS. Geophys Res Lett 31:L24605. doi:10.1029/2004GL021435
Farrell WE (1972) Deformation of the earth by surface loads. Rev Geophys Space Phys 10(3):761–797
Kato H, Rodell M, Beyrich F, Cleugh H, van Gorsel E, Liu H, Meyers TP (2007) Sensitivity of land surface simulations to model physics, land characteristics, and forcings, at four CEOP sites. J Meteorol Soc Jpn 85a:187–204
Liang X, Lettenmaier DP (1994) A simple hydrologically based model of land surface water and energy fluxes for general circulation models. J Geophys Res 99(D7):14,415–14,428
Luthcke SB, Sabaka TJ, Loomis BD, Arendt AA, McCarthy JJ, Camp J (2013) Antarctica, Greenland, and Gulf of Alaska land-ice evolution from an iterated GRACE global mascon solution. J Glaciol 59(216). doi:10.3189/2013JoG12J147
Ma C, Sauber JM, Bel LJ, Clark TA, Gordon D, Himwich WE, Ryan JW (1990) Measurement of horizontal motions in Alaska using very long baseline interferometry. J Geophys Res 95(B13):21991–22011
MacMillan DS, Gipson JM (1994) Atmospheric pressure loading parameters from very long baseline interferometry observations. J Geophys Res 99:18,081–18,087
MacMillan D, Boy J-P (2004) Mass loading effects on crustal displacements measured by VLBI. In: IVS 2004 general meeting proceedings, pp 476–480
Milly PCD, Shmakin AB (2002) Global modeling of land water and energy balances. Part I: the land dynamics (LaD) model. J Hydrometeorol 3:283–299
Nothnagel A (2009) Conventions on thermal expansion modelling of radio telescopes for geodetic and astrometric VLBI. J Geod 83(8):787–792. doi:10.1007/s00190-008-0284-z
Petrov L, Boy J-P (2004) Study of the atmospheric pressure loading signal in very long baseline interferometry observations. J Geophys Res 109:B03405. doi:10.1029/2003JB002500
Rodell M et al (2004) The global land data assimilation system. Bull Am Meteorol Soc 85(3):381–394
Rowlands DD, Luthcke SB, Klosko SM, Lemoine FGR, Chin DS, McCarthy JJ, Cox CM, Anderson OB (2005) Resolving mass flux at high spatial and temporal resolution using GRACE intersatellite measurements. Geophys Res Lett 32:L04310. doi:10.1029/2004GL021908
Sabaka TJ, Rowlands DD, Luthcke SB, Boy J-P (2010) Improving global mass flux solutions from Gravity Recovery and CLimate Experiment (GRACE) through forward modeling and continuous time correlation. J Geophys Res 115:B11403. doi:10.1029/2010JB007533
Schuh H, Estermann G, Cretaux JF, Merge-Nguyen M, van Dam T (2003) Investigation of hydrological and atmospheric loading by space geodetic techniquesl. In: International Association of Geodesy Symposia, vol 126. Springer, Berlin
Syed TH, Famigliett JS, Rodell M, Chen J, Wilson C (2008) Analysis of terrestrial water storage changes from GRACE and GLDAS. Water Resour Res 44:W02433. doi:10.1029/2006WR005779
Tesmer V, Steigenberger P, van Dam T, Mayer-Gurr T (2011) Vertical deformations from homogeneously processed GRACE and global GPS long-term series. J Geod 85:291–310. doi:10.1007/s00190-010-0437-8
Tregoning P, Watson C, Ramillien G, McQueen H, Zhang J (2009) Detecting hydrologic deformation using GRACE and GPS. Geophys Res Lett 36:L15401. doi:10.1029/2009GL038718
Tregoning P, Ramillien G, McQueen H, Zwarte D (2009) Glacial isostatic adjustment and nonstationary signals observed by GRACE. J Geophys Res 114:B06406. doi:10.1029/2008JB006161
van Dam T, Blewitt G, Heflin MB (1994) Atmospheric pressure loading effects on Global Positioning System coordinate determinations. J Geophys Res 99:23,939–23,950
van Dam T, Herring TA (1994) Detection of atmospheric pressure loading using very long baseline interferometry measurements. J Geophys Res 99:4505–4518
van Dam T, Wahr J, Milly PCD, Shmakin AB, Blewitt G, Lavallee D, Larson KM (2001) Crustal displacements due to continental water loading. Geophys Res Lett 28(4):651–654
van Dam T, Wahr J, Lavalee D (2007) A comparison of annual vertical crustal displacements from GPS and Gravity Recover and Climate Experiment (GRACE) over Europe. J Geophys Res 112:B03404. doi:10.1029/2006JB004335
Acknowledgments
We would like to thank D. Rowlands for providing advice on using and interpreting NASA GSFC mascon data. We thank M. Rodell for his help in using NASA GSFC GLDAS data. J.-P. Boy helped us check out our loading algorithms. We also acknowledge the valuable suggestions made by the reviewers. We acknowledge the International VLBI Service for Geodesy and Astrometry (IVS) for providing the VLBI data that we used in our analysis.
Author information
Authors and Affiliations
Corresponding author
Rights and permissions
About this article
Cite this article
Eriksson, D., MacMillan, D.S. Continental hydrology loading observed by VLBI measurements. J Geod 88, 675–690 (2014). https://doi.org/10.1007/s00190-014-0713-0
Received:
Accepted:
Published:
Issue Date:
DOI: https://doi.org/10.1007/s00190-014-0713-0