Abstract
Diverse rice germplasm comprising 112 genotypes was evaluated for yield traits across three environments. Pooled and environmentwise analysis of variance revealed heterogeneity in the data and significant environment interactions for all the yield traits. As per AMMI (additive main effects and multiplicative interaction) and GGE (genotype and genotype x environment interaction) biplots, the influence of environment was significant and varying on all the component yield traits including grain yield and was not significant in case of flowering date. Dry season at Maruteru in 2014–15 (E1) was the most discriminative and representative environment for favourable plant growth in terms of plant height, panicle number and panicle length. None of the environments represented ideal environment for the favourable expression of grain number while all the environments were equally informative for thousand grain weight and grain yield. Panicle number, grain number and thousand grain weight were contributing to grain yield across the environments. Three genotypes Panthdhan 12, Konark and Udaygiri were the most stable genotypes for grain yield with favourable combination of associated yield genes for all the traits, viz. 1000 grain weight, the number of grains per panicle, the number of filled grains per panicle, productive tillers and plant height with higher yield, and grouped in one cluster. Genotyping using previously reported markers revealed that favourable alleles of yield genes associated with the number of productive tillers were predominantly found followed by alleles for the number of grains/filled grains per panicle correlating with the superior phenotypic value of the respective trait. The information on association of yield stability with reported yield genes from this study is useful in marker-assisted breeding studies for yield improvement and can be confirmed with various sets of genotypes under multi-environment testing. The identified superior genotypes are potential components in future breeding programmes and the development of stable adaptable varieties. The present study suggests that yield stability could be effectively achieved with targeted improvement of component yield traits associated with favourable alleles.
Similar content being viewed by others
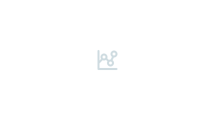
Avoid common mistakes on your manuscript.
Introduction
Rice farming is the backbone of agriculture in Asia as over 90% of the world’s rice is produced and consumed in this region. In India, rice is grown throughout the year across diverse ecologies including irrigated lowland, rainfed shallow lowland and upland, high elevated hills, below mean sea level deep water submerged conditions and also under inland and coastal saline ecologies. Improving rice production per unit area and per unit time will be a major challenge in future due to the expanding population of rice consumers in the world (Balakrishnan et al. 2016). Identification of high-yielding stable genotypes for multiple environments as in the case of Indian scenario is a challenge in breeding programmes. Therefore, consideration of effect of genotype by environment (G × E) interactions is essential in evaluation of genotypes for variety development (Kempton et al. 1997; Atlin 2000, Abebe et al. 2023). As grain yield is a complex quantitative trait, with high environment interactions, continued improvement of grain yield remains the top priority in most of the breeding programmes. Hence, selection of genotypes based on performance in single environment is not effective for varietal identification (Yan et al. 2002; Shrestha et al. 2012). It was suggested that an increase in grain yield could be effectively achieved through yield component improvement since yield components have higher heritability than grain yield (Xiong et al. 1992). It is essential to carry out selection based on yield stability evaluation than average performance in multiple environment conditions (Kang 1993; Tariku et al 2013; Islam et al. 2015; Balakrishnan et al. 2016).
Considering the genetic background and unpredictable environmental factors which prevail at different locations and over time, differential responses are observed from the improved genotypes when tested across the environments (Krishnamurthy et al. 2016). The variable genotypic responses in different environments are called genotype × environment (G × E) interactions which goes back to the classical work of Allard and Bradshaw (1964). In the plant breeding programmes, the G × E interactions play a key role in identification of the most desirable genotypes, mega-environments, representative locations and other adaptation targets (Krishnamurthy et al. 2016). The presence of G × E makes identification of the real potential of a genotype in specific location in which climate varies from year to year more challenging (Haji and Hunt 1999). When G × E interaction is present, the environmental factor that could play a major role causing differential crop performance is to be identified and desirable breeding strategies must be determined based on the same (Asenjo et al. 2003). The quantification of G × E is an important consideration in plant breeding programmes, because it reduces the speed of genetic advancement through selection (Hill et al. 1975). Selection of genotypes for stability and adaptability is required as a prior to recommendation in case of a crop such as rice which is grown in diverse ecologies.
There are a number of statistical methods reported for the evaluation of G × E interaction and its relationship with genotypic stability (Krishnamurthy et al. 2015). Stability analysis can be conducted on replicated trials over several environments following environmentwise analysis of variance and pooled analysis of variance. Several stability statistics have been proposed to estimate G × E. The traditional measures use the coefficient of variation (Francis et al. 1978), environmental variance (Lin et al. 1986), stability variance (Shukla et al. 1972), regression-based parameters (Finlay et al. 1963) and stability analysis (Eberhart et al. 1966). AMMI (additive main effect and multiplicative interaction) and GGE (genotype and genotype × environment interaction) biplots are excellent tools for visual data analysis for different environments. AMMI method has been effective because it captures a large portion of the G × E sum of squares and it clearly separates main and interaction effects (Gauch and Zobel 1997). AMMI is a two-step approach: it first applies the additive analysis of variance (ANOVA) model to two-way data and then applies the multiplicative principal components analysis (PCA) model to the residual from the additive model, i.e. to the interaction. The GGE biplot methodology (Yan 2002; Yan and Kang 2003; Yan and Tinker 2006) consists of a set of biplot interpretation methods, whereby important questions regarding genotype evaluation and test environment evaluation can be visually addressed. The concept of GGE originates from analysis of multi-environment testing (MET) of crop cultivars. The yield of a cultivar (or any other measure of cultivar performance) in an environment is a mixed effect of genotype main effect (G), environment main effect (E) and genotype × environment interaction (G × E). In normal METs, E accounts for 80% of the total yield variation, and G and G × E each account for about 10% (Gauch and Zobel 1997; Yan et al. 2000). For the purpose of cultivar evaluation, however, only G and G × E are relevant (Gauch and Zobel 1997). Furthermore, both G and G × E must be considered in cultivar evaluation, thus the term GGE (Yan et al. 2000).
The past few decades showed tremendous advancement in understanding the crop genomics, and several yield-related genes were detected in rice genome. Genes related to grain number, grain size and grain filling, viz. Gn1a, Gs3 and Gif1, were identified (Ashikari et al. 2005; Fan et al. 2006; Wang et al. 2008), and several of yield genes were cloned and characterized further for utilization in breeding programmes. Understanding genetic architecture and status of allelic variation available in germplasm is of paramount importance to initiate further breeding and mapping studies. However, studies on characterization of Indian cultivars for already known genes and their effect on yield superiority or stability are very limited. We evaluated the performance of diverse rice genotypes across three environments to assess the G × E interactions and identify stable high-yielding genotype(s) through the yield stability and adaptability analysis using the AMMI and GGE models. Further, these genotypes were evaluated for the presence of favourable alleles of the known yield genes and understand the effect of their presence in the performance of superior stable genotypes.
Materials and methods
Locations
Field experiments were taken up at Regional Agricultural Research Station (RARS), Maruteru (latitude 15°58' N; longitude: 80°05' E; 10MSL), Acharya NG Ranga Agricultural University (ANGRAU), Andhra Pradesh, during rabi (dry season) of 2014–15 (E1) and ICAR-Indian Institute of Rice Research (ICAR-IIRR) farm located in International Crops Research Institute for the Semi-Arid Tropics (ICRISAT) campus Patancheru (latitude: 17°53' N; longitude: 78°27' E; 543MSL), Hyderabad, Telangana, India, during kharif (wet season) 2015 (E2) and 2016 (E3).
Plant material
A total of 112 diverse rice genotypes (Supplementary Table S1) comprising land races, improved varieties, breeding lines, aromatic cultures, mutant lines (CN), MT lines (Moroberekan/Vijetha) and NERICA lines—NEwRICe for Africa (derived lines of Oryza glaberrima/Oryza sativa) were used for morphological and molecular characterization.
Field experimental details
Seeds of the materials under study were sown in nursery beds, and 25-day-old seedlings were transplanted in the field with single seedling per hill in all the field trials. Seedlings were transplanted in two rows of 2 m length, with a spacing of 15 × 10 cm in randomized complete block design (RCBD) with two replications. Normal package of practices and fertilizer application were followed; weeds, insects and diseases were controlled using standard herbicides and pesticides as required to avoid yield loss. These same parameters were followed uniformly across the seasons and locations. In all the three environments, the genotypes were evaluated for seven yield traits, viz. days to flowering (DFF), plant height (PH) in cm, the number of productive tillers (PN), panicle length (PL) in cm, the number of grains per panicle (GN), thousand grain weight (TW) in g and grain yield (SPY) in g.
Stability analysis
Analysis of variance was computed for individual environment; then, a combined analysis of variance was performed, considering both environments and genotypes as fixed using PB tools (Version 1.4, http://bbi.irri.org/products) and R (R Core Team 2012) with RCBD. Significance of all effects was tested against mean square of error. The performance of all the genotypes was tested over three environments and was assessed using stability models, viz. (1) additive main effects and multiplicative interaction (AMMI) (Gauch and Zobel et al. 1997) and (2) GGE biplot or site regression model (Yan and Kang et al. 2003). These models were used to interpret and visualize the stability and G × E (Genotype Environment Interaction) patterns. In the AMMI model, only the G × E term is absorbed in the multiplicative component, whereas in the GGE model, the main effects of genotypes (G) plus the G × E are absorbed into the multiplicative component. The AMMI model (Gauch et al. 1988) was used in analysing the stability and interaction for yield traits. The AMMI model is a combination of analysis of variance (ANOVA) and principal component analysis (PCA). The G × E interaction was evaluated with the AMMI model by considering the first two principal components. ANOVA model was used to analyse the trait data with main effects of genotype and environment without the interaction; then, a principal component analysis was integrated using the standardized residuals. These residuals include the experimental error and the effect of the GEI. The analytical model can be written as
where Yij. is the mean yield of ith genotype in jth environment, μ is the overall mean, δi is the genotypic effect, βj is the environment effect, λk is the singular value for PC axis k, ik is the genotype eigenvector value for PC axis n, βjk is the environment eigenvector value for PC axis, k and "ϵij are the residual error assumed to be normally and independently distributed (0, σ2/r), σ2 is the pooled error variance and r is the number of replicates. GGE biplots display both G (genotype) and GE (genotype environment) variation (Kang et al. 1993) for genotype evaluation. The GGE biplot is based on the site regression (SREG) linear–bilinear model (Cornelius et al. 1996; Crossa and Cornelius 1997; Crossa et al. 2002). The site regression model as a multiplicative model in the bilinear terms shows the main effects of cultivars plus the cultivar × environment interaction (GGE) and the model is
The GGE biplot graphically represents G and G × E effect present in the multi-location trial data using environment-centred data. GGE biplots were used to evaluate (1) mega-environment analysis (which-won-where pattern), where genotypes can be recommended to specific mega-environments, (2) genotype evaluation, where stable specific genotypes can be recommended across all locations, and (3) location evaluation, which explains discriminative power of target locations for genotypes under study (Yan and Tinker 2006). Sum of square percentage was computed as percentage of sum of squares of components of stability analysis of variance per total sum of squares to know the contribution of each component, viz. genotype environment and GEI. Correlation analysis was performed with Statistical Tool for Agricultural Research (STAR) using Pearson’s correlation coefficient method. Significance levels are indicated as: ∗ P < 0.05, ∗ ∗ P < 0.01, ∗ ∗ ∗ P < 0.001.
Molecular screening
A mini preparation procedure for the extraction of total genomic DNA (modified method of Zheng et al. Zheng et al. 1995) from the leaf samples of 112 genotypes was adopted. NanoDrop method was used to know the concentration and purity of the isolated DNA. DNA amplification was carried out in 10 μl volumes in a Bio-Rad PCR. Each reaction mixture contained 3 μl of genomic DNA (50 ng/μl), 0.5 μl of each primer (at a concentration of 0.2 μM), 1 μl of 10 × PCR buffer with MgCl2, 1 μl of 2.5 mM dNTP mixture, 0.1 μl of 5 units/μl Taq DNA polymerase and 3.9 μl of PCR-grade water. The list of the primer sequence information is given in Supplementary Table S2. The temperature profile of the first PCR cycle was 95 °C for 5 min and 55–60 °C for 2 min, followed by 35 cycles of 1 min at 95 °C, 1 min at 55–60 °C and 2 min at 72 °C. The final extension was at 72 °C for 10 min. The amplified products were separated in 4 per cent agarose gel prepared in 1X TBE buffer stained with ethidium bromide. The gel was run in 0.5X TBE buffer at constant voltage of 120 V for a period of 1 h to 2 h. The gel was visualized in UV transilluminator and photographs taken using Alpha Digidoc gel documentation instrument. Clearly resolved, unambiguous bands were scored visually for their presence or absence with each primer. The scores were obtained in the form of matrix with ‘1’ and ‘0’, which indicate the presence and absence of bands in each variety, respectively.
Results
Environmental conditions
Among the test environments, total crop growing period was observed as extended by almost a month in E1 (dry season 2014–15 at Maruteru) compared to the crop growing period of E2 and E3 (two wet seasons at Hyderabad in 2015 and 2016), respectively. Low night temperatures during the initial establishment in nursery resulted in poor growth of the seedlings and caused delay in the transplanting in E1. The grain ripening and maturity in both the wet seasons at Hyderabad in 2015 (E2) and 2016 (E3) coincided with drop in night temperatures. Since all the three experiments were taken up under completely irrigated conditions, differences in rainfall or the number of rainy days across the seasons did not show any significant effect in the crop growth. The crop experienced highest bright sunshine hours after transplanting till maturity in dry season at Maruteru in 2014–15 (E1) with greater wind speed. Mean evaporation rate was highest during wet season at Hyderabad in 2015 (E2) (Table 1).
Variability of yield and yield-related traits
Individual environmentwise ANOVA revealed the extent of variability available in the genotypes for all the studied traits. Effect of the genotypes was significant for all the traits across the environments except for panicle number in wet season at Hyderabad in 2016 (E3) and thousand grain weight in dry season at Maruteru in 2014–15 (E1). However, ANOVA computed based on pooled data of all the three environments indicated significant genotype, environment and their interaction effects on all the yield traits (Table 2). Interaction effect (σ2ge) is observed as the most important component contributing to phenotypic variance (Fig. 1). Among the components of phenotypic variance (σ2p), maximum contribution of genotypic variance (σ2g) to the extent of 74.63% was observed for the trait, days to fifty per cent flowering followed by environmental variance (σ2e) of 52.91% to plant height and variance due to interaction (σ2ge) of 44.47 and 41.06% to grain yield and panicle number, respectively (Fig. 1).
There was a wide range in flowering time among the genotypes in both dry season at Maruteru in 2014–15 (E1) and wet season at Hyderabad in 2015 (E2) with late flowering in most of the genotypes in E1 and early flowering in wet season at Hyderabad in 2016 (E3) with an overall mean of 65 days across the three environments. The genotypes grew taller in dry season at Maruteru in 2014–15 (E1) compared to both the wet seasons, and stunted growth was observed in wet season at Hyderabad in 2015 (E2) with an average of 95 cm across the environments. Similar to the flowering time, panicle number also showed a wide range of variation in dry season at Maruteru in 2014–15 (E1). Majority of the genotypes (75%) had low panicle number and short panicles in wet season at Hyderabad in 2015 (E2) while very low grain number was observed in dry season at Maruteru in 2014–15 (E1). As far as thousand grain weight is concerned, not much variation was observed across the environments in terms of minimum and maximum values and pooled environmental average was 20 g. Grain yield range was narrow in dry season at Maruteru in 2014–15 (E1) and wet season at Hyderabad in 2016 (E3) while maximum outliers were observed in wet season at Hyderabad in 2015 (E2) (Fig. 2). Environmentwise and pooled mean phenotypic performance of the 112 genotypes for the yield traits under study along with descriptive statistics are given in Supplementary Tables S3 and S4.
Box plot showing the differences in yield and related traits among genotypes. a Box plot for days to flowering; b box plot for plant height; c box plot for panicle number; d box plot for panicle length; e box plot for grain number; f box plot for test weight; g box plot for single plant yield; dots represent outliers
Pooled and environment analysis of the Pearson correlation coefficients revealed positive correlation of grain yield with panicle number, grain number and thousand grain weight across the environments and it was highly significant with panicle number and thousand grain weight in dry season at Maruteru in 2014–15. Though grain yield showed non-significant positive correlation with panicle length in the pooled analysis, it was non-significant negative in both dry season at Maruteru in 2014–15 and wet season at Hyderabad in 2016 and non-significant positive in wet season at Hyderabad in 2015. Both days to flowering and plant height were non-significant and negatively correlated with grain yield in the pooled analysis while they were non-significant and variable across the seasons and environments. Correlation between days to flowering and grain yield was non-significant and negative in dry season at Maruteru in 2014–15 and wet season at Hyderabad in 2015 while the same was non-significant and positive in wet season at Hyderabad in 2016. Correlation between plant height and grain yield was non-significant and positive in dry season at Maruteru in 2014–15 while the same was non-significant and negative in both the wet seasons at Hyderabad in 2015 and 2016. Environmentwise correlations are graphically depicted in Fig. 3, and pooled correlation coefficients are presented in Table 3.
Graphical representation of the correlation matrices of yield traits in three seasons. a Correlogram of rabi 2014–15 season at Regional Agricultural Research Station (RARS), Maruteru, ANGRAU, Andhra Pradesh, India—534,122; b and c ICAR-Indian Institute of Rice Research (ICAR-IIRR) farm located in International Crops Research Institute for the Semi-Arid Tropics (ICRISAT) campus Patancheru, Hyderabad, Telangana, India, during kharif season 2015 (E2) and kharif season 2016 (E3), respectively
Stability analysis
For the stability analysis, a combination of season and location was considered as an environment. Weather data of the three environments revealed relatively low mean temperature, high relative humidity and rainfall in wet season at Hyderabad in 2016 (E3), more number of bright sunshine hours and high wind speed in dry season at Maruteru in 2014–15 (E1) and very high amount of evaporation in wet season at Hyderabad in 2015 (E2). For days to flowering, environment–vector view of the GGE biplot indicated that the influence of environment on flowering date was not significant, but flowering pattern in dry season at Maruteru (E1) and wet season at Hyderabad in 2015 (E2) was similar as compared in wet season at Hyderabad in 2016 (Fig. 4A). Most of the genotypes expressed stability in flowering time across the environments except two genotypes: Sneha (G63) and WGL 915 (G82).
Influence of environments on the trait plant height was variable across the locations. Environment–vector view of the GGE biplot indicated that dry season at Maruteru in 2015 (E1) was more favourable for trait expression while plant growth was reduced during wet season at Hyderabad in 2015 (E2) (Fig. 4B) which is also evident from the box and whisker plots. Dry season at Maruteru in 2014–15 (E1) is the most discriminative and representative environment for favourable plant growth in terms of height. In case of panicle number (or) the number of productive tillers per plant, as observed in case of plant height, the number of productive tillers per plant was also variable and poorly expressed in wet season at Hyderabad in 2015 (E2) (Fig. 4C). Poor expression in panicle number is also depicted in box and whisker plots with genotypes under 3rd quartile having only 8.5 panicles (Fig. 2c). Wet season at Hyderabad in 2015 (E2) is both least discriminating and least representative compared to the other two environments while dry season at Maruteru was relatively favourable for expression of higher number of productive tillers on an average (Fig. 4C). AMMI1 biplots indicated highest number of productive tillers per plant in WGL 347 (G80) followed by Vajram (G73), Pantdhan 12 (G74), Satya (G58), Himalaya 2216 (G23) and NLR 34242 (G48) (Fig. 5a). Average environment coordinate (AEC) view of the GGE biplot indicates that they were all stable in trait expression and hence are ideal genotypes for panicle number (Fig. 6a). Panicle length was poorly expressed in wet season at Hyderabad in 2015 (E2) compared to dry season at Maruteru in 2014–15 (E1) and wet season at Hyderabad in 2016 (E3) (Fig. 4D). AMMI1 biplot depicted highest panicle length (> 26 cm) in aromatic rice line 1 (G85) followed by aromatic rice line 2 (G84) and WGL915 (G82) (Fig. 5d); however, as per AEC view of the biplot, only G85 and G84 were stable with greater stability in the former genotype. G85 with highest panicle length and greater stability can be considered as ideal genotype for the trait panicle length (Fig. 6b). Specific influence by the test environments was observed for this trait which was quite similar to the effect that was observed with panicle number (Fig. 4D).
As per the AMMI1 biplot, high grain number (> 200) was observed in seven genotypes, viz. JGL 11470 (G28), Vasundhara (G76), MTU 1061 (G41), SRAC 34997 (G65), Kavya (G33), JGL 3855 (G31) and Ravi 003 (G2) (Fig. 4E), with stable trait expression in the order of G33 > G31 > G28 > G2 > G76 > G41 > G65 as depicted in AEC view of the GGE biplot. G28 can be considered as the ideal genotype with high grain number and greater stability (Fig. 6c). Environment–vector view of the GGE biplot indicated variable influence of the test environments on grain number. Further, dry season at Maruteru in 2015 (E1)) was least informative and none of the environments represented ideal environment for the favourable expression of grain number. MGD 103 (G38), JGL 3855 (G31), BPT 5204 (G13), JGL 17004 (G29), NLR 30491 (G47), Ramappa (G55) and MTU 3626 (G7) are the generally adapted genotypes (Fig. 4E). Seven genotypes, viz. Tella Hamsa (G69), Nilagiri (G45), VL Dhan (G98), Pokkali (G95), Triguna (G70), PR 118 (G52) and PSB 68 (G53), recorded high TW as per AMMI1 biplot (Fig. 5d). AEC view of the GGE biplot indicated greater stability in terms of TW in Nilagiri (G45) followed by PSB 68 (G53), PR 118 (G52) and VL Dhan (G98) (Fig. 5f). Environment–vector view of the GGE biplot indicated equally informative test environments and wet season at Hyderabad in 2015 (E2) was the most ideal environment for the favourable expression of TW (Fig. 4F).
High grain yield (higher than standard check G6-NDR 359) was observed in eight genotypes, viz. Udaygiri (G71), Vajram (G73), Pantdhan 12 (G74), Konark (G35), MGD 101 (G37), Kavya (G33), Vasundhara (G76) and WGL 11427 (G78), as per AMMI1 biplot (Fig. 5e). However, in terms of stability, only three genotypes were stable and Pantdhan 12 (G74) is the highly stable genotype followed by Konark (G35) and Udaygiri (G71). Considering high yield and stability, Pantdhan 12 (G74) and Konark (G35) are the ideal genotypes (Fig. 6e). Positive correlation was observed among the three test environments which was highly significant between wet season at Hyderabad in 2015 (E2) and wet season at Hyderabad in 2016 (E3) (Fig. 4G). Wet season at Hyderabad in 2016 (E3) was least informative while both E1 (rabi 2015) and wet season at Hyderabad in 2015 (E2) are equally discriminating environments. However, wet season at Hyderabad in 2015 (E2) being closer to the AEA is more representative than dry season at Maruteru in 2014–15 (E1). Hence, E2 can be considered as ideal among the three test environments (Fig. 4G).
Molecular characterization
Amplification of genomic DNA of the 112 rice genotypes with 25 gene-specific SSR markers related to yield traits showed 17 markers with clear amplification and 12 markers were found to show more than two alleles per marker. The polymorphic information (PIC) content varied from 0.51 (S9) to 0.83 (S3204 and RM 18600) with an average of 2.25. PCR-based marker analysis of the yield genes in 112 rice genotypes revealed presence of trait-specific allele in majority of the genotypes with high mean performance for such traits based on stability analysis (Table 4).
All the rice genotypes identified with high panicle number based on AMMI1 biplots were also marker positive to S4603, a gene-specific marker linked to panicle number. All the genotypes with high mean performance for grain number identified from biplot analysis were marker positive to grain number-specific genes, dep1 and APO1 except Ravi003 (G2) and Kavya (G33). However, Kavya (G33) has favourable alleles of EP3 associated with filled grains per panicle. Two genotypes VL Dhan (G98) and Pokkali (G95) with high mean performance for thousand grain weight were marker positive for HGW and SRS, genes for high thousand grain weight. Vajram (G73), Pantdhan 12 (G74), Konark (G35) and WGL 11427 (G78) have EP3 and APO1 meant for high grain number. Vasundhara (G76) has both EP3 and APO1 for grain number and panicle number and WGL 11427 for APO1 for panicle number. It is interesting to note that the genotypes identified with high grain yield, Udaygiri (G71) and Kavya, have favourable alleles for all the traits, viz. thousand grain weight, the number of grains per panicle, the number of filled grains per panicle, productive tillers and plant height with higher yield. Some of the high-yielding germplasm lines like IR 1552, HIM 799, NLR34242 and RNR19186 are having the positive alleles for the traits, the number of grains per panicle and plant height. Additionally, the positive markers linked to the traits, viz. productive tillers and 1000 grain weight, have contributed to their high yield in the lines MTU1010, Vasumati and T309.
Dendrogram with DARwin
Genetic diversity among 112 rice genotypes was determined based on the Jaccard’s pairwise similarity coefficient with the scoring data generated based on marker base pair position specificity. The dendrogram generated from the unweighted pair group arithmetic average (UPGMA) cluster analysis broadly placed 112 rice germplasm into three major clusters (Fig. 7). The cluster II was the largest cluster comprising 44 genotypes with two subdivisions followed by cluster III possessing 40 in two subdivisions and cluster I possessing 28 genotypes in two subdivisions. In cluster I, grain yield varied from a minimum of 19.7 g (Taramati) to a maximum of 24.83 (Udaygiri) with an average of 21.89 g. In cluster II, grain yield varied from a minimum of 19.97 g (Lalithagiri) to a maximum of 23.32 (MGD 101) with an average of 21.49 g. In cluster III, grain yield varied from a minimum of 20.38 g (Tetep) to a maximum of 22.86 (NLR 40065) with an average of 21.45 g. All the stable genotypes for grain yield Pantdhan 12, Konark and Udaygiri identified in the present study had fallen in cluster I, while the stable genotypes for panicle number (WGL 347, Vajram, Pantdhan 12, Satya, Himalaya 2216 and NLR 34242 in both Cluster I and II, panicle length (aromatic rice lines 1 and 2) and thousand grain weight (Nilagiri) in cluster II and grain number (JGL 11470) in cluster III. The details of the genotypes present in each cluster are mentioned in Table 1.
Discussion
Grain yield is a complex quantitative trait with multiple contributing traits highly influenced by genotype × environment interaction effects. The success of any crop improvement programme depends on the identification of superior and stable varieties among the diverse genotypes. A variety can be considered superior, if it has potential for high yield under favourable environments, and at the same time with a greater phenotypic stability. Stability also denotes consistency in rank relative to other cultivars in a given set of environments (Ghritlahre et al. 2011). Wide spread cultivation of rice in various agroecological environments and the unpredicted effects of climate change makes the cultivation of stable and adaptable genotypes more desirable (Bose et al. 2012; Vanave et al. 2014; Balakrishnan et al. 2016). Stability and GEI studies are very important for the efficient breeding and adoption in multi-environment conditions (Kempton et al. 1997; Atlin et al. 2000; IRRI 2006; Liang et al. 2015,).
Correlation coefficient analysis is widely used to measure the degree and direction of relationships between various traits including grain yield (Tiwari et al. 2019). In this study, we found panicle number, grain number and thousand grain weight contributing to grain yield irrespective of the season/location/year similar to the findings of Senguttuvel et al. (2021). Similar to our findings, Karim et al. (2022) also reported positive correlation of the grain yield with panicle number and thousand grain weight; however, there were no such correlations with grain number. On the other hand, correlation of the grain yield with panicle length, plant height and days to flowering was varying across the seasons and environments in the present study similar to the findings of Balakrishnan et al. (2016) and with ecotypes as reported by Li et al. (2019). Contrarily, Karim et al. (2022) observed positive correlation of grain yield with plant height in both the seasons. These results depicted that increasing the number of panicles, grains and thousand grain weight that were stable over all the environments would enhance grain yield and thus could be used as an indirect selection criterion for the overall improvement of grain yield.
In our study, analysis of variance indicated significant differences among the genotypes for all the yield traits studied indicating the presence of vast genetic variability in the experimental material. Mean sum of squares due to various components as well as their linear components including genotype × environment interactions were highly significant for grain yield and component traits. Therefore, stability parameters could be used reliably for predicting genotypic performances. Similar findings were also reported by Pandey et al. (2020); Saidaiah et al. (2011) and Sreedhar et al. (2011) and Wasan et al. (2018). One rabi and two kharif season data were used, and significant seasonal variation was observed for the yield traits. The seasonal variations were mainly attributed to the difference in weather parameters particularly temperature extremities and the number of bright sunshine hours. Similarly, Balakrishnan et al (2016) reported significant seasonal variation for the yield traits. Box plot is a convenient way of graphically depicting variation in a group of numerical data. It displays varieties in samples of a statistical population without making any assumptions of the underlying statistical distribution (McDermott et al. 2012). Several stresses like drought, salinity and extreme temperature greatly affect genotypic performance along with environmental conditions. Experimental plot in wet season at Hyderabad in 2015 (E2) was affected with salinity stress and environment–vector view of the biplot, and box and whisker plots indicated the same in terms of reduced plant height, panicle number and panicle length among the genotypes. Further, a yield reduction of -4.97% and -19.69% over the best check (MTU 1010) was observed in the stress environment in the high-yielding stable genotypes Pant Dhan 12 and Konark, respectively. Panicle number plays an important role in enhancement of the grain yield and is greatly affected by both environmental conditions and management (Sadras et al. 2007; Garcia et al. 2015; Wang et al. 2017). Restricted plant growth in terms of plant height, short panicles and lower number of productive tillers in wet season at Hyderabad in 2015 (E2) could be due to soil conditions as the plot was affected by sodicity. The reason for salinity or sodicity is majorly due to the irrigation water. Similar results were reported by Sumanth et al. 2017; Oniya et al. 2017. Excess salt in soil adversely affects plant growth, development and productivity when osmotic stress reduces water uptake by roots (Munns and Tester 2008). Direct accumulation of salts disturbs metabolic processes and all major morpho-physiological and yield-related traits including tiller number, panicle length, spikelet number per panicle (Khatun et al. 1995), grain filling (Rao et al. 2013), plant biomass (Zeng et al. 2007) and photosynthesis (Ismail et al. 2007; Baker 2008), leading to significantly decreased yield.
Box plots revealed low grain number in dry season at Maruteru in 2014–15. Spikelet sterility and grain chaffiness are generally observed during rabi season due to heat stress. Rice plants at the reproductive stage, including the processes of panicle initiation, male and female gametophyte development, anthesis, pollination and fertilization, are more susceptible to heat stress than at the vegetative stage (Arshad et al 2017; Jagadish et al 2015). Heat stress impairs panicle initiation and spikelet development, leads to deformed floral organs and reduces spikelet number and size (Sita et al. 2017; Xu et al. 2020). Experiment in dry season was conducted with timely irrigation while wet season experiments were conducted with adequate rainfall and supplemental irrigation. Despite the differences in growing seasons and locations, comparable yield levels among the genotypes were observed in dry season at Maruteru in 2014–15 (E1) and wet season at Hyderabad in 2015 (E2). Thus, rainfall had only a secondary impact on grain yield under irrigated ecology. Zhang et al 2019 reported similar secondary impact of the rainfall and temperature on stability of grain yield in rice genotypes. Thus, irrigated ecology maintains a stabilized ecosystem and a microenvironment which support for the uniform expression of genotypes even under variable weather parameters.
As per the stability analysis, three genotypes were stable and high yielding. Pantdhan 12 (G74) was identified as highly stable genotype followed by Konark (G35) and Udaygiri (G71). Considering high yield and stability, Pantdhan 12 (G74) and Konark (G35) are also detected as the most ideal genotypes (Fig. 6e). GGE biplots with an intersection of average environment axis (AEA) among environmental vectors depicted not only the discriminating ability and representativeness of the environments but also the general and specific adaptation of the genotypes to the test environments. Genotypes RP Bio 248 (G18), MTU 1121 (G14), RP Bio-7 K (G19) and IR 64 (G24) had a general adaptation for days to flowering while improved Pusa Basmati (G85), WGL11427 (G78) and Minghui 63 (G100) (Fig. 4A) were the generally adapted genotypes for plant height (Fig. 4B). MGD 103 (G38), JGL 3855 (G31), BPT 5204 (G13), JGL 17004 (G29), NLR 30491 (G47), Ramappa (G55) and MTU 3625 (G7) for grain number (Fig. 4E), improved Pusa Basmati (G85), WGL 11427 (G78) and Minghui 63 (G100) for panicle length (Fig. 4D), WGL 347 (G80), Satya (G58), NLR 40058 (G49), BPT 1235 (G12), Himalaya 799 (G22), P1144 (G4), Saket 4 (G72) and NL 60 (G111) for panicle number are the generally adapted genotypes (Fig. 4C). In case of grain yield, 17 genotypes are falling on either side of average environmental axis and are generally adapted. Udaygiri (G71) and Pantdhan 12 (G74) are close to ideal environment (Fig. 4G), while 7 genotypes, viz. Vajram (G73), Lalithagiri (G37), JGL 11470 (G28), Abhilash (G11), Jaya (G27), NLR 40058 (G49) and WGL11427 (G78), with specific adaptation to E2 and 8 genotypes, Kavya (G33), Ranbir Basmati (G89), Acharmati (G3), Minghiu 63 (G100), WGL32100 (G79), Nilagiri (G45), JGL 3855 (G31) and Badhshabhog (G9), were specifically adapted to E1 (Fig. 4G). For grain yield, which-won-where view of the GGE biplot grouped the environments into two sectors with E2 and E3 falling in the same sector while E1 was independent of them. Udaygiri (G71), followed by Vajram (G73), Pantdhan 12 (G74), improved Pusa Basmati (G85), WGL 11427 (G78) and NDR 359 (G6) were the winning cultivars in E2 while JGL 1798 (G30) followed by Ramappa (G55) were the winning cultivars in E1 (Fig. 6f). Which-won-where biplot indicated the suitability of JGL 1798 to rabi season and suitability of Udaygiri, Vajram and Panthshan 12 to kharif season.
Days to flowering, plant height and thousand grain weight are genotype-specific traits while tiller number, productive tiller number and grain number are greatly influenced by the environmental factors and crop management practices. In the present study, days to flowering is highly genotype-specific as genotypic variance (σ2g) as high as 74.63% is controlling the phenotype while plant height is greatly influenced by the environment and phenotypic variance for thousand grain weight is attributed to unexplained residual effect. Balakrishnan et al. 2016 reported plant height and 1000 grain weight as the most stable traits across the seasons. Contrarily, thousand grain weight was reported as highly unstable by Arumugam et al. 2007. The genotypes with high grain yield and stability in the present study did not show neither high value nor stability for the component traits for grain yield. This clearly signifies the complexity of grain yield and importance of assessing stability of the component traits along with grain yield. Since all the genotypes did not exhibit a uniform stability and response pattern for to the component yield traits, it is difficult to generalize stability for all genotypes relative to the component traits. Difficulty in generalizing the stability of the genotypes relative to all the observations was earlier reported by Ahmad and Masoud (2011). Thus, the stable expression of grain yield is independent of any specific component, but an outcome of interactions of various components which are genotype-specific.
As in our diversity study based on presence of yield-related genes, Hien et al. 2007 also constructed dendrogram using unweighted pair group method with arithmetic mean based on the SSR marker analysis and the tested rice varieties were clustered into major groups. Markers specific to the number of productive tillers and the number of grains per panicle contributed majorly towards higher yield in cluster I, whereas, in cluster II, markers for the number of productive tillers, 1000 grain weight and the number of grains per panicle have contributed to enhanced yield of the germplasm lines. In cluster III, 1000 grain weight-specific markers contributed more towards higher yield. The accessions in three different clusters are distant and some important traits like the number of productive tillers, 1000 grain weight and grains per panicle aid in forming same cluster which can be useful in developing mapping populations for distinct traits as suggested by Lakhar and Tanti (2017). Hence, the germplasm with higher yield in different clusters can be selected based on the respective gene-linked markers for yield-related traits. No significant differences were found in minimum, maximum and average yield among the clusters; however, the most stable genotypes identified in the present study were grouped together in the first cluster. It is interesting to observe no definitive patterns in the trait combinations influencing stability of the grain yield. Traits in different clusters can help in making crosses successfully that might help in plant breeding programme.
Though the associations of genotype and phenotype have been widely studied, the effect of environment on various alleles, interaction and their expression is mostly overlooked in genetic analysis of complex traits like yield. There are only a few reports on the evaluation of the effect of yield genes on yield stability in rice. In the present investigation, the rice genotypes identified with high panicle number based on AMMI and GGE biplots were also marker positive to S4603, a gene-specific marker linked to panicle number indicating the stable marker–trait associations. Almost all the genotypes with high mean performance for the trait grain number identified from biplot analysis were marker positive to dep1 and APO1 genes for grain number. The genotypes identified with high grain yield have favourable allelic combinations for all the yield traits, viz. thousand grain weight, grain number, panicle number and plant height (sd1 + APO1 + dep1 and EP3 + APO1). The genotypes with stability for yield traits and with favourable combination of associated yield genes will serve as potential donors for incorporating stable trait combinations. Kim et al. (2018) evaluated the effect of yield genes and reported a strong genetic gain with the use of grain number enhancing genes in elite indica cultivars. They found no significant difference in grain number per panicle between the two Gn1a alleles while the OsSPL14/WFP allele increased grain number per panicle by 10.6–59.3% across cropping seasons and generations. Reyes et al. (2021) reported significant yield improvement with a combination of Gn1a + OsSPL14 the in NERICA background while the same was ineffective in progenitor background. Pulindala et al. (2022) introgressed four yield genes GS3, GS5, qsw5 and LP1 into Samba Mahsuri (BPT 5204) marker-assisted gene pyramiding and found yield advantage of 22–50% in the introgression lines with a four-gene combination across the environment over the best high-yielding check. Dong et al. (2021) reported enhanced grain yield and plant architecture with targeted mutagenesis of OsPDCD5. They found 6.25–20.13% yield enhancement in 11 popular or newly bred rice cultivars compared to the corresponding wild type in OsPDC5 knockout lines. The presence of allelic combinations and contributions to the stable yield performance help in genomic predictions for crop improvement. Stability analysis models helped in the identification of superior genotypes with both high mean yield and stability coupled with stable expression of associated genes of the component yield traits across the environments. Stable yielding genotypes with combination of associated favourable yield genes can be used in breeding programmes to develop varieties with high yield, greater stability and adaptation.
Conclusion
Across the environments, days to flowering was stable in all the genotypes except Sneha and WGL 915. Dry season at Maruteru in 2014–15 (E1) is the most discriminative and representative environment for favourable plant growth in terms of height, panicle number and panicle length. However, none of the environments represented an ideal environment for the favourable expression of grain number while all the environments were equally informative for thousand grain weight and grain yield. Two aromatic rice lines 1 and 2 were the ideal genotypes for panicle length, and JGL 11470 is the most ideal genotype for grain number. Greater stability for thousand grain weight was observed in Nilagiri. Three genotypes Panthdhan 12, Konark and Udaygiri were the most stable genotypes for grain yield with a favourable combination of associated yield genes sd1 + APO1 + dep1 and EP3 + APO1. Panicle number, grain number and thousand grain weight were contributing to grain yield across the environments. Further, it is interesting to observe grouping of the stable genotypes for grain yield in the first cluster based on yield gene-specific markers. The information on association of yield stability with reported yield genes from this study is useful in marker-assisted breeding studies for yield improvement and can be confirmed with various sets of genotypes under multi-environment testing. The identified superior genotypes might be useful for the development of potential stable genotypes in future breeding programmes. The present study suggests that yield stability could be effectively achieved with targeted improvement of component yield traits associated with favourable alleles.
Data availability
Relevant data are included in this paper and its associated Supplementary Information (SI).
References
Abebe D, Mohammed W, Tesfaye A (2023) Genotype X environment interaction and stability analysis in upland rice (Oryza sativa L.) varieties in Ethiopia. J Crop Sci Biotechnol 26:51–62. https://doi.org/10.1007/s12892-022-00161-5
Ahmad R, Masoud T (2011) Stability analysis of grain yield and its components of rice (Oryza sativa L.) genotypes. Electron J Plant Breed 2:484–487
Allard RW, Bradshaw AD (1964) Implication of genotype × environmental interaction in applied plant breeding. Crop Sci 5:503–506
Arshad MS, Farooq M, Asch F, Krishna JSV, Prasad PVV, Siddique KHM (2017) Thermal stress impacts reproductive development and grain yield in rice. Plant Physiol Biochem 115:57–72
Arumugam M, Rajanna MP, Vidhyachandra B (2007) Stability of rice genotypes for yield and yield components over extended dates of sowing under Cauvery command area in Karnataka. Oryza 44:104–107
Asenjo CA, Bezus R, Acciaresi HA (2003) Genotype environment interactions in rice (Oryza sativa L.) in temperate region using the joint regression analysis and AMMI methods. Cereal Res Commun 31:97–104
Ashikari M, Sakakibari H, Lin S, Matsuaka M (2005) Cytokinin oxidase regulates rice grain production. Science 309(5735):741–745
Atlin GN, Baker RJ, McRae KB, Lu X (2000) Selection response in subdivided target regions. Crop Sci 40:7–13. https://doi.org/10.2135/cropsci2000.4017
Baker NR (2008) Chlorophyll fluorescence: a probe of photosynthesis in vivo. Annu Rev Plant Biol 59:89–113
Balakrishnan D, Subrahmanyam D, Badri J, Krishnam Raju A, Venkateswara Rao Y, Kavitha B, Sukumar M, Malathi S, Revathi P, Padmavathi G, Ravindra Babu V, Neelamraju S (2016) Genotype × environment interactions of yield traits in backcross introgression lines derived from Oryza sativa cv Swarna/Oryza nivara. Front Plant Sci 7:1530. https://doi.org/10.3389/fpls.2016.01530
Bose LK, Nagaraju M, Singh ON (2012) Genotype × environment interaction and stability analysis of lowland rice genotypes. J Agric Sci 57:1–8. https://doi.org/10.2298/JAS1201001B
Cornelius PL, Crossa L, Seyedsadr MS (1996) Statistical test and estimators of multiplicative models for genotype-by-environment interaction. In: Kang MS, Gauch HG (eds) Genotype-by-environment interaction. CRC Press, Boca Raton, pp 199–234
Crossa J, Cornelius PL (1997) Sites regression and shifted multiplicative model clustering of cultivar trials sites under heterogeneity of variances. Crop Sci 37:406–415. https://doi.org/10.2135/cropsci1997.0011183X003700020017x
Crossa J, Cornelius PL, Yan W (2002) Biplots of linear-bilinear models for studying crossover genotype × environment interaction. Crop Sci 42:619–633. https://doi.org/10.2135/cropsci2002.0619
Dong S, Dong X, Han X, Zhang F, Zhu Y, Xin X, Wang Y, Hu Y, Yuan D, Wang J, Huang Z, Niu F, Hu Z, Yan P, Cao L, He H, Fu J, Xin Y, Tan Y, Mao B, Zhao B, Yang J, Yuan L, Luo X (2021) OsPDCD5 negatively regulates plant architecture and grain yield in rice. Proc Natl Acad Sci 118(29):9118. https://doi.org/10.1073/pnas.2018799118
Eberhart SA, Russell WA (1966) Stability parameters for comparing varieties. Crop Sci 6:36–40. https://doi.org/10.2135/cropsci1966.0011183X000600010011x
Fan C, Xing Y, Mao H, Lu T, Han B, Xu C, Li X, Zhang Q (2006) Gs3, a major QTL for grain length and weight and minor QTL for grain width and thickness in rice, encodes a putative transmembrane protein. Theor Appl Genet 112:1164–1171
Finlay KW, Wilkinson GN (1963) The analysis of adaptation in a plant breeding programme. Aust J Agric Res 14:742–754. https://doi.org/10.1071/AR9630742
Francis TR, Kannenberg LW (1978) Yield stability studies in short season-maize. I. A descriptive method for grouping genotypes. Can J Plant Sci 58:1029–1034
Garcia GA, Dreccer MF, Miralles DJ, Serrago RA (2015) High night temperatures during grain number determination reduce wheat and barley grain yield: a field study. Glob Chang Biol 21:4153–4164. https://doi.org/10.1111/gcb.13009
Gauch HG (1988) Model selection and validation for yield trials with interaction. Biometrics 44:705–715. https://doi.org/10.2307/2531585
Gauch HG (2006) Statistical analysis of yield trials by AMMI and GGE. Crop Sci 46:1488–1500
Gauch HG, Zobel RW (1997) Identifying mega-environments and targeting genotypes. Crop Sci 37:311–326. https://doi.org/10.2135/cropsci1997.0011183X003700020002x
Ghritlahre SK, Sarial AK (2011) G × E interaction and adaptability of rice cultivars in SRI and normal production systems. Cereal Res Commun 39(4):589–597
Haji HM, Hunt LA (1999) Genotype x environment interactions and underlying environmental factors for winter wheat in Ontario. Can J Plant Sci 79:497–505
Hien NL, Sarhadi WA, Hirata Y, Oikawa Y (2007) Genetic diversity of morphological responses and relationships among Asia aromatic rice (Oryza sativa L.) cultivars. Tropics 16(4):343–355
Hili J (1975) Genotype-environment interaction: a challenge to plant breeding. J Agric Sci 85:477–499
Huang X, Qian Q, Liu Z, Sun H, He S, Luo D, Xia G, Chu C, Li J, Fu X (2009) Natural variation at the DEP1 locus enhances grain yield in rice. NNat Genet 41:494–497.
Ikeda K, Ito M, Nagasawa N, Kyozuka J, Nagato Y (2007) Rice aberrant panicle organization 1, encoding an F-box protein, regulates meristem fate. Plant J 51:1030–1040.
IRRI (2006) Bringing hope, improving lives: strategic plan 2007–2015. IRRI, Los Banos, CA
Islam MR, Sarker MRA, Sharma N, Rahman MA, Collard BCY, Gregorio GB et al (2015) Assessment of adaptability of recently released salt tolerant rice varieties in coastal regions of South Bangladesh. Field Crops Res 190:34–43. https://doi.org/10.1016/j.fcr.2015.09.012
Ismail AM, Heuer S, Tomson JT, Wissuwa M (2007) Genetic and genomic approaches to develop rice germplasm for problem soils. Plant Mol Biol 65:547–570
Jagadish S, Murty M, Quick W (2015) Rice responses to rising temperatures–challenges, perspectives and future directions. Plant Cell Environ 38:1686–1698. https://doi.org/10.1111/pce.12430
Kang MS (1993) Simultaneous selection for yield and stability in crop performance trials: consequences for growers. Agron J 85:754–757. https://doi.org/10.2134/agronj1993.00021962008500030042x
Karim ST, Begum H, Lopena V, Borromeo T, Virk P, Hernandez JE, Jose E, Hernandez G, Collard BCY, Yoichiro Y (2022) Genotypic variation of yield-related traits in an irrigated rice breeding program for tropical Asia. Crop Environ 1(3):173–181
Kempton RA, Fox PN, Cerezo M (1997) Statistical methods for plant variety evaluation. Chapman and Hall, London, pp 1–8
Khatun S, Rizzo CA, Flowers TJ (1995) Genotypic variation in the effect of salinity on fertility on rice. Plant Soil 173:239–250
Kim CK, Kim J S, Lee GS, Park BS, Hahn JH (2008) PlantGM: a database for genetic markers in rice (Oryza sativa) and Chinese cabbage (Brassica rapa). Bioinformation 3(2):61.
Kim SR, Ramos JM, Hizon RJM, Ashikari M, Virk PS, Torres EA, Nissila E, Jena KK (2018) Introgression of a functional epigenetic OsSPL14/WFP allele into elite indica rice genomes greatly improved panicle traits and grain yield. Sci Rep 8(1):1–12
Kitagawa K, Kurinami S, Oki K, Abe Y, Ando T, Kono I, Yano M, Kitano H, Iwasaki Y (2010) A novel kinesin 13 protein regulating rice seed length. Plant Physiol 51:1315–1329.
Krishnamurthy SL, Pundir P, Singh YP, Sharma SK, Sharma PC, Sharma DK (2015) Yield stability of rice lines for salt tolerance using additive main effects and multiplicative interaction analysis–AMMI. J Soil Saline Water Qual 7(2):98–106
Krishnamurthy SL, Sharma SK, Sharma DK, Sharma PC, Singh YP, Mishra VK, Burman D, Maji B, Bandyopadhyay BK, Mandal S, Sarangi SK, Gautam RK, Singh PK, Manohara KK, Marandi BC, Singh DP, Padmavathi G, Vanve PB, Patil KD, Thirumeni S, Verma OP, Khan AH, Tiwari S, Shakila M, Ismail AM, Gregorio GB, Singh RK (2016) Analysis of stability and genotype–environment interaction of rice genotypes across saline and alkaline environments in India. Cereal Res Commun 44(2):349–360
Li J, Chu H, Zhang Y, Mou T, Wu C, Zhang Q, Xu J (2012) The rice HGW gene encodes a ubiquitin-associated (UBA) domain protein that regulates heading date and grain weight. PloS one 7(3):e34231.
Li R, Li M, Ashraf U, Liu S, Zhang J (2019) Exploring the relationships between yield and yield-related traits for rice varieties released in China from 1978 to 2017. Front Plant Sci 10:543
Liang S, Ren G, Liu J, Zhao X, Zhou M, McNeil D (2015) Genotype by-environment interaction is important for grain yield in irrigated lowland rice. Field Crops Res 180:90–99. https://doi.org/10.1016/j.fcr.2015.05.014
Lin CS, Binns MR, Lefkovitch LP (1986) Stability analysis: where do we stand. Crop Sci 26:894–900
McDermott B, Coe R (2012) An easy introduction to biplots for multi environment trials. Statistical Services Centre, University of Reading, UK and World Agroforestry Centre, Kenya, Nairobi
Munns R, Tester M (2008) Mechanisms of salinity tolerance. Annu Rev Plant Biol 59:651–681
Onyia VN, Okechukwu EC, Atuguwu AI, Akpan NM (2017) Genetic variability studies on twelve genotypes of rice (Oryza sativa L.) for growth and yield Performance in south eastern Nigeria. Not Sci Biol 9(1):110–115
Pandey V, Singh SK, Korada M, Singh DK, Khaire AR, Habde S, Majhi PK (2020) Stability analysis in rice (Oryza sativa L.) genotypes with high grain zinc. Indian J Agric Res 54(6):689–698
Piao R, Jiang W, Harn TH, Choi MS, Qiao Y, Chu SH, Park JH, Woo MO, Jin Z, An Z (2009) Map based cloning of ERECT PANICLE 3 gene in rice. Theor Appl Genet 119:1497–1506.
Pulindala S, Bommisetty R, Withanawasam DMS, Kommana M, Kalluru S, Keerthi I, Chintala S, Vemireddy LR (2022) Enhancement of yield through marker-assisted pyramiding of yield contributing genes in rice (Oryza sativa L.) var. BPT 5204. Euphytica 218:148. https://doi.org/10.1007/s10681-022-03104-x
R Core Team (2012) R: A language and environment for statistical computing. R Foundation for Statistical Computing, Vienna
Rao PS, Mishra B, Gupta SR (2013) Effects of soil salinity and alkalinity on grain quality of tolerant, semi-tolerant and sensitive rice genotypes. Rice Sci 20:284–291
Reyes VP, Angeles-Shim RB, Mendioro MS, Manuel M, Carmina C, Lapis RS, Shim J, Sunohara H, Nishiuchi S, Kikuta M, Makihara D (2021) Marker-assisted introgression and stacking of major QTLs controlling grain number (Gn1a) and number of primary branching (WFP) to NERICA cultivars. Plants 10(5):844. https://doi.org/10.3390/plants10050844
Sadras VO (2007) Evolutionary aspects of the trade-off between seed size and number in crops. Field Crop Res 100:125–138. https://doi.org/10.1016/j.fcr.2006.07.004
Saidaiah P, Kumar SS, Ramesha MS (2011) Stability analysis of rice (Oryza sativa L.) hybrids and their parents. Indian J Agric Sci 81(2):111–115
Senguttuvel P, Sravanraju N, Jaldhani V, Divya B, Beulah P, Nagaraju P, Manasa Y, Prasad AH, Brajendra P, Gireesh C, Anantha MS (2021) Evaluation of genotype by environment interaction and adaptability in lowland irrigated rice hybrids for grain yield under high temperature. Sci Rep 11(1):15825.
Shrestha S, Asch F, Dusserre J, Ramanantsoanirina A, Brueck H (2012) Climate effects on yield components as affected by genotypic responses to variable environmental conditions in upland rice systems at different altitudes. Field Crops Res 134:216–228
Shukla GK (1972) Some statistical aspects of partitioning genotype environmental components of variability. Heredity 29:237–245. https://doi.org/10.1038/hdy.1972.87
Sita K, Sehgal A, Hanumantharao B, Nair RM, Vara Prasad PV, Kumar S, Gaur PM, Farooq M, Siddique KHM, Varshney RK, Nayyar H (2017) Food legumes and rising temperatures: effects, adaptive functional mechanisms specific to reproductive growth stage and strategies to improve heat tolerance. Front Plant Sci 8:1658
Sreedhar S, Dayakar RT, Ramesha MS (2011) Genotype × environment interaction and stability for yield and its components in hybrid rice cultivars (Oryza sativa L.). Int J Plant Breed Genet 5:194–208
Sumanth V, Suresh BG, Jalandhar BR, Srujana G (2017) Estimation of genetic variability, heritability and genetic advance for grain yield components in rice (Oryza sativa L.). Res J Phytochem 6(4):1437–1439
Tariku S, Taddesse L, Bitew M, Asfaw M (2013) Genotype by environment interaction and grain yield stability analysis of rice (Oryza sativa L.) genotypes evaluated in north western Ethiopia. Net J Agric Sci 1:10–16
Tiwari DN, Tripathi SR, Tripathi MP, Khatri N, Bastola BR (2019) Genetic variability and correlation coefficients of major traits in early maturing rice under rainfed lowland environments of Nepal. Adv Agric. https://doi.org/10.1155/2019/5975901
Vanave PB, Apte UB, Kadam SR, Thaware BL (2014) Stability analysis for straw and grain yield in rice (Oryza sativa L.). Electron J Plant Breed 5:442–444
Wang E, Wang J, Zhu X, Hao W, Wang L, Li Q, Zhang L, He W, Lu B, Lin H, Ma H, Zhang G, He Z (2008) Control of rice grain-filling and yield by a gene with a potential signature of domestication. Nat Genet 40:1370–1374
Wang D, Huang J, Nie L, Wang F, Ling X, Cui K (2017) Integrated crop management practices for maximizing grain yield of double-season rice. Crop Sci Rep 7:38982. https://doi.org/10.1038/srep38982
Wasan J, Tidarat M, Sompong C, Bhalang S, Jirawat S (2018) Evaluation of stability and yield potential of upland rice genotypes in North and Northeast Thailand. J Integr Agric 17(1):28–36
Xiong HP, Jiang JG, Yu CM, Guo YL (1992) Study on ramie yield components and their predicting methods. China’s Fibre Crops 3:5–10
Xu J, Henry A, Sreenivasulu N (2020) Rice yield formation under high day and night temperatures-a prerequisite to ensure future food security. Plant Cell Environ 43:1595–1608
Yan W, Hunt LA, Sheng Q, Szlavnics Z (2000) Cultivar evaluation and mega-environment based on the GGE biplot. Crop Sci. 40:597–605
Yan W (2002) Singular-value partitioning in biplot analysis in multi-environment trial data. Agron J 94:990–996
Yan W, Kang MS (2003) GGE biplot analysis: a graphical tool for breeders, geneticists, and agronomists, 1st edn. CRC Press, Boca Raton
Yan W, Tinker NA (2006) Biplot analysis of multi-environment trial data: principles and applications. Can J Plant Sci 86:623–645
Zeng D, Yan M, Wang Y, Liu X, Qian Q, Li J (2007) Du1, encoding a novel Prp1 protein, regulates starch biosynthesis through affecting the splicing of Wx b pre-mRNAs in rice (Oryza sativa L.). Plant Mol Biol 65:501–509
Zhang H, Zhou GS, Liu DL, Wan B, Xiao DP, He L (2019) Climate-associated rice yield change in the northeast China plain: a simulation analysis based on CMIP5 multi-model ensemble projection. Sci Total Environ 666:126–138. https://doi.org/10.1016/j.scitotenv.2019.01.415
Zheng K, Subudhi PK, Domingo J, Maopantay G, Huang N (1995) Rapid DNA isolation for marker assisted selection in rice breeding. Rice Genet Newsl 12:48
Acknowledgements
The authors are thankful to ICAR-Indian Institute of Rice Research (ICAR-IIRR), Regional Agricultural Research Station (RARS), Maruteru, ANGRAU, for providing research facilities.
Funding
Funding for this study was received from the ICAR-Indian Institute of Rice Research (ICAR-IIRR)-Institute/IRC Project-GEY/CI/BR/25: ‘Broadening the genetic base of indica rice and modify plant type by introgressing traits from Tropical japonica’.
Author information
Authors and Affiliations
Contributions
Aleena Dasari carried out phenotyping, genotyping and manuscript draft preparation; Divya Balakrishnan analysed the data and reviewed the manuscript; Santosha Rathod analysed the data; Rao PVR, Reddy LN, Neeraja CN, Vanisree S, Ranjith KN and Sundaram RM provided the facilities for phenotyping and genotyping of the genotypes and Jyothi Badri planned the experiments, conceptualized, drafted, edited and reviewed the manuscript. All the authors read and approved the final manuscript.
Corresponding author
Ethics declarations
Competing interests
The authors have no relevant financial or non-financial interests to disclose.
Additional information
Communicated by Ahmad Mohammad Alqudah.
Supplementary Information
Below is the link to the electronic supplementary material.
Rights and permissions
Springer Nature or its licensor (e.g. a society or other partner) holds exclusive rights to this article under a publishing agreement with the author(s) or other rightsholder(s); author self-archiving of the accepted manuscript version of this article is solely governed by the terms of such publishing agreement and applicable law.
About this article
Cite this article
Dasari, A., Balakrishnan, D., Rathod, S. et al. Multi-environment testing revealed the effect of yield genes on the grain yield stability in diverse rice germplasm. CEREAL RESEARCH COMMUNICATIONS 52, 1127–1144 (2024). https://doi.org/10.1007/s42976-023-00446-7
Received:
Accepted:
Published:
Issue Date:
DOI: https://doi.org/10.1007/s42976-023-00446-7