Abstract
This study investigates three models to predict the torsional strength of FRP-strengthened reinforced concrete (RC) structural beams: artificial neural network (ANN), nonlinear regression model (NLR), and linear regression model (LR). The researchers examined data from over 96 tested FRP-strengthened beams to develop these prediction models. The models account for 10 distinct variables (input parameters), such as the RC beam’s width and height, the FRP sheet’s thickness and elastic modulus, the yield stress of the longitudinal and transverse steels, the compressive strength of the concrete, the effective width of the FRP strips along the beam’s length, the center-to-center spacing of the FRP strips, the angle of wrapping, and the number of FRP layers. The beam’s torsional strength is an appropriate parameter. Several statistical measures, including correlation coefficient (R2), root mean squared error (RMSE), mean absolute error (MAE), Scatter Index (SI), and objective (OBJ) values, were employed to assess the efficiency of the presented models. With R2, RMSE, MAE, OBJ, and SI values of 0.99, 3.07 kN m, 2.41, 2.63, and 0.17 kN m, respectively, the results demonstrated that the ANN model outperformed the other models in predicting the ultimate torsional strength of strengthened RC beams. This study provides an important database that may be used as a benchmark for future predicts of the torsional strength of strengthened RC beams. The influence of each parameter on the torsional strength of these beams was further studied using sensitivity analysis. The results showed a highly accurate prediction of the torsional strength of FRP-strengthened RC beams.
Similar content being viewed by others
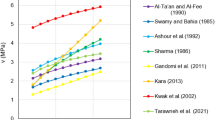
Explore related subjects
Discover the latest articles, news and stories from top researchers in related subjects.Avoid common mistakes on your manuscript.
1 Introduction
It is a challenging and vital task to determine the torsional behavior of reinforced concrete beams, especially when they are strengthened with FRP. However, there has been little investigation on the torsional behavior of these beams, even though there have been many studies and experiments on their bending behavior within specific environmental conditions.
A difficult characteristic of structural behavior is twisting. Torsional stresses develop when shear stresses are not applied through the shear center. The performance of beams reinforced with FRP under torsional loading has been the subject of numerous investigations, with some remarkable laboratory findings.
Standard techniques for calculating Tu may not be compatible with strengthened RC beams. This parameter may be changing, according to ongoing study. To predict the results of experiments, engineers need to use less complicated techniques and mathematical formulas. This demand results from Tu’s depending on several variables. Soft computing techniques might be regarded as an acceptable response. These methods may be used to generate alternatives and solutions for both linear and nonlinear problems when mathematical models are unable to sufficiently explain how the main features of the problem are connected.
Artificial intelligence systems for evaluating and predicting the torsional strength of strengthened RC beams are becoming increasingly popular in the field of RC strengthening research to provide the construction sector with new ideas and strategies for application.
Machine learning has become a powerful technique for assessing the performance of materials and structures. Machine learning has been a potent tool for analyzing structural and material performance over the past decade (Ghafor et al. 2022). Machine learning models are extremely applicable and adaptable because they depend on enormous quantities of measured or accumulated real-world data with characteristics having a wide range of values. ANN and M5P-tree models were two famous soft computing models used in civil and material engineering. Identifying the network parameters, such as the number of hidden layers and neurons, using the ANN algorithm is a time-consuming trial-and-error approach. The M5P algorithm, however, is a reliable method that offers comprehensible linear regression for the inputs and outputs in a nonlinear modeling approach to the splitting process (Almasabha et al. 2022; Alshboul et al. 2022; Kooh et al. 2022; Amlashi et al. 2019).
The main goal of this research is to determine how input parameters, such as the reinforced concrete beam’s width, its height, the FRP sheet’s thickness, its modulus of elasticity, the longitudinal bars’ yielding stress, the transverse bars’ yielding stress, the concrete’s compressive strength, the width of the FRP strips along the length of the beam per center-to-center distance between the FRP strips, the angle at which the FRP is aligned, and the number of layers of the FRP, affect the ultimate torsional strength of RC beam. The following goals were attained through the use of numerous modeling approaches with a significant quantity of experimental data, including 96 tested results: (i) using statistics for assessing how things, such as the reinforced concrete beam’s width, its height, the FRP sheet’s thickness, its modulus of elasticity, the longitudinal bars’ yielding stress, the transverse bars’ yielding stress, the concrete’s compressive strength, the width of the FRP strips along the length of the beam per center-to-center distance between the FRP strips, the angle at which the FRP is aligned, and the number of layers of the FRP, affect the ultimate torsional strength of RC beam; (ii) to ensure that the models may be used by the construction sector without requiring any testing or laboratory evaluation; and (iii) identify the model that accurately predicts the ultimate torsional strength of the RC beam, and utilize that model as a base for further investigation and assessment. The development of soft computing models for predicting the ultimate torsional strength of RC beams for effective application in the construction field is another significant contribution of this work.
2 Literature review
Research on the torsional strength of reinforced concrete beams strengthened with FRP sheets was done by Ghobarah et al. (2002). Different configurations of glass fiber-reinforced polymer (GFRP) and carbon fiber-reinforced polymer (CFRP) were used to strengthen the beams. According to the findings, the full wrap arrangement offered the reinforced concrete beams a performance enhancement that was superior to other configurations. The 45° reinforced beam was also shown to be more effective in pure torsion than the 90° one, as the vertical fibers were kept in tension until failure (Askandar and Mahmood 2019; Askandar et al. 2022).
The effects of glass fiber-reinforced polymer (GFRP) sheets on pure torsion were investigated by Panchacharam and Belarbi (2002). Their study concentrated on several FRP configurations, including end restraint effects, fully wrapped strengthened beams, and U-shaped wrapping. According to the findings, 0° fibers were more ductile and had higher strength than 90° fibers. It was discovered that the continuous wrapping arrangement gave better confinement and was, therefore, more efficient than the strip wrapping option. Due to the poorly regulated shear flow, the U-shaped wrapping configuration was shown to have less resistance. On the other hand, the end restraint strengthened the beam.
To compare the effectiveness of carbon fiber-reinforced polymer (CFRP) and glass-fiber-reinforced polymer (GFRP) sheets for torsional strengthening, Ameli et al. (2005) carried out a laboratory investigation in 2005. Two patterns were put to the test: full-length and strip-form full-wrapped U shapes, as well as full-length and strip-form full-wrapped. The results showed that GFRP-reinforced beams achieved 110% in contrast to the control beam and that the greatest torsional strength improvement with CFRP was 143%. Compared to the U-shaped configuration, the full-wrapped configuration had the highest strength. The study also identified FRP rupture and FRP debonding as two popular failure modes when using FRP sheets. Hii and Al-Mahaidi (2006) studied the torsional behavior of beams with various cross-sections in 2006 (hollow and solid). The cracking and deformation patterns in the beams were seen using photogrammetry. According to the results, the solid cross-section beam had a higher load capacity for cracking, although the total load capacity was nearly equal.
Mohammadizadeh et al. (2009) conducted an experiment in 2009 to investigate the torsional behavior of 16 reinforced concrete beams. One or two thicknesses of CFRP sheets were used to strengthen the beams. The usage of FRP sheets greatly improved the beams’ torsional strength, according to the findings. In contrast to the full wrapping arrangement, the U-shaped wrapping configuration had less of an impact on enhancing the torsional capacity. The application of an artificial neural network for predicting the torsional strength of reinforced concrete beams was investigated by Arslan (2010). To assess the torsional and shear capability of T-beams reinforced with CFRP sheets, Deifalla and Ghobarah (2010) carried out an experiment in 2010.
The effects of applying CFRP on reinforced beams with and without conventional reinforcement were examined by Mahmood and Mahmood (2011). The experiment involved strengthening the beams with a full strip with spacing (d/2) or two U-shaped strips and applying a pure torsional load. According to the findings, reinforced beams had a better torsional performance than unreinforced ones. In addition, the final torsional strength of the beams was raised by 79, 74, and 68%, respectively, by the complete strip, U-shaped strip, and strip torsion configurations.
In order to improve the torsional and bending behavior of RC beams, Tudu (2012) carried out an experimental investigation in 2012. According to the outcomes, the entire wrapping pattern outscored the other models. In 2013, experiments on beams strengthened with GFRP under torsion and bending stresses were conducted by Jariwala et al. (2013). The results exhibited that the full wrapping arrangement was superior to other configurations in terms of enhancing torsional resistance. The addition of corner strips increased the ductility of the beams, and it was discovered that utilizing diagonal FRP strips was more efficient than using vertical strips. Three researchers, Rafeeq (2016), Behera et al. (2016), and Elwan (2017), carried out lab tests in 2016 and 2017 to investigate the effects of increasing the torsional strength of CFRP-reinforced beams.
The application of fiber-reinforced polymers (FRP) has become an innovative method of strengthening RC elements. The load-bearing capacity and durability of concrete structures can be significantly enhanced using FRP materials, such as CFRP. FRP is a great choice for strengthening RC elements in buildings, bridges, and other infrastructure due to its high tensile strength and resistance to corrosion. There will be minimal disruption to the existing structure during installation due to its lightweight design and flexibility (Al-Rousan 2015, 2022; Alhassan et al. 2021; Al-Rousan and Issa 2017; Al-Rousan and Abo-Msamh 2019, 2020). The artificial neural network (ANN) is a computation approach to solving a problem that can generate definitive findings with little effort and expense by simulating the capacity of the human brain to learn from the past and make predictions. In the field of structural and earthquake engineering, ANN has grown significantly in recent years, particularly in discovering correlations between data (Ghafor et al. 2022; Ahmadi et al. 2014; Naderpour et al. 2010, 2011, 2016a, 2011; b; Kheyroddin et al. 2014; Rezazadeh Eidgahee et al. 2015; Shafabakhsh et al. 2010; Naderpour and Alavi 2017; Hosseini Vaez et al. 2017).
The theory of torsion in reinforced concrete beams strengthened with FRP is explored in this study, followed by a discussion of the current regulations in this area. The relationship between input and output data to calculate the ultimate torsional strength is then developed utilizing information from relevant experiments in the field of FRP-reinforced RC beams and by developing prediction models.
In addition, there are limitations to the soft computing methods used to predict Tu for strengthened RC beam characteristics. An ANN model was utilized by Naderpour and Fakharian to calculate the Tu of strengthened RC beams. To create the ANN models, they combined 9 input parameters from the literature with 56 experimental results. They concluded that it is an effective strategy to estimate the Tu of strengthened RC beams employing FRP fiber using an ANN model (Naderpour and Fakharian 2017).
This study was carried out to predict the torsional strength of a strengthened RC beam using three separate models and ten input parameters due to the lack of investigations on the development of various models.
3 Torsion of RC beams strengthened with FRP sheet
A representation of a typical reinforced concrete beam undergoing torsion evaluation is shown in Fig. 1. The hollow cross-section of the beam is subjected to pure torsion. The figure demonstrates how the torsion is supported by concrete, reinforcement, and FRP sheets operating simultaneously.
The full torsional capacity of RC beams strengthened with FRP may be evaluated according to a variety of design codes that consider the superposition principle from both the CFRP and steel reinforcement. The ultimate torsional strength, Tu, of the investigated FRP reinforced beams is obtained by adding the contributions from the fibers and the reinforced concrete beam per Eq. (1):
The design Eq. (2) proposed by ACI 318-19 can be used to determine the ultimate torsional strength of a reinforced concrete beam, Tu,RC (Askandar and Mahmood 2020; Habeeb Askandar and Darweesh Mahmood 2020; ACI440.2R-17 2017; ACI318M-19 2019):
Based on the design model in the 2001 FIB Bulletin-14 (FIB 2001), an externally applied FRP laminate will only improve the torsional strength if it completely encircles the beam’s cross-section, allowing the FRP’s tensile forces of the beam, allowing the tensile forces carried by the FRP on each side of the cross-section to form a continuous loop:
Equations (6) and (7) are used to calculate the contribution of the FRP to the strength Tu,FRP for beams reinforced with U-shaped FRP (FIB 2001):
Ghobarah et al. (2002) calculated the contribution of the FRP to the ultimate torsional strength, Tu,FRP, using Eqs. (8) and (9):
All the variables used in the equations stated above are included in Table 1. The features of the FRP sheets, such as the modulus of elasticity, which can vary depending on the kind of GFRP or CFRP, sheet thickness, effective width, and number of layers, have significant effects on torsional strength. FRP strengthening configurations are shown in Fig. 2.
4 Methodology and models
4.1 Data collection
96 datasets were gathered for this study from numerous literature sources and placed into an excel sheet. The information was then divided into three groups, statistically examined, and sorted at random. 70% of the data were included in the largest group, which was referred to as the training dataset and utilized to create models. The models were tested and validated using the remaining two groups, each of which contained 15% of the datasets (Yu et al. 2018; Qadir et al. 2019). The variables database and the measured torsional strength for each sample are summarized in Table 2. Based on the authors’ searches, the vast majority of earlier research investigating the impact of FRP Sheets, which are used to strengthen RC beams, on torsional strength was gathered.
The input dataset is shown in Table 2 and includes (a) the reinforced concrete beam’s width (mm), (b) its height (mm), (c) the FRP sheet’s thickness (mm), (d) its modulus of elasticity (MPa), (e) the longitudinal bars’ yielding stress (MPa), (f) the transverse bars’ yielding stress (MPa), (g) the concrete’s compressive strength (MPa), (h) the width of the FRP strips along the length of the beam (mm) per center-to-center distance between the FRP strips (mm), (i) the angle at which the FRP is aligned (deg.), and (j) the number of layers of the FRP (no.).
The enhanced RC beam’s torsional strength was predicted using the presented dataset, which included the 10 independent components described above. This prediction was made in comparison to the observed reported Tu (kN m). Utilizing all of the mentioned input characteristics is intended to decrease the quantity of laboratory test batches required to quickly optimize the number of components for a specific Tu. Figure 3 illustrates the method used in this experiment.
4.2 Modeling
It is impossible to draw a direct connection between actual Tu and predicted Tu. Several additional elements and input variables are examined in the data analysis in the following part. These involve the dimensions (width, height, and thickness) of the RC beam; elastic modulus; the yielding stresses of the longitudinal and transverse bars; the compressive strength of the concrete; and factors about the FRP sheet. These parameters are the number of layers, the alignment angle, the width of the strips along the length of the beam, and the distance between the centers of the strips. In order to measure the impact of each parameter on Tu, three distinct soft computing approaches are offered, as shown below.
4.2.1 Linear relationship model (LR)
As previously stated, the goal of this work is to develop a model that assesses the impact of the greatest possible number of parameters on the Tu of strengthened RC beam. Equation (10) illustrates the use of linear regression as a general technique for evaluating Tu:
where \({\alpha }_{1}\) and \({\alpha }_{2}\) represent the model’s inputs, ultimate torsional strength (Tu), and FRP thickness (tFRP), respectively. After taking all the perimeters influencing the torsional strength, the equation becomes as shown in Eq. (11):
Here, b stands for a reinforced concrete beam’s width in millimeters, h for its height in millimeters, and tfrp for the thickness of FRP in millimeters. wfrp stands for width of FRP strips along the length of the beam (mm); Sf stands for center to center spacing of FRP strips (mm); \({\theta }_{{\text{FRP}}}\) stands for angle of FRP alignment (degree); and nlayer stands for FRP number of layers. Efrp stands for modulus of elasticity of the FRP sheet (MPa); fyl stands for yielding stress of longitudinal bars (MPa); fyt stands for yielding stress of transverse bars.
The model’s parameters are \({\alpha }_{1}, {\alpha }_{2}, {\alpha }_{3}, {\alpha }_{4}, {\alpha }_{5}, {\alpha }_{6}, {\alpha }_{7}. {\alpha }_{8}, {\alpha }_{9}, {\alpha }_{10}, {\text{and}} {\alpha }_{11}\). Equation (11) can be used to expand Eq. (10) since all factors can be altered linearly. Tu may be influenced by a variety of elements that interact with one another, but this is not always the case. To accurately predict the torsional strength, the model must be updated frequently.
4.2.2 Nonlinear model (NLR)
Part of basic, Eq. (12) can be used to create an NLR model (Ghafor et al. 2022). Equation (3) can be used to predict the Tu by stating the relationship between the various components in Eqs. (10) and (11):
The LR model’s parameters are the same as those that were previously established. In addition, in a similar way to the LR model, the sum of error squares and the least square method was employed to calculate the values of each parameter using the EXCEL program and a solver.
4.2.3 ANN model
The opposite of feed-forward neural networks is an artificial neural network (ANN) (Ghafor et al. 2022). Input, output, and hidden layers make up the three different layers that comprise these networks. The input layer is in charge of receiving input data; the output layer is in charge of creating predictions and categorizations; and the hidden layer (or layers) stands in between the input and output levels, allowing data to transfer from one layer to the next.
Trial cycles show that the number of hidden layers is not constant and can be altered to enhance the model’s performance. In order to identify the ideal system configuration, the study’s authors analyzed a number of factors, including the number of hidden layers, neurons, momentum, learning rate, and iterations.
They discovered that when the ANN had one hidden layers, each with 5 neurons (as shown in Fig. 4), 2000 iterations, a learning rate of 0.2, and a momentum of 0.1, the maximum efficiency was attained. The lowest MAE and RMSE values, as well as the highest R2 value, were obtained by these settings. Equations (13)–(15) (Ghafor et al. 2022; Quinlan 1992; Malerba et al. 2004) provide the equations for the ANN model.
Identify the best-hidden layer and neurons for an ANN model based on the model’s R2, MAE, and RMSE performances, as stated in Tables 2 and 3.
From linear node 0:
From sigmoid node 1:
From sigmoid node 2:
4.3 Assessment criteria for the developed models
A variety of indicators were used to evaluate the proposed models’ efficacy, and the outcomes were computed using the equations provided. To ensure a thorough and comprehensive examination of a model’s performance, it is crucial to apply multiple evaluation methodologies. A more thorough evaluation of the model’s capabilities and constraints is made possible by the use of a variety of metrics:
According to the earlier formulations, Tp and Ta stand for the predicted and actual values of the route pattern, respectively. a′ and p′, respectively, represent the means of the actual and predicted values. The letters tr, tst, and N stand for trained datasets, tested datasets, and the number of patterns (collected data) in the connected dataset, respectively. In terms of the SI parameter, a model performs (poorly) when it is larger than 0.3, (fairly) when it is between 0.2 and 0.3, (well) when it is between 0.1 and 0.2, and (excellently) when it is less than 0.1 (Ghafor et al. 2022; Li et al. 2013).
5 Results and analysis
5.1 The LR model
The relation between measured and predicted Tu for both training and assessment is shown in Fig. 5a, b. The values of each parameter in the current model were calculated using the sum of error squares and the least squares method. This model suggests that the Tu of strengthened RC beams is greatly influenced by the FRP sheet thickness and the number of layers. Equation (21) illustrates the LR model equation with different weight parameters:
The tFRP has the most influence on the Tu, as shown in the equation above. This might be in line with the experimental results that have been published in the literature. The assessment parameters for this model’s R2, RMSE, and MAE are 0.844, 7.51, and 5.48 MPa, respectively.
Furthermore, as shown in Sect. 5, the current model’s OBJ and SI values for the training dataset are 7.09 and 0.37, respectively.
5.2 NLR model
As a training and testing dataset, Fig. 6a, b illustrates the relation between predicted and real Tu. The most significant factors affecting the Tu, according to the current model, are the angle of FRP alignment (θFRP), FRP thickness (tFRP), FRP number of layers (nlayer) and width of FRP strips throughout the length of the beam per Center to center spacing of FRP strips (Wf/Sf). This was supported by numerous experimental programs from earlier studies, as shown in Table 2. Equation (22), which offers a formula for the NLR model with various variable parameters, states as follows:
A measurement of this model’s R2, RMSE, and MAE is 0.9645, 3.61, and 2.75 MPa. The OBJ and SI values for the training dataset are 3.44 and 0.18, respectively.
5.3 ANN model
Comparison of predicted and actual Tu for training and testing datasets is shown in Fig. 7a, b. The training datasets have an error line of + 5 and − 18%, whereas the testing data have an error line of + 12 and − 15%, which is better than the other suggested models. The assessment parameters for R2, RMSE, and MAE are 0.9864, 3.07, and 2.40, respectively. The current model’s OBJ and SI values for the training set are 2.63 and 0.17, respectively.
6 Model comparisons
To assess how well each model predicted the torsional strength of the strengthened RC beam, statistical indicators from Sect. 5 were employed. Figures 8, 9 and 10 for R2 values, RMSE, and MAE, respectively, reveal that the ANN model has a higher R2 and lower RMSE and MAE values when compared to the LR and NLR. Based on testing datasets, Fig. 11 displays model Tu estimates for strengthened RC beams. Figure 12 also displays the residual error for all models that utilize the training and testing datasets. The estimated and predicted Tu evaluations in Figs. 13 and 14 for the ANN model are comparable, indicating that the ANN model outperforms other models.
The OBJ values for all developed models are displayed in Fig. 13. The pressures for the LR, NLR, and ANN models are 7.09, 3.40, and 2.63 kN m, respectively. The OBJ value of the ANN model was 29 and 170% less than that of the NLR model, and NLR model, respectively. This further illustrates that for beams strengthened with FRP sheets, the ANN approach is more reliable in predicting the Tu.
The SI values for the supplied models during the training and testing processes are shown in Fig. 14. The figure shows that, based on their performance as determined by the Scatter Index (SI) values, the three machine learning models linear regression (LR), non-linear regression (NLR), and artificial neural networks (ANN) are compared in this study. The observed SI values for the data-trained set were 0.37 for LR, 0.18 for NLR, and 0.17 for ANN. On the other hand, it was discovered that the SI values for the data-tested set were 0.18 for ANN, 0.30 for NLR, and 0.44 for LR. Noteworthy SI values include 0 and 0.1, which denote excellent performance, 0.1–0.2, which denotes good performance, 0.2–0.3, which denotes fair performance, and 0.3–0.4, which denotes poor performance. In the data-tested set, the ANN model exhibited good performance based on these classifications, while the NLR and LR models performed fair and poorly, respectively. In the training phase, the SI value of the ANN model is 118% lower than that of the LR model, and in the testing phase, it is 144% lower.
In addition, the ANN model revealed lower SI values in the NLR model, including decreases of 6 and 67% in training and testing, respectively. This demonstrated that the ANN model can predict the Tu of FRP-strengthened RC beams more accurately and competently than NLR, and LR models. Hoang et al. concluded the same result. In 2023, the flexural moment of FRP-strengthened RC beams was predicted using ANN (Le Hoang et al. 2023).
In conclusion, it is important to note that any models with allowable errors can be utilized to predict the Tu of FRP-enhanced RC beams without the need for experimental programs. In addition, compared to other models, the ANN model can produce more accurate findings.
7 Sensitivity analysis
The models that were developed show an outstanding ability to accurately predict the ultimate torsional strength (Tu) of RC-strengthened beams. However, it is also crucial to explain how the model’s output varies depending on the parameters that were selected. This feature significantly contributes to the development of user confidence in the predictive model.
Establishing a mechanistic understanding of the link between input and output variables requires conducting sensitivity analysis. This study enables the removal of unimportant factors by assessing the effects of various input parameters on the result. As a result, this lessens model complexity and minimizes training time.
Various unique training datasets were used for the sensitivity analysis, each of which only contained one input variable that was retrieved at a time. Specific evaluation criteria, such as R2, RMSE, and MAE, were uniquely constructed for each training dataset. The calculation of the relative contribution of each model parameter was then done. Figure 15 shows the results of the sensitivity analysis.
The results of this investigation overwhelmingly support the concept that the θFRP is the most important factor influencing Tu prediction.
8 Conclusion
Significant time and cost savings can be achieved by increasing the prediction models’ accuracy and reliability for the torsional strength of RC beams. This study investigated 96 different strengthening configurations by analyzing data from earlier studies. Important conclusions established by this investigation are as follows:
-
1.
The models developed in this study generally provide an efficient method to predict the torsional strength of FRP-strengthened reinforced concrete (RC) beams, particularly the ANN model. As a result, fewer substantial experimental experiments and trial batches in the lab may be performed.
-
2.
Based on the statistical evaluation and sensitivity analysis, the ANN model outperforms the other two models. For training and testing datasets, the model’s R2 values are 0.99, and 0.93 respectively. Furthermore, the ANN model’s training dataset has additional sensitivity indicators for the RMSE, MAE, OBJ, and SI, which are 3.07 MPa, 2.41 MPa, 2.63, and 0.17, respectively. Thus, the ANN model is appropriate to be utilized in the preliminary design of the torsional strength of FPR-strengthened RC beams and possesses a higher degree of generality and predictive capacity.
-
3.
The predicted torsional strengths of the FRP-strengthened RC beam with the ANN model were within + 5 and − 18% of the measured torsional strength of the FRP-strengthened RC beam for the training datasets. However, this value was increased to + 20 and − 45% for the other remaining models.
-
4.
The obtained results show that the angle of FRP alignment (θFRP), and the width of FRP strips per c/c spacing of FRP strips (wf/Sf) are the most significant parameters for predicting the torsional strength of the FRP-strengthened RC beams.
Data availability
All data are available within the study.
References
ACI318M-19 (2019) Building code requirements for structural concrete. American Concrete Institute, Farmington Hills
ACI440.2R-17 (2017) Design and construction of externally bonded FRP systems for strengthening concrete structures. American Concrete Institute, Farmington Hills
Ahmadi M, Naderpour H, Kheyroddin A (2014) Utilization of artificial neural networks to prediction of the capacity of CCFT short columns subject to short term axial load. Arch Civ Mech Eng 14(3):510–517
Alhassan M, Al-Rousan R, Ababneh A (2021) Anchoring of the main CFRP sheets with transverse CFRP strips for optimum upgrade of RC beams: parametric experimental study. Constr Build Mater 293:123525
Almasabha G et al (2022) Machine learning algorithm for shear strength prediction of short links for steel buildings. Buildings 12(6):775
Al-Rousan R (2015) Effect of CFRP schemes on the flexural behavior of RC beams modeled by using a nonlinear finite-element analysis. Mech Compos Mater 51:437–446
Al-Rousan R (2022) Influence of opening sizes on the flexural behavior of heat-damaged reinforced concrete slabs strengthened with CFRP ropes. Case Stud Constr Mater 17:e01464
Al-Rousan R, Abo-Msamh I (2019) Bending and torsion behaviour of CFRP strengthened RC beams. Mag Civ Eng 8(92):48–62
Al-Rousan R, Abo-Msamh I (2020) Impact of anchored CFRP on the torsional and bending behaviour of RC beams. Mag Civ Eng 4(96):79–93
Al-Rousan RZ, Issa MA (2017) Flexural behavior of RC beams externally strengthened with CFRP composites exposed to severe environment conditions. KSCE J Civ Eng 21:2300–2309
Alshboul O et al (2022) Optimization of the structural performance of buried reinforced concrete pipelines in cohesionless soils. Materials 15(12):4051
Ameli M, Ronagh H, Dux P (2005) Experimental investigations on FRP strengthening of beams in torsion. FRP Compos Civ Eng 587–592
Amlashi AT et al (2019) Soft computing based formulations for slump, compressive strength, and elastic modulus of bentonite plastic concrete. J Clean Prod 230:1197–1216
Arslan MH (2010) Predicting of torsional strength of RC beams by using different artificial neural network algorithms and building codes. Adv Eng Softw 41(7–8):946–955
Askandar N, Mahmood A (2019) Comparative investigation on torsional behaviour of RC beam strengthened with CFRP fabric wrapping and near-surface mounted (NSM) steel bar. Adv Civ Eng 2019:1–15
Askandar NH, Mahmood AD (2020) Torsional strengthening of RC beams with continuous spiral near-surface mounted steel wire rope. Int J Concr Struct Mater 14(1):7
Askandar NH, Mahmood AD, Kurda R (2022) Behaviour of RC beams strengthened with FRP strips under combined action of torsion and bending. Eur J Environ Civ Eng 26(9):4263–4279
Behera GC, Rao TG, Rao C (2016) Torsional behaviour of reinforced concrete beams with ferrocement U-jacketing—experimental study. Case Stud Constr Mater 4:15–31
Chalioris CE (2008) Torsional strengthening of rectangular and flanged beams using carbon fibre-reinforced-polymers—experimental study. Constr Build Mater 22(1):21–29
Deifalla A, Ghobarah A (2010) Strengthening RC T-beams subjected to combined torsion and shear using FRP fabrics: experimental study. J Compos Constr 14(3):301–311
Elwan S (2017) Torsion strengthening of RC beams using CFRP (parametric study). KSCE J Civ Eng 21(4):1273–1281
FIB (2001) Externally bonded FRP reinforcement for RC structures. International Federation for Structural Concrete (fib), Lausanne
Ghafor K et al (2022) Computing models to predict the compressive strength of engineered cementitious composites (ECC) at various mix proportions. Sustainability 14(19):12876
Ghobarah A, Ghorbel M, Chidiac S (2002) Upgrading torsional resistance of reinforced concrete beams using fiber-reinforced polymer. J Compos Constr 6(4):257–263
Habeeb Askandar N, Darweesh Mahmood A (2020) Torsional strengthening of RC beams with near-surface mounted steel bars. Adv Mater Sci Eng 2020:1–11
Hii AK, Al-Mahaidi R (2006) Experimental investigation on torsional behavior of solid and box-section RC beams strengthened with CFRP using photogrammetry. J Compos Constr 10(4):321–329
Hosseini Vaez SR, Naderpour H, Barati M (2017) Estimating the behavior of RC beams strengthened with NSM system using artificial neural networks. J Struct Constr Eng 4(4):16–28
Jariwala VH, Patel PV, Purohit SP (2013) Strengthening of RC beams subjected to combined torsion and bending with GFRP composites. Procedia Eng 51:282–289
Kandekar S, Talikoti R (2018) Study of torsional behavior of reinforced concrete beams strengthened with aramid fiber strips. Int J Adv Struct Eng 10(4):465–474
Kheyroddin A, Naderpour H, Ahmadi M (2014) Compressive strength of confined concrete in CCFST columns. J Rehabil Civ Eng 2:71–80
Kooh MRR et al (2022) Machine learning approaches to predict adsorption capacity of Azolla pinnata in the removal of methylene blue. J Taiwan Inst Chem Eng 132:104134
Le Hoang TT et al (2023) An artificial neural network for predicting the ultimate bending moments in reinforced concrete beams with fiber-reinforced polymer strengthening. Asian J Civ Eng 1–11
Li M-F et al (2013) General models for estimating daily global solar radiation for different solar radiation zones in mainland China. Energy Convers Manag 70:139–148
Ma S, Bunnori NM, Choong KK (2016) Evaluation of ultimate strength of reinforced concrete beams strengthened with FRP sheets under torsion. In: MATEC web of conferences. EDP Sciences
Mahmood MN, Mahmood AS (2011) Torsional behavior of prestressed concrete beams strengthened with CFRP sheets. In: 16th international conference on composite structures (ICCS 16)
Malerba D et al (2004) Top-down induction of model trees with regression and splitting nodes. IEEE Trans Pattern Anal Mach Intell 26(5):612–625
Mohammadizadeh MR et al (2008) Behavior of high-strength concrete beams strengthened with CFRP sheets in torsion. In: FRP composites in civil engineering: proceedings of the 4th international conference on FRP composites in civil engineering, 22–24 July 2008, Zurich, Switzerland
Mohammadizadeh MR, Fadaei M, Rounagh H (2009) Improving torsional behaviour of reinforced concrete beams strengthened with carbon fibre reinforced polymer composite. Iran Polym J 18
Naderpour H, Alavi S (2017) A proposed model to estimate shear contribution of FRP in strengthened RC beams in terms of adaptive neuro-fuzzy inference system. Compos Struct 170:215–227
Naderpour H, Fakharian P (2017) Predicting the torsional strength of reinforced concrete beams strengthened with FRP sheets in terms of artificial neural networks. J Struct Constr Eng 10
Naderpour H, Kheyroddin A, Amiri GG (2010) Prediction of FRP-confined compressive strength of concrete using artificial neural networks. Compos Struct 92(12):2817–2829
Naderpour H et al (2011) Estimating the behavior of FRP-strengthened RC structural members using artificial neural networks. Procedia Eng 14:3183–3190
Naderpour H, Fakharian P, Hosseini F (2016a) Prediction of behavior of FRP-confined circular reinforced concrete columns using artificial neural network. In: 8th Natl Conf Concr. Tehran, Iran
Naderpour H, Vosughifar H, Ghobakhloo E (2016b) Evaluation of effective parameters on wave diffraction of far-fault ground motions using artificial neural networks. Sharif J Civ Eng 32(1.1):13–23
Panchacharam S, Belarbi A (2002) Torsional behavior of reinforced concrete beams strengthened with FRP composites. In: First FIB Congress, Osaka, Japan
Qadir W, Ghafor K, Mohammed A (2019) Characterizing and modeling the mechanical properties of the cement mortar modified with fly ash for various water-to-cement ratios and curing times. Adv Civ Eng 2019
Quinlan JR (1992) Learning with continuous classes. In: 5th Australian joint conference on artificial intelligence. World Scientific
Rafeeq R (2016) Torsional strengthening of reinforced concrete beams using CFRP composites. Portland State University, Portland
Rezazadeh Eidgahee D, Fasihi F, Naderpour H (2015) Optimized artificial neural network for analyzing soil-waste rubber shred mixtures. Sharif J Civ Eng 31(1.1):105–111
Shafabakhsh G, Naderpour H, Fasihi F (2010) Optimized ANN algorithm for analyzing the road flexible pavements. J Model Eng 8(21):45–56
Shafabakhsh G, Naderpour H, Noroozi R (2011) Optimized ANN algorithm for analyzing the road rigid pavements. J Transp 3(1):43–54
Soluit AK et al (2007) Torsional behavior of RC beams strengthened with fiber reinforced polymer sheets. Eng Res J 114:102–119
Tudu C (2012) Study of torsional behaviour of rectangular reinforced concrete beams wrapped with GFRP
Yu K, Yu J, Lu Z (2018) Mechanical characteristics of ultra high performance strain hardening cementitious composites. Strain-hardening cement-based composites: SHCC4 4. Springer, Berlin
Zhang J, Lu Z, Zhu H (2001) Experimental study on the behaviour of RC torsional members externally bonded with CFRP. In: FRP composites in civil engineering. Proceedings of the international conference on FRP composites in civil engineering. Hong Kong Institution of Engineers, Hong Kong Institution of Steel Construction
Funding
This research received no external funding.
Author information
Authors and Affiliations
Contributions
Conceptualization, investigation, writing—original draft, NHA; methodology, resources, GBJ; validation, writing—review and editing, GHA. All the authors have read and agreed to the published version of the manuscript.
Corresponding author
Ethics declarations
Conflict of interest
The authors declare no conflict of interest.
Additional information
Publisher's Note
Springer Nature remains neutral with regard to jurisdictional claims in published maps and institutional affiliations.
Rights and permissions
Springer Nature or its licensor (e.g. a society or other partner) holds exclusive rights to this article under a publishing agreement with the author(s) or other rightsholder(s); author self-archiving of the accepted manuscript version of this article is solely governed by the terms of such publishing agreement and applicable law.
About this article
Cite this article
Askandar, N.H., Jumaa, G.B. & Ahmed, G.H. Modeling for torsional strength prediction of strengthened RC beams. Multiscale and Multidiscip. Model. Exp. and Des. 7, 2535–2553 (2024). https://doi.org/10.1007/s41939-023-00353-8
Received:
Accepted:
Published:
Issue Date:
DOI: https://doi.org/10.1007/s41939-023-00353-8