Abstract
Over the past thirty years, Nashik, India, witnessed rapid urbanization, elevating Land Surface Temperatures (LSTs). This study presents a comprehensive approach for analyzing the spatiotemporal dynamics of LST in Nashik by utilizing Thermal Infrared Radiation bands from LANDSAT datasets. Regional LST maps from mid-May in 1992, 2003, 2013, and 2022, produced using the single channel algorithm, analyze LST changes and distributions. Land Cover Maps are developed to analyse the LST changes across different Land Classes. Further, statistical relationships are established across LSTs and landscape indicators like Normalized Difference Built-Up Index (NDBI), Normalized Difference Bareness Index (NDBaI), Normalized Difference Vegetation Index (NDVI), and Normalized Difference Water Index (NDWI). A consistent increase in citywide LST is detected as the area coverage for hottest (40–45)°C LST band grew from 30.47 km2 in 1992 to 132.16 km2, 141.47 km2 and 174.85 km2 in 2003, 2013 and 2022 respectively. In contrast the cooler (30–40)°C band coverages fell from 305.39 km2 in 1992 to 203.12 km2, 194.66 km2and 144.82 km2 in 2003, 2013 and 2022 respectively. In 2022, an unprecedented 16.25 km2 area experienced extreme LST (45–50)°C, a trend unseen in prior decades. Noteworthy, Built-up areas and Barelands showed much higher (40–45)°C LSTs than vegetated areas and water bodies (30–40)°C. Statistical relations also affirm these patterns: Stronger LST corelations (ρ = 0.66) to NDBI indicate higher temperatures in densely built-up areas. In comparison, negative LST correlations with NDVI (ρ = -0.61) and NDWI (ρ = -0.66) signify cooler zones with vegetation and water bodies. These findings emphasize water-rich areas with vegetation moderating temperatures, while built-up areas elevate heat. Thus, municipalities must prioritize awareness, green spaces, water conservation, cooling technologies, and zoning regulations to counteract this warming trend.
Similar content being viewed by others
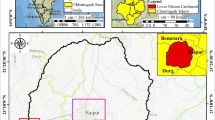
Avoid common mistakes on your manuscript.
Introduction
Rapid urbanization in various Indian cities, driven by growing urban populations seeking residences and better economic opportunities, has led to substantial changes, converting natural landscapes into vast urban expanses (Kumari et al. 2020; Chanu et al. 2021). Urbanization, industrialization, and infrastructure advancements have a profound impact on urban environmental systems, modifying Land Use Land Cover (LULC) and changing energy interactions at the Earth's surface. This rapid alteration of the natural landscape transforming vegetation, agriculture, trees, and forests into impermeable surfaces like concrete and asphalt during urban expansion diminishes albedo and surface reflectivity (Gupta et al. 2020; Galve et al. 2022). Urban surfaces, unlike natural landscapes with higher surface albedo, absorb more sunlight, resulting in increased solar radiation absorption and elevated Land Surface Temperature (LST). Moreover, the transition from vegetated areas, which usually provide cooling effects through evapotranspiration and shading, to heat-absorbing urban structures significantly contributes to heightened heat storage within these surfaces, further exacerbating the increase in LST (Chanu et al. 2021; Gupta et al. 2020; Das et al. 2020).
Higher LSTs within city limits also exemplifies the Urban Heat Island (UHI) which negatively impacts the urban environment and public health. UHIs characterize urban areas that exhibit higher temperatures than surrounding rural regions due to human-induced alterations to the city's built environment (Kumar et al. 2021; Gupta et al. 2021). UHIs exacerbate the energy demand for cooling buildings, straining existing electrical grids and increasing energy consumption and greenhouse gas emissions. Further, high urban LSTs and UHI phenomenon amplifies health causing heat-related illnesses and respiratory problems, particularly affecting vulnerable populations and potentially increasing heat-related fatalities (Shukla and Jain 2019; Chaturvedi et al. 2022). Besides, UHIs can disrupt the natural water cycle, as higher temperatures increase evaporation rates and affect rainfall patterns potentially leading to reduced water availability and drought conditions in urban areas (Kumar et al. 2021; Lakra and Sharma 2019). UHI also worsens social disparities, disproportionately affecting economically disadvantaged communities by reducing their access to cooling resources and green spaces, heightening health and social inequalities (Mathew and S. P, and S. Khandelwal 2021).
LSTs rise and UHI arise from increased urban density and infrastructure, including tall buildings with large surface areas that absorb and store heat. Heat-absorbing materials like concrete and asphalt exacerbate the UHI effect by retaining and slowly releasing heat (Shukla and Jain 2021; Saleem et al. 2020). The scarcity of vegetation, such as parks and trees, in urban areas disrupts natural cooling mechanisms, leading to higher LSTs. This effect is visible by studying the relationships between the Normalized Difference Vegetation Index (NDVI) and LST. NDVI, serving as a measure of vegetation density and health, underscores the influence of green cover on surface temperatures as higher NDVI values in areas abundant with vegetation, such as forests, parks, and agricultural lands, exhibit strong negative correlations with LSTs (Guha 2021; Malik et al. 2019; Biswas and Ghosh 2022). Human activities, like industries, vehicles, and air conditioning, contribute significantly to UHIs by generating additional heat. Further, declining urban water bodies worsen UHI (Shukla and Jain 2019; Moumane, et al. 2021; Tariq et al. 2022). Natural water bodies (lakes, ponds, rivers) cool surroundings through evaporation and transpiration. However, urbanization replaces or covers them, reducing cooling capacity (Gupta et al. 2019). Human activities' influence is evident through the assessment of Normalized Difference Built-Up Index (NDBI) and its inter-relationships with LST. NDBI assesses built-up areas and urban growth, offering insights into surfaces occupied by human-made structures. Surfaces with higher NDBI values, like roads, buildings, and paved areas, correspond to elevated LST values, highlighting the impact of urbanization and impervious surfaces on higher temperatures in urban environments (Chetia et al. 2020; Maity et al. 2020). Further, besides local effects rising LSTs are also linked to global climate change as rising global temperatures intensify the UHI effect, posing challenges in managing heat-related risks and energy consumption (Halder et al. 2021).
Urbanization lead transformation of urban landscape results in elevated LSTs. However, pace of LST change is not uniform and depends on regional characteristics. Thus, periodically monitoring the spatial and temporal variations of LST dynamics can help identify the magnitude of LST change across different time spans and also pinpoint the most vulnerable areas experiencing the highest LST rise (Harod et al. 2021; Thakur et al. 2021). This vital information is crucial for prioritising the allocation of urban resources. However, physically examining LSTs in urban areas is challenging, labour-intensive, and susceptible to errors caused by both system limitations and human factors. Moreover, the scarcity of historical surface temperature datasets hinders the ability to conduct comprehensive comparative LSTs assessments (Chaturvedi et al. 2022; Das and Angadi 2020). In comparison, Remote Sensing (RS) offers a convenient approach to monitor LSTs, providing valuable data and insights into surface temperature patterns and their distribution within urban areas (Parmar et al. 2021; Guha and Govil 2022). The evolution of remote sensing, particularly through satellite-based observations facilitated by LANDSAT, MODIS (Moderate Resolution Imaging Spectroradiometer), and Sentinel series satellites, has streamlined the periodic analysis of environmental changes in urban regions. When combined with Geographic Information Systems (GIS) and spectral indices, these technologies enable precise monitoring of LST alterations (Sam and Balasubramanian 2023; Nabizada, et al. 2022). RS leverages Thermal Infrared Radiation (TIR) sensors on satellites, allowing comprehensive monitoring and analysis of LSTs on a large scale by capturing intricate thermal information emitted from the Earth's surface (Neog 2021). This technological capability supports observing temporal changes in UHIs across seasons and years, offering a longitudinal view of LST elevation and pinpointing hotspots within urban areas. The use of long-term Remote Sensing datasets also aids in recognizing trends and assessing the effectiveness of implemented measures for mitigating urban heat over extended periods (Thakur et al. 2021; Gohain et al. 2020).
Further, RS facilitates the analysis of land surface characteristics and land cover changes that influence LSTs. It identifies specific land use and cover types linked with increased surface temperatures, such as impermeable surfaces, bare soil, or limited green spaces. Further, RS GIS data, particularly multispectral imagery, enables LST estimation and its correlation with indices like Normalized Difference Vegetation Index (NDVI), Normalized Difference Built-Up Index (NDBI), Normalized Difference Bareness Index (NDBaI) and Normalized Difference Water Index (Nabizada, et al. 2022; Guha and Govil 2021; Mukherjee and Singh 2020). These indices help predict LST distribution by delineating built-up areas, vegetation density, and water content. In urban regions, higher NDBI values indicate UHI effect, while lower NDVI and higher NDBaI values lead to increased LST due to reduced vegetation cover. Water bodies with high NDWI values exhibit lower LST due to the cooling effect (Jana, et al. 2020; Vani and Prasad 2020). However, site-specific analysis remains crucial owing to the complexities and variations in these relationships. To summarize, RS-GIS furnishes essential insights for urban planners and policymakers, empowering them to prioritize interventions that can alleviate the escalation of LSTs and UHIs.
This study analysis the spatiotemporal LST changes in a rapidly developing city of Nashik, India during the past three decades. Nashik’s rapid urbanization categorised by the replacement of its natural landscape and water bodies with impermeable concrete and asphalt materials has resulted in gradual warming of its urban environment producing higher LST values. This study undertakes the following three objectives.
-
RO1. Conduct a spatio-temporal assessment of LST change during 1992, 2003, 2013, and 2022.
-
RO2. Evaluate LST distribution patterns and correlations with landscape indicators.
-
RO3. Develop Regression models between LST with NDBI, NDBaI, NDVI and NDWI to assess relative influences.
This study's novelty stems from its innovative analysis of three decades of LST dynamics in Nashik, systematically correlating them with regional landscape indicators. This approach allows for a deeper understanding of how these landscape features influence the temperature changes within the urban environment. This region hasn't undergone a similar investigation before, making this study's findings vital for urban planners to manage escalating urban temperatures. The rest of the paper is structured as follows. The "Literature review" Section presents a focussed Literature Review highlighting the salient features of long term LST change assessment studies conducted across different regions. Further the role of NDBI, NDVI, NDBaI and NDWI on LST estimation is also discussed. The "Materials and methods" Section describes the geographical and climatic conditions of Nashik region. Next, a stepwise methodology describing LANDSAT data retrieval, LST estimation, change detection and correlation and regression analysis between LST and NDBI, NDVI, NDBaI and NDWI is presented. The "Results" Section presents the specific results followed by pertinent discussions and mitigation measures in the "Discussions" Section to control region wide LST rise. Finally, the "Conclusions" Section presents the concluding remarks followed by the scope for further research.
Literature review
The spatiotemporal LST assessment using RS data has gained attention in recent years due to its utility in various fields like agriculture, urban planning, climate studies, and environmental monitoring (Shukla and Jain 2021; Arulbalaji et al. 2020). This approach synthesis RS-GIS technologies to capture thermal information and analyze the spatial and temporal variations of LST over different land cover types across diverse time periods. Several studies focused on the retrieval and analysis of LST from thermal infrared sensors on-board satellite platforms such as MODIS and LANDSAT (Das et al. 2020; Kumar et al. 2021; Mathew and S. P, and S. Khandelwal 2021). The spatial resolution of these sensors allows for the identification of fine-scale temperature patterns within urban areas, rural landscapes, and natural environments (Chanu et al. 2021; Gohain et al. 2020).
For example, Chakraborti et al. (Chakraborti et al. 2019) utilised the LANDSAT ETM + and OLI satellite data from 2002 and 2015 to analyse LST changes in Hyderabad by assessing neighbourhood-level landscape metrics using a Geographical Weightage Regression model. Gupta et al. (Gupta et al. 2020) studied LST changes in Jaipur from 2008 to 2011 due to urban development, using MODIS data and analyzing LST variations alongside land use changes, especially impervious surface areas. John et al. (John et al. 2020) examined LULC and LST changes in Wayanad, India between 2004 and 2018 using IRS P6 LISS-III imagery for LULC classification, and LST data derived from the ETM + thermal band. LULC changes had a discernible impact on LST, resulting in a significant 1.75 °C decrease, driven by a negative correlation with vegetation. Using MODIS LST data from 2001 to 2017, Ritesh et al. (Ritesh et al. 2020) investigated the evolution of coal fires in Jharia coalfields. Using trend decomposition models on LST pixel time series data, the study annually characterized coal fire trends and distinguished them from non-coal fire trends. Sam & Balasubramanian (Sam and Balasubramanian 2023) used LANDSAT and MODIS to track LULC and LST changes (2000–2020) along Kanyakumari coast. Settlement areas rose by 49.89%; Agriculture Land fell by 20.09%. Salt Pans peaked at 31.57 °C LST; Waterbodies remained cooler at 28.9 °C. Further, Ayanlade et al. (Ayanlade et al. 2021) investigated urban LST changes in four Nigerian cities across various ecological zones. Using LANDSAT TM/ETM data (1984–2012) and LANDSAT OLI/TIRS data (2015–2019) alongside RS-GIS techniques, they analyzed the influence of diverse land cover types on urban LST intensity. Bala et al. (Bala et al. 2021) explored Varanasi's UHI dynamics from 1989 to 2018 using LANDSAT data, unveiling an amplified UHI intensity rising from 0.36 to 0.87. The Land Cover Contribution Index highlights water and vegetation negatively impacted UHI while bare soil and built-up areas contributed positively.
LANDSAT's higher spatial resolution (15–30 m) compared to MODIS (250 m to 1 km) allows more detailed LST estimations, making LANDSAT datasets a preferred choice for researchers studying the impact of LST changes on the urban landscape (Nabizada, et al. 2022). For example, Gupta et al. (Gupta et al. 2019) investigated the cooling impact of water bodies on LSTs in urban areas, with a focus on Sukhna Lake in Chandigarh and the Sabarmati River in Ahmedabad. Substantial temperature reductions were observed: an average decrease of 7.51 °C in summer and 3.12 °C in winter near Sukhna Lake, and 1.57 °C in summer and 1.71 °C in winter within 200 to 300 m of the Sabarmati River's bank. Neog (Neog 2021) used LANDSAT TM and OLI/TIRS data (2008–2018) to examine land use effects on LST in Agartala Municipal area and found growth in built-up areas and population increased mean LST (25.71 °C to 26.29 °C in summer, 21.48 °C to 26.05 °C in winter). Correlation analysis revealed a stronger relationship between NDBI, NDVI, population density, and LST, highlighting LST amplification. In their study, Suhail and Khan (2019) (Suhail and Khan 2019) examined UHIs in NOIDA, India using LANDSAT-8 OLI-TIRS data, identifying two UHI clusters in the city's northern and mid-eastern areas linked to dense construction and industrial zones. The study highlighted a direct clear correlation between urbanization and intensifying UHIs within city centres. Aha et al. (Aha et al. 2020) investigated Kolkata city urban expansion's impact on UHI Intensity using LANDSAT data. Findings show rising LST from 27.01 °C to 33.86 °C and increased built-up areas from 6.93% to 27.10% between 1988–2018. Strong correlations (R2 = 0.84–0.99) between LANDSAT bands and LST were found. However, no clear link emerged between different built-up clusters and LST. LANDSAT datasets also enable seasonal LST change assessment. In their study, Guha et al. (Guha, Govil, Gill, et al., 2020) examined Raipur City's seasonal variations in LST and NDBI using 64 LANDSAT images from 1991 to 2019. LST consistently correlated positively with NDBI with strongest correlations occurring post-monsoon (0.72), followed by monsoon (0.69), pre-monsoon (0.67), and winter (0.57), varying across land types. Further, Guha et al. (Guha et al. 2020) reused the 64 LANDSAT images to assess the seasonal variations in LST and NDWI values across different land cover types. Again, post monsoon (0.42) NDWI values strongly correlated with LST followed by monsoon (0.34), pre-monsoon (0.25), and winter (0.04). NDBI-LST correlations outweighed NDWI-LST, highlighting the greater influence of built-up areas on LST than water content in the landscape. (Kumari et al. 2020) utilized LANDSAT 8 (OLI/TIRS) data for Mumbai, Chennai, Delhi, and Kolkata, investigating (LST) variations using the mono-window algorithm (MWA) and split-window algorithm (SWA). The study analyzed correlations between NDBI and NDVI with LST patterns, revealing urban development and vegetation cover impacts. Maithani et al. (Maithani et al. 2020) used TIRS data to assess LST changes in Dehradun during COVID-19 lockdown by comparing LSTs from April 2020 with those from April 2019, April 2018, and May 2017. Notable, high-density wards had minimal LST reduction, while open spaces and lower-density areas saw significant decreases. Likewise, Ghosh et al. (Ghosh et al. 2020) analyzed the Covid 19 lockdown effects on four Indian megacities. Utilizing remote sensing data, they constructed an Environmental Quality Index incorporating PM10, LST, NDMI, NDVI, and NDWI, highlighting the temporary enhancement in the cities' environmental quality during the stringent lockdown. Kumari et al. (Kumari et al. 2019) analysed the LST rise produced by the replacement of forests with thermal power plants in Singrauli, India. Elsewhere, Saleem et al. (Saleem et al. 2020) studied the UHI phenomenon in Lahore, Faisalabad, and Multan districts in Pakistan using LANDSAT data from 1998 and 2017. UHI effects were evident, with temperature rises of 2 °C in Lahore over two decades and similar trends observed in Faisalabad and Multan districts.
Notable, several computational techniques like single-channel algorithms, split-window algorithms, and radiative transfer models, CA, ANN have been adopted for extracting LST trends from RS datasets (Lastname, et al. 2021). For example, Lakra and Sharma (Lakra and Sharma 2019) applied the window algorithm to analyse the linkage between LULC, elevation, and LST in Ajmer, India employing LANDSAT 5 and LANDSAT 8 satellite imagery. Abdullah-Al-Faisal et al. (Tariq and Shu 2020) employed Cellular Automata-Markov Chain models to analyze urban growth and LST changes in Faisalabad from 1990 to 2048 using LANDSAT data. John et al. (John et al. 2021) applied the principal component analysis to study LULC and LST associations in Kerala, India, from 1990 to 2017 to screen key spatial and temporal patterns. Harod et al. (Harod et al. 2021) investigated the influence of various surface emissivity estimation methods on LST retrieval accuracy. Using LANDSAT data and ASTER GED, emissivity was estimated through different vegetation index (VI) models. LST retrieval employed Statistical Mono Window (SMW) and Generalized Single Channel (GSC) algorithms, validated against ground measurements in eight Indian sites. Minor differences were observed in LST accuracy among emissivity methods, with SMW outperforming GSC. Galve et al. (Galve et al. 2022) presented a comparative assessment of Single-Channel (SC) and Split-Window (SW) algorithms and a novel simplified Single Band Atmospheric Correction (L-SBAC) method based on atmospheric correction parameters and emissivity to estimate LSTs in Barrax, Spain. L-SBAC outperformed SC and SW algorithms. Noteworthy, each method has its own advantages and limitations, and the choice of method depends on the available data, sensor characteristics, and research objectives (Tariq and Shu 2020; Aithal et al. 2019; Njoku and Tenenbaum 2022).
Several authors also analyzed the relationships between LST and indices such as NDBI, NDBaI, NDVI, and NDWI provide valuable insights into the thermal behaviour of land surfaces (Halder et al. 2021; Das and Angadi 2020; Parmar et al. 2021). Guha and Govil (Guha and Govil 2021) examined the spatiotemporal relationship between LST and NDVI in Raipur City, India, from 2002 to 2018. They find a consistent negative correlation between LST and NDVI across the area, with variations in high and low LST zones. In the Narmadapuram region, Malik et al. (Malik et al. 2019) used LANDSAT-8 data to investigate the relationships between LST-NDBI and LST-NDVI across various seasons. They found strong positive correlations (R2: 0.965–0.991) between LST-NDBI and strong negative correlations (R2: 0.911–0.993) between LST-NDVI underlying the NDBI's warming effect and NDVI's cooling effect on LST. Thakur et al. (Thakur et al. 2021) studied land use changes in the Indian Sundarbans using satellite images from 2000, 2010, and 2017. Mangrove forests and plantations had lower NDVI, and settlements showed the most significant LST rise, indicating increased non-vegetated areas and ecosystem stress. Choudhury et al. (Choudhury et al. 2019) studied LST changes in West Bengal's Asansol-Durgapur Development Region from 1993 to 2015, revealing strong correlations between LST and NDVI, NDBI, and NDWI. Mukherjee and Singh (Mukherjee and Singh 2020) explored Surat and Bharuch, India, finding increased built-up areas, reduced green spaces, and higher LST. They observed a negative LST-NDVI correlation, indicating that as urbanization expanded, green spaces reduced, and LST increased. Similarly, N. Das et al. (Das et al. 2021) in Asansol, eastern India, found rising annual temperatures due to urban, industrial, and coal mining expansion. They noted negative LST-NDVI and NDWI correlations but a positive association with NDBI, suggesting future temperature increases. Jana et al. (Jana, et al. 2020) in Doon Valley observed significant urbanization, especially near the city center and roads, with significant negative LST-NDVI relationships, indicating urbanization's impact on temperature rise and ecological stability. Researchers also adopted other indicators to substantiate their findings. Like in 2022 study by Biwas and Ghosh (Biswas and Ghosh 2022) for Kolkata, LST showed strong positive linkages with NDBI and negatively correlated with NDVI and Modified Normalized Difference Water Index (MNDWI) underscoring the need for green city initiatives through sustainable land use planning. In Panaji and Tumkur, India Ramaiah et al. (Ramaiah and Manish 2020) investigated the relationship between LST and urban factors like Enhanced Built-up and Bareness Index (EBBI), Modified Normalized Difference Water Index (MNDWI), and Soil Adjusted Vegetation Index (SAVI). The study revealed strong negative correlations between MNDWI-LST in Panaji and SAVI-LST in Tumkur, highlighting water bodies and Urban Green Spaces cooling effects on LST in these two cities. Sultana & Satyanarayana (Sultana and Satyanarayana 2000) examined the spatiotemporal relationship across UHI and land use changes using indices like Enhanced Built-up and Bareness Index, Dry Built-up Index, and Dry Bare-Soil Index. Indices differentiated built-up areas (BA) from drylands (DL), showing consistently higher UHI intensities in DL compared to BA during summer and winter.
In most studies, NDBI derived from Near-Infrared (NIR) and Shortwave Infrared (SWIR) bands exhibited higher LSTs and intensified Urban Heat Island (UHI) phenomena in densely built-up areas (Maity et al. 2020; Guha and Govil 2022). In comparison NDVI, obtained from NIR and red-light bands, indicates cooler temperatures due to vegetation, while NDWI, utilizing green and NIR bands, suggests lower LSTs linked to water bodies, implying cooling effects in green or water-abundant regions (Vani and Prasad 2020; Das and Das 2019). However, these correlations differ based on location, regional land cover, climate, sensor choice, atmosphere, calibration, and spatial scale. Hence, site specific investigations are necessary for developing exact inference. This study presents a comprehensive framework to analyze the spatiotemporal LST dynamics in Nashik, India employing high-resolution LANDSAT datasets from 1992 to 2022. LST changes are analysed both at the regional scale and at various land cover levels i.e., built-up areas, bare lands, water bodies, and vegetated lands. Further, correlation-regression relationships are developed between LST and NDBI, NDBaI, NDVI, and NDWI indicators to understand the role of ground features towards LST mitigation. As no past study focussed LST assessment in this region, this study’s findings shall guide urban planning efforts to combat rising LST and UHI phenomena.
Materials and methods
Study area
Figure 1 illustrates Nashik City, situated in Maharashtra, India, a historically and culturally significant area positioned by the Godavari River. The city's geography is characterized by hills, valleys, and plateaus, with notable features such as Brahmagiri Hill and the Trimbakeshwar plateau. The climate in Nashik is classified as hot semi-arid, which is typical of the surrounding Deccan Plateau. Summers in Nashik are scorching, with temperatures soaring up to around 40 °C. Being situated in a rain shadow region, the city receives relatively lower rainfall compared to other parts of Maharashtra. However, the monsoon season, spanning from June to September, brings moderate to heavy rainfall, revitalizing the region and contributing to its agricultural productivity. During the winter months, Nashik experiences cooler temperatures, averaging between 8 and 10 °C, providing relief from the intense summer heat. The geographical features of Nashik, including its position along the Godavari River and amidst the Western Ghats, have played a significant role in the city's urban growth.
The river serves as a lifeline, supporting agricultural activities and contributing to the region's prosperity. The stunning backdrop of the Western Ghats adds to the city's charm and has influenced the development of its varied topography. Further, the city's strategic location, combined with its semi-arid climate and the seasonal rejuvenation during monsoon, contribute to the region's agricultural productivity. Covering a total area of 337 \({{\text{km}}}^{2}\) in and around Nashik, this study aims to comprehensively analyze the effects of both current and future developments. To visualize the impact of peak thermal stresses in the region, the study specifically focuses on generating LST maps during the period from the 10th to the 15th of May, when LST levels are at their highest. This strategic timeframe allows for a detailed examination of the thermal dynamics and their implications in the area under study.
Datasets
In the initial stage of the research, a collection of mid-resolution (30 × 30 m) LANDSAT datasets is obtained from the Earth Explorer repository of the United States Geological Survey (USGS). The LANDSAT program, jointly operated by NASA and USGS since 1972, provides free mid-resolution datasets, facilitating periodic assessments of spatiotemporal changes (Wikipedia, “Landsat program” 2021; Tarawally et al. 2019). Table 1 displays the specific datasets obtained for this research, which include LANDSAT 5, LANDSAT 7, and LANDSAT 8 corresponding to the years 1992, 2003, 2013, and 2022. For consistency, a uniform shape file is employed to clip the common area of interest in all images using Quantum GIS software.
Methodology
The methodology used for analyzing Land Surface Temperature Trends in Nashik from 1992 to 2022 includes five key steps, illustrated in Fig. 2.
-
STEP 1: LST MAPS GENERATION
LST Maps development involve the following steps.
-
1.
PRE-PROCESSING: This involves pre-processing the TIR band data to correct for any atmospheric effects and to convert digital numbers (DN) to radiance values using the equation:
-
2.
Conversion to Brightness Temperature: This stage involves converting the radiance values to brightness temperature using the equation:
K1 and K2 are sensor-specific constants provided in the LANDSAT metadata files. They account for sensor calibration and conversion factors.
-
3.
Atmospheric Correction: The correction compensates for atmospheric effects on TIR data, ensuring accurate surface temperature information by removing influences like water vapour and aerosol attenuation.
-
4.
Surface Emissivity Calculation: Surface emissivity estimation is crucial for precise LST retrieval, determining a surface's thermal radiation emission ability.
-
5.
LST Retrieval: The Single Channel algorithm is used for temperature-emissivity separation, employing brightness temperature and surface emissivity values to estimate surface LST.
where: C1 is the first radiation constant (1.1910427 × 10^8) in μm * K. Wavelength is the wavelength of the TIR band used.
-
6.
Post-processing and Mapping: After obtaining LST values, post-processing tasks such as spatial filtering, interpolation, or outlier removal are performed. Finally, different colour legends are assigned to represent different temperature ranges.
-
STEP 2: LC MAPS GENERATION
Nashik region LC maps for 1992, 2003, 2013 and 2022 were developed using the Maximum Likelihood Algorithm (MLC) within the QGIS-SCP Plugin. MLC relies on user supplied LC spectral signatures to classify unseen pixels. MLC execution involves three main steps namely data preparation, maximum likelihood estimation, and classification. In data preparation, training samples are collected for each class based on spectral signatures, and in the parameter estimation step, the MLC algorithm determines the probability distribution parameters for each class. By assuming a multivariate Gaussian distribution, the mean vector (μ) and covariance matrix are estimated for each class using the following equations.
where \({n}_{k}\) is the number of training samples for class k and \({X}_{i}\) represent the Spectral feature vector of the i-th training sample. Further, T represents the transpose operation.
In the final classification phase, ML evaluates the probability of each pixel being associated with each class using the estimated parameters. The Likelihood (L_k) for a pixel with spectral feature vector X belonging to class k is calculated using the following multivariate Gaussian distribution equation.
where d signifies the dimensionality of the spectral feature vector, |Σ_k| implies the determinant of the covariance matrix \({\Sigma }_{{\text{k}}}\), \({(X}_{i }-{\upmu }_{k}\)) denotes the difference between the spectral feature vector X and the mean vector \({\upmu }_{k}\) and finally \({{\Sigma }_{{\text{k}}}}^{-1}\) represents the inverse of the covariance matrix \({\Sigma }_{{\text{k}}}\). Finally, each pixel is allocated to the class with the highest Likelihood. MLC algorithm assumes class independence and normal distribution while not considering the spatial context. The chosen study area is categorized into four LC classes: Built-up, Vegetation, Water body, and Bareland. Built-up areas encompass roads, residential and commercial, buildings whereas rivers, lakes, and wetlands encompass waterbodies. Further, Vegetation covers trees, gardens, forests, and cropped farmlands whereas Barelands include open areas, uncropped agricultural lands and other and remining land-cover types. LC map accuracy is assessed using Kappa statistics, quantifying agreement between classified and actual ground features. One hundred actual ground control points (25 per class) were selected across the entire study area to compare the actual and predicted LC class labels. A minimum kappa accuracy threshold of 0.80 was required to accept the MLC classification results.
-
STEP 2: LST CHANGE DETECTION
The second stage i.e., LST Change Detection involves a comparative assessment of LST maps during 1992, 2002, 2013, and 2022. Further, Table 2 provides statistical properties, summarizing the minimum, average, and maximum temperatures for these periods. Area coverage under five LST intervals (ranging from 25 °C to 50 °C) is displayed in Fig. 3 and Table 3. LST maps for the four periods are presented in Fig. 4. The change in area coverage (in square kilometres) under each temperature band indicates the LST changes during the specified periods.
-
STEP 3: NDBI, NDBaI, NDWI and NDVI Maps Generation
This step includes computing landscape indices for the entire region using suitable frequency bands from the multi-spectral LANDSAT dataset. NDBI identifies urban areas utilizing differences in Near-Infrared (NIR) and Shortwave Infrared (SWIR) reflectance. NDBaI evaluates bare land by utilizing SWIR and red bands. NDVI gauges vegetation density by measuring differences between NIR and red bands. NDWI recognizes water bodies by assessing differences in green and NIR bands.
-
STEP 4: CORRELATION ANALYSIS
In this stage, a correlation matrix is developed to assess the relationship between LST and NDBI, NDBaI, NDVI, and NDWI. This involves calculating the values of these indices, extracting LST values, and computing correlation coefficients to understand their influence on temperature variations in the study area.
-
Step 1: Data Preparation: Nashik region is divided into a 50 mx 50 m grid covering 13444 ground points to extract LST and landscape indicators data. All five parameters are arranged in a tabular manner to develop 13444 rows by 5 columns matrix.
-
Step 2: Compute the mean and Standard Deviation for each variable.
Mean of variable X measures the central tendency of the dataset.
Standard deviation of variable X measures the variability or dispersion of the data points around the mean.
-
Step 3: Calculate the covariance between each pair of variables. Covariance measures the joint variability between two variables.
-
Step 4: Calculate the correlation coefficient (Pearson correlation) between each pair of variables. The correlation coefficient ranges from -1 to + 1 and indicates the strength and direction of the linear relationship between two variables.
Correlation coefficient between variables X and Y:
-
Step 5: Assemble Correlation Matrix Create a matrix (n x n) to store the correlation coefficients for all pairs of variables.
The correlation matrix is analysed by studying the pairwise correlation values. Positive values (closer to + 1) indicate a positive linear relationship, negative values (closer to -1) indicate a negative linear relationship, and values close to 0 suggest a weak or no linear relationship between the variables.
-
STEP 5: REGRESSION ANALYSIS
Several Linear Regression models are affixed between LST and four independents (NDBI, NDBaI, NDVI and NDWI) during the four periods to assess the relative influence of four inputs on the model output. Each of these models follows the concise form as shown by Eq. (16):
In this context, \({\upbeta }_{o}\) denotes the constant or intercept in the model, while \({\upbeta }_{1}\) serves as the regression coefficient, providing insight into the influence of the corresponding independent variable. Additionally, \(\upvarepsilon\) captures the error term, encompassing variations in LST that remain unexplained by the independent variable.
The primary objective of these linear regression models is to precisely estimate the coefficients (\({\upbeta }_{o}\) and \({\upbeta }_{1}\)) that best fit the data for their respective years minimizing squared errors between predicted and actual LST values. Goodness of Model Fit (\({{\text{R}}}^{2}\)) quantifies the proportion of LST variance explained by independent variables, with higher values indicating better fit. Higher R-squared values (closer to 1) indicate a better fit. Notably, in this context, \({y}_{i}\), \(\overline{{y }_{i}}\), and \(\widehat{{y}_{i}}\) represent actual, average, and predicted LST values as defined in Eq. 17.
Results
LST change assessment
Table 2 illustrates the minimum, average, and maximum Land Surface Temperatures (LSTs) for the years 1992, 2002, 2013, and 2022: (27.52, 37.24, 43.24)°C; (25.87, 38.72, 47.00)°C; (29.96, 39.54, 46.78)°C; and (27.63, 40.32, 50.23)°C, respectively. During 1992–2022, minimum LST ranged from lowest 25.87 °C in 2003 to highest 29.96 °C in 2013. Further, a clear warming trend is detected as the region's mean and max LST values rose consistently from 1992 to 2022, growing by 8.06% and 15.36% respectively each decade. Further, the difference between the max and mean LST rose steadily by 20.02% each decade.
Figure 3 illustrates the LST distributions for 1992, 2003, 2013, and 2022, represented by blue, yellow, green and red colours, respectively. The density plots indicate that the coolest LSTs were detected in 1992, while the hottest temperatures were recorded in 2022. Further, LSTs during 2013 were generally higher than 2003 signalling a warming trend. Noticeably, 1992 and 2003 contain a higher data density in the (35–40)°C band than later years showing major representation in the (40–50)°C interval. Markedly, a gradual rightward shift is seen with the passage of time as recent LST histogram plots show greater density towards elevated temperature values than earlier years implying that LST increase is not a localised phenomenon and experienced in most areas of Nashik as the vast majority of data points display higher LST values than previous decades. Further, a prominent data density higher than 45 °C is seen for the first time during 2022.
LC maps were developed to classify LST changes across different LC classes across the four periods. MLC algorithm was adopted to develop these four maps which are shown in Fig. 4. Further their classification accuracies are represented in Table 3. All four LC maps exhibited high kappa values exceeding 0.90, especially with Built-Up class achieving high kappa exceeding 0.95. In comparison, Kappa for Vegetation and Bareland ranged between (0.81–0.95) and (0.87–0.95) across the four years.
Built-up coverage has notably increased in the study area over the past three decades. Table 2 outlines the minimum, mean, and maximum Land Surface Temperatures (LSTs) for the four main land cover classes in the Nashik region. The mean LSTs for the years 1992, 2003, 2013, and 2022 exhibit consistent warming trends across different land types. Bare lands consistently display the highest mean LST, ranging from 38.43 °C in 1992 to 41.15 °C in 2022. Built-up areas also show a steady increase in mean LST, rising from 36.77 °C in 1992 to 40.52 °C in 2022. In contrast, water bodies maintain cooler LSTs, varying from 31.29 °C in 2003 to 35.13 °C in 2022. Vegetated areas, while cooler, experience a gradual LST rise from 36.08 °C in 1992 to 38.84 °C in 2022.
The minimum and maximum LST values shown in Fig. 5 display LST rise across different land cover types. Notably, water bodies constantly exhibit the lowest minimum LST, fluctuating from 25.87 °C to 30.16 °C. In comparison, built-up and barelands show higher minimum LST values. The minimum LST values recorded for built-up and Barelands ranged from (30.8–32.3)°C and (28.8–33.6)°C, respectively. Similar to water bodies, vegetated areas displayed lower minimum LST values, ranging from 27.67 °C to 30.58 °C. Analyzing maximum LST values in Fig. 5, it is evident that unvegetated Barelands experienced the highest LST across all four periods rising from 43.34 °C in 1992 to 49.54 °C in 2022. Built-Up areas comprising commercial buildings and human settlements also experienced high LST values and a consistent LST warming trend as LST rose from 41.07 °C in 1992 to 48.36 °C in 2022. Similar to min and mean LSTs tends, water bodies comprising rivers, ponds displayed the lowest values for maximum LST ranging from 38.48 °C in 1992 to 41.19 °C in 2023. Vegetated maintains relatively stable maximum LST, varying from 41.83 °C to 44.3 °C were much found cooler than bare lands and built-up spaces.
The LST maps for the four periods are shown in Fig. 6, followed by area coverages in different LST intervals in Fig. 7 and Table 4. In the 1992 LST map (Fig. 7a), the largest area of 248.30 km2 falls within the temperature range of (30–35)°C. The coolest LSTs ranging (25–30)°C are observed around the city center, particularly near Panchvati and Tapovan along the Godavari River, with several surrounding areas like Thate Nagar, ISCON, Sahdev Nagar, and Police Colony experiencing cool temperatures ranging (30–35)°C. Similar cooling effect is seen in areas located near to Darna River along the south-eastern end. Adjoining these cooler zones, an area of 57.09 km2 is observed in the temperature range of (35–40)°C, encompassing places such as Mahatmanagar, Hirawadi, Maneksha, Samtanagar, and Tagorenagar. Smaller patches covering around 30.47 km2, characterized by elevated LSTs ranging from (40–45)°C, are visible along the Adagaon, Masrul, Vrindavan in the East and Wadalagaon and Pathardi along the Southwest.
Upon examining the 2003 LST map (Fig. 7b), it is evident that small patches of high LSTs observed in 1992, ranging from (40–45)°C, have extended inward toward the city center reaching areas up to Ayodhya Nagar in the East, Hanuman Nagar in the North, Kalpatru in the South, and Dhurav Nagar in the West. This expansion represents a significant increase of 333%, equalling 132.16 km2. Further, the previously concentrated areas characterized by lower LSTs within (30–35) °C range, highlighted in green in the 1992 map, have fragmented into smaller patches. As shown in Fig. 8, this fragmentation led to a reduction in coverage by 35%, with the area decreasing from the initial 57.09 km2 to 35.66 km2. As observed in the 1992 map, cooler LST regions persist near the Godavari River, specifically along Panchvati, Tapovan, and Sawarkar Nagar. However, the most significant feature in the 2003 map is the inward expansion and consolidation of the high LST regions in the (40–45)°C range. This resulted in an 81 km2 reduction in the coverage of LSTs ranging from (35–40)°C and the fragmentation of lower LST regions in the (30–35)°C range. Additionally, in the 2003 LST map, new small patches of extreme high LSTs ranging from (40–45)°C appeared towards the southwest region near Pandav caves and Sidharth Nagar in the east.
Upon analysing the 2013 LST map (Fig. 7c), one of the most notable features is the significant decrease in cooler green patches, which has occurred due to the consolidation and expansion of hot yellow and orange patches. This shift in temperature distribution has led to a significant reduction of up to 75% in the coverage of LST falling within (30–35)°C range, resulting in the coverage area shrinking from 35.66 km2 to 8.65 km2. Consequently, this change resulted in 12% increase in the coverage of LST falling within the (35–40)°C range and a 7% increase in the area covered by LST within (40–45)°C range. The hottest LST ranging (40–45)°C is observed across Pandav Nagar to Samita Nagar in the south, Dhruvak and Jaswant Nagar in the west, Warvandi to Ayodhya Nagar in the north, and Konark and Bapu Nagar in the east. In comparison, the coolest LST ranging (30–35) °C are concentrated in regions near water bodies, particularly near Panchvati, Jalapur, and Chendi, located close to the Darna river along the south-eastern end. Further, the coverage of extreme LST values, ranging from (45–50) °C, increased marginally than 2003 levels, near Narhar Nagar and Pandav caves.
The most recent 2022 LST map (Fig. 7d) for the Nashik region revealed a worrying trend as almost all areas experienced higher LST than previous decades. The coverage of the lowest LST band, ranging (30–35)°C reduced along the Godavari and Darna rivers along city center and south-eastern ends. However cooler regions are spotted along the northern extent of the Godavari River and its tributary near Mungsare and Jalalpur regions. There has been a reduction of up to 32% in the coverage of LST falling within the (35–40)°C range, while the area covered by LST values ranging from (40–45)°C has experienced a 23% increase. From a visual perspective, the LST band between 40 °C and 45 °C demonstrates a noticeable radial consolidation, expanding both in coverage and intensity. These warm regions extend from Hirawadi to Warwandi along north, Indira Nagar to Pathardi Gaon along south, Hirawadi to Sultanpur in east and Shramik Nagar to Mahatma Nagar along western part of the city. The most striking feature is the emergence of an area spanning 16.25 km2 under the extremely high (45–50)°C temperature band, which was previously unseen during past decades. Until 2013, the areas with extreme temperatures were primarily concentrated along the city's south-west direction, near Pandav Nagar. However, by 2022, such extreme temperatures have been detected in all directions. These high LST regions are now observed near Mhasural Gaon in the north, Konark Nagar and Sidhartnagar in the east, the airport area along the city's south, and Padav Nagri along the south-west. The expansion of these extreme LST areas poses a serious risk of severe thermal discomfort and can even prove fatal under prolonged exposure.
A consistent warming trend is seen across the four LST maps. While climate change contributes to Nashik region's LST increase, significant changes in land-use also play a crucial role. This relationship is confirmed by linking LST with landscape indicators like NDBI, NDBaI, NDVI, and NDWI. Figure 9 displays NDBI values for the Nashik Region during 1992, 2003, 2013, and 2022. NDBI quantifies urbanization, with negative NDBI values signify natural or non-built-up areas. The data reveals a significant range of variation in NDBI values across these years, underscoring changing urban dynamics. Across the three decades, NDBI ranged from a minimum of -0.45 in 1992 to a maximum of 0.64 during 2022.
Figure 10 illustrates NDBaI maps delineating the non-vegetated areas within the Nashik Region for the years 1992, 2003, 2013, and 2022. NDBaI serves as a metric quantifying the presence of bare or non-vegetated terrain across the region. Notably, considerable variation exists in NDBaI values throughout these years, indicating dynamic shifts in bare land coverage. During the three decades the NDBaI ranged from a minimum value of -0.75 in 1992 to a maximum of 0.27 in 2003. Similarly, Fig. 11 illustrates NDVI maps portraying vegetation density changes in the Nashik Region across 1992, 2003, 2013, and 2022. Notably, the data showcases considerable variations in NDVI values, indicating shifts in vegetation cover. Across the three decades the NDVI values ranged from a minimum of -0.46 in 2022 to a maximum of 0.61 during 1991.
Figure 12 exhibits NDWI maps representing the Nashik Region across 1992, 2003, 2013, and 2022. NDWI serves as a crucial metric for assessing water content or the presence of water bodies within an area. The data presented in this figure reveals noteworthy variations in NDWI values, offering insights into changes in water bodies within the Nashik Region over time. Across the study period spanning three decades NDWI ranged between a minimum of -0.48 in 2013 to a maximum of 0.61 in 1992.
Correlation analysis
The correlation matrices in Fig. 13 illustrate the association between LST and different landscape indicators. LST consistently shows positive correlations with NDBI and NDBaI, while displaying negative correlations with NDVI and NDWI across all four periods. The correlations between LST and NDBI ranges from 0.65 to 0.78, and with NDBaI, the range was 0.073 to 0.29. In comparison, LST correlations with NDWI ranged from -0.76 to -0.45, and with NDVI, the range was -0.57 to -0.78. These findings clearly imply that LST were higher in bare lands and built-up spaces than vegetated areas and waterbodies. Further, the plots reveal strong positive associations between LST and NDBI, suggesting that built-up areas with concrete and paved surfaces experience the highest LST. Bare lands also exhibit high LST, though slightly lower than built-up spaces. Notably, LST's positive correlations with NDBI are considerably higher than with NDBaI, indicating that buildings, paved and concrete surfaces experience much higher LST than bare lands.
Conversely, LST shows negative correlations with NDVI and NDWI, indicating lower LST values in dense vegetated areas like parks and forests and water bodies like rivers, lakes, and ponds. Across all four periods, LST displayed stronger negative correlations with NDWI compared to NDVI, highlighting that water bodies have the most significant cooling effect. Further strong positive correlations are visible between NDVI and NDWI across the four periods. However, negative correlations are seen across NDBaI and NDBI with NDVI and NDWI. This association between vegetation and water content suggests a complementary relationship where regions with abundant water resources tend to foster richer vegetation cover, leading to moderated temperatures. On the other hand, barren lands and paved surfaces with diminished capacity to retain moisture or promote green coverage reflect heat and radiation leading to higher LST in those areas.
Regression analysis
Regression analysis is conducted between LST and NDBI, NDBaI, NDWI, and NDVI to represent their associations in Fig. 14 and establish a statistical relationship among various parameters. Figure 14 visually summarizes these findings for the four time periods. In 1992, NDBI showed a reasonable positive correlation with LST (R2 = 0.43, Correlation = 0.65), indicating a link to higher temperatures in built-up areas. NDBaI had a weaker relationship (R2 = 0.03, Correlation = 0.18), suggesting a lesser impact. NDVI exhibited a strong negative correlation (R2 = 0.46, Correlation = -0.68), highlighting vegetation's cooling effect. NDWI displayed a moderately negative correlation (R2 = 0.43, Correlation = -0.65), emphasizing the cooling impact of water bodies. In 2003, NDBI showed a strong positive correlation with LST (R2 = 0.62, Correlation = 0.78), indicating urban development's warming effect. NDBaI had a weaker relationship (R2 = 0.08, Correlation = 0.29). NDVI exhibited a significant negative correlation (R2 = 0.58, Correlation = -0.76), highlighting vegetation's cooling influence. NDWI also showed a strong negative correlation (R2 = 0.62, Correlation = -0.78), emphasizing water bodies' cooling impact.
In 2013, NDBI showed highest positive correlation with LST (R2 = 0.40, Correlation = 0.64), linking built-up areas to higher temperatures. NDBaI had a weaker relationship (R2 = 0.07, Correlation = 0.26). NDVI exhibited a notable negative correlation (R2 = 0.29, Correlation = -0.54), highlighting vegetation's cooling impact. Similarly, NDWI displayed a moderate negative correlation (R2 = 0.40, Correlation = -0.64), emphasizing water bodies' cooling effect. Finally, in 2022, NDBI showed a moderate positive correlation with LST (R2 = 0.33, Correlation = 0.57), linking built-up areas to higher temperatures. NDBaI had a weak relationship (R2 = 0.01, Correlation = 0.07). NDVI exhibited a notable negative correlation (R2 = 0.20, Correlation = -0.45), highlighting vegetation's cooling impact. NDWI displayed a moderate negative correlation (R2 = 0.33, Correlation = -0.57), emphasizing water bodies' cooling effect. Across the four time periods, a consistent pattern emerges where NDBI consistently strongly correlated with LST, indicating a proportional direct between built-up areas and higher temperatures. Conversely, NDVI and NDWI consistently indicates a reasonable negative correlation with LST, emphasizing vegetation and water bodies cooling effect.
Discussions
The rapid urban growth in Nashik region led to a substantial LST rise during the past three decades. The step wise analysis presented in this study revealed the expansion and consolidation of higher LST areas, particularly in the (40–45)°C range, leading to the replacement of lower LST regions with hotter ones. In 1992, just 9.1% of the region experienced LSTs exceeding 40 °C which rose to 39.27%, 42.04%, and 51.96% in 2003, 2013, and 2022, respectively, indicating a concerning 35% increase each decade. Also, the presence of exceedingly high 45–50 °C LSTs in 2022, spanning 16.25 km2 and peaking at 50.23 °C, is concerning. Over the four periods, built-up areas and barren lands consistently displayed significantly higher LSTs compared to vegetated areas and water bodies. The deficiency of moisture and vegetation led to reduced evaporative cooling, causing built-up and bare surfaces to absorb and retain more heat, consequently resulting in elevated temperatures. Statistical analysis also showed NDBI and NDBaI consistently correlates positively with LST indicating urban warming. NDVI and NDWI consistently exhibits a strong negative correlation highlighting vegetation's cooling effect.
The surge in LSTs triggers an intensified UHI effect, impacting various dimensions directly linked to urban sustainability and human comfort. These rising temperatures exacerbate water evaporation, contributing to soil aridification, which further compounds existing water scarcity issues. The effect extends to agricultural productivity, with reduced water availability impacting crop yields and potentially affecting domestic water resources crucial for sustenance. Simultaneously, the amplified LSTs expedite the degradation of urban infrastructure, leading to thermal expansion-related damages in roads, buildings, and utilities. These damages, aside from elevating maintenance expenses, also pose a direct threat to structural integrity, compromising the safety and functionality of critical urban facilities. In addition to infrastructural challenges, the rising temperatures disrupt urban ecosystems. This disturbance affects biodiversity as habitats and migration patterns of various plant and animal species alter, causing imbalances in the delicate ecological equilibrium within the city. The agricultural sector also faces adverse effects as increasing LSTs cause heat stress in crops, negatively impacting agricultural production and reducing crop yields. Consequently, food security may get compromised, leading to economic and social challenges for the region.
As temperatures rise, the comfort and quality of life for residents’ decline, rendering outdoor activities less feasible and diminishing the city's liveability. People working outdoors, such as labourers and construction workers, are hit hardest by the thermal distress caused by the rising LSTs, potentially leading to serious health issues and safety concerns in the workforce. Moreover, potential declines in productivity across various sectors due to extreme heat can impact the overall economic landscape of the city. Higher LST also restrict outdoor recreation opportunities due to the health risks and discomfort posed by extreme heat. The surge LST also heightens heat-related fatalities, notably impacting vulnerable populations like the very young and elderly. Recent 2022 reports highlighted the highest heatstroke deaths in six years in Maharashtra, with Nashik documenting 4 fatalities among 381 cases, signalling a notable local concern (Livemint, “Maharashtra 2022). Higher LSTs also increase the city-wide energy demands for building cooling. The heat released from these mechanical systems further increase urban LSTs creating a negating feedback loop. Vulnerable communities might face disproportionate impacts, amplifying disparities in resource access and resilience to extreme heat, potentially widening the gap in quality of life between different segments of the urban populace.
Addressing the escalating LST in Nashik demands a multifaceted urban planning approach. Municipal and Urban Planning authorities should prioritize the creation and preservation of green spaces, such as parks and urban forests especially areas away from Godavari and Drona rivers experiencing higher LSTs. Strategic placement of trees along streets and in parking lots can increase evapotranspiration, and reduce the absorption of solar radiation, effectively lowering surface temperatures. Further, preserving and creating water bodies like ponds and lakes is essential for Nashik's temperature regulation and heat reduction. Water bodies serve as heat sinks, provide evaporative cooling, and play a crucial role in maintaining groundwater levels, making them indispensable for sustainable urban planning. Moreover, incorporating cool and reflective pavements, cool roofs, shading structures, and urban green infrastructure, such as green walls and vegetated facades, should be integrated into urban development plans to effectively mitigate heat absorption and enhance thermal comfort in Nashik. Finally, reinforcing urban planning zoning regulations to prioritize open spaces, greenery preservation, and restricting heat-absorbing materials in new developments will be pivotal in curbing rising temperatures and nurturing a more comfortable urban living environment in Nashik.
Conclusions
This research presented a comprehensive approach to analyze the spatio-temporal Land Surface Temperatures (LST) dynamics in Nashik, India during the past three decades. The single-channel algorithm was applied on LANDSAT datasets for the years 1992, 2003, 2013, and 2022 to generate regional LST maps. Further, Land Cover Maps were developed for the four periods to assess LST changes across different Land classes. Nashik’s water bodies, built up areas, vegetated and bare lands all experienced rising LSTs with 2022 marking the highest values. Areas with LSTs exceeding 40 °C surged from 9.1% to 51.96% between 1992 and 2022, signifying a worrying 35% increase rate. Simultaneously, the warmer (30–40)°C areas decreased from 90.75% in 1992 to 43.03% in 2022, indicating a concerning loss of cooler green spaces. Moreover, in 2022, a 16.25 km2 region experienced extreme LSTs ranging from (45–50)°C, an unprecedented event in previous decades. Further, correlation and regression revealed higher LSTs in built-up and barren areas (positively linked with NDBI and NDBaI) but lower temperatures in vegetated zones and water bodies (negatively associated with NDVI and NDWI). Stronger LST correlation with NDBI suggests heightened temperatures in areas with concrete surfaces. Conversely, negative LST correlations with NDVI/NDWI indicate cooler zones with vegetation and water bodies, emphasizing the latter's cooling impact. Positive NDVI and NDWI correlations imply that regions abundant in water resources support richer vegetation, moderating temperatures, while barren lands and paved surfaces elevate temperatures by reflecting heat.
Elevated LSTs present multifaceted challenges to urban sustainability and human well-being. These rising temperatures intensify water evaporation, exacerbating water scarcity and agricultural concerns. Concurrently, heightened LSTs contribute to urban infrastructure damage and disrupt ecosystems, impacting biodiversity. Heat stress diminishes crop yields, posing risks to food security. Additionally, soaring temperatures restrict outdoor activities, impact city liveability, and amplify heat-related fatalities, particularly among vulnerable populations, widening disparities in urban resources and affecting overall quality of life. Slowing the alarming trend of escalating LST in Nashik demands multifaceted urban planning led by Municipal and Urban Planning authorities. Prioritizing green spaces, strategic tree plantation, water body conservation, and implementing cooling technologies such as reflective pavements, cool roofs, and shading structures are imperative measures.
Reinforcing zoning regulations for open spaces and restricting heat-absorbing materials in new developments are pivotal steps to nurture a more comfortable urban living environment in Nashik while curbing rising temperatures. Finally, promoting public awareness and engagement shall be crucial for nurturing collective efforts towards climate resilience and mitigation. The current study adopted LANDSAT datasets to monitor long term LST changes spanning three decades. Follow up studies can adopt additional satellite datasets like Sentinel-2, MODIS, ASTER and GOES for LST mapping for this region. Further, future studies can study seasonal and diurnal LST patterns in the region. The utilization of higher-resolution datasets has the potential to enhance the precision of the findings. Moreover, researchers can also apply advanced Machine Learning algorithms to project future LST dynamics and their impact on health, energy and infrastructure.
Data availability
The data that support the findings of this study are available on request from the corresponding author.
References
Abdullah-Al-Faisal et al (2021) Assessment and prediction of seasonal land surface temperature change using multi-temporal landsat images and their impacts on agricultural yields in Rajshahi Bangladesh. Environ. Challenges 4(January):100147. https://doi.org/10.1016/j.envc.2021.100147
Aha PRS, Andopadhyay SUB, Umar CHK (2020) Multi-approach synergic investigation between land surface temperature and land-use land-cover. J Earth Syst Sci 129:74. https://doi.org/10.1007/s12040-020-1342-z
Aithal BH, Chandan MC, Nimish G (2019) Assessing land surface temperature and land use change through spatio-temporal analysis: a case study of select major cities of India. Arab J Geosci 12(11):367. https://doi.org/10.1007/s12517-019-4547-1
Arulbalaji P, Padmalal D, Maya K (2020) Impact of urbanization and land surface temperature changes in a coastal town in Kerala, India. Environ Earth Sci 79:1–18. https://doi.org/10.1007/s12665-020-09120-1
Ayanlade A, Aigbiremolen MI, Oladosu OR (2021) Variations in urban land surface temperature intensity over four cities in different ecological zones. Sci Rep 11(1):1–17. https://doi.org/10.1038/s41598-021-99693-z
Bala R, Prasad R, Yadav VP (2021) Quantification of urban heat intensity with land use / land cover changes using landsat satellite data over urban landscapes. Theor Appl Climatol 145:1–12
Biswas S, Ghosh S (2022) Estimation of land surface temperature in response to land use / land cover transformation in Kolkata city and its suburban area, India. Int J Urban Sci ISSN. https://doi.org/10.1080/12265934.2021.1997633
Chakraborti S, Banerjee A, Sannigrahi S, Pramanik S, Maiti A, Jha S (2019) Assessing the dynamic relationship among land use pattern and land surface temperature: a spatial regression approach. Asian Geogr 36(2):93–116. https://doi.org/10.1080/10225706.2019.1623054
Chanu CS, Elango L, Shankar GR (2021) A geospatial approach for assessing the relation between changing land use/land cover and environmental parameters including land surface temperature of Chennai metropolitan city India. Arab J Geosci 14:2. https://doi.org/10.1007/s12517-020-06409-0
Chaturvedi S, Shukla K, Rajasekar E, Bhatt N (2022) A spatio-temporal assessment and prediction of Ahmedabad’s urban growth between 1990–2030. J Geogr Sci 32(9):1791–1812. https://doi.org/10.1007/s11442-022-2023-4
Chetia S, Saikia A, Basumatary M, Sahariah D (2020) When the heat is on : urbanization and land surface temperature in Guwahati, India. Acta Geophys. 68:0123456789. https://doi.org/10.1007/s11600-020-00422-3
Choudhury D, Das K, Das A (2019) Assessment of land use land cover changes and its impact on variations of land surface temperature in Asansol-Durgapur development region. Egypt J Remote Sens Sp Sci 22(2):203–218. https://doi.org/10.1016/j.ejrs.2018.05.004
Das S, Angadi DP (2020) Land use-land cover (LULC) transformation and its relation with land surface temperature changes: a case study of Barrackpore subdivision, West Bengal, India”. Remote Sens Appl Soc Environ 19(March):100322. https://doi.org/10.1016/j.rsase.2020.100322
Das M, Das A (2020) Assessing the relationship between local climatic zones (LCZs) and land surface temperature (LST) – a case study of sriniketan-santiniketan planning area (SSPA), West Bengal, India”. Urban Clim. 32(April):100591. https://doi.org/10.1016/j.uclim.2020.100591
Das DN, Chakraborti S, Saha G, Banerjee A, Singh D (2020) Analysing the dynamic relationship of land surface temperature and landuse pattern: a city level analysis of two climatic regions in India. City Environ Interact 8:100046. https://doi.org/10.1016/j.cacint.2020.100046
Das N, Mondal P, Sutradhar S, Ghosh R (2021) Assessment of variation of land use/land cover and its impact on land surface temperature of Asansol subdivision. Egypt J Remote Sens Sp Sci 24(1):131–149. https://doi.org/10.1016/j.ejrs.2020.05.001
Galve JM, Sánchez JM, García-Santos V, González-Piqueras J, Calera A, Villodre J (2022) Assessment of land surface temperature estimates from landsat 8-TIRS in a high-contrast semiarid agroecosystem. algorithms intercomparison. Remote Sens. 14:8. https://doi.org/10.3390/rs14081843
Ghosh S, Das A, Hembram TK, Saha S, Pradhan B, Alamri AM (2020) Impact of COVID-19 induced lockdown on environmental quality in four indian megacities using landsat 8 OLI and TIRS-derived data and mamdani fuzzy logic modelling approach. Sustainability 12(13):5464
Gohain KJ, Mohammad P, Goswami A (2020) Assessing the impact of land use land cover changes on land surface temperature over Pune city, India. Quat Int. https://doi.org/10.1016/j.quaint.2020.04.052
Guha S, Govil H (2021) An assessment on the relationship between land surface temperature and normalized difference vegetation index. Environ Dev Sustain 23(2):1944–1963. https://doi.org/10.1007/s10668-020-00657-6
Guha S, Govil H (2022) Annual assessment on the relationship between land surface temperature and six remote sensing indices using landsat data from 1988 to 2019. Geocarto Int 37(15):4292–4311. https://doi.org/10.1080/10106049.2021.1886339
Guha S, Govil H, Besoya M (2020) An investigation on seasonal variability between LST and NDWI in an urban environment using landsat satellite data, Geomatics. Nat Hazards Risk 11(1):1319–1345. https://doi.org/10.1080/19475705.2020.1789762
Guha S (2021) Dynamic seasonal analysis on LST-NDVI relationship and ecological health of Raipur City, India. Ecosyst Heal Sustain 7(1):1927852. https://doi.org/10.1080/20964129.2021.1927852
Gupta N, Mathew A, Khandelwal S (2019) Analysis of cooling effect of water bodies on land surface temperature in nearby region: a case study of Ahmedabad and Chandigarh cities in India. Egypt J Remote Sens Sp Sci 22(1):81–93. https://doi.org/10.1016/j.ejrs.2018.03.007
Gupta N, Mathew A, Khandelwal S (2020) Spatio-temporal impact assessment of land use / land cover (LU-LC) change on land surface temperatures over Jaipur city in India. Int J Urban Sustain Dev 12(3):283–299. https://doi.org/10.1080/19463138.2020.1727908
Gupta M et al (2021) Transmission dynamics of the COVID-19 epidemic in India and modeling optimal lockdown exit strategies. Int J Infect Dis 103:579–589. https://doi.org/10.1016/j.ijid.2020.11.206
Halder B, Bandyopadhyay J, Banik P (2021) Evaluation of the climate change impact on urban Heat Island based on land surface temperature and geospatial indicators. Int J Environ Res 15(5):819–835. https://doi.org/10.1007/s41742-021-00356-8
Harod R, Eswar R, Bhattacharya BK (2021) Effect of surface emissivity and retrieval algorithms on the accuracy of land surface temperature retrieved from landsat data effect of surface emissivity and retrieval algorithms on the accuracy of land surface temperature retrieved from landsat data. Remote Sens Lett 12(10):983–993. https://doi.org/10.1080/2150704X.2021.1957511
Jana C, Mandal D, Shrimali SS, Alam NM, Kumar R, Sena DR, Kaushal R (2020) Assessment of urban growth effects on green space and surface temperature in Doon Valley, Uttarakhand, India. Environ Monit Assess 192:1–17. https://doi.org/10.1007/s10661-020-8184-7
John J, Bindu G, Srimuruganandam B, Wadhwa A, Rajan P (2020) Land use/land cover and land surface temperature analysis in wayanad district, India, using satellite imagery. Ann GIS 26(4):343–360. https://doi.org/10.1080/19475683.2020.1733662
John J, Chithra NR, Thampi SG (2021) Assessment of land surface temperature dynamics over the Bharathapuzha River basin, India. Acta Geophys 69(3):855–876. https://doi.org/10.1007/s11600-021-00593-7
Kumar A, Agarwal V, Pal L, Chandniha SK, Mishra V (2021) “Effect of land surface temperature on urban Heat Island in Varanasi City, India”, J — Multidiscip. Sci J 4(3):420–429. https://doi.org/10.3390/j4030032
Kumari M, Sarma K, Sharma R (2019) Using Moran’s I and GIS to study the spatial pattern of land surface temperature in relation to land use/cover around a thermal power plant in Singrauli district, Madhya Pradesh, India. Remote Sens Appl Soc Environ 15(February):100239. https://doi.org/10.1016/j.rsase.2019.100239
Kumari B, Tayyab M, Ahmed IA, Razi M, Baig I (2020) Longitudinal study of land surface temperature ( LST ) using mono- and split-window algorithms and its relationship with NDVI and NDBI over selected metro cities of India. Arab J Geosci 13:1–19
Lakra K, Sharma D (2019) Geospatial assessment of urban growth dynamics and land surface temperature in Ajmer region, India. J Indian Soc Remote Sens 47(6):1073–1089. https://doi.org/10.1007/s12524-019-00968-w
Livemint, “Maharashtra: At least 25 died due to heatstroke; highest in 6 years | Mint,” 2022. https://www.livemint.com/news/india/maharashtra-at-least-25-died-due-to-heatstroke-highest-in-6-years-11651492722863.html (accessed Jan. 23, 2024)
Maithani S, Nautiyal G, Sharma A (2020) Investigating the effect of lockdown during COVID-19 on land surface temperature: study of Dehradun City, India. J Indian Soc Remote Sens 48(9):1297–1311. https://doi.org/10.1007/s12524-020-01157-w
Maity DS, Srivastava DGL, Mane SP (2020) Comprehensive analysis of land surface temperature with different indices using Landsat–8 (OLI/TIRS) data in Kanpur Metropolis, India. Akshar Wangmay Special Issue, p. 1
Malik MS, Shukla JP, Mishra S (2019) Relationship of LST, NDBI and NDVI using Landsat-8 data in kandaihimmat. Indian J Geo Mar Sci 48(January):25–31
Mathew A, Sarah P, Khandelwal S (2022) Investigating the contrast diurnal relationship of land surface temperatures with various surface parameters represent vegetation, soil, water, and urbanization over Ahmedabad city in India. Energy Nexus 5:2021. https://doi.org/10.1016/j.nexus.2022.100044
Moumane A et al (2021) (2022) monitoring long-term land use, land cover change, and desertification in the ternata oasis, middle Draa Valley. Morocco Remote Sens Appl Soc Environ 26(October):100745. https://doi.org/10.1016/j.rsase.2022.100745
Mukherjee F, Singh D (2020) Assessing land use-land cover change and its impact on land surface temperature using LANDSAT data: a comparison of two urban areas in India. Earth Syst Environ 4(2):385–407. https://doi.org/10.1007/s41748-020-00155-9
Nabizada AF et al (2022) Spatial and temporal assessment of remotely sensed land surface temperature variability in Afghanistan during 2000–2021. Climate 10(7):111. https://doi.org/10.3390/cli10070111
Neog R (2021) Evaluation of temporal dynamics of land use and land surface temperature ( LST ) in Agartala city of India. Environ Dev Sustain. 24:0123456789. https://doi.org/10.1007/s10668-021-01572-0
Njoku EA, Tenenbaum DE (2022) Quantitative assessment of the relationship between land use/land cover (LULC), topographic elevation and land surface temperature (LST) in Ilorin, Nigeria. Remote Sens Appl Soc Environ 27:100780. https://doi.org/10.1016/j.rsase.2022.100780
Parmar M, Bhawsar Z, Kotecha M, Shukla A, Rajawat AS (2021) Assessment of land degradation vulnerability using geospatial technique: a case study of Kachchh District of Gujarat, India. J Indian Soc Remote Sens 49(7):1661–1675. https://doi.org/10.1007/s12524-021-01349-y
Ramaiah MMR, Manish RA (2020) Land cover influences on LST in two proposed smart cities of India : comparative analysis using spectral indices manish. Land. 9:292
Ritesh M, Chatterjee RS, Dheeraj K (2020) Ce pt us t”. Geocarto Int. https://doi.org/10.1080/10106049.2020.1818853
Saleem MS, Ahmad SR, Rehman SU, Javed MA (2020) Impact assessment of urban development patterns on land surface temperature by using remote sensing techniques: a case study of Lahore, Faisalabad and Multan district. Environ Sci Pollut Res 27(32):39865–39878. https://doi.org/10.1007/s11356-020-10050-5
Sam SC, Balasubramanian G (2023) Spatiotemporal detection of land use/land cover changes and land surface temperature using landsat and MODIS data across the coastal kanyakumari district, India. Geod Geodyn 14(2):172–181. https://doi.org/10.1016/j.geog.2022.09.002
Shukla A, Jain K (2019) Modeling urban growth trajectories and spatiotemporal pattern: a case study of Lucknow City, India. J Indian Soc Remote Sens 47(1):139–152. https://doi.org/10.1007/s12524-018-0880-1
Shukla A, Jain K (2021) Analyzing the impact of changing landscape pattern and dynamics on land surface temperature in Lucknow city, India. Urban Urban Green 58:126877. https://doi.org/10.1016/j.ufug.2020.126877
Suhail M, Khan MS (2019) “Assessment of urban Heat Islands effect and land surface temperature of Noida, India by using landsat satellite data”, M?APAN-journal metrol. Soc India. https://doi.org/10.1007/s12647-019-00309-9
Sultana S, Satyanarayana ANV (2019) Assessment of urbanisation and urban heat island intensities using landsat imageries during 2000–2018 over a sub-tropical Indian City”. Sustain. Cities Soc. 52(September):101846. https://doi.org/10.1016/j.scs.2019.101846
Tarawally M, Wenbo X, Weiming H, Mushore TD, Kursah MB (2019) Land use/land cover change evaluation using land change modeller: a comparative analysis between two main cities in Sierra Leone. Remote Sens Appl Soc Environ 16(September):100262. https://doi.org/10.1016/j.rsase.2019.100262
Tariq A, Shu H (2020) CA-markov chain analysis of seasonal land surface temperature and land use landcover change using optical multi-temporal satellite data of Faisalabad, Pakistan. Remote Sens 12(20):1–23. https://doi.org/10.3390/rs12203402
Tariq A, Yan J, Mumtaz F (2022) Land change modeler and CA-markov chain analysis for land use land cover change using satellite data of Peshawar, Pakistan. Phys Chem Earth 128(October):103286. https://doi.org/10.1016/j.pce.2022.103286
Thakur S et al (2021) Assessment of changes in land use, land cover, and land surface temperature in the mangrove forest of Sundarbans, northeast coast of India. Environ Dev Sustain 23(2):1917–1943. https://doi.org/10.1007/s10668-020-00656-7
Vani M, Prasad PRC (2020) Assessment of spatio-temporal changes in land use and land cover, urban sprawl, and land surface temperature in and around Vijayawada city, India. Environ Dev Sustain 22(4):3079–3095. https://doi.org/10.1007/s10668-019-00335-2
Wikipedia Landsat program (2021) https://en.wikipedia.org/wiki/Landsat_program. Accessed 21 May 2021
Acknowledgements
The Authors acknowledge the technical support provided by Indian Institute of Information Technology, Pune for conducting this research work.
Spatiotemporal Analysis of Land Surface Temperature Trends in Nashik, India: A 30-Year Study from 1992 to 2022
Funding
No funding was received was conducting this research. This work is undertaken as a part of corresponding author’s PhD research work.
Author information
Authors and Affiliations
Contributions
Kratika Sharma: Original Idea, Conceptualization, Data Collection, Data Analysis, Paper Writing.
Ritu Tiwari: Conceptualization, Paper Writing, Paper Review.
Arun Kumar Wadhwani : Conceptualization, Paper Review.
Shobhit Chaturvedi : Conceptualization, Paper Review.
Corresponding author
Ethics declarations
Competing interests
The authors declare no competing interests.
Additional information
Communicated by: H. Babaie
Publisher's Note
Springer Nature remains neutral with regard to jurisdictional claims in published maps and institutional affiliations.
Rights and permissions
Springer Nature or its licensor (e.g. a society or other partner) holds exclusive rights to this article under a publishing agreement with the author(s) or other rightsholder(s); author self-archiving of the accepted manuscript version of this article is solely governed by the terms of such publishing agreement and applicable law.
About this article
Cite this article
Sharma, K., Tiwari, R., Wadhwani, A.K. et al. Spatiotemporal analysis of land surface temperature trends in Nashik, India: A 30-year study from 1992 to 2022. Earth Sci Inform 17, 2107–2128 (2024). https://doi.org/10.1007/s12145-024-01260-3
Received:
Accepted:
Published:
Issue Date:
DOI: https://doi.org/10.1007/s12145-024-01260-3