Abstract
In the context of the new normal, enhancing digitalization to empower the transition to a green economy is a critical instrument to promote China’s economic transition from virtual to real sectors. It is also a necessary approach to realize the high-quality economic development in China. Based on panel data of 282 prefecture-level and above cities in China from 2011 to 2020, the study employs panel regression, spatial metrics, and other methods to explore the impact of urban digitization on the transition to a green economy from the dimensions of direct and indirect transmission mechanism, as well as heterogeneous effects. The findings reveal that digitalization not only exerts a positive effect on the green transition but also generates significant spatial spillover effects. The influence of digitization level on green economic transition exhibits notable regional heterogeneity. Advancement in digitization can foster green economic transition by catalyzing green technological innovation. While digitalization contributes to the green transition by optimizing the structure of energy consumption, its mediating effect is relatively modest. Therefore, it is essential to fortify the supply of digital innovative technology and strengthen digitalization and green technology innovation to jointly facilitate the transition to a green economy. This necessitates the implementation of differentiated development paths for digitization-enabled green economic transition in various regions.
Similar content being viewed by others
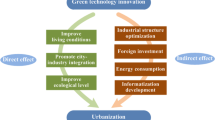
Explore related subjects
Discover the latest articles, news and stories from top researchers in related subjects.Avoid common mistakes on your manuscript.
Introduction
In recent years, China’s total economic output has risen by leaps and bounds. However, this rapid growth has been accompanied by increased energy consumption and severe environmental pollution. The crude development model has led to an increase in pollutant emissions, resulting in environmental problems such as “three wastes” emissions, deforestation, and soil erosion. According to the Global Environmental Performance Index Report released by Yale University in 2022, China ranks 160th out of 180 countries, indicating a lag in China’s environmental performance on a global scale. The quality of the ecological environment has become a hard constraint on China’s economic development. Given these facts, China must undergo a profound change to achieve high-quality economic development (Wang et al. 2022a, b). A comprehensive green transformation of the economy has become the main theme in the new era (Wang et al. 2021a). This shift aligns with the strategic objectives outlined in the report to the twentieth CPC National Congress, which emphasizes the urgency of transitioning to a greener, low-carbon development model. Consequently, China’s economic growth increasingly relies on the exploration of sustainable green development pathways to realize the transition from the original crude development model to a green development model (Li et al. 2021).
The burgeoning digital economy, fueled by technologies such as the Internet and cloud computing and propelled by emerging sectors like mobile payments, e-commerce, and the sharing economy, is experiencing rapid expansion. According to the Global Digital Economy White Paper (2022) released by the China Academy of Information and Communication Research, the scale of China’s digital economy in 2021 reached nearly 45.5 trillion, with a nominal year-on-year growth of 16.2%, accounting for 39.8% of GDP. This places China second globally, following the United States. Digitization, which lies at the core of digital economy, prioritizes digitized knowledge and information as key production factors, promoting the integration and development of digital technologies such as 5G, the Internet, artificial intelligence, and blockchain with the real economy through industrial digitization (Firdaus et al. 2022). It propels the digital upgrading and evolution of traditional industries, enhancing the efficiency of traditional production factors. It provides substantial technological and data support for the green development of the economy. Digital technology, characterized by high technology content, low energy consumption, low pollution, and low emission, is “cleaner” than physical elements. It can reduce the cost and information asymmetry of resource search, factor allocation, and green technology innovation due to its scalability, openness, and relevance (Pradhan et al. 2020). It can assist enterprises in fully acquiring, integrating, reorganizing, and effectively utilizing all kinds of environmental resources and factors for pollution control (Zhao et al. 2023b). The Plan for the Overall Layout of a Digital China further underscores the need for a synergistic transition of digitization and greening, recognizing the critical role of digital technology in facilitating green industrial transition.
Integrating digitalization, intelligence, and greening into the entire framework and giving full play to the catalytic role of digital transition in enabling green economic development are the fundamental requirements for constructing a unified national market and realizing the goal of a Beautiful China in the new era. Digitization enhances resource utilization and promotes economic development towards high efficiency, low energy consumption, and greening (Han et al. 2024). Thus, the questions arise: Can digital development serve as a new driving force for the transition to a green economy? Is there a spatial effect between the two? What is the mechanism of digital empowerment for the transition to a green economy? The exploration of these issues will provide reference for relevant academic research, policy formulation, and the transition to a green economy. This is of great significance to better utilize the advantages of digital economy in the process of energy saving and emission reduction, promoting the transition to a green economy, fostering the shift in the mode of economic growth, and realizing the goal of “double carbon.”
Literature review
The term “digital economy” was initially introduced by Don Tapscott (1996) in Prospects and Risks in the Age of Network Intelligence and then further promoted by the US Department of Commerce. It is defined as a series of innovative economic activities that leverage information and communication technology (ICT) to facilitate a wide range of applications. Since then, various research institutions have successively put forward related concepts such as network economy, virtual economy, and information economy, all of which are underpinned by the ICT industry. With advancements in information technology and “Internet + ,” the scope of “digital economy” has been continually expanded (Teece 2018). Regarding the scope of the digital economy, Moulton (1999) suggests that the digital economy mainly includes e-commerce, information technology, the corresponding ICT infrastructure, and industries related to information transmission, communication, and computing. Research in digital economy application mainly explores the measurement and analysis of digital economy (Goldfarb and Tucker 2019) and the dividend effect, in which the dividend effect focuses on the impact of digital economy upon high-quality development (Ma and Zhu 2022), innovative development (Nambisan et al. 2019; Li et al. 2022), green and low-carbon development (Zhao et al. 2023a, b; Porter and Linder 1995; Chen 2022), and coordinated development (Evans 2019).
The concept of digitization originates from and is part of digital economy. Related academic studies mainly encompass the connotation, measurement methods, and economic effects of digitization. Among these, the economic effects of digitization have been widely discussed by academics, predominantly at the micro, meso, and macro levels. At the micro level, it is chiefly reflected in the digital transition of enterprises (Wen et al. 2022) and the effect of improving business performance (Zhang and Dong 2023). At the meso-level, research has been conducted around the industrial upgrading effect of digital transition, including its impact on the industrial innovation development and low-carbon development (Liu et al. 2023; Jin et al. 2023). At the macro level, research has been centered around the upgrading effect of digital transition on economic development, which mainly includes the impacts on real economic transformation and development (Goldfarb and Tucker 2019), economic growth (Heo et al. 2019), high-quality urban development (Pagani and Pardo 2017), and urban environmental effects (Bharadwaj et al. 2013).
The concept of green development can be traced back to the 1960s when the American scholar Boulding first proposed the concept of circular economy, as well as a series of following discussions by Daley, Pierce, and others on steady state economy, green economy, ecological economy, and so on. Academic research mainly focuses on the connotation (Iradian 2009), evaluation, and influencing factors of green development. Regarding the evaluation of the level of green development, it mainly focuses on three dimensions: first, selecting a specific innovative variable for measurement, such as the level of pollutant emissions, structuralization indicators, and green innovation indicators; second, measuring the level of green development comprehensively based on the indicator system using multi-dimensional indicators by downgrading the dimension (GGGI 2019; UNEP 2008); third, measuring green total factor productivity using the data envelopment analysis method based on the input–output efficiency to assess green development performance (Foster 2017). In terms of the factors influencing green development, more scholars have found that the elements of external dynamics (institutional incentives, environmental policy tools, green credit support, and resource inputs) and internal dynamics (green innovation elements, technological progress elements, human capital, energy structure, foreign investment, industrial structure upgrading, and enterprise performance) are the driving forces of green development. This is established by constructing the theoretical model of the driving mechanism and empirical regression model (Sun and Sun 2021; Huang and Li 2017; Bowen and Hepburn 2014; Gaputo et al. 2016).
Existing literature on the impact of digitalization on the green economic transition is relatively limited. More scholars focus on the impact of digitalization on green development of industry, especially within industrial or manufacturing sectors. Chen et al. (2023) found that digital transition significantly boosts the green development of manufacturing industries and can indirectly promote it by improving the production of green products and the level of technological innovation and green investment, thereby demonstrating the “green effect.” Gaputo et al. (2016) discovered that the Internet of Things technology facilitates value creation, technology revitalization, and industrial restructuring in manufacturing. Some scholars, from the perspective of microenterprises, examine the impact of digitization on enterprise total factor productivity (Peng et al. 2022; Acemoglu and Restrepo 2018), enterprise green technology innovation (Feng et al. 2022), and enterprise performance (Li et al. 2018; Briel et al. 2018). Other scholars believe that the development of digital economy can enhance green ecological efficiency and environmental governance and that the performance of environmental governance can be enhanced by strengthening the level of environmental regulation and green technological innovation (Xu et al. 2022). Only a few scholars have conducted relevant studies on the impact of digital economy on green and low-carbon development, green innovation, etc. (Wang et al. 2022a). Zhang et al. (2023) argued that the digital economy can significantly enhance the level of green economic development, with environmental regulation playing a double-threshold role on the relationship between digital economy and green economy. Zhang et al. (2022) suggested that digital economy can promote green development through technological innovation and industrial restructuring.
While research on the digital economy and green economy has flourished in their respective spheres, there is a scarcity of literature that integrates digitization and the transition to a green economy within a unified analytical framework. Although some scholars have made preliminary explorations, they have mostly focused on the digital economy perspective. This study delves into the mechanisms through which digitalization impacts the transition to a green economy and analyzes its spatial effects. This approach is beneficial for leveraging the advantages of digitization, promoting the digital transformation of green and low-carbon technologies, and advancing changes in the mode of economic growth and the sources of growth momentum. The marginal contributions to the existing literature are as follows: first, it takes the perspective of prefecture-level cities by taking 282 prefecture-level and above cities in China as research objects. Basic panel regression models and spatial econometric regression models are set up to examine the direct and spatial impact effects of digitization on the green economic transition. Second, to investigate the indirect impact mechanism of digitization level on green economic transition, two mediating variables, namely, green technology innovation and energy consumption structure, are selected to examine the indirect impact of digitization on green economic transition. Finally, the study delves into the impact of digitization on green economic transition in different regions of China from the perspective of heterogeneous effects. This provides a reference for how different regions can implement digitization to promote the dividend effect of green economic transition.
Theoretical analysis and research hypotheses
Digitalization, through the integration of data, algorithms, and application scenarios, promotes the digital transition of traditional market elements such as labor and capital. By digitizing all factors, it improves the efficiency of factor use and accelerates the transition of traditional high-energy-consuming industries, such as labor-intensive industries towards new technology-intensive industries, thereby elevating the level of green development. Digitalization uses new digital technologies such as artificial intelligence, 5G technology, cloud computing, the Internet, and blockchain to promote the transformation of traditional industries to intelligence and green (Dewan and Kraemer 2000) by taking information and data as key elements. With its precise information retrieval, collection, analysis, and professional assessment capabilities, digital technology can solve information asymmetry issues, improve market efficiency, and support the green transition of traditional industries by locating and identifying green projects with both high investment value and ecological benefits. The application of digital technology enables real-time monitoring of ecological and environmental changes in the production process, effectively aiding in pollution source control, reducing resource waste, and cutting pollutant emissions. The use of industrial Internet, Internet of Things, and other digital technologies reduces transaction costs of production and marketing, urban–rural, and internal–external connectivity and increases enterprise output efficiency and technology level. This leads to more efficient energy use in enterprises, reduced energy consumption, and a greener transition of business and industries. In addition, through mobile Internet and other technologies, the sharing of information platforms makes it easier for the public to provide relevant environmental pollution behaviors, urging the government and business sectors to increase pollution emission control and promote the development of green economic transition (Basu and Fernald 2007).
Digital technology plays a pivotal role in improving the efficiency of energy and resource use and in promoting the development and utilization of renewable energy sources, thereby reducing the demand for energy and raw materials. Under certain conditions, digitalization can promote the decoupling of economic growth from environmental pollution. The environmental Kuznets curve shows that, in the medium to long term, as the economy evolves from mainly producing industrial goods to being service-oriented, the structural and technological effects of economic activity lead to a reduction in the negative environmental impact of economic development. Information and communication services are considered key drivers of labor mobility from the secondary to the tertiary sector. The development of high-tech service industries has contributed to the decoupling of economic growth from environmental pollution. Industrial Internet technology not only digitally tracks and monitors all aspects of the product but also enables on-demand supply and efficient production for energy efficiency (Kokina and Blanchette 2019). The theory of ecological modernization holds that information technology, artificial intelligence, and other forms of digitalization can fundamentally enhance the efficiency of factor resource allocation and promote the transformation of industrial civilization to ecological civilization, ultimately realizing the green economic transition.
Digitalization provides convenient market space for production, distribution, flow, and exchange via interconnected network technology such as transportation, logistics, and information. Digitalization breaks the traditional constraints of time and space in the flow of factor resources, erasing regional boundaries and market segmentation. The enhanced efficiency in information transfer significantly reduces the spatial distance between different regions, leading to more frequent economic activities between different regions and efficient integration of market factors across the domain of space and time. Digitalization enhances the breadth and depth of market factor transmission and transfer, facilitating the sharing of data elements in different regions. This forms a networked innovation ecology, which will have an impact on the green economic transition of both the local and neighboring regions, accelerating the process of market integration. The rapid advancement and spread of advanced digital technologies have significantly improved the capacity of information transmission, storage, and processing between different regions, rapidly reducing the processing cost and enhancing the data mobility and accessibility. The information symmetry among market subjects has been improved, eliminating regional information barriers. The information dissemination capacity and efficiency across different spaces have been enhanced, fostering the green economic transition in different regions. Based on this, the following hypothesis is proposed:
Hypothesis 1:
Digitization has a positive contribution and spatial spillover effect on the development of green economic transition.
Digitization can accelerate green technology innovation by promoting the innovation and accumulation of human capital in the process of data flow. It encourages cooperative institutions such as enterprises, governments, and universities to improve R&D efficiency and conduct scientific research on green technology barriers. With the continuous advancement of environmental regulations and environmental technology standards, enterprises can enhance their competitive advantages and market competitiveness by implementing green technology innovation, thus boosting the green economic transition. From the producer’s perspective, digitalization can optimize and reorganize product manufacturing, design, R&D, workflow, and resource utilization through effective data sharing and use. Digitalization enables the optimal allocation of production and pollution control production factors, allowing enterprises to obtain the Metcalfe effect, reduce marginal innovation costs, enhance innovation levels, and provide technological support for green innovation (Takalo and Tooranloo 2021). The government incentivizes enterprises to adopt green production and bolsters their willingness for green technology innovation through the implementation of environmental regulations and real-time monitoring of enterprises, thereby improving their innovation capacity of green technology. The advantages of digital technology, such as intelligence and digitization, provide conducive environmental conditions and diverse elements of innovation for green technological advancements, thus boosting the green economic transition (Luo et al. 2023).
Digitalization, by monitoring, collecting, and evaluating the big data of production and manufacturing from energy-intensive industries, provides a feasible and referential path for green economic transition. It effectively reduces pollution emission levels and production energy consumption. Digitalization encourages the optimization of the energy consumption structure by optimizing the energy consumption market, leading enterprises to innovate actively to optimize and improve the energy structure, thereby promoting the green economic transition. However, the promotion and application of digital technology directly increases energy intensity, and the energy demand caused by the development of digitally empowered economy will increase energy consumption, thus adversely affecting the green economic transition (Li et al. 2022). The use of digitalization in the electric power and energy industries will consume a large amount of electrical energy. The large use of coal power will increase coal consumption, thus exacerbating carbon emissions and posing challenges to the green economic transition (Nasir et al. 2022). Based on the above analysis, the following hypothesis is proposed:
Hypothesis 2:
The level of digitalization can promote green economic transition by enhancing green technology innovation and altering the energy consumption structure.
In the process of industrialization, a unified national market has not yet been fully established, leading to significant disparities in the level of digitization across different regions and types of cities due to varying geographic environments, resource endowments, and industrial bases. This results in different impacts on the development of green economic transition. Regions such as eastern coast and other economically advanced areas, with a robust industrial foundation and high levels of technological innovation, have greater green transition power. The compensation effect of these regions is prominent, contributing to an elevated level of green economic transition. Conversely, in economically weaker areas like the western or the northeastern regions of the country, where the level of marketization is less advanced and the industrial base is relatively weak, there are more high energy-consuming and high-polluting enterprises, and the industry is still based on the extensive and quantitative growth. While the digital transition can promote the industrial production capacity, it may also lead to increased emission of pollutants. Given the bottlenecks in technological innovation, the cost of pollution control cannot be compensated by the revenue from increased production capacity. These regions are still in a phase of rapid economic development, where environmental considerations are sometimes sacrificed for economic benefits. Digitalization may have a limited effect on the level of green transition and development in these regions. As the level of digitization increases, the innovation capacity of enterprises gradually improves, and enterprises have the awareness to improve the level of green technology innovation and thus promote the green economic transition. Compared with non-resource cities, the level of green economic transition in resource cities is lower, with problems such as high difficulty of transition and insufficient motivation for change. There is an obvious difference in the effect of digitization on green economic transition in different types of cities. Based on this, the following hypothesis is proposed:
Hypothesis 3:
The effect of digitalization on the development of green economic transition exhibits regional heterogeneity.
Methodology and data
Based on the theoretical analysis and the research objectives previously outlined, this article mainly focuses on the impact of digitalization on green economic transition and on the mediating roles of green technology innovation and energy consumption structure. The variable settings of this study are as follows.
Variable settings
Explanatory variable: green economic transition (GET)
In February 2021, the “Guiding Opinions on Accelerating the Establishment of a Sound Green, Low-Carbon and Cyclic Development Economic System” was issued by State Council of the Communist Party of China Central Committee. This document laid out a comprehensive framework for developing a green, low-carbon, and cyclic economic system from the perspective of building a green, low-carbon, and cyclic development production, circulation, and consumption system, promoting the green upgrading of infrastructures, constructing a green technological innovation system, and perfecting the legal and regulatory policy system. Based on this, green economic transition index (GET) is constructed, which is mainly grounded in the theory and concept of green economic transition, and primarily focuses on the “Green Transition of Production (Industrial Transition, Green Innovation), Green Transition of Ecology (Pollution Control, Low-Carbon Development, Environmental Quality), and Green Transition of Life (Infrastructure, Green Consumption).” The GET is mainly constructed from the spatial perspective of the “three lives” to build a trinity of green economic transition index (Table 1).
Core explanatory variable: level of digitization (Dige)
The indicator system is established from three dimensions—digital infrastructure, digital industrialization, and industrial digitization—to determine the weights by combining the weighting method (Ren et al. 2021) (Table 2).
Mediating variables
This study selected green technology innovation and energy consumption structure as mediating variables (Chen et al. 2023):
-
1)
Green technology innovation level (Gp). This is measured by taking the logarithm of the total number of green utility model patent applications and green invention patent applications.
-
2)
Energy consumption structure (Ene). This is represented by the proportion of coal consumption to GDP.
Control variables
This study selected economic development, wage level, population size, intensity of government expenditures, industrial structure, the degree of foreign direct investment, and infrastructural development as the seven control variables (Zeng et al. 2023; Lin and Shao 2006).
(1) Economic development level (pGDP): measured by the logarithm of GDP per capita. (2) Wage level (Wage): measured by the average wage of employees. (3) Population size (POP): measured by the density of urban population. (4) Government expenditure intensity (Gov): expressed by the share of local fiscal expenditures in GDP. (5) Industrial structure (Ind): measured by the proportion of the secondary industry value-added in GDP. (6) Foreign direct investment (FDI): expressed by the total amount of foreign direct investment actually utilized. (7) Infrastructural development (Infra): measured by the per capita road area. All price data are smoothed with 2008 as the base period.
Research methods
Utility function method
The utility function synthesis method is used for the assignment.
Step 1: Normalize the indicators.
Positive indicators:
Negative indicators:
Zi represents the normalized value of the data. Xi is the original value of the data, while Xmax and Xmin are the maximum and the minimum value of the original data set, respectively.
Step 2: Calculate the weight and synthesize the data. However, it is important to note that the synthesized index method has certain defects. For instance, if one index scores high, it will overshadow other indexes with lower scores. This can result in a higher overall score and lead to evaluation bias.
Assuming that the individual utility function of residents is as follows:
E, S, and D represent the indicators of green transition of production, ecology, and life, respectively. Each region calculates \(\alpha ,\beta ,\gamma\) separately and synthesizes the comprehensive index of green economic transition using individual utility function.
First, calculate the gap between each region’s score on these three Tier 1 indicators and the highest-scoring:
Second, different weights are assigned to each of the three indicators according to the degree of “shortcomings” in each region:
Finally, the individual utility function is applied to calculate the green transition development score for each region.
Panel regression model
To examine the direct impact of digitization on green economic transition, the following baseline model is set:
GET represents the level of green economic transition. Dige represents the level of digitization, while control stands for the control variables. i and t represent the city and year, respectively. εit is the random error term.
Spatial regression model
Due to the free flow mechanisms of market factors such as data and technology, digitization tends to be spatially distributed and universally connected. Therefore, the level of digitization in a specific region cannot be viewed in isolation. There are usually spatial effects on the level of digitization in different cities. This study builds up spatial Durbin model (SDM):
\(\delta\) represents the impact of development level on local economic transition to the level of neighboring green economic transition. X is the matrix of control variables. W stands for the matrix of spatial weights, using the geographic distances of 282 prefecture-level and above cities in China as weights. The meanings of the remaining variables are as previously defined. \({\mu }_{it}\) is the vector of random error terms, and \(\lambda\) is the autoregressive coefficient of spatial error. \({\varepsilon }_{it}\) obeys the normal distribution. The other variables maintain the previous defined meanings.
Study area and data sources
Based on data availability, panel data of 282 prefecture-level and above cities in China spanning from 2011 to 2020 are used as samples for analysis. The sample excludes cities like Bijie, Qinzhou, Haidong, Sansha, Suihua, Lhasa, Tongren, Turpan, Hami, Danzhou, and various autonomous prefectures and leagues due to significant data missing. The relevant data for various indicators are mainly sourced from China Statistical Yearbook, China Urban Statistical Yearbook, China Environmental Statistical Yearbook, the EPS database, and the CNRDS database. Some missing data are supplemented from neighboring years in the region using mean replacement and interpolation methods. The descriptive statistics for the main variables are presented in Table 3.
Empirical results and discussion
Benchmark regression analysis
The likelihood ratio and Hausman test are employed for model selection and comparison. The results indicate that the fixed effect model is used for estimation as shown in Table 4. Models (1) and (2) represent the linear effects of digitization level on green economic transition before and after incorporating control variables, respectively. After adding control variables, the influence coefficient is 0.0182, suggesting that an enhancement in digitization can significantly promote green economic transition. This aligns with the findings of Ma and Zhu (2022) and Wang et al. (2021a, b), which demonstrated that digitization can suppress pollutant emissions and promote the development of green economy (Shen et al. 2024). Digitalization, through the use of mobile Internet and other technologies, accelerates the flow of data and other factor markets. This provides technical support for green economic transition and promotes the transition of traditional high-energy-consuming industries, such as labor-intensive industries, towards new industries such as technology-intensive industries, so as to enhance the level of green transition. Furthermore, a rising level of digitalization promotes the continuous improvement and optimization of industrial structure. This not only reduces resource mismatch and pollution emissions but also enhances production efficiency and transaction efficiency (Sibt-e-Ali et al. 2024). Digitalization has demonstrated a strong driving force across all areas of production and life, including green production, green consumption, and green distribution. It has become a new driving force for China’s green development.
The regression results of the control variables in model (2) indicate that the influence coefficients of both the levels of economic development and wage on the advancement of green economic transition are significantly positive. As the levels of economic development and the wage improve, there is a corresponding enhancement in people’s living standards, and the atmosphere of the whole society’s green transition is strengthened, which promotes the development of green economic transition. The influence coefficient of population density in relation to the green economic transition is significantly positive. An increase in population means a rise in human capital, which provides talent support for economic development, thereby enhancing the technological effect, which in turn promotes the progress of green economic transition. The influence coefficient of government support is significantly positive, and government financial support can increase green innovation and R&D investment to promote green transition. In contrast, the influence coefficient of the proportion of the secondary industry on the green economic transition is significantly negative, indicating that the secondary industry is still dominated by high-pollution and high-energy-consuming industries, and an increase of the proportion of the secondary industry is not conducive to the development of green economic transition. Therefore, the orderly development of the tertiary industry could be an effective measure to enhance green economic transition in the future. The influence coefficient of foreign direct investment on the green economic transition is 0.0413, statistically significant at the 5% significance level. Through the “demonstration effect” of foreign investment and the “learning effect” of domestic enterprises, the green performance of enterprises is enhanced, thus promoting green economic transition. Lastly, the influence coefficient of infrastructure on green economic transition is significantly positive, and infrastructure provides a conducive ecological environment for the green development of regional economy.
Mediating effect
Academia typically uses the stepwise method to measure the mediating effect in mechanism tests. But this method may yield biased estimates of mediating effect due to the endogeneity issues with the mediating variables (Bullock et al. 2010). In light of this, this study, referencing Zhang (2020), utilizes the following mediating effect test method for mechanism testing: it introduces a mediating variable to analyze only the impact of the core explanatory variables on the dependent variable. The impact of this mediating variable on the dependent variable is either evident or can be deduced based on the economic theory and the findings of related literature. Based on this, the following mediating effect model is constructed:
Med, the mediating variable, mainly includes the level of green technology innovation (Gp) and energy consumption structure (Ene). \({\beta }_{1}\) reflects the effect of the digitization level on the mediating variables (Burki and Dahlstrom 2017).
In Table 5, model (1) shows the effect of digitization level on green technology innovation. The influence coefficient is 4.0391, indicating an increase in digital development can significantly promote green technology innovation. Digital development encourages enterprises to innovate through technical support, facilitates the transition and upgrading of traditional industries, supports the growth of green industries such as clean production and clean energy, and promotes green innovation. An enhanced level of digitalization encourages enterprises to engage in green technological innovation, leading to improved technologies and processes, growth in environmental protection industries, reduced energy consumption, and ultimately, the promotion of green economic transition.
Model (2) in Table 5 shows the impact of digitalization on energy consumption structure. The development of digitalization can significantly improve the energy consumption structure, but the influence coefficient is relatively small at − 0.0013, indicating that the effect of digitalization on improving the energy structure is limited. The energy consumption structure in China is still dominated by traditional coal, and the transition to more sustainable energy sources requires a high level of digitization. Currently, the level of digitalization is not sufficient to make a significant impact on the optimization of the energy structure. However, the importance of optimizing the energy structure to enhance green economic transition is undeniable. Given that China’s current level of digitization is still relatively low, relying only on digital transition to optimize the energy consumption structure is challenging. Future efforts should focus on accelerating the research and development of green technology, promoting technological research, and optimizing the energy consumption structure.
Heterogeneity analysis
To examine the impact of digitization level on the development of green economic transition across different regions in a more detailed way, this study categorized 282 prefecture-level and above cities of the country into four major segments: eastern, central, western, and northeastern regions, for the purpose of heterogeneity analysis.The results are shown in Table 6.
The influence coefficients of digitization on green economic transition in the eastern and central regions are significantly positive, 0.0221 and 0.0364, respectively. This indicates a particularly strong effect of digitalization in the central region, suggesting that the digital development strategy helps to promote the green economic transition there. In the central region, most of the industrial value chain is at the middle and low-end level, and the level of industrial high-end and intelligence is low. At this time, increasing the level of digitization will have a significant impact on green economic transition. The enhancement in digitization drives green economic growth, improving enterprise productivity and energy efficiency. The greening dividend effect is obvious. It contributes to reducing pollution emissions and aiding the green economic transition. Conversely, in the eastern region, the level of digitalization development is higher. The dividend effect of digitalization on the green transition shows a weakening trend. The eastern region is dominated by strategic emerging industries, which itself has a higher level of greening, thus weakening the promotion effect.
The impact of digitization on green economic transition in western region is not significant. Many cities in the western region are rich in resource. As the digitization level rises, so does the industrial energy demand, leading to a significant rise in energy extraction and consumption. Therefore, while digitization enhances the efficiency of industrial green development, it simultaneously escalates energy demand. These conflicting influences on green economic transition neutralize each other; thus, the impact of the effect is not significant in this region.
The influence of digitalization on the development of green economic transition in northeast China shows a U-shaped trend of initially decreasing and then increasing. When the level of digitalization is low, digitalization is not conducive to green economic transition. However, as digitization level rises, its positive influence of green economic transition gradually becomes apparent. The current industrial structure of the northeast region is more backward, and the economic efficiency is poor. When the digitization level is low, enterprises are trying to expand their production capacity and improve their productivity during the process of enhancing intelligence. There is less awareness of green transition, leading to increased energy consumption and pollutant emissions during the process of increasing productivity, which adversely impacts green economic transition. Yet, as the level of digitization gradually increases, enterprises start to integrate ecological civilization and environmental protection in the expansion of production capacity. This shift leads to a balance between increasing production capacity and protecting environment, resulting in reduced energy consumption and pollution, thus promoting the development of green economic transition.
To examine the effect of digitization on green economic transition under resource endowment heterogeneity, cities are categorized into two types of samples: resource-based cities and non-resource-based cities, to facilitate heterogeneity analysis. The results, as shown in Table 7, reveal that the influence coefficient of digitization on green economic transition in resource-based cities is not significant, while the improvement of digitization level in non-resource-based cities can significantly promote green economic transition. This may attribute to the lower economic development level in resource-based cities, and more cities are resource-rich areas, compared with non-resource-based cities. As digitalization level increases, it leads to rising industrial energy demand, resulting in a significant increase in the amount of energy extraction and consumption. The enhancement of green industrial development leads to an increase in energy demand and results in conflicting impacts on green economic transition, thereby neutralizing the overall effect on green economic transition. In non-resource-based cities, as the level of digitization improves, the awareness of green development is also gradually enhanced, enhancing the quality of green economic development, thus promoting green economic transition. Hypothesis 3 is verified.
Robustness test
(1) Replacement of explanatory variables. The industrial sector is the main source of material wealth production as well as pollution emissions, and thus, it is the central to the green economic transition. Industrial sector data is used to represent the level of green economic transition. Industrial sulfur dioxide (SO2) serves as a proxy variable for the level of green economic transition. As a major pollutant emitted by industrial enterprises, the volume of SO2 emissions reflects the degree of green development within the industry. Re-conducting the regression with this approach, as shown in Table 8, model (1), reveals that an increase in the level of digitization significantly reduces industrial SO2 emissions, thus promoting green economic transition and reinforcing the robustness of the conclusion.
(2) Staged regression method. In July 2015, the release of the State Council’s Guiding Opinions on Actively Promoting the “Internet Plus” Action implies that the development of the digital economy has risen to a national strategy, and the scale has been rapidly expanding since 2016. Based on this, the sample is divided into two time periods: 2011–2015 and 2016–2020, resulting in sub-sample regression results (Table 8, models (2) and (3)). In the 2011–2015 period, digital development had a negative inhibitory effect. This may be attributed to a weaker national focus on both digital development and ecological civilization ideology before 2015. The main purpose of improving the level of digitalization was to increase production capacity and scale through technological innovation, which was not mainly used for green transition. Consequently, enterprises expanded production capacity but also increased pollutant emissions, adversely impacting green economic transition. In 2016–2020, the level of digitization significantly improved green economic transition, with a regression coefficient notably larger than the benchmark regression. This indicates that with the improvement of “Internet + ”, digitization and awareness of ecological civilization were gradually enhanced. Enterprises focused on both enhancing digitalization transition to expand production capacity and at the same time embracing green development, thus promoting the green economic transition.
(3) Excluding municipalities and provincial capitals, the results of the regression are shown in Table 8, model (4). The influence coefficient of digitization on the green economic transition remains significantly positive.
(4) Endogeneity discussion. While digitization affects green economic transition, as the level of green economic transition increases, the need for green transition propels enterprises to undergo digital transition, which may hinder the development of digitization. If a bidirectional causal relationship exists between digitization and green economic transition, it may trigger estimation bias or inconsistency in the results. To address this, the following two methods are adopted. First, to mitigate the “first-mover advantage” of economically advanced cities in adopting digitalization, province fixed effects and province-year interaction effects are introduced to exclude the changes in the macro-systemic environment brought about by the adoption of digitalization technology. Table 9 model (1) shows that the estimated coefficient of digitization on the green economic transition remains significantly positive and relatively unchanged, indicating the robustness of the estimation results. Second, the instrumental variable method is used to solve the endogeneity issue. The lagged item of the digitization level is used as an instrumental variable, with estimation conducted using 2SLS method. The first-stage regression results confirm the validity of the chosen instrumental variable. The results of the second-stage regression indicate that digitalization still contributes significantly to green economic transition, reaffirming the robustness of the conclusion after considering endogeneity.
Further analysis: spatial spillover effects
Due to the varying geographic locations of different cities, there are disparities in their physical conditions, green production and living environments, resource endowments, ecological resources, and environmental performance. These factors only affect a city’s own green economic transition but also impact development of green economic transition in neighboring areas. Therefore, spatial autocorrelation between digitalization and the development level of green economic transition is measured. Moran’s I was utilized to test the spatial correlation. The results, calculated using Matlab on the spatial index of the green economic transition and the digitization, are shown in Table 10. It shows that both the development level of green economic transition and digitization have significant spatial correlation, with an accompanying probability of ≤ 0.05. This indicates that the two variables exhibit the characteristics of agglomeration in the spatial distribution.
By applying the LR test, the spatial Durbin model (SDM) is established for regression. Through Hausman test, a fixed effects model was found to be regressed, and finally, the spatial Durbin model (SDM) and fixed effects model are selected for main regression analysis. The results of the spatial regression model are presented as models (1) and (2) in Table 11. The influence coefficient of digitalization on the development of green economic transition is significantly positive, as are the spatial spillover effects. As depicted in model (2), the influence coefficient of local digitalization development on the green economic transition in the surrounding area is significantly positive with a value of 0.7640, indicating that digitalization in one area can promote the green economic transition in surrounding cities. The enhancement of digitalization level leads to a strong scale agglomeration effect and diffusion effect on economic development, accelerates the flow of factor market, and optimizes the market-oriented allocation level in both local and peripheral cities, thus promoting the green economic transition of peripheral cities. The influence coefficient of the green economic transition of neighboring cities on the region’s transition is 1.2621, with a significant positive influence, indicating that the green economic transition of neighboring cities will have a positive driving effect on the region. The findings indicate that the development of green economic transition in different regions exhibits a mimetic effect. Regions accelerating innovation and green industrial transition will lead to the implementation of similar policies in neighboring regions, and the flow of green elements between regions promotes the development of green economic transition.
To further verify the spatial effect of digitization on the development of green economic transition, the spatial weight matrix of economic geography (W1) and the inverse of GDP per capita difference (W2) are constructed, replacing the previous spatial weight matrix. In W1, the non-diagonal element is calculated as the product of the inverse geographic distance between cities and the ratio of the city’s GDP per capita to the GDP per capita of 282 cities, with a diagonal element of 0. For W2, the non-diagonal element is the inverse of the absolute difference in GDP per capita between two cities. The results indicate that the sign and significance of the coefficients do not change significantly (Table 11, models (3) and (4)). It shows that digital empowerment has significantly positive spatial spillover effects on the green economic transition.
The direct effect analysis can reflect the impact of digitalization on the green economic transition within a specific region, while the indirect effect analysis reveals the impact of digitalization on the green economic transition of other regions. Therefore, the results of the indirect effect analysis can represent the spatial spillover effect, illustrating how the level of digitalization can affect the green economic transition. The spatial effect of digitization on green economic transition has been decomposed, with the results shown in Table 12. The direct, indirect, and total effects of digitization on green economic transition are 0.0232, 0.3386, and 0.3618, respectively, all of which are significant. Digitization not only promotes the development of green economic transition within a specific region but also has a spatial diffusion effect, which also plays a significant role in promoting the development of green economic transition in neighboring cities. This could be attributed to a “copycat effect” that the increase in the level of digitization would have on the surrounding areas, and the governments of the surrounding areas will be motivated by the comparison effect, thus promoting the green economic transition by increasing administrative actions or financial inputs.
Digitalization can facilitate the transition to a green economy through energy saving and emission reduction. The underlying logic may lie in the organic integration of digital technology, which contains technological advancement and green attributes, with the original production method. This integration can help realize the lean management of the production process, thereby reducing the total energy consumption across the entire life cycle of a product. It can facilitate the transformation of the production process into a cleaner and greener one. The ultimate performance of the digital improvement can reduce the level of pollutant emissions, thus realizing the transition to a green economy. Digitization can yield an energy use efficiency improvement effect and green technology progress effect. It can steer the industry’s production mode towards low energy consumption and low emissions, ultimately promoting the transition to a green economy.
Conclusion, implications, and further study directions
Actively leveraging digitalization as an internal driving force of marketization, it is crucial to study the impact of core elements of digitalization on green economic transition. Various methodologies, including panel regression, spatial econometric model, two-stage least squares, and GMM are established to study the impact of digitalization on green economic transition. The main conclusions are as follows.
-
(1)
Digitalization has a positive and facilitating effect on green economic transition, along with a notable positive spatial spillover effect. The development of digitalization technology not only has a positive spatial spillover effect on the green transition of local economy but also promotes the green transition of neighboring cities driven by the flow of market factors. The integration of digital technology, digital infrastructure, and traditional industries further promotes the green transition of industries. It unlocks the value of data in the entire field, improves the efficiency of the whole production process, reduces the energy consumption of the entire chain, and realizes the dual enhancement of production efficiency and energy efficiency.
-
(2)
The impact of digitization on green economic transition exhibits regional heterogeneity. In the eastern and central regions, digitization has a positive effect on green economic transition. However, in the western region, the impact is not significant. In the northeastern region, the effect of digitization on green economic transition shows a “U”-shaped trend of decreasing and then increasing. From the perspective of city types, the influence coefficient of digitization on green economic transition in resource-based cities is not significant, while the level of digitization in non-resource cities can significantly promote green economic transition.
-
(3)
The level of digitization influences green economic transition through the mediating effect of green technology innovation and energy consumption structure. The improvement of digitization level can boost green economic transition by fostering green technological innovation in enterprises. Digitalization contributes to green economic transition by optimizing the energy consumption structure, although the mediating effect is relatively modest. Given that China’s current level of digitization is still low, relying only on digital transition to optimize the energy consumption structure is more challenging. Therefore, future efforts should focus on accelerating the research of green technology, promoting technological research, and optimizing the energy consumption structure.
Based on these research conclusions, the following recommendations are proposed:
-
(1)
Accelerate the upgrading of the digitization level. Adopt “Industrial Brain + Future Factory” model as the core structure to integrate digital technology in the process of “greening”, thereby jointly advancing green economic transition. Accelerate the construction of digital infrastructure and promote the establishing of an urban environmental information data platform. Actively promote the digital and intelligent transition of cities, aligning industrialization, informatization, and greening efforts for synergistic development. Encourage enterprises to realize whole-process digital transformation in R&D and design, production services, and operation and management. Utilize digitalization to promote green development such as improving quality, increasing efficiency, lowering costs, and reducing consumption. Encourage enterprises to use big data in collecting vital production and management data, and carry out “intelligent + green” transition of all elements. Apply digital technologies such as artificial intelligence, big data, and blockchain to promote green economic transition. Accelerate the pilot demonstration of intelligent and green manufacturing. Prompt enterprises to build industrial Internet facilities and platforms, encouraging and guiding universities and research institutions to collaborate with enterprises on multi-faceted industrial digital transition technology.
-
(2)
Strengthen digitalization and green technology innovation to jointly promote the transition to a green economy. Promote the application of digital technology in the process of “greening.” Accelerate the construction of a market-based green technology innovation system, and realize the integration of digital technology and green innovation. Accelerate the integration and intersection of digital technology with new energy development, pollution control, clean technology, green manufacturing, and other new fields. Encourage enterprises to establish research and development platforms, focusing on research in green, low-carbon, energy-saving, and environmental protection technologies. Deeply explore the potential for innovation and synergistic development between digitalization and green technologies, particularly in specialized, unique, new, and science and technology-based enterprises and in monopolistic leading enterprises. Promote the digital transformation of energy infrastructure to build smart energy, reduce energy intensity, and realize the economical use of resources. Enhance the digital content of green technological innovation, reduce pollutant emissions, and boost the development of the transition to a green economy.
-
(3)
Implement differentiated development paths for digitization-enabled green economic transition in different regions. It can guide cities to implement a differentiated digital industrial layout with local characteristics, rationally allocating and optimizing data elements to avoid the convergent development of digital industries and the wasteful duplication of limited resources. In the western region, which has the advantage of resource and energy enrichment, the focus should be on promoting the digital development of industries and the use of clean energy generation methods such as hydro, photovoltaic, and wind power. This can help cultivate and grow energy-saving and environmental protection and other green industries and create a green recycling industrial system. The central region, as an energy-intensive or chemical industry cluster, should strengthen the digital transformation of high-pollution and high-energy-consuming industries, improve the level of resource conservation and intensification, and boost green, low-carbon, and recycling development. The eastern region should continue to promote the penetration and integration of digital technology with the real economy and cultivate internationally leading digital technology research and development and high-precision industries.
This article attempts to explain green economic transition through the lens of digitization. However, it does not thoroughly analyze whether green transition also impacts digitization, an area ripe for future research. Additionally, while various factors affect the green economic transition, due to data limitations, this study does not include all control variables. It is necessary to collaborate with relevant departments to obtain more data and conduct a more comprehensive analysis of the impact mechanisms involved. Lastly, the selection of indicators for the green economic transition is highly subjective and may require further exploration in future studies.
Data availability
The data that support the findings of this study are available from the author upon reasonable request.
References
Acemoglu D, Restrepo P (2018) The race between man and machine: implications of technology for growth, factor shares and employment. Am Econ Rev 108(6):1488–1542. https://doi.org/10.1257/aer.20160696
Basu S, Fernald J (2007) Information and communications technology as a general-purpose technology: evidence from US industry data. Ger Econ Rev 8(2):146–173. https://doi.org/10.1111/j.1468-0475.2007.00402.x
Bharadwaj A, El Sawy OA, Pavlou PA, Venkatraman N (2013) Digital business strategy: toward a next generation of insights. MIS Quarterly 37:471–482. https://doi.org/10.25300/MISQ/2013/37:2.3
Bowen A, Hepburn C (2014) Green growth: an assessment. Oxf Rev Econ Policy 30(3):407–422. https://doi.org/10.1093/oxrep/gru029
Briel FV, Davidsson P, Recker J (2018) Digital technologies as external enables of new venture creation in the IT hardware sector. Entrep Theory Pract 42(1):47. https://doi.org/10.1177/1042258717732779
Bullock JG, Green DP, Ha SE (2010) Yes, but what’s the mechanism? (don’t expect an easy answer). J Pers Soc Psychol 98(4):550. https://doi.org/10.1037/a0018933
Burki U, Dahlstrom R (2017) Mediating effects of green innovations on interfirm cooperation. Australas Mark J 25(2):149–156. https://doi.org/10.1016/j.ausmj.2017.05.001
Chen P (2022) Relationship between the digital economy, resource allocation and corporate carbon emission intensity: new evidence from listed Chinese companies. Environ Res Commun 4(7):75005. https://doi.org/10.1088/2515-7620/ac7ea3
Chen S, Yang Y, Wu TT (2023) Research on the impact of digital transformation on green development of manufacturing enterprises. Front Energ Res 10:1045328. https://doi.org/10.3389/fenrg.2022.1045328
Dewan S, Kraemer KL (2000) Information technology and productivity: evidence from country-level data. Manage Sci 46(4):548–562. https://doi.org/10.1287/mnsc.46.4.548.12057
Evans O (2019) Repositioning for increased digital dividends: Internet usage and economic well-being in Sub-Saharan Africa. J Glob Inform Technol Manag 22(1):47–70. https://doi.org/10.1080/1097198x.2019.1567218
Feng H, Wang FY, Song GM, Liu LL (2022) Digital transformation on enterprise green innovation: effect and transmission mechanism. Int J Environ Res Public Health 19(17):10614. https://doi.org/10.3390/ijerph191710614
Firdaus R, Xue Y, Gang L et al (2022) Artificial intelligence and human psychology in online transaction fraud. Front Psychol 13(10):947234. https://doi.org/10.3389/fpsyg.2022.947234
Foster JB (2017) The long ecological revolution. Monthly Rev 69(6):1–16
Gaputo A, Marzi G, Pellegrini MM (2016) The Internet of things in manufacturing innovation processes development and application of a conceptual framework. Bus Process Manag J 22(2):383–402. https://doi.org/10.1108/bpmj-05-2015-0072
GGGI (2019) Technical report No.5, Seoul: Global Green Growth Institute.
Goldfarb A, Tucker C (2019) Digital economics. J Econ Lit 57(1):3–43
Han YR, Javaid MQ, Ali MSE et al (2024) Revisiting the nexus between digital trade, green technological innovation, and environmental sustainability in BRICS economies. Environ Sci Pollut Res 31(6):8585–8607. https://doi.org/10.1007/s11356-023-31661-8
Heo PS, Lee DH (2019) Evolution of the linkage structure of ICT industry and its role in the economic system: the case of Korea. Inform Technol Dev 25(4):424–454. https://doi.org/10.1080/02681102.2018.1470486
Huang JW, Li YH (2017) Green innovation and performance: the view of organizational capability and social reciprocity. J Bus Ethics 145(2):1–16. https://doi.org/10.1007/s10551-015-2903-y
Iradian G (2009) What explains the rapid growth in transition economies? IMF Staff Pap 56(4):811–851. https://doi.org/10.1057/imfsp.2009.1
Jin CY, Xu AT, Zhu YH, Li JC (2023) Technology growth in the digital age: evidence from China. Technol Forecast Soc Chang 187:122221. https://doi.org/10.1016/j.techfore.2022.122221
Kokina J, Blanchette S (2019) Early evidence of digital labor in accounting: innovation with robotic process automation. Int J Account Inform Syst 35:100–131. https://doi.org/10.1016/j.accinf.2019.100431
Li L, Su F, Zhang W et al (2018) Digital transformation by SME entrepreneurs: a capability perspective. Inf Syst J 28(6):1129–1157. https://doi.org/10.1111/isj.12153
Li WQ, Chien FS, Ngo QT, Nguyen TD et al (2021) Vertical financial disparity, energy prices and emission reduction: empirical insights from Pakistan. J Environ Manage 294:112946. https://doi.org/10.1016/j.jenvman.2021.112946
Li J, Chen L, Chen Y, He J (2022) Digital economy, technological innovation, and green economic efficiency-empirical evidence from 277 cities in China. Manage Decis Econ 43(3):616–629. https://doi.org/10.1002/mde.3406
Li M, Peng MYP, Nazar R et al (2022) How does energy efficiency mitigate carbon emissions without reducing economic growth in post COVID-19 era. Front Energ Res 10(4):832189. https://doi.org/10.3389/fenrg.2022.832189
Lin WT, Shao BBM (2006) The business value of information technology and inputs substitution: the productivity paradox revisited. Decis Support Syst 42(2):493–507. https://doi.org/10.1016/j.dss.2005.10.011
Liu MY, Li CY, Wang S (2023) Digital transformation, risk-taking, and innovation: evidence from data on listed enterprises in China. J Innov Knowl 8:100332. https://doi.org/10.1016/j.jik.2023.100332
Luo S, Yimam N, Li Y et al (2023) Digitalization and sustainable development: how could digital economy development improve green innovation in China? Bus Strateg Environ 32(4):1847–1871. https://doi.org/10.1002/bse.3223
Ma D, Zhu Q (2022) Innovation in emerging economies: research on the digital economy driving high-quality green development. J Bus Res 45:801–813. https://doi.org/10.1016/j.jbusres.2022.03.041
Moulton BR (1999) GDP and the digital economy: keeping up with the changes. Understanding Digit Econ Data 4(5):34–48. https://doi.org/10.7551/mitpress/6986.003.0004
Nambisan S, Wright M, Feldman M (2019) The digital transformation of innovation and entrepreneurship: progress, challenges and key themes. Res Policy 48(8):1–9. https://doi.org/10.1016/j.respol.2019.03.018
Nasir MH, Wen J, Nassani AA et al (2022) Energy security and energy poverty in emerging economies: a step towards sustainable energy efficiency. Front Energy Res 10:834614. https://doi.org/10.3389/fenrg.2022.834614
Pagani M, Pardo C (2017) The impact of digital technology on relationships in a business network. Ind Mark Manage 8:185–192. https://doi.org/10.1016/j.indmarman.2017.08.009
Peng C, Jia XY, Zou Y (2022) Does digitalization drive corporate green transformation? Based on evidence from Chinese listed companies. Front Environ Sci 10:963878. https://doi.org/10.3389/fenvs.2022.963878
Porter ME, Linder CL (1995) Toward a new conception of the environmental-competitiveness relationship. J Econ Perspect 9:97–118. https://doi.org/10.1257/jep.9.4.97
Pradhan P, Arvin B, Nair M et al (2020) Sustainable economic growth in the European Union: the role of ICT, venture capital, and innovation. Rev Financ Econ 38(1):34–62. https://doi.org/10.1002/rfe.1064
Ren S, Hao Y, Xu L et al (2021) Digitalization and energy: how does internet development affect China’s energy consumption? Energy Econ 98:105220. https://doi.org/10.1016/j.eneco.2021.105220
Shen Y, Ur Rahman S, Hafiza NS et al (2024) Does green investment affect environment pollution: evidence from asymmetric ARDL approach? PLoS ONE 19(4):e0292260. https://doi.org/10.1371/journal.pone.0292260
Sibt-e-Ali M, Xia XQ, Javed K, et al (2024) Greening the future: assessing the influence of technological innovation, energy transition and financial globalization on ecological footprint in selected emerging countries. Environ Dev Sustain (5): https://doi.org/10.1007/s10668-024-05076-5
Sun Y, Sun H (2021) Green innovation strategy and ambidextrous green innovation: the mediating effects of green supply chain integration. Sustainability 13(9):4876–4895. https://doi.org/10.3390/su13094876
Takalo SK, Tooranloo HS (2021) Green innovation: a systematic literature review. J Clean Prod 279(10):122474. https://doi.org/10.1016/j.jclepro.2020.122474
Tapscott D (1996) The digital economy: promise and peril in the age of networked intelligence. New York, McGraw-Hill. https://doi.org/10.5465/ame.1996.19198671
Teece DJ (2018) Profiting from Innovation in the digital economy: enabling technologies, standards, and licensing models in the wireless world. Res Policy 47(8):1367–1387. https://doi.org/10.1016/j.respol.2017.01.015
UNEP (2008) Green economy indicators-brief paper. France, Paris
Wang HR, Cui HR, Zhao QZ (2021a) Effect of green technology innovation on green total factor productivity in China: Evidence from spatial Durbin model analysis. J Clean Prod 288(3):125624. https://doi.org/10.1016/j.jclepro.2020.125624
Wang L, Chen YY, Ramsey TS (2021b) Will researching digital technology really empower green development? Technol Soc 66(8):101638. https://doi.org/10.1016/j.techsoc.2021.101638
Wang CG, Liu TS, Zhu Y et al (2022a) Digital economy, environmental regulation and corporate green technology innovation: evidence from China. Int J Environ Res Public Health 19(21):14084. https://doi.org/10.3390/ijerph192114084
Wang SG, Sun L, Iqbal S (2022b) Green financing role on renewable energy dependence and energy transition in E7 economies. Renew Energy 200:1561–1572. https://doi.org/10.1016/j.renene.2022.10.067
Wen HW, Zhong QM, Lee CC (2022) Digitalization, competition strategy and corporate innovation: evidence from Chinese manufacturing listed companies. Int Rev Financ Anal 82:102166. https://doi.org/10.1016/j.irfa.2022.102166
Xu S, Yang CY, Huang ZH, Failler P (2022) Interaction between digital economy and environmental pollution: new evidence from a spatial perspective. Int J Environ Res Public Health 19(9):5074. https://doi.org/10.3390/ijerph19095074
Zeng HL, Chau KY, Waqas M (2023) Does green finance and renewable energy promote tourism for sustainable development: empirical evidence from China. Renew Energy 207(5):660–671. https://doi.org/10.1016/j.renene.2023.03.032
Zhang C (2020) Clans, entrepreneurship, and development of the private sector in China. J Comp Econ 48(1):100–123. https://doi.org/10.1016/j.jce.2019.08.008
Zhang HY, Dong SZ (2023) Digital transformation and firms’ total factor productivity: the role of Internal control quality. Financ Res Lett 57:104231. https://doi.org/10.1016/j.frl.2023.104231
Zhang Z, Fu WK, Ma L (2022) The impact of digital economy on green development in China. Frontier in Environmental Science 10:991278. https://doi.org/10.3389/fenvs.2022.991278
Zhang WW, Zhang SQ, Bo L, Haque M, Liu ER (2023) Does China’s regional digital economy promote the development of a green economy? Sustainability 15(2):1564. https://doi.org/10.3390/su15021564
Zhao CY, Liu ZQ, Yan XF (2023a) Does the digital economy increase green TFP in cities? Int J Environ Res Public Health 20(2):1442. https://doi.org/10.3390/ijerph20021442
Zhao JG, Rahman SU, Afshan S et al (2023b) Green investment, institutional quality, and environmental performance: evidence from G-7 countries using panel NARDL approach. Environ Sci Pollut Res 30:100845–100860. https://doi.org/10.1007/s11356-023-29332-9
Funding
This work was supported by the Project of National Social Science Foundation of China (No. 19BJL061).
Author information
Authors and Affiliations
Contributions
Xiaoqian Meng: formal analysis, data curation, methodology, and writing—original draft. Chuanqing Wu: conceptualization, funding acquisition, and writing—review and editing. All authors have read and agreed to the published version of the manuscript.
Corresponding author
Ethics declarations
Ethical approval
There are no human subjects or animals in this article and ethical approval is not applicable.
Consent to participate
There are no human subjects or animals in this article, and consent to participate is not applicable.
Consent for publication
Not applicable (this manuscript does not include any individual person’s information).
Conflicts of interest
The authors declare no competing interests.
Additional information
Responsible Editor: Eyup Dogan
Publisher's note
Springer Nature remains neutral with regard to jurisdictional claims in published maps and institutional affiliations.
Rights and permissions
Springer Nature or its licensor (e.g. a society or other partner) holds exclusive rights to this article under a publishing agreement with the author(s) or other rightsholder(s); author self-archiving of the accepted manuscript version of this article is solely governed by the terms of such publishing agreement and applicable law.
About this article
Cite this article
Meng, X., Wu, C. Empirical evidence on digitization enabling the transition to a green economy in China. Environ Sci Pollut Res 31, 51790–51805 (2024). https://doi.org/10.1007/s11356-024-34613-y
Received:
Accepted:
Published:
Issue Date:
DOI: https://doi.org/10.1007/s11356-024-34613-y