Abstract
This study examined the sensitivity of reference crop evapotranspiration (ET0) to climatic variables in a humid region in Iran. ET0 was estimated using the FAO-56 Penman–Monteith (PMF-56), Blaney–Criddle (BC), and Hargreaves–Samani (HG) methods. Sensitivity analysis was performed by two distinct methods which were (i) changing the value of a certain climatic parameter in a range between ± 20% of its long-term mean with an increment of 5%, and calculating the percentage of change in ET0, while the other parameter values were kept constant; and (ii) calculating the sensitivity coefficients (SCs) for each of the climatic variables. For each of the climatic parameters, the Iso-SC maps were plotted using the Arc-GIS software. Results indicated that the most sensitive parameter for ET0 was the maximum air temperature (Tmax) by PMF-56 and HG methods. Increasing Tmax up to 20% led to an increase in ET0 between 8.5 and 15%, at the selected stations by PMF-56. In contrast, the less sensitive parameter for ET0 was the minimum air temperature (Tmin) for PMF-56 and Tmean for HG. For PMF-56, increasing the minimum relative humidity (RHmin) to 20% led to a decrease in ET0 in the range between 0.5 and 5%. The highest values of SC in the cases of Tmax and Tmin were found to be equal to 0.8 and 0.53, respectively. Similarly, the SC in the case of RHmin varied between − 0.29 and − 0.0038. This range for wind speed was between 0.06 and 0.22 and in the case of sunshine hours it was between 0.272 and 0.385. These findings would be useful in the scientific management of water resources in the region.
Similar content being viewed by others
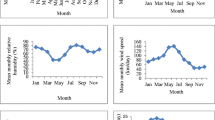
Explore related subjects
Discover the latest articles, news and stories from top researchers in related subjects.Avoid common mistakes on your manuscript.
Introduction
Evapotranspiration is a key component of the hydrological cycle (Ashrafzadeh et al. 2018). Accurate estimation of reference crop evapotranspiration (ET0) is a prerequisite for the prediction of crop evapotranspiration (ETc) or crop water use. Practically, ETc is estimated by multiplying ET0 with crop coefficient Kc. Due to the difficulties in the direct measurement of water flux through crops (Annandale and Stockle 1994; Kite and Droogers 2000; Xie and Zhu 2013), ETc is calculated by means of ET0 (ETc = ET0 × Kc). Indeed, direct measurement of ET0 is time-consuming, cumbersome, and expensive. Therefore, ET0 is estimated using the empirical or the combination (energy and mass transfer) methods. The best example of the combination method is the Penman method (Penman 1948). Examples of popular empirical methods are those proposed by Blaney and Criddle (1950), Thornthwaite (1948), and Jensen and Haise (196e) which require only daily air temperature and radiation data. More complex, physically-based models require daily data for air temperature, solar radiation, vegetative canopy, wind speed, and relative humidity discussed by Allen et al. (1998), such as FAO56-PM model (PMF-56). This model combines the Penman method with canopy surface characteristics proposed by Monteith (1965) and the combined method is called the Penman–Monteith method.
The PMF-56 has been a popular model (Rana and Katerji 2000; Kite and Droogers 2000; Goyal 2004; Dinpashoh 2006; Jhajharia et al. 2014; Nouri et al. 2017) and is known to be the most suitable model in different climates (Allen et al. 1998; Xu et al. 2006). This model employs some local geographic parameters (such as latitude, longitude, and altitude of the site) and different meteorological parameters, such as maximum air temperature (Tmax), minimum air temperature (Tmin), wind speed (U), net solar radiation (Rn), and vapor pressure deficit (VPD) for the ET0 estimation. Since the net Rn measurements are not readily available, this parameter can be estimated by employing the actual sunshine hours (n). Moreover, VPD can be estimated by using the recorded values of maximum relative humidity (Rhmax) and minimum relative humidity (Rhmin). It is important to evaluate the sensitivity of ET0 model to different meteorological parameters, because at a certain site, meteorological parameters change at different rates due to climate change.
On the other hand, many other empirical models have been calibrated in many areas using the PMF-56 as a benchmark (Almorox et al. 2018; Farzanpour et al. 2019). Furthermore, available meteorological data are usually affected by errors that originate from various sources, such as sensor calibration, adjustment of measurement sets, and reading and/or recording data. Sensitivity analysis assesses how a certain change in one climatic parameter influences the model output (McCuen 1974). The most widely used procedure for sensitivity analysis is to study the fluctuations of model output due to the variability of one parameter, while other parameters are kept fixed (Kannan et al. 2007; Sharifi and Dinpashoh 2014; Goyal 2004).
In recent years, several studies have been conducted on the estimated ET0 and the sensitivity analysis of ET0 in different regions. Saxton (1975) analyzed the sensitivity of ET0 obtained by the Penman model (Penman 1948) and showed that the ET0 estimation was most sensitive to the sunshine duration. Ley et al. (1994) analyzed the sensitivity of Penman–Wright (Wright 1982) alfalfa reference ET0 model to different climatic variables and showed that the model output was highly sensitive to Tmax and Tmin. Gong et al. (2006) did a sensitivity analysis of the PMF-56 model to different meteorological variables in the Changjiang basin, China. Estévez et al. (2009) performed sensitivity analysis of the Penman equation in Spain and found that ET0 was more sensitive to sunshine duration. Liu et al. (2010) investigated the sensitivity of ET0 to different climatic variables in the Yellow River basin in China and found that ET0 was highly sensitive to sunshine duration. Gao et al. (2016) assessed the sensitivity of ET0 to different climatic parameters during the growing season and showed that ET0 was more sensitive to solar radiation and less sensitive to Tmean. Liu et al. (2016) investigated the sensitivity of ET0 to climatic variables in southeastern China, using data from 57 weather stations and found that ET0 was more sensitive to wind speed and Tmean. The impact of changes in climatic parameters on ET0 in arid and semi-arid regions in Iran was investigated by Eslamian et al. (2011) who reported that the PMF-56 model was sensitive to air temperature and relative humidity. Sharifi and Dinpashoh (2014) examined the sensitivity of PMF-56 model to climatic variables in Iran and showed that ET0 was more sensitive to Tmean, but was less sensitive to actual vapor pressure (ea). They used the methodology discussed by Goyal (2004) in which a certain meteorological parameter was changed in the range of − 20% to + 20% with a 5% step and by fixing the values of other meteorological parameters and finding the change in ET0 percentage. They did not use the sensitivity coefficient in their study, which is the main difference with the present study. Moreover, stations used by Sharifi and Dinpashoh (2014) were selected from arid and semi-arid climates (except a station namely Anzali) but in the present study all the stations used to be from the humid region (i.e., Caspian Sea shoreline) which is the main second difference with the work of Sharifi and Dinpashoh (2014). Nouri et al. (2017) reported that ET0 in arid regions of Iran was more sensitive to solar radiation and wind speed. Shiri (2017) investigated the performances of different ET0 models using the recorded and estimated meteorological parameters and compared the results with the corresponding gene expression programming (GEP) model (based on the same input parameters that employed in ET0 models) at hyper-arid regions. The results showed that the GEP models outperform the corresponding empirical and semi-empirical models. Almorox et al. (2018) assessed the Penman–Monteith Temperature (PMT) approach for the estimation of monthly ET0. The performance of the PMT method is evaluated and compared with the Hargreaves–Samani (HG) equation using the measured long-term monthly data of the FAO global climatic dataset New LocClim. The results showed the performance of PMT method was better comparing the HG method. Farzanpour et al. (2019) comprised 20 reference evapotranspiration equations in a semi-arid region of Iran. They used the two data management scenarios, namely, local and cross-station scenarios in their study for the purpose of calibrating the applied equations against the standard PMF-56 model. The obtained results revealed that the cross-station calibration might be a good alternative for local calibration of the ET0 models. Aydın et al. (2019) investigated the sensitivity of reference evapotranspiration and soil evaporation to climate change in the Eastern Mediterranean Region in Turkey for a baseline period (1994–2003). Daily reference evapotranspiration was computed using the PMF-56 model and results showed that reference evapotranspiration was more sensitive to the net radiation in all seasons.
Guilan province is one of the main agricultural production areas of Iran, which produces rice and other cereals that meet an important portion of food demand in the country. This region, located on the southern shore of the Caspian Sea, has a humid climate. To the best of our knowledge, the sensitivity analysis of ET0 by PMF-56, Blaney–Criddle (BC), and HG models to climatic variables has not yet been carried out in humid areas of Iran. Therefore, the objectives of the present study were to (1) estimate ET0 using the PMF-56, BC, and HG models in the humid region of Iran, and (2) assess the sensitivity of PMF-56, BC, and HG models to climatic parameters in this region.
Materials and methods
Study area
The study area is the Guilan province with an area of 14,044 km2 located between 36° 33′ E and 38° 27′ E latitudes and 48° 32′ N and 50° 36′ N longitudes. The province is limited from north to the Caspian Sea and Republic of Azerbaijan, from the west and southeast to the Alborz Mountains, and from the east to the Mazandaran Province. The mountains of this province are covered by dense tall forest trees (Isazadeh et al. 2017; Dinpashoh et al. 2019). Figure 1 shows the location of the study area. The climate of this area is humid with hot summers and mild winters. The mean annual rainfall in this region varies from 1000 to 1850 mm and the relative air humidity is often high (more than 85%) throughout the year due to the vicinity of the Caspian Sea. In the present study, five weather stations, namely Anzali, Astara, Rasht, Lahijan, and Manjil, were selected and their climatic data were gathered from the Meteorological Organization of Guilan Province. The values of Tmax, Tmin, Tmean, Rhmax, Rhmin, wind speed, and actual sunshine duration in the period of 2005–2014 were used to estimate ET0 by the PMF-56, BC, and HG models (Table 1).
Methods
FAO 56 Penman–Monteith Method
In the present study, the FAO-56 Penman–Monteith (PMF-56) method (Allen et al. 1998) was used to estimate monthly reference crop evapotranspiration:
where ET0 is the reference crop evapotranspiration (mm day−1), ∆ is the slope of the saturation vapor pressure curve (kPa °C−1), U2 is the wind speed at a 2-m height (m s−1), Rn is the net radiation at the crop surface (MJ m−2 day−1), G is the soil heat flux (MJ m−2 day−1), γ is the psychometric constant (kPa °C−1) which is assumed to be 0.665 × 10−3, T is the mean daily air temperature at 2-m height (°C), es is the saturation vapor pressure, ea is the actual vapor pressure (kPa), and (es − ea) is the saturation vapor pressure deficit (kPa). More details of the model are presented in Allen et al. (1998).
Blaney–Criddle method
The Blaney–Criddle method is one of the earliest methods of the ET0 estimation methods.
The modified version of this model is expressed by the following equation (Blaney and Criddle 1950).
where the coefficients and b depend on the relative humidity of the air, the ratio of actual hours of sunshine to the maximum possible sunshine hours, and the wind speed. Moreover, the Tmean is the mean monthly temperature (°C), and p is the coefficient of the percentage of annual sunshine hours in a given month that was extracted from the relevant table (Blaney and Criddle 1950).
Hargreaves–Samani method
The Hargreaves–Samani method for calculating evapotranspiration only requires the maximum, minimum, and mean of daily temperatures, which can be calculated in a 24-h, or weekly, or 10-day, or monthly time scales. By this method, the following equation was used in this study.
In that method, the\( \mathrm{term}\ \sqrt{T_{\mathrm{max}}-{T}_{\mathrm{min}}} \) is the difference between the minimum air temperature and the maximum air temperature, Tmean is the mean temperature in °C and the Ra is extraterrestrial solar radiation (Hargreaves and Samani 1985).
Sensitivity analysis
In the present study, the sensitivity of PMF-56, BC, and HG ET0 models to climatic variables was analyzed by two distinct approaches. The first method is based on changing the climatic parameter value by a certain percentage and calculating the corresponding percentage change in ET0, while keeping the other parameters constant at their long-term mean values. This was accomplished for five selected stations in the study area by changing the variables from − 20 to + 20% with ± 5% increments that were deemed to influence ET0. These variables included six climatic parameters: Tmax, Tmin, Tmean, Rhmax, Rhmin, wind speed, and sunshine hours. In the case of Rhmax, the range of change was selected to be between − 20% and 0 with a + 5% increase, because at most of the stations the amount of Rhmax exceeded 100% after addition of some percentages to its mean value that was physically meaningless.
The second approach was based on the calculation of the sensitivity coefficients (SCs) defined as the ratio of the variation of ET0 rate to the variation of climatic variable rate (McCuen 1974; Liu et al. 2012). The PMF-56 is a multivariable model and many consecutive partial derivatives are needed to carry out the sensitivity analysis. This process is cumbersome and is not easy to use. Furthermore, different climatic variables have different scales and dimensions. Therefore, converting the variables to their non-dimensional form can simplify sensitivity analysis (McCuen 1974; Beven 1979). The sensitivity coefficient was first used by McCuen (1974) and Saxton (1975) and has since been widely applied (Gong et al. 2006; Estévez et al. 2009; Huo et al. 2013; Nouri et al. 2017). The relative SC for the ith variable (vi) is given by
where SCi is the sensitivity coefficient for the ith climatic variable and vi denotes the ith variable affecting ET0. In this study, Eq. (2) was used to assess the sensitivity of ET0 to the selected climatic variables. A positive (negative) value of SC indicates the increase (decrease) of ET0 when the given input climatic variable increases and other variables are kept constant at their mean values. For instance, a value of 0.1 for SC implies that an increase of 10% of the value of a certain climatic variable would lead to an increase in the amount of ET0 percentage equal to 1%.
Results and discussion
Monthly patterns of climatic parameters for a representative station, namely Anzali, are shown in Fig. 2. It is noted that although similar plots were prepared for other stations, for the sake of brevity, all of them are not shown here. For all the stations, the lowest values of Tmax and Tmin belonged to the cold month. However, air temperature rose to its peak level (for both Tmin and Tmax) in July. For all the stations, the other climatic parameters reached their maximum in August. The difference of Tmean between the hottest and coldest months was about 19.21 (°C) at station Anzali. This value was about 19.37, 20.8, 18.62, and 20.17 (°C) at stations Rasht (February, August), Manjil (January, August), Lahijan (January, August), and Astara (February, July), respectively. The Rh parameter reached its maximum and minimum values in cold and hot months, respectively. The overall averages of Rhmax and Rhmin through the time period were found to vary from 63 to 95% (Anzali), 57 to 89% (Lahijan), 51 to 95% (Astara), 38 to 87% (Manjil), and 57 to 98% (Rasht), respectively. It is seen from Fig. 2 that the curvatures of Rhmax, Rhmin, and Rhmean were nearly similar to each other at a certain station. In the area, the large values of Rh led to decreased crop water requirements.
At all of the stations (except Manjil), the maximum wind speed was experienced in March. At Manjil, which usually experiences high winds throughout the year, the highest wind speed occurred in July. At this station, many turbine plants have been installed for harvesting wind energy. The maximum amount of wind speed was about 7.4 (at Anzali), 2 (at Lahijan), 6 (at Astara), 16 (at Manjil), and 5 (at Rasht) knots, respectively. As can be seen from Fig. 2, there was no apparent similarity between the patterns of monthly sunshine hours and air temperature. The minimum amount of sunshine hours belonged to February for all the stations (except Manjil). At Manjil, the minimum value of this parameter occurred in December. As the length of day increased, the cumulative sunshine hours increased as well. The range of monthly sunshine hours in the area varied from 231 h (at the station Lahijan) to about 497 h at Manjil.
At five selected stations, the maximum value of Tmean in the warmest month of a year belonged to Manjil (equal to 28.01 °C) and the lowest value of Tmean in the coldest month belonged to Astara (equal to 5.95 °C). Therefore, the overall average of the maximum difference of Tmean between the coldest and hottest months was equal to 20.8 °C, which was experienced at Manjil. This value for other stations was less than 20.8 and more than 18.62 °C. The pattern of monthly Rh at two stations (Lahijan and Manjil) was seen to be quite different from that at the other three stations. At stations Lahijan and Manjil, the range of Rh fluctuation was negligible from month to month. However, this was not true in the case of other stations. It seems that the reason was the geographic location of these two sites, which are located in the foothills of the Alborz Mountains. These chain mountains act as an obstacle against the flowing air streams from north to south.
On the other hand, rapid urbanization near the station Rasht increases the surface roughness that reduces wind speed to some degree. The other three stations are located near the Caspian Sea. As stated before, among all the five stations, Manjil has a higher potential in capturing wind energy. The station Lahijan is known as the calm site, at which wind speed is often less than 2 knots. The sunshine duration is relatively high at station Manjil compared with other stations. The monthly sunshine duration exceeded 500 h in July, which is about two times greater than that at the other stations. Probably the high altitude of this site led to this result. Figure 3 shows the trend lines of the annual ET0 time series of all selected stations. As can be seen from Fig. 3a, all the stations (except Manjil) have an increasing trend for annual ET0. At Manjil, a decreasing trend was experienced for annual ET0. The reason for this can be attributed to the increasing trend in Rh, and the decreasing trend in both wind speed and sunshine hours. The steepest slope of annual ET0 trend line belonged to the coastal station, namely Anzali. Figure 3b illustrates that all the stations have a positive trend. It can be seen at Fig. 3b that the Astara station has a significant positive trend. And the Rasht station has a modest rise trend. Also, these positive trends for all stations can be seen from Fig. 3c. At Fig. 3c, Astara, Anzali, and Rasht stations have the most positive significance trends, respectively. In total, Fig. 3b and c are different from Fig. 3a. Figure 3c illustrates significant rise trends for all stations because this figure related to HG models. The HG model includes maximum, minimum, and mean temperature. Also, Fig. 3b–d depended on mean temperature. So we can see rise trends for these figures. In contrast to the last figures, Fig. 3a is related to the PMF-56 model. This model applied a wide range of parameters as mentioned above. So we can see fluctuate trends in these figures.
Figure 4 shows the trends in seasonal ET0 at station Anzali. It can be seen that in all seasons, (except autumn) increasing trends dominated for all of the tree models. During the fall, in the period of 2005–2014, there was a decreasing trend in ET0. However, the steepest trend belonged to the spring ET0 at the port station Anzali for all figures. The negative significance trend belonged to the HG model (Fig. 4c). The results illustrate the temperature parameters did not cause a reverse change in the seasonal evapotranspiration (Fig. 4b, c). So the temperature parameter has an important role in seasonal evapotranspiration in this station. It can be seen in Fig. 4a to c that the trend slope goes up steeply. So the PMF-56 model because of other parameters illustrated the modest rise trend slope for the evapotranspiration.
Figure 5 shows the seasonal ET0 trends at Astara station. It can be seen that except for the fall season, the ET0 time series in other seasons had increasing trends. The spring ET0 showed the steepest upward trend at Astara. No significant trend was observed in the fall ET0 time series at station Astara except in spring for Fig. 5c. The steepest slope belonged to the HG model for spring season and the most negative trend belonged again to the HG model for fall season. So, same as Fig. 4, the temperature parameter has an important role in this station.
Figure 6 shows the seasonal ET0 trends in Lahijan. There was an upward trend for the spring ET0 time series. However, in the case of the other three seasons, ET0 trends were not statistically significant. At the mentioned site, moderate upward trend line slopes existed for three parameter (sunshine duration, average temperature, and wind speed) time series, which led to the increasing spring ET0. Trends in other three season ET0 were not statistically significant. In Fig. 6a and c, it can be seen the summer season has negative slope trends. In Fig. 6b, the summer season has a moderate positive trend. Same as other stations, the fall season has negative slope trends for evapotranspiration. It can be seen the HG model has the most significant negative and positive trend slope for fall and spring seasons, respectively. The results illustrate the maximum and minimum temperature increase the trend slope.
Figure 7 depicts the seasonal trends in ET0 at station Manjil. It can be seen from this figure that in all four seasons, downward trends in ET0 were observed at station Manjil. The steepest negative trend line slope belonged to summer followed by spring. Such decreasing trends in seasonal ET0 led to a downward trend in annual ET0 at Manjil (see Fig. 3a). Such decreasing trends in wind speed time series at the station Manjil are shown in Fig. 8. As it is obvious from Fig. 8, the mean annual wind speed witnessed a decrease through the time period. More precisely, the mean annual wind speed was close to 14 (m/s) in the beginning of time period (year 2005), whereas it declined to nearly 10 (m/s) at the end of the time period (i.e., the year 2014). Inspection of trends in different climatic time series in summer at Manjil showed that not only the Rh time series had an increasing trend but wind speed and sunshine hours observations (in summer) had decreasing trends. Such combined variation in the climatic parameters caused the summer ET0 time series to exhibit a negative trend. It can be seen in Fig. 7b all seasons have a positive trend except fall. Also, Fig. 7c illustrates negative trends for summer and fall seasons.
Figure 9 shows seasonal ET0 trends at station Rasht. This station is the largest city in the Guilan province. As can be seen, the fall ET0 time series showed a decreasing trend. A similar result was observed at Anzali as mentioned before. On the other hand, the steepest upward trend in seasonal ET0 belonged to spring. At this station, no statistically significant trend was detected for winter and summer ET0 time series. It can be seen in Fig. 9a and c the winter season has a decreasing trend. Also, in Fig. 9b, the summer has a decreasing trend because the BC model used just mean temperature.
In addition to annual and seasonal ET0, trend analysis was also carried out for monthly ET0 time series. However, for the sake of brevity, the results are highlighted only for the hot month, in which crop water requirement was high. At some of the stations, the hot month was July, and at some others it was August. Figure 10 shows trends in ET0 for the selected stations in the hot month. As can be seen, two out of the five stations (namely Anzali and Astara) exhibited upward ET0 trends. The slope of the trend line at Astara was slightly more than that at Anzali. However, at two stations (namely Manjil and Rasht), ET0 had decreased. The steepest downward trend was observed at Manjil. The reason can be attributed to the large distance between the station location and the Caspian Sea. In addition, as mentioned before, the wind speed at Manjil was considerably higher than that at other stations. It can be seen in Fig. 10c all the stations have increasing trends. In Fig. 10b, just Rasht station has a decreasing trend.
Figure 11 shows the relative change in ET0 due to the relative change in a meteorological parameter. It can be seen in Fig. 11a, at Anzali station, in response to the change in Tmin by 20%, ET0 varied by about 2.5%. This value was about 3.5% for the wind speed and about 7% and 9% for sunshine hours and Tmax, respectively. Irmak et al. (2006) noted that on an annual average, a 1 °C increase in Tmax resulted in an increase of ET0 of the stations (in the USA) between 0.06 and 0.11 mm/day. At the four stations (Anzali, Astara, Manjil, and Rasht), ET0 had the lowest sensitivity to Tmin followed by wind speed, Rhmax, sunshine hours, and Tmax, respectively. But at Lahijan, Rhmin had a reverse relationship with ET0. Wind speed, Tmin, Tmax, and sunshine hours were recognized, respectively, as the most effective parameters for ET0. Therefore, it can be concluded that the most important parameter for ET0 at Lahijan was sunshine hours.
At the other four stations, the most sensitive parameter was Tmax (Fig. 11a). Among all the selected stations, Manjil gained the first rank by 17% change in ET0 in response to a 20% change in Tmax. Following Manjil, Astara and Rasht stations were in the second and third ranks, respectively (Fig. 11a). The least amount of changes in ET0 among the stations belonged to Lahijan station, at which a 20% change in Tmax led to a 6% change in ET0. Irmak et al. (2006) found that during the summer months, ET0 was most sensitive to solar radiation (Rs) at humid locations of the USA (Fort Pierce and Rockport). At Santa Barbara having a Mediterranean-type climate in the USA, the sensitivity of ET0 to wind speed was reported to be minimal during the summer months. It can be seen in Fig. 11b the BC model used just one parameter namely mean temperature. Among all the selected stations, Manjil gained the first rank by 12% change in ET0 in response to a 20% change in Tmean. At the Fig. 11-c, it can be seen at the Anzali station, in response to the change in Tmax by 20%, ET0 varied about 63%. This value was about 12% for Tmean and 48% for Tmin. at Fig. 11-c, ET0 had the lowest sensitivity to Tmean and had the most sensitivity to Tmax (fig. 11-c) for five stations (Anzali, Astara, Lahijan, Manjil and Rasht).
Table 2 shows the correlation coefficients between monthly ET0 and two local parameters, namely, latitude and altitude. It can be seen that latitude had an inverse relationship with ET0 in all months (Table 2a and c). For latitude, all of the correlation coefficients were negative (Table 2a and c). At the Table 2b, July, August, and October have a significant correlation with latitude values. This implied that as the latitude of the sites increased, ET0 decreased. On the other hand, in all the months, the correlation coefficient obtained between altitude and ET0 was positive. This implied that as the elevation of a station increased, ET0 increased as well. It seems that there were strong winds existing at the stations with higher altitudes. On the other hand, such stations had relatively less Rh compared with the stations with lower elevations. Therefore, the combined effects of these changes led to an increasing ET0 with the increasing altitude of the sites.
Figure 12 shows the SC changes versus the altitudes of stations in Guilan Province. It can be seen in Fig. 12a, the SC for three parameters (Tmax, Tmin, and wind speed) had a direct upward relationship. However, in the case of sunshine hours, Rhmax, and Rhmin, the values of SC decreased with the increasing altitude of the stations. The steepest slope of the fitted upward line belonged to Tmin. However, the steepest slope of the mentioned lines belonged to the sunshine hours parameter. Also, it can be seen from Fig. 12b the SC for minimum and mean temperature had upward relationship. However, in the case of maximum temperature, the SC had downward relationship. It cannot be seen from Fig. 12 the BC model values, since the value of SC for BC model was equal to 1 (BC model has only one parameter, namely mean air temperature).
Figure 13a and c show the percent change of ET0 versus the percent change in Tmax in the months of August, October, and April. And Fig. 13b shows the percent change of ET0 versus the percent change in Tmean. It can be seen that station Manjil had the largest changes in ET0. However, these changes at Manjil were the highest in August and the lowest in April (Fig. 13a–c). Station Lahijan had the lowest changes in ET0 (%) with changes in Tmax among all the stations. At this station, August showed the maximum changes of ET0 among the other two months. It can be concluded that in warm months of a year (August and July), changes in ET0 versus change in Tmax for Fig. 13a and c and Tmean for Fig. 13b were more than those of other months.
The distribution of SC for different meteorological parameters across the study area is shown in Fig. 14. It can be seen that Tmax which had the lowest value of SC in the region (red color) is located in the eastern part of the province (Lahijan station) (Fig. 14a). However, the highest value of SC belonged to the southern part of the region. Station Manjil is located in this part of the study area. The SC of the Manjil station for Tmax was about 0.8 (for Lahijan this value was about 0.31). The lowest value of SC for Tmin belonged to the Caspian Sea shoreline. However, similar to SC for Tmax, the highest value of SC for Tmin was observed in the southern parts of the region. The highest value of SC for Tmin was about 0.53 at station Manjil, while the lowest value belonged to the Astara station (SC equal to 0.077).
In the case of Rhmin and wind speed, it can be seen that SC varied between − 0.294 and − 0.038 across the area. The lowest value of SC for Rhmin, which is shown by the red line, belonged to station Anzali. On the other hand, the highest value belonged to station Lahijan. The negative value of SC for Rhmin was observed for all the stations. This was due to an increase in the Rhmin time series, which led to a decrease in ET0. The spatial distribution of SC for wind speed is shown on the right-hand side of the middle panel of Fig. 14a. It can be seen that the SC for wind speed varied between 0.06 and 0.22 across the area. The lowest value of SC for wind speed belonged to the eastern part of the area, in which Lahijan had an SC value equal to 0.06. On the contrary, the highest values of SC for wind speed were seen in the southern and northern parts of the area in which the highest value was equal to 0.22 calculated for Anzali.
It can be seen from Fig. 14a that the SC for sunshine hours varied from 0.274 (at Manjil) to about 0.385 (at Lahijan). The pattern of SC for sunshine hours was very similar to that of Tmax. However, in the case of Rhmax, it can be seen from Fig. 14a that all values of SC were negative. Also, the pattern of SC for Rhmax was similar to that of Rhmin. It is noted that we used the inverse distance weighted (IDW) method to draw the spatial distribution of SC maps (Ha et al. 2011; Tao et al. 2015; Hodam et al. 2017). It can be seen that Tmax which had the lowest value of SC in the region (red color) is located in the eastern part of the province (Lahijan station) (Fig. 14b) and the highest value is located in Anzali station (blue color). The lowest value of SC for Tmin belonged to the Caspian Sea shoreline located in Anzali station, and the highest value located in Astara, Rasht, and Lahijan. The lowest value of SC for Tmean belonged to the eastern and western province located in Lahijan and Astara stations, and the highest value located in Manjil. It is not visible the BC model values, from Fig. 14, since the value of SC for this model was equal to 1 (BC model has only one parameter, namely mean air temperature).
Gong et al. (2006) reported that the less effective parameter for ET0 was wind speed in the Yangtze River basin. The results for Guilan province are in accord with the findings of Gong et al. (2006). However, the sensitivity coefficient was calculated for only 2 months (January and July) in the aforementioned work. In our study, we used such a coefficient for the months in which the value of the considered parameter reached its highest value. In the present study, increasing relative humidity (20%) showed a negative effect on ET0 which was between − 5.73 and − 0.66 at stations Anzali and Lahijan, respectively. These findings are consistent with those of Goyal (2004) which showed that a change of 20% in actual vapor pressure had a 7% change in ET0 in the arid zone of Rajasthan, India. On the other hand, Liu et al. (2016) showed that in the southwest of China, the relative humidity showed a higher sensitivity than did wind speed which are not considered the findings of our study. It is mentioned that Liu et al. (2016) used PMF-56 for ET0 calculation which is the same method as in our study. According to Liu et al. (2016), the coefficient for mean wind speed ranged from 0.12 to 0.43. However, the SC for wind speed was found between 0.06 and 0.22, which was less than that of Liu et al. (2016). Furthermore, Liu et al. (2016) found that SCs of the sites for relative humidity were negative and ranged from − 0.11 to − 0.003. These values for Guilan province were about − 0.038 to − 0.29, which were more sensitive than that of Liu et al. (2016).
In a similar work but for the arid region of Iran, Nouri et al. (2017) reported positive SCs for Tmean, radiation, and wind speed which are consistent with our findings for temperate climate area of Iran. On the other hand, Nouri et al. (2017) reported negative SC for Rh which is in agreement with our findings for Guilan province. Zuo et al. (2012) studied the sensitivity of ET0 to key meteorological parameters in Wei River basin, China, and showed that the sensitivity coefficient for maximum air temperature in summer in the upper region was lower than that in the middle-lower region. They also found that the relative humidity showed a strong negative sensitivity to ET0 which implies that an increase in relative humidity reduced ET0 to some degree. This is in agreement with our findings for north of Iran. Liu et al. (2010) conducted a study on the temporal trends of ET0 and its sensitivity to meteorological parameters in Yellow River basin in China. Their results showed that the increasing trend of ET0 was mainly due to the significant increase in air temperature as well as a decrease in Rh. Mosaedi et al. (2017) found an increasing trend of ET0 for two semi-arid stations, namely Mashhad and Tabriz, in Iran. On the other hand, they found decreasing trends in ET0 for stations Tehran, Anzali, and Shiraz.
Increasing trends which were found in our study were not consistent with those of Mosaedi et al. (2017). It seems that the difference in the results of the two studies is due to the use of different time periods (1963–2007 for the mentioned study instead of 2005 to 2014 in our study). It was found that Rh and wind speed were the two main variables having the inverse effect on ET0 at two stations, namely Tehran and Anzali, while the wind speed and air temperature were found to be the two responsible climatic parameters at Shiraz (Mosaedi et al. 2017). Also, according to Mosaedi et al. (2017), the main two parameters having a direct significant effect on ET0 at station Tabriz were Rh and sunshine hours. Zhang et al. (2010) reported that the vapor pressure deficit was the most effective parameter during cool months in the Shiyang River basin in northwest China. However, in the summertime, wind speed played an important role in the ET0 fluctuation. This does not coincide with our findings because at all five selected stations in Guilan province, Tmax was found to be the most effective parameter for ET0.
Sharifi and Dinpashoh (2014) reported that ET0 was most sensitive to Tmean at the six stations around Iran at the annual time scale, whereas ea was the less effective parameter for ET0 at most of the stations. In a recent study, Nouri et al. (2017) found that in arid climates of Iran, annual ET0 exhibited a greater sensitivity to Rs and wind speed. They reported that in arid southern coastal stations of Iran (in the vicinity of the Persian Gulf and Oman Sea), ET0 showed a higher sensitivity to Rh, which is not in agreement with the findings obtained here for humid coastal stations in northern Iran.
Conclusion
In the present study, three types of analysis were done for ET0 at five humid stations in north of Iran. These methods were trend analysis using non-parametric methods and sensitivity analysis (by three methods explained in the Materials and Methods section) for the ET0 time series in the north of Iran. ET0 was estimated using the standard PMf-56, BC, and HG methods. In this regard, the months with high values of certain climatic parameter were considered for sensitivity analysis. Each of the meteorological parameters was changed from − 20 to 20% with an increment step of 5% and then ET0 variations were computed using constant mean values of other climatic parameters. On the other method, the values of sensitivity coefficient SCs were calculated for each of the climatic parameters and for all of the six selected stations. Results showed that for all the stations (except Lahijan), ET0 showed the highest sensitivity to Tmax by PMF-56; Tmax for HG methods is sensitive parameter for all stations. The BC method just used one parameter namely Tmean. The duration of sunshine hours was found to be the second more sensitive parameter to ET0 at the selected stations by PMF-56. On the contrary, ET0 was found to be less sensitive to Tmin BY PMF-56 and wind speed in the Caspian Sea coastal stations and less sensitive to Tmen by HG method. Increasing water demands due to upward trends in Tmax at the most points in Iran and other countries require that the available freshwater should be wisely used for sustainable agriculture and food security in the study area and the whole of Iran. Proper use of water resources in Iran, particularly in areas with high agricultural activities, is urgently needed to mitigate the inverse effects of global warming. For further study, the methodology explained by McVicar et al. (2007) or Roderick et al. (2007) used for accounting for the contributions of change of different climatic variables to ET0 in different regions in Iran can be recommended. Also, it is recommended to analyze the trends in class A pan evaporation in the same area and compare the results with the findings of this study for future studies.
References
Ashrafzadeh A, Malik A, Jothiprakash A, Ghorbani MA, Biazar SM (2018) Estimation of daily pan evaporation using neural networks and meta-heuristic approaches. ISH Journal of Hydraulic Engineering:1-9. https://doi.org/10.1080/09715010.2018.1498754
Allen RG, Pereira LS, Raes D, Smith M (1998) Crop evapotranspiration-Guidelines for computing crop water requirements-FAO Irrigation and drainage paper 56. Fao, Rome, 300(9), D05109
Almorox J, Senatore A, Quej VH, Mendicino G (2018) Worldwide assessment of the Penman–Monteith temperature approach for the estimation of monthly reference evapotranspiration. Theor Appl Climatol 131(1-2):693–703
Annandale JG, Stockle CO (1994) Fluctuation of crop evapotranspiration coefficients with weather: a sensitivity analysis. Irrig Sci 15(1):1–7
Aydın M, Watanabe T, Kapur S (2019) Sensitivity of reference evapotranspiration and soil evaporation to climate change in the Eastern Mediterranean Region. In: Watanabe T., Kapur S., Aydın M., Kanber R., Akça E. (eds) Climate Change Impacts on Basin Agro-ecosystems. The Anthropocene: Politik—Economics—Society—Science, vol 18. Springer, Cham
Beven K (1979) A sensitivity analysis of the Penman-Monteith actual evapotranspiration estimates. J Hydrol 44(3-4):169–190. https://doi.org/10.1016/0022-1694(79)90130-6
Blaney HF, Criddle WD (1950) Determining water requirements in irrigated areas from climatological and irrigation data. USDA SCS-TP-96. U.S. Dept. of Agriculture, Washington, DC
Dinpashoh Y (2006) Study of reference crop evapotranspiration in IR of Iran. Agric Water Manag 84(1-2):123–129. https://doi.org/10.1016/j.agwat.2006.02.011
Dinpashoh Y, Singh VP, Biazar SM, Kavehkar S (2019) Impact of climate change on streamflow timing (case study: Guilan Province). Theor Appl Climatol, 1-12
Estévez J, Gavilán P, Berengena J (2009) Sensitivity analysis of a Penman–Monteith type equation to estimate reference evapotranspiration in southern Spain. Hydrol Process 23(23):3342–3353. https://doi.org/10.1002/hyp.7439
Farzanpour H, Shiri J, Sadraddini AA, Trajkovic S (2019) Global comparison of 20 reference evapotranspiration equations in a semi-arid region of Iran. Hydrol Res 50(1):282–300
Gao Z, He J, Dong K, Bian X, Li X (2016) Sensitivity study of reference crop evapotranspiration during growing season in the West Liao River basin, China. Theor Appl Climatol 124(3-4):865–881
Gong L, Xu CY, Chen D, Halldin S, Chen YD (2006) Sensitivity of the Penman–Monteith reference evapotranspiration to key climatic variables in the Changjiang (Yangtze River) basin. J Hydrol 329(3-4):620–629. https://doi.org/10.1016/j.jhydrol.2006.03.027
Goyal RK (2004) Sensitivity of evapotranspiration to global warming: a case study of arid zone of Rajasthan (India). Agric Water Manag 69(1):1–11. https://doi.org/10.1016/j.agwat.2004.03.014
Ha W, Gowda PH, Oommen T, Marek TH, Porter DO, Howell TA (2011) Spatial interpolation of daily reference evapotranspiration in the Texas High Plains. In World Environmental and Water Resources Congress 2011: Bearing Knowledge for Sustainability (pp. 2796-2804). https://doi.org/10.1061/41173(414)291
Hargreaves GH, Samani ZA (1985) Reference crop evapotranspiration from temperature. Appl Eng Agric 1(2):96–99. https://doi.org/10.13031/2013.26773
Hodam S, Sarkar S, Marak AG, Bandyopadhyay A, Bhadra A (2017) Spatial interpolation of reference evapotranspiration in India: comparison of IDW and Kriging Methods. Journal of the Institution of Engineers (India): Series A 98(4):511–524. https://doi.org/10.1007/s40030-017-0241-z
Huo Z, Dai X, Feng S, Kang S, Huang G (2013) Effect of climate change on reference evapotranspiration and aridity index in arid region of China. J Hydrol 492:24–34. https://doi.org/10.1016/j.jhydrol.2013.04.011
Irmak S, Payero JO, Martin DL, Irmak A, Howell TA (2006) Sensitivity analyses and sensitivity coefficients of standardized daily ASCE-Penman-Monteith equation. J Irrig Drain Eng 132(6):564–578. https://doi.org/10.1061/(ASCE)0733-9437(2006)132:6(564)
Isazadeh M, Biazar SM, Ashrafzadeh A (2017) Support vector machines and feed-forward neural networks for spatial modeling of groundwater qualitative parameters. Environ Earth Sci 76(17):610. https://doi.org/10.1007/s12665-017-6938-5
Jhajharia D, Dinpashoh Y, Kahya E, Choudhary R, Singh VP (2014) Trends in temperature over Godavari River basin in southern peninsular India. Int J Climatol 34:1369–1384
Kannan N, White SM, Worrall F, Whelan MJ (2007) Sensitivity analysis and identification of the best evapotranspiration and runoff options for hydrological modelling in SWAT-2000. J Hydrol 332(3-4):456–466. https://doi.org/10.1016/j.jhydrol.2006.08.001
Kite GW, Droogers P (2000) Comparing evapotranspiration estimates from satellites, hydrological models and field data. J Hydrol 229(1-2):3–18. https://doi.org/10.1016/S0022-1694(99)00195-X
Ley TW, Hill RW, Jensen DT (1994) Errors in Penman-Wright alfalfa reference evapotranspiration estimates: I. Model Sensitivity Analyses. Transactions of the ASAE 37(6):1853–1861. https://doi.org/10.13031/2013.28276
Liu Q, Yang Z, Cui B, Sun T (2010) The temporal trends of reference evapotranspiration and its sensitivity to key meteorological variables in the Yellow River Basin, China. Hydrol Process 24(15):2171–2181. https://doi.org/10.1002/hyp.7649
Liu C, Zhang D, Liu X, Zhao C (2012) Spatial and temporal change in the potential evapotranspiration sensitivity to meteorological factors in China (1960–2007). J Geogr Sci 22(1):3–14. https://doi.org/10.1007/s11442-012-0907-4
Liu T, Li L, Lai J, Liu C, Zhuang W (2016) Reference evapotranspiration change and its sensitivity to climate variables in southwest China. Theor Appl Climatol 125(3-4):499–508. https://doi.org/10.1007/s00704-015-1526-7
McCuen RH (1974) A sensitivity and error analysis of procedures used for estimating evapotranspiration. Water Resour Bull 10(3):486–497. https://doi.org/10.1111/j.1752-1688.1974.tb00590.x
McVicar TR, Van Niel TG, Li L, Hutchinson MF, Mu X, Liu Z (2007) Spatially distributing monthly reference evapotranspiration and pan evaporation considering topographic influences. J Hydrol 338(3-4):196–220
Monteith JL (1965) Evaporation and environment. 19th Symp., Society for Experimental Biology, University Press, Cambridge, U.K., 19, 205-234.
Mosaedi A, Sough MG, Sadeghi SH, Mooshakhian Y, Bannayan M (2017) Sensitivity analysis of monthly reference crop evapotranspiration trends in Iran: a qualitative approach. Theor Appl Climatol 128(3-4):857–873. https://doi.org/10.1007/s00704-016-1740-y
Nouri M, Homaee M, Bannayan M (2017) Quantitative trend, sensitivity and contribution analyses of reference evapotranspiration in some arid environments under climate change. Water Resour Manag 31(7):2207–2224. https://doi.org/10.1007/s11269-017-1638-1
Penman HL (1948) Natural evaporation from open water, bare soil and grass. Proc R Soc London A193, No. 1032, 120-145). DOI: https://doi.org/10.1098/rspa.1948.0037
Rana G, Katerji N (2000) Measurement and estimation of actual evapotranspiration in the field under Mediterranean climate: a review. Eur J Agron 13(2-3):125–153. https://doi.org/10.1016/S1161-0301(00)00070-8
Roderick ML, Rotstayn LD, Farquhar GD, Hobbins MT (2007) On the attribution of changing pan evaporation. Geophys Res Lett, 34(17)
Saxton KE (1975) Sensitivity analyses of the combination evapotranspiration equation. Agric Meteorol 15(3):343–353. https://doi.org/10.1016/0002-1571(75)90031-X
Sharifi A, Dinpashoh Y (2014) Sensitivity analysis of the Penman-Monteith reference crop evapotranspiration to climatic variables in Iran. Water Resour Manag 28(15):5465–5476. https://doi.org/10.1007/s11269-014-0813-x
Shiri J (2017) Evaluation of FAO56-PM, empirical, semi-empirical and gene expression programming approaches for estimating daily reference evapotranspiration in hyper-arid regions of Iran. Agric Water Manag 188:101–114
Tao XE, Chen H, Xu CY, Hou YK, Jie MX (2015) Analysis and prediction of reference evapotranspiration with climate change in Xiangjiang River Basin, China. Water Science and Engineering 8(4):273–281
Thornthwaite CW (1948) An approach toward a rational classification of climate. Geogr Rev 38:55–94
Wright JL (1982) New evapotranspiration crop coefficients. Proceedings of the American Society of Civil Engineers. J Irrig Drain Div 108(IR2):57–74
Xie H, Zhu X (2013) Reference evapotranspiration trends and their sensitivity to climatic change on the Tibetan Plateau (1970–2009). Hydrol Process 27(25):3685–3693. https://doi.org/10.1002/hyp.9487
Xu CY, Gong L, Jiang T, Chen D, Singh VP (2006) Analysis of spatial distribution and temporal trend of reference evapotranspiration and pan evaporation in Changjiang (Yangtze River) catchment. J Hydrol 327(1-2):81–93. https://doi.org/10.1016/j.jhydrol.2005.11.029
Zhang X, Kang S, Zhang L, Liu J (2010) Spatial variation of climatology monthly crop reference evapotranspiration and sensitivity coefficients in Shiyang River basin of northwest China. Agric Water Manag 97(10):1506–1516. https://doi.org/10.1016/j.agwat.2010.05.004
Zuo D, Xu Z, Yang H, Liu X (2012) Spatiotemporal variations and abrupt changes of potential evapotranspiration and its sensitivity to key meteorological variables in the Wei River basin, China. Hydrol Process 26(8):1149–1160. https://doi.org/10.1002/hyp.8206
Acknowledgments
The authors are thankful for the reviewers and editor of the Journal for their critical comments which improved the quality of the present paper, significantly.
Author information
Authors and Affiliations
Corresponding author
Additional information
Responsible editor: Philippe Garrigues
Publisher’s note
Springer Nature remains neutral with regard to jurisdictional claims in published maps and institutional affiliations.
Rights and permissions
About this article
Cite this article
Biazar, S.M., Dinpashoh, Y. & Singh, V.P. Sensitivity analysis of the reference crop evapotranspiration in a humid region. Environ Sci Pollut Res 26, 32517–32544 (2019). https://doi.org/10.1007/s11356-019-06419-w
Received:
Accepted:
Published:
Issue Date:
DOI: https://doi.org/10.1007/s11356-019-06419-w