Abstract
Introduction
Changes in skin phenotypic characteristics are based on skin tissue. The study of the metabolic changes in skin tissue can help understand the causes of skin diseases and identify effective therapeutic interventions.
Objectives
We aimed to establish and optimize a non-targeted skin metabolome extraction system for skin tissue metabolomics with high metabolite coverage, recovery, and reproducibility using gas chromatography/mass spectrometry.
Methods
The metabolites in skin tissues were extracted using eleven different extraction systems, which were designed using reagents with different polarities based on sequential solid-liquid extraction employing a two-step strategy and analyzed using gas chromatograph/mass spectrometry. The extraction efficiency of diverse solvents was evaluated by coefficient of variation (CV), multivariate analysis, metabolites coverage, and relative peak area analysis.
Results
We identified 119 metabolites and the metabolite profiles differed significantly between the eleven extraction systems. Metabolites with high abundances in the organic extraction systems, followed by aqueous extraction, were involved in the biosynthesis of unsaturated fatty acids, while metabolites with high abundances in the aqueous extraction systems, followed by organic extraction, were involved in amino sugar and nucleotide sugar metabolism, and glycerolipid metabolism. MeOH/chloroform-H2O and MeOH/H2O-chloroform were the extraction systems that yielded the highest number of metabolites, while MeOH/acetonitrile (ACN)-H2O and ACN/H2O-IPA exhibited superior metabolite recoveries.
Conclusion
Our results demonstrated that our research facilitates the selection of an appropriate metabolite extraction approach based on the experimental purpose for the metabolomics study of skin tissue.
Similar content being viewed by others
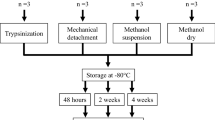
Avoid common mistakes on your manuscript.
1 Introduction
The skin is the largest organ in the human body, consisting of a fibroblast-dominated connective tissue dermis and an overlying keratinized epidermis (Benson, 2012; Johansson & Headon, 2014; Sengel, 1990). As a barrier between the body and the external environment, the skin protects the body from UV rays, chemicals, and microorganisms, preventing infection as well as pressure, and pain, and resists abrasion from external objects (Rinn et al., 2006). Excessive external stimuli, such as UV rays (Dupont et al., 2013), air pollutants (Vierkötter et al., 2010), smog (Morita et al., 2009), and microbial attacks, induce skin oxidative stress, inflammation, immunosuppression, aging, and cancer (Abel & Haarmann-Stemmann, 2010). Skin diseases, such as psoriasis, vitiligo, and acne, have long plagued human beings. Metabolomics has been widely used to diagnose and explore treatments for human diseases (Aa et al., 2010; Cai & Dong, 2021; Li et al., 2017; Sévin et al., 2015). The occurrence of skin diseases is based on the skin tissue, and analyzing metabolite changes in the skin tissue may be helpful in understanding skin diseases and finding effective treatment measures.
Compared to metabolomic samples, such as serum and urine (Misra et al., 2018; Naz et al., 2014), tissues are solid and heterogeneous. In addition, skin tissues from different parts of an organism exhibit differences in their external characteristics, such as thickness, hairlessness, absence of sweat glands, and lighter color in palmoplantar skin compared to non-palmoplantar skin (Rinn et al., 2008; Yamaguchi et al., 1999, 2004, 2008, 2009; Zhao et al., 2019). In metabolomics experiments, the tissue is homogenized to obtain a homogeneous solution, which helps the quenching and extraction solvents to fully enter (Naz et al., 2014). Furthermore, homogeneous solvents should contain a proportion of polar solvents to facilitate contact with tissues primarily composed of water (Naz et al., 2014). For instance, Hu et al. used 50 mM ammonium bicarbonate (NH4HCO3; pH 8.1) to homogenize thawed skin tissue (Hu et al., 2012). Most researchers add the extraction solvent directly during sample homogenization (Buszewska-Forajta et al., 2019; Misra et al., 2018; Nizioł et al., 2021), allowing the homogenization and extraction processes to be performed simultaneously.
The efficiency of metabolite extraction depends on the selection of extraction solvents with different polarities and ratios. Extraction approaches can be optimized based on the structure and chemistry of tissues (Anwar et al., 2015). The organic solvent, methanol, and the inorganic solvent, water, are the most frequently used solvents for metabolite extraction. Perchloric acid has also been used to rapidly quench and extract water-soluble metabolites(Aa et al., 2010; Ima et al., 2019); however, due to the strong oxidizing properties of perchloric acid, it is only widely used in easily degradable tissues that are highly oxidative (Salek et al., 2011), and after homogenization, potassium hydroxide (KOH) (Salek et al., 2011) or potassium bicarbonate (KHCO3) (Ima et al., 2019) should be used for neutralization. This protocol is commonly applied in nuclear magnetic resonance (NMR)-based metabolomics. Dettmer et al. proposed that polar metabolites can be extracted using isopropanol, ethanol, methanol, acetonitrile, water, methanol/water, or mixtures of these solvents, whereas more lipophilic metabolites can be extracted using chloroform, chloroform/methanol/water mixtures, or ethyl acetate (Dettmer et al., 2011). Some researchers have also attempted to use methyl tert-butyl ether (MTBE) or dichloromethane instead of chloroform for extraction; however, the results tend to differ for different samples (Masson et al., 2010; Vorkas et al., 2018; Zhang et al., 2019). Compared with two-phase extraction, single-phase extraction is more commonly used in untargeted metabolomics, which can be used to effectively mitigate the loss of metabolites when they are collected from organic and inorganic phases (Buszewska-Forajta et al., 2019; Foroutan et al., 2020; Nizioł et al., 2021; Salek et al., 2011).
Solvent addition during the extraction process is also important. Wu et al. studied flounder liver and found that, compared with the stepwise and simultaneous addition of all solvents, the metabolomic data obtained using the two-step strategy were more accurate, less variable, and of higher quality (Wu et al., 2008). The application of the two-step extraction strategy in other studies has also achieved good extraction efficiency (Buszewska-Forajta et al., 2019; Masson et al., 2010). Anwar et al. also noted that changing the order of the added extraction reagents could affect the metabolite extraction results (Anwar et al., 2015).
Due to the heterogeneity of tissues, researchers have found it difficult to obtain a general extraction approach that is able to cover all metabolites, resulting in high metabolite abundance and good reproducibility for different tissues (Misra et al., 2018; Zukunft et al., 2018). The purpose of this study was to optimize and establish a stable, untargeted metabolomic approach with high metabolite coverage and recovery. This approach can be used to study changes in pathological skin tissue on a metabolic level and to identify differential metabolite markers.
In this study, reagents with different polarities (from strong to weak: water (H2O) > methanol (MeOH) > acetonitrile (ACN) > dichloromethane (DCM) > chloroform > isopropyl alcohol (IPA) > hexane) were used to develop 11 extraction solvent systems. Metabolites in non-volar skin were extracted using the 11 extraction solvent systems in which reagents were added using a two-step aqueous extraction process, and organic extraction was performed in different sequences. The feasibility of various extraction solvents was evaluated using multivariate analysis, coefficient of variation (CV), hierarchical cluster analysis (HCA), metabolite coverage, and relative peak area analysis, and the optimal extraction solvent was screened to provide experimental parameters for skin tissue metabolomics experiments.
2 Materials and methods
2.1 Preparation of skin tissue
Skin was collected from the hind paws of eight-week-old male and female C57BL/6 mice. After the mice were sacrificed by vertebral dislocation, the dorsal (non-volar) aspects of the skin from their hind paws were carefully cut using scissors and cleaned with phosphate buffered saline (PBS) for all subsequent experiments. The animal experiments were conducted according to ethical guidelines for the care and use of animals, with approval from the Jeonju University Animal Experimental Ethics Committee (jjIACUC-20220610-2022-0405-B1).
2.2 Homogenization and metabolite extraction
The extraction of metabolites from skin tissue was based on a two-step strategy (Anwar et al., 2015; Masson et al., 2010; Nizioł et al., 2021; Vorkas et al., 2015). The ratio of the regents in every extraction solvent system was based on the previous literatures and improved appropriately (Anwar et al., 2015; Masson et al., 2010; Salek et al., 2011; Vorkas et al., 2018). The experimental design is shwon in detail in Tables 1 and 2. Five repeat samples were prepared for each treatment, and the metabolite extraction process is shown in Fig. 1.
For S1-S6, after washing with PBS, the non-volar skin samples were placed in a 1.5 mL tube, where 900 µL of organic extraction solvent was added (or S7-S11, 900 µL aqueous extraction solvent was added), ultrasonic homogenization was performed for 30 s, and samples were placed on ice for 5 min intervals three times with an ultrasonic homogenizer (Vibra Cell, VCX 750, USA). Thereafter, 120 µL of pre-cold distilled water was added (for S7-S11, 120 µL of organic extraction solvent was added), samples were vortexed for 30 min, stored at -20 ºC for 1 h and centrifuged at 14,000 ×g for 15 min at 4ºC. Finally, the protein precipitates were removed, and subsequently dried using a vacuum centrifugal concentrator (N-BIOTEK, NB-503CIR, Korea) for derivatization. All reagents utilized in the aforementioned 11 solvent systems were of high-performance liquid chromatography (HPLC) grade. Methanol and acetonitrile were procured from Fisher Scientific (United States), while dichloromethane, chloroform, and hexane were sourced from Sigma-Aldrich (United Kingdom).
2.3 Derivatization of samples and gas chromatography-mass spectrometry (GC-MS) analysis
Sample derivatization was performed according to the protocol described in previous studies (Kim et al., 2018). Approximately 10 µL of 40 mg/mL methoxyamine hydrochloride (Sigma-Aldrich, United Kingdom) in pyridine (Sigma-Aldrich, India) was added to each dried skin tissue sample and shaken at 30 ºC for 90 min, for derivatization. Thereafter, 45 µL N-methyl-N-(trimethylsilyl) trifluoroacetamide (MSTFA, Sigma-Aldrich, Switzerland) was added and the samples were shaken at 37℃ for 30 min. Finally, a fatty acid methyl ester mixture (C8-C30) was added before GC-MS analysis to calculate the retention index.
A GC-qMS instrument (Shimadzu QP2010 Plus, Shimadzu, Tokyo, Japan) was used for derivatized sample analysis using an autosampler (AOC-20i + s, Shimadzu, Tokyo, Japan) and a primary Rtx-5MS capillary column (Cat. No. 13,623 − 6850, Restek, Bellefonte, PA, USA, 30 m × 0.25 mm × 0.25 μm). Approximately 1 µL of the derivatized sample was injected with a split ratio of 50:1 into the GC–MS system at 280 °C with helium as a carrier gas and a flow rate of 1.5 mL min− 1. The heating procedure of the oven temperature was as follows: maintained at 60 ºC for 3 min, increased to 220 °C at a rate of 5 ºC min− 1 and kept for 4 min, then increased to 320 °C at a rate of 10 °C min− 1, and maintained at 320 °C for a final 6 min. The transfer line to the mass spectrometer was maintained at 280 °C. The ion source temperature was 250 °C. Mass signals were acquired in full scan mode and collected at 3333 scans/s with a range of 50–800 m/z after a 3 min filament delay.
2.4 Data processing
First, the acquired mass spectral data were transformed into FAD-style. The Automated Mass Spectrometry Deconvolution and Recognition System (AMDIS) was used for deconvolution to remove noise, and to perform peak alignment, retention time calibration, and to match with the personal standard library (Davies, 1998). During the experimental run, the retention time, intensity, and peak shape of the injected chromatogram of quality control (QC) samples was checked (Masson et al., 2011), and the CV of the peak area was calculated; CV < 30% indicates good overall stability of the analytical platform.
GC-MS peaks were identified by comparing the mass spectral peaks and retention times with those of reference compounds in a personal standard library. The ELU-style results were uploaded to http://spectconnect.mit.edu, which is a software implementation that can systematically detect components that are conserved across samples without the need for a reference library or manual curation (Styczynski et al., 2007). Metabolites were identified through comparison with compounds in a personal standard library and the National Institute of Standards and Technology (NIST) MS library.
After identification, a data matrix was obtained with the peak area of the metabolite and sample number on the coordinate axis. Multivariate analyses were performed using unsupervised principal component analysis (PCA), supervised partial least squares discriminant analysis (PLS), and orthogonal partial least squares - discriminant analysis (OPLS) with SIMCA 14.1 software (Tsugawa et al., 2011; Wold & Sjöström, 1977). The peak area data matrix of the metabolites was normalized by median, Log transformation, and auto scaling, and subsequently uploaded to MetaboAnalyst5.0 (https://www.metaboanalyst.ca/) for HCA and pathway analysis. Other data analyses were performed using omicstudio. cn/tool and Origin 2021 software (OriginLab, Northampton, MT, USA).
3 Results and discussion
3.1 Overview of metabolites
Eleven different solvent systems, including MeOH-H2O (S1), MeOH/chloroform-H2O (S2), MeOH/ACN-H2O (S3), ACN/IPA-H2O (S4), MeOH/H2O-H2O (S5), Hexane/MeOH/IPA-H2O (S6), MeOH/H2O-chloroform (S7), MeOH/H2O-IPA (S8), MeOH/H2O-DCM (S9), ACN/H2O-chloroform (S10), and ACN/H2O-IPA (S11), were used to extract metabolites from non-volar skin tissue samples using a two-step extraction process. A total of 119 metabolites were identified using a standard library and the NIST library. The basic information on these metabolites is presented in Table S1. The number of identified metabolites in this study was higher than that in previous studies that performed skin tissue metabolomics with 96 and 34 identified metabolites (Bu et al., 2022; Tarentini et al., 2021). These metabolites are involved in key pathways, such as the citrate cycle (TCA cycle), aminoacyl-tRNA biosynthesis, biosynthesis of unsaturated fatty acids, arginine biosynthesis, pantothenate and CoA biosynthesis, glutathione metabolism, pyruvate metabolism, nitrogen metabolism, and glutamine, and glutamate metabolism. Among the 119 metabolites, nine metabolites were not registered in the Human Metabolome Database (HMBD), and 18 did not exist in the Kyoto Encyclopedia of Genes and Genomes (KEGG), including glycerol 1-hexadecanoate, heptadecanoic acid, N-acetyl-L-serine, methyl beta-glucopyranoside, erythronic acid, n-octacosane, n-docosane, methylphosphate, xylonic acid, 1-monooctadecanoylglycerol, glucopyranose, hexosanol, methioninamide, myo-inositol-2-phosphate, octadecadienoic acid, octadecatrienoic acid, n-pentadecane, and octadecenoic acid. However, some of these metabolites are involved in important metabolic reactions, such as glyceric acid-3-phosphate, which is an important intermediate metabolite in glycolysis (Lorenz et al., 2023).
3.2 Reproducibility assessment of metabolite extraction methods
Reproducibility is an important indicator for evaluating extraction quality. The median value of the CV of all detected metabolites is used to reflect the reproducibility of the method (García-Cañaveras et al., 2016; Pasikanti et al., 2008; Wedge et al., 2011). For GC-MS measurements, a median percentage of CV (% CV) higher than 30% was considered as an experimental outlier (Zarate et al., 2016). The % CV of the metabolite profiles of skin samples for all 11 solvent systems was analysed (Fig. 2). Among all solvent systems, the median % CV of S6 was found to be the highest (48.37%), whereas the % CV was less than 30% for all other solvent systems. With the exception of S6, if listed from small to large, the systems would be arranged as follows: S5 < S9 < S11 < S3 < S4 < S2 < S8 < S7 < S10 < S1. The median % CV of S5 and S1 was 6.511% and 18.59%, respectively, indicating that the sample data stability under these 10 extraction solvent systems was good, and the analytical test results were highly reproducible.
Percentage coefficient of variation (% CV) was used to evaluate the reproducibility of each metabolite according to the 11 different extraction solvents. S1: MeOH-H2O, S2: MeOH/Chloroform-H2O, S3: MeOH/ACN-H2O, S4: ACN/IPA-H2O, S5: MeOH/H2O-H2O, S6: Hexane/MeOH/IPA-H2O, S7: MeOH/H2O-chloroform, S8: MeOH/H2O-IPA, S9: MeOH/H2O-DCM, S10: ACN/H2O-chloroform, S11: ACN/H2O-IPA. Box plots represent the median ± interquartile range, and whiskers represent the 5th and 95th percentiles (n = 5)
3.3 Multivariate analysis
Multivariate analysis reflects the reliability of a method (Xu et al., 2019). After the peak area matrix data of all the metabolites obtained through skin metabolite identification were preprocessed (Masson et al., 2011), multivariate analyses, including PCA, PLS-DA, and OPLS-DA, were conducted to explain the differences in the extraction effects of the 11 extraction solvents, with the exception of S6. The PCA score plot indicated that principal component 1 (PC1) and principal component 2 (PC2) explained 54.9% of the variation between the extraction solvents (Fig. 3a) (R2X = 0.799, Q2 = 0.509). In PLS-DA, PC1 and PC2 explained 64.6% of the variation among extraction solvents (Fig. 3b) (R2X = 0.802, R2Y = 0.735, Q2 = 0.438). Cross-validation of the OPLS-DA model showed that PC1 explained 38.1% of the variation (Fig. 3c) (R2X = 0.863, R2Y = 0.859, Q2 = 0.659). R2 and Q2 exceeded 0.5 by a substantial amount, indicating that the models had goodness of fit and a good predictive ability. The model performed 200 random permutations to determine whether it was overfitted according to the intercepts of the R2 and Q2 regression lines and the Y-axis. Both R2 and Q2 were lower than the original values (Fig. 3d), indicating that the model was successfully established.
Multivariate analysis of metabolites extracted from 11 solvent systems (n = 5). (a) principal component analysis (PCA) score plot; (b) partial least squares discriminant analysis (PLS-DA) score plot; (c) orthogonal partial least squares - discriminant analysis (OPLS-DA) score plot; (d) OPLS-DA model permutation. R2 represents the interpretation rate of matrices of the built model, and Q2 represents the prediction ability of the model
The score plot in the multivariate analysis reflects the cluster degree and the degree of dispersion among the samples. A sample outside the confidence ellipse is considered abnormal (Yang et al., 2022). The different colors in the PCA score plot represent the metabolite samples obtained using different extraction solvent systems (Fig. 3a). The metabolite samples extracted using the same solvent were relatively clustered, indicating that the differences among the same-group samples were small. The PLS-DA and OPLS-DA score plots also indicated that samples extracted using the same solvent system gathered well (Fig. 3b, c). The metabolite samples extracted using S5 were close to the edge of the confidence ellipse, indicating that the sample confidence was lower than he metabolite samples in other solvent systems.
3.4 Metabolic profiles under different extraction solvent systems
HCA was used to evaluate variability among replicate samples and metabolic profile features as a whole (Kapoore et al., 2015; Peterson et al., 2016; Ser et al., 2015; Yang et al., 2022). Samples from the S1, S2, S3, and S4 solvent systems clustered together, and the metabolite abundance was similar. Similarly, samples from the S5, S7, S8, S9, S10, and S11 extraction systems were clustered together, and the metabolite abundance was similar (Fig. 4).
Hierarchical clustering analysis (HCA) of 119 metabolites extracted from non-volar skin tissue using 10 extract solvents. Each of the vertical columns represents the samples from different extraction solvents, whereas each horizontal row represents a different metabolite. The color represents the relative expression level of the metabolite in the sample set. The left-hand side is the metabolite clustering dendrogram, and the top is the sample clustering dendrogram. Clustering similarity was evaluated based on the Pearson correlation coefficient and Euclidean method
Venn diagrams reflect the overlap of metabolites detected using different methods (García-Cañaveras et al., 2016). Sixty-two metabolites were detected in all extraction solvent systems, and 12 metabolites were detected in all other solvent systems with the exception of S6, in addition, the set size of S5 and S6 were the lowest (Fig. 5a). Considering the reproducibility and reliability of the method, we only analyzed S1-S4 and S7-S11. Extraction solvents S1–S4 shared 76 metabolites, S1 and S2 produced 14 metabolites, and S2 extracted the highest number of metabolites (104) (Fig. 5b). The S7–S11 extraction solvent systems shared 78 metabolites, and S7 produced 16 metabolites alone, exhibiting obvious advantages over the other solvents (Fig. 5c).
(a) A venn diagram for the visualization of intersecting sets, revealing the number of shared and unique metabolites in skin tissue after extraction using 11 extraction solvent systems. (b) Four-way 2D-venn diagrams showing the effect of S1–S4 extraction solvents on the extractability of the quantified metabolites in skin tissue. (c) Five-way 2D-venn diagrams showing the effect of the S7-S11 extraction solvents on the extractability of the quantified metabolites in skin tissue
Further analysis of the HCA results revealed that the abundance of metabolites, including 4-hydroxyproline, acetamide, arachidonic acid, ascorbic acid, carbamic acid, cholesterol, cholest-5-ene, ribofuranose, ructose-6-phosphate, glycerol, glyceric acid-3-phosphate, galactose, glutamine, linoleic acid, maleic acid, octadecadienoic acid, octadecenoic acid, octadecanoic acid, oleic acid, oxalacetic acid, oxalic acid, palmitic acid, pentadecanoic acid, succinic acid, tetracosanoic acid, and xylonic acid, exhibited high abundances in S1-S4 (Fig. 4). Most of these metabolites are fatty and organic acids, which are mainly involved in the biosynthesis of unsaturated fatty acids, linoleic acid metabolism, alanine, aspartate, and glutamate metabolism, and the citrate cycle (TCA cycle) (Fig. 6a); the p values of all these pathways were less than 0.05. This indicates that studies related to lipid metabolism could implement extraction approaches in which an organic solvent is added during homogenization, followed by the addition of water to the vortex for the extraction of non-polar metabolites.
Metabolites including uracil, 2-keto-gluconic acid, butanal, butanoic acid, citric acid, fructose, galactose, dodecanoic acid, erythronic acid, ethanamine, fumaric acid, galacturonic acid, gamma-aminobutyric acid, gluconic acid, glucosamine, glyceric acid, glyceric acid-3-phosphate, glycerol, arabinose, aspartic acid, cysteine, malic acid, methioninamide, mannose-6-phosphate, mesoxalic acid, ethylmalonic acid, N-Acetyl-serine, phosphoric acid, and putrescine were more abundant in S7-S11 (Fig. 4). These metabolites are mainly involved in alanine, aspartate and glutamate metabolism, amino sugar and nucleotide sugar metabolism, glycerolipid metabolism, and the citrate cycle (Fig. 6b).This indicates that studies involving metabolism beyond lipids may employ extraction approaches that involve the addition of MeOH: H2O (1:1, v: v) or ACN: H2O (1:1, v: v) during homogenization followed by the introduction of an organic solvent into the vortex for the extraction of polar metabolites.
3.5 Relative peak area analysis
The intensities of different metabolites are used to evaluate the coverage and efficiency of the method, usually using the sum of the normalized peak area (Fritsche-Guenther et al., 2020), median peak height (Peterson et al., 2016), and relative peak area (Xu et al., 2021). With regards to the metabolite extraction method implementing organic extraction followed by aqueous extraction, the peak areas of the metabolites were normalized to the sum value of the S2 group, and the relative peak area of the amino acids, fatty acids, organic acids, and sugar metabolites in the skin tissue extracts from the S1, S3, and S4 extract solvents were calculated and compared (Fig. 7a). In the S1 group, with the exception of amino acids, the relative peak area of the other types of metabolites were lower when compared to those in the S2 group. In the S3 group, the relative peak area of amino acids, fatty acids, and organic acids were 1.423, 1.267, and 1.154 times higher than those in the S2 group, respectively. As for the S4 group, the relative peak area of all types of metabolites were lower than those in S3. According to the above results, the recovery rate of the metabolites extracted from S3 was the highest among the S1–S4 extraction solvent systems. Therefore, the S3 solvent is recommended for the extraction of non-polar metabolites.
Comparison of the relative peak area of various metabolites in skin tissue extracted from S1-S4(a) and S7-S11(b) extraction solvents. The peak area of metabolites is normalized to the sum of the mean values of each metabolite in S2(a) and S7(b), respectively. The data are displayed as mean ± SD, n = 5.The same lowercase letter on the bar indicates that there was no difference between the two groups in terms of extracted metabolites of the same type (p < 0.05)
The results of the comparison of the relative peak areas of various metabolites among the S7–S11 extraction solvents are shown in Fig. 7b. Compared with S7, the extraction efficiency of several common metabolites (except organic acids) in S8 was lower. The relative peak area of sugars and sugar alcohols in S7 were slightly higher than that in the other solvent systems. However, the relative peak area of fatty, amino, and organic acids in solvents S7 were lower than those in S9, S10, and S11. In particular, the relative peak area of amino acids and fatty acids extracted with S11 were the highest compared to the solvents, suggesting that metabolite recovery was high in S11, and the advantage was substantial.
S2 was extracted with 900 µL of MeOH/Chloroform (2:1)-120 µL of H2O, and S7 was extracted with 900 µL of MeOH/H2O (1:1)-120 µL chloroform, which suggested that the highest number of metabolites were extracted using the traditional MeOH/chloroform/H2O extraction combination, which were consistent with previous studies (Buszewska-Forajta et al., 2019; Foroutan et al., 2020).
4 Conclusion
This study is the first to evaluate different extraction solvent systems for metabolomics study of skin tissue. To evaluate different extraction solvent systems for skin tissue metabolomics, non-target metabolite profiling was performed using gas chromatography/mass spectrometry and high metabolite coverage, recovery, and reproducibility were compared. Metabolites with high abundances in the extraction systems with organic extraction, followed by aqueous extraction, were involved in the biosynthesis of unsaturated fatty acid metabolism, while metabolites with high abundances in the systems with aqueous extraction, followed by organic extraction, were involved in amino sugar and nucleotide sugar metabolism, and glycerolipid metabolism. MeOH/chloroform-H2O and MeOH/H2O-chloroform were the extraction systems that yielded the most metabolites, while MeOH/ACN-H2O and ACN/H2O-IPA exhibited higher metabolite recoveries.
Although it has been difficult to find a method that can extract metabolites comprehensively with high abundance and high repetition rate for skin tissue metabolomics thus far, this study demonstrated that these results are highly significant for skin metabolomics experiments, and the most suitable extraction approach can be selected according to the purpose of the experiment.
Data availability
No datasets were generated or analysed during the current study.
References
Aa, J., Wang, G., Hao, H., Huang, Q., Lu, Y., Yan, B., Zh, W., Liu, L., & Kang, A. (2010). Differential regulations of blood pressure and perturbed metabolism by total ginsenosides and conventional antihypertensive agents in spontaneously hypertensive rats. Acta Pharmacologica Sinica, 31, 930–937. https://doi.org/10.1038/aps.2010.86.
Abel, J., & Haarmann-Stemmann, T. (2010). An introduction to the molecular basics of aryl hydrocarbon receptor biology. https://doi.org/10.1515/bc.2010.128.
Anwar, M. A., Vorkas, P. A., Li, J. V., Shalhoub, J., Want, E. J., Davies, A. H., & Holmes, E. (2015). Optimization of metabolite extraction of human vein tissue for ultra performance liquid chromatography-mass spectrometry and nuclear magnetic resonance-based untargeted metabolic profiling. The Analyst, 140, 7586–7597. https://doi.org/10.1039/c5an01041a.
Benson, H. A. (2012). Skin structure, function, and permeation. Topical and Transdermal Drug Delivery: Principles and Practice, 1st ed.; Benson, HAE, Watkinson, AC, Eds, 1–22. https://doi.org/10.1002/9781118140505.
Bu, T., Zhng, M., Lee, S. H., Cheong, Y. E., Park, Y., Kim, K. H., Kim, D., & Kim, S. (2022). GC-TOF/MS-based metabolomics for comparison of volar and non-volar skin types. Metabolites, 12, 717. https://doi.org/10.3390/metabo12080717.
Buszewska-Forajta, M., Patejko, M., Macioszek, S., Sigorski, D., Iżycka-Świeszewska, E., & Markuszewski, M. J. (2019). Paraffin-embedded tissue as a novel matrix in metabolomics study: Optimization of metabolite extraction method. Chromatographia, 82, 1501–1513. https://doi.org/10.1007/s10337-019-03769-y.
Cai, Y., & Dong, Q. (2021). Metabonomics research accelerates discovery of medical biomarkers. E3S Web of Conferences, 03048. https://doi.org/10.1051/e3sconf/202124503048.
Davies, T. (1998). The new automated mass spectrometry deconvolution and identification system (AMDIS). Spectroscopy Europe, 10, 24–27.
Dettmer, K., Nürnberger, N., Kaspar, H., Gruber, M. A., Almstetter, M. F., & Oefner, P. J. (2011). Metabolite extraction from adherently growing mammalian cells for metabolomics studies: Optimization of harvesting and extraction protocols. Analytical & Bioanalytical Chemistry, 399, 1127–1139. https://doi.org/10.1007/s00216-010-4425-x.
Dupont, E., Gomez, J., & Bilodeau, D. (2013). Beyond UV radiation: A skin under challenge. International Journal of Cosmetic Science, 35, 224–232. https://doi.org/10.1111/ics.12036.
Foroutan, A., Fitzsimmons, C., Mandal, R., Piri-Moghadam, H., Zheng, J., Guo, A., Li, C., Guan, L. L., & Wishart, D. S. (2020). The bovine metabolome. Metabolites, 10, 233. https://doi.org/10.3390/metabo10060233.
Fritsche-Guenther, R., Bauer, A., Gloaguen, Y., Lorenz, M., & Kirwan, J. A. (2020). Modified protocol of harvesting, extraction, and normalization approaches for gas chromatography mass spectrometry-based metabolomics analysis of adherent cells grown under high fetal calf serum conditions. Metabolites, 10, 2. https://doi.org/10.3390/metabo10010002.
García-Cañaveras, J. C., López, S., Castell, J. V., Donato, M. T., & Lahoz, A. (2016). Extending metabolome coverage for untargeted metabolite profiling of adherent cultured hepatic cells. Analytical and Bioanalytical Chemistry, 408, 1217–1230. https://doi.org/10.1007/s00216-015-9227-8.
Hu, Z. P., Kim, Y. M., Sowa, M. B., Robinson, R. J., Gao, X., Metz, T. O., Morgan, W. F., & Zhang, Q. (2012). Metabolomic response of human skin tissue to low dose ionizing radiation. Molecular BioSystems, 8, 1979–1986. https://doi.org/10.1039/c2mb25061f.
Ima, B., Song, Z. C., Iv, C., & Mvi, A. (2019). Kidney harvesting and metabolite extraction for metabolomics studies in rodents - ScienceDirect. Methods in Cell Biology, 154, 15–29. https://doi.org/10.1016/bs.mcb.2019.05.009.
Johansson, J. A., & Headon, D. J. (2014). Regionalisation of the skin. Seminars in Cell & Developmental Biology, 25–26, 3–10. https://doi.org/10.1016/j.semcdb.2013.12.007.
Kapoore, R. V., Coyle, R., Staton, C. A., Brown, N. J., & Vaidyanathan, S. (2015). Cell line dependence of metabolite leakage in metabolome analyses of adherent normal and cancer cell lines. Metabolomics, 11, 1743–1755. https://doi.org/10.1007/s11306-015-0833-4.
Kim, S., Lee, H., & Kim, K. H. (2018). Metabolomic elucidation of recovery of Melissa officinalis from UV-B irradiation stress. Industrial Crops and Products, 121, 428–433. https://doi.org/10.1016/j.indcrop.2018.05.002.
Li, B., He, X., Jia, W., & Li, H. (2017). Novel applications of metabolomics in personalized medicine: A mini-review. Molecules, 22, 1173. https://doi.org/10.3390/molecules22071173.
Lorenz, M., Fritsche-Guenther, R., Bartsch, C., Vietzke, A., Eisenberger, A., Stangl, K., Stangl, V., & Kirwan, J. A. (2023). J.I.J.o.M.S. Serum starvation accelerates intracellular metabolism in endothelial cells. 24, 1189. https://doi.org/10.3390/ijms24021189.
Masson, P., Alves, A. C., Ebbels, T. M., Nicholson, J. K., & Want, E. J. (2010). Optimization and evaluation of metabolite extraction protocols for untargeted metabolic profiling of liver samples by UPLC-MS. Analytical Chemistry, 82, 7779–7786. https://doi.org/10.1021/ac101722e.
Masson, P., Spagou, K., Nicholson, J. K., & Want, E. J. (2011). Technical and Biological Variation in UPLC – MS-Based untargeted metabolic profiling of liver extracts: Application in an experimental toxicity study on Galactosamine. Analytical Chemistry, 83, 1116–1123. https://doi.org/10.1021/ac103011b.
Misra, B. B., Upadhayay, R. P., Cox, L. A., & Olivier, M. (2018). Optimized GC–MS metabolomics for the analysis of kidney tissue metabolites. Metabolomics, 14, 1–14. https://doi.org/10.1007/s11306-018-1373-5.
Morita, A., Torii, K., Maeda, A., & Yamaguchi, Y. (2009). Molecular basis of tobacco smoke-induced premature skin aging. Journal of Investigative Dermatology Symposium Proceedings, 53–55. https://doi.org/10.1038/jidsymp.2009.13.
Naz, S., Moreira dos Santos, D. C., Garcia, A., & Barbas, C. (2014). Analytical protocols based on LC–MS, GC–MS and CE–MS for nontargeted metabolomics of biological tissues. Bioanalysis, 6, 1657–1677. https://doi.org/10.4155/bio.14.119.
Nizioł, J., Copié, V., Tripet, B. P., Nogueira, L. B., Nogueira, K. O., Ossoliński, K., Arendowski, A., & Ruman, T. (2021). Metabolomic and elemental profiling of human tissue in kidney cancer. Metabolomics, 17, 1–15. https://doi.org/10.1007/s11306-021-01779-2.
Pasikanti, K. K., Ho, P. C., & Chan, E. C. (2008). Development and validation of a gas chromatography/mass spectrometry metabonomic platform for the global profiling of urinary metabolites. Rapid Communications in Mass Spectrometry: An International Journal devoted to the Rapid Dissemination of Up-to‐the‐minute. Research in Mass Spectrometry, 22, 2984–2992. https://doi.org/10.1002/rcm.3699.
Peterson, A. L., Walker, A. K., Sloan, E. K., & Creek, D. J. (2016). Optimized method for untargeted metabolomics analysis of MDA-MB-231 breast cancer cells. Metabolites, 6, 30. https://doi.org/10.3390/metabo6040030.
Rinn, J. L., Bondre, C., Gladstone, H. B., Brown, P. O., & Chang, H. Y. (2006). Anatomic demarcation by positional variation in fibroblast gene expression programs. PLoS Genetics, 2, e119. https://doi.org/10.1371/journal.pgen.0020119.
Rinn, J. L., Wang, J. K., Liu, H., Montgomery, K., Van De Rijn, M., & Chang, H. Y. (2008). A systems biology approach to anatomic diversity of skin. Journal of Investigative Dermatology, 128, 776–782. https://doi.org/10.1038/sj.jid.5700986.
Salek, R., Cheng, K. K., & Griffin, J. (2011). The study of mammalian metabolism through NMR-based metabolomics, Methods in enzymology, Elsevier. pp. 337–351. https://doi.org/10.1016/B978-0-12-385118-5.00017-7.
Sengel, P. (1990). Pattern formation in skin development. The International Journal of Developmental Biology, 34, 33–50. https://doi.org/10.1387/IJDB.2203463.
Ser, Z., Liu, X., Tang, N. N., & Locasale, J. W. (2015). Extraction parameters for metabolomics from cultured cells. Analytical Biochemistry, 475, 22–28. https://doi.org/10.1016/j.ab.2015.01.003.
Sévin, D. C., Kuehne, A., Zamboni, N., & Sauer, U. (2015). Biological insights through nontargeted metabolomics. Current Opinion in Biotechnology, 34, 1–8. https://doi.org/10.1016/j.copbio.2014.10.001.
Styczynski, M. P., Moxley, J. F., Tong, L. V., Walther, J. L., Jensen, K. L., & Stephanopoulos, G. N. (2007). Systematic identification of conserved metabolites in GC/MS data for metabolomics and biomarker discovery. Analytical Chemistry, 79, 966–973. https://doi.org/10.1021/ac0614846.
Tarentini, E., Odorici, G., Righi, V., Paganelli, A., Giacomelli, L., Mirisola, V., Mucci, A., Benassi, L., D’Aversa, E., Lasagni, C., Kaleci, S., Reali, E., & Magnoni, C. (2021). Integrated metabolomic analysis and cytokine profiling define clusters of immuno-metabolic correlation in new-onset psoriasis. Scientific Reports, 11, 10472. https://doi.org/10.1038/s41598-021-89925-7.
Tsugawa, H., Tsujimoto, Y., Arita, M., Bamba, T., & Fukusaki, E. (2011). GC/MS based metabolomics: Development of a data mining system for metabolite identification by using soft independent modeling of class analogy (SIMCA). BMC Bioinformatics, 12, 1–13. https://doi.org/10.1186/1471-2105-12-131.
Vierkötter, A., Schikowski, T., Ranft, U., Sugiri, D., Matsui, M., Krämer, U., & Krutmann, J. (2010). Airborne particle exposure and extrinsic skin aging. Journal of Investigative Dermatology, 130, 2719–2726. https://doi.org/10.1038/jid.2010.204.
Vorkas, P. A., Isaac, G., Anwar, M. A., Davies, A. H., Want, E. J., Nicholson, J. K., & Holmes, E. (2015). Untargeted UPLC-MS profiling pipeline to expand tissue metabolome coverage: Application to cardiovascular disease. Analytical Chemistry, 87, 4184–4193. https://doi.org/10.1021/ac503775m.
Vorkas, P. A., Abellona, U. M., & Li, J. V. (2018). Tissue multiplatform-based Metabolomics/Metabonomics for enhanced Metabolome Coverage. Methods in Molecular Biology, 1738, 239–260. https://doi.org/10.1007/978-1-4939-7643-0_17.
Wedge, D. C., Allwood, J. W., Dunn, W., Vaughan, A. A., Simpson, K., Brown, M., Priest, L., Blackhall, F. H., Whetton, A. D., Dive, C., & Goodacre, R. (2011). Is serum or plasma more appropriate for intersubject comparisons in metabolomic studies? An assessment in patients with small-cell lung cancer. Analytical Chemistry, 83, 6689–6697. https://doi.org/10.1021/ac2012224.
Wold, S., & Sjöström, M. (1977). SIMCA: A method for analyzing chemical data in terms of similarity and analogy. ACS Publications. https://doi.org/10.1021/bk-1977-0052.ch012.
Wu, H., Southam, A. D., Hines, A., & Viant, M. R. (2008). High-throughput tissue extraction protocol for NMR-and MS-based metabolomics. Analytical Biochemistry, 372, 204–212. https://doi.org/10.1016/j.ab.2007.10.002.
Xu, X., Zang, Q., Zhang, R., Liu, J., He, J., Zhang, R., & Abliz, Z. (2019). Systematic optimization and evaluation of sample pretreatment methods for LC-MS-based metabolomics analysis of adherent mammalian cancer cells. Analytical Methods, 11, 3014–3022. https://doi.org/10.1039/C9AY00792J.
Xu, F., Song, C., Liu, W., & Chen, G. (2021). Protocol for intracellular and extracellular metabolite detection in human embryonic stem cells. STAR Protocols, 2, 100740. https://doi.org/10.1016/j.xpro.2021.100740.
Yamaguchi, Y., Itami, S., Tarutani, M., Hosokawa, K., Miura, H., & Yoshikawa, K. (1999). Regulation of keratin 9 in nonpalmoplantar keratinocytes by palmoplantar fibroblasts through epithelial-mesenchymal interactions. The Journal of Investigative Dermatology, 112, 483–488. https://doi.org/10.1046/j.1523-1747.1999.00544.x.
Yamaguchi, Y., Itami, S., Watabe, H., Yasumoto, K., Abdel-Malek, Z. A., Kubo, T., Rouzaud, F., Tanemura, A., Yoshikawa, K., & Hearing, V. J. (2004). Mesenchymal-epithelial interactions in the skin: Increased expression of dickkopf1 by palmoplantar fibroblasts inhibits melanocyte growth and differentiation. Journal of Cell Biology, 165, 275–285. https://doi.org/10.1083/jcb.200311122.
Yamaguchi, Y., Passeron, T., Hoashi, T., Watabe, H., Rouzaud, F., Yasumoto, K., Hara, T., Tohyama, C., Katayama, I., Miki, T., & Hearing, V. J. (2008). Dickkopf 1 (DKK1) regulates skin pigmentation and thickness by affecting Wnt/beta-catenin signaling in keratinocytes. The Faseb Journal, 22, 1009–1020. https://doi.org/10.1096/fj.07-9475com.
Yamaguchi, Y., Morita, A., Maeda, A., & Hearing, V. J. (2009). Regulation of skin pigmentation and thickness by Dickkopf 1 (DKK1). The Journal of Investigative Dermatology. Symposium Proceedings / the Society for Investigative Dermatology, Inc. [And] European Society for Dermatological Research, 14, 73–75. https://doi.org/10.1038/jidsymp.2009.4.
Yang, K., Lv, T., Wu, J., Zhang, X., Xue, Y., Yu, P., & Liu, Q. (2022). The Protective Effect of Electroacupuncture on the renal cortex of SHR: A metabonomic analysis. Biomedical Chromatography, e5338. https://doi.org/10.1002/bmc.5338.
Zarate, E., Boyle, V., Rupprecht, U., Green, S., Villas-Boas, S. G., Baker, P., & Pinu, F. R. (2016). Fully automated trimethylsilyl (TMS) derivatisation protocol for metabolite profiling by GC-MS. Metabolites, 7, 1. https://doi.org/10.3390/metabo7010001.
Zhang, T., Xu, J., Liu, Y., & Liu, J. (2019). Metabolomic profiling for identification of potential biomarkers in patients with dermatomyositis. Metabolomics, 15, 1–8. https://doi.org/10.1007/s11306-019-1539-9.
Zhao, X., Psarianos, P., Ghoraie, L. S., Yip, K., Goldstein, D., Gilbert, R., Witterick, I., Pang, H., Hussain, A., & Lee, J. H. (2019). Metabolic regulation of dermal fibroblasts contributes to skin extracellular matrix homeostasis and fibrosis. Nature Metabolism, 1, 147–157. https://doi.org/10.1038/s42255-018-0008-5.
Zukunft, S., Prehn, C., Röhring, C., Möller, G., Hrabě de Angelis, M., Adamski, J., & Tokarz, J. (2018). High-throughput extraction and quantification method for targeted metabolomics in murine tissues. Metabolomics, 14, 1–12. https://doi.org/10.1007/s11306-017-1312-x.
Funding
This work was supported by the National Research Foundation of Korea (NRF) for funding this work by the Young Researcher Program (number 2020R1G1A100826811).
Author information
Authors and Affiliations
Contributions
TB and SK: conception and design, investigation, collection and assembly of data, data analysis and interpretation, writing-original draft preparation, writing-review and editing, and final approval of the manuscript. SK: project administration. All authors have read and agreed to the published version of the manuscript.
Corresponding author
Ethics declarations
Ethics approval and consent to participate
The animal experiments were conducted according to ethical guidelines for the care and use of animals, with approval from the Jeonju University Animal Experimental Ethics Committee (jjIACUC-20220610-2022-0405-B1).
Conflict of interest
The authors declare no conflicts of interest.
Additional information
Publisher’s Note
Springer Nature remains neutral with regard to jurisdictional claims in published maps and institutional affiliations.
Electronic supplementary material
Below is the link to the electronic supplementary material.
Rights and permissions
Springer Nature or its licensor (e.g. a society or other partner) holds exclusive rights to this article under a publishing agreement with the author(s) or other rightsholder(s); author self-archiving of the accepted manuscript version of this article is solely governed by the terms of such publishing agreement and applicable law.
About this article
Cite this article
Bu, T., Kim, S. Development of metabolome extraction strategy for metabolite profiling of skin tissue. Metabolomics 20, 48 (2024). https://doi.org/10.1007/s11306-024-02120-3
Received:
Accepted:
Published:
DOI: https://doi.org/10.1007/s11306-024-02120-3