Abstract
This study is a retrospective analysis of an elite cross from the Spanish National Barley Breeding Program. This was the most successful cross produced in the breeding program in the past 20 years. The progeny from this cross has been investigated at two points in the program, before and after selection, through the analysis of allelic frequencies at a number of genetic loci with molecular markers. Shifts in allelic frequencies after selection allowed the identification of genomic regions with selection footprints likely due to the breeding process. The cross was replicated in three different years, and therefore, the three progenies represent different selection histories but, in all cases, were preferentially selected compared to the lines from other crosses used in the program. The progenies were sampled at two generations, before conscious selection (F2) and after six generations of selection (F8). The F2 plants were genotyped with microsatellites, whereas 31 F8 lines were surveyed for SNP and presence/absence variation polymorphisms using a genotyping-by-sequencing system (DArTseq). The DArTseq markers were aligned to the barley physical map, and, after curation, over 3,000 were still available for the analysis. Overall, 15 genomic regions in the F8 lines had allele frequencies beyond chosen thresholds, indicating selection, eight toward parent Orria and seven toward Plaisant. These selection footprints partially validated QTLs detected through classical linkage mapping in a RIL population of the same cross. These validated selection footprints convey useful information for barley breeding, either through marker-assisted selection or through genomic selection.
Similar content being viewed by others
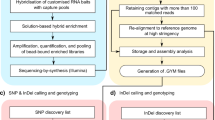
Avoid common mistakes on your manuscript.
Introduction
The use of molecular markers has become an important tool for genetic analysis and crop improvement (Rae et al. 2007; Varshney et al. 2007b). They are most commonly used for the exploration of genetic diversity, for the identification of genomic regions influencing traits of interest and for the selection of desirable phenotypes through the use of populations designed specifically for that purpose (Stuber et al. 1992; Mather 2002). But molecular markers can also be used to analyze existing populations and derive conclusions about the selective forces that shaped their genomes. This approach has been used extensively to analyze natural populations (Linhart and Grant 1996), but can also be attempted to analyze the outcome of breeding programs. Selection over generations, either natural or artificial, increases the frequencies of favorable alleles for the fitness of the organisms and, at the same time, decreases the frequencies of less favorable alleles, therefore resulting in shifts in allele frequencies at the population level (Allard 1996; Falconer and Mackay 1996; Danquah and Barrett 2002; Wisser et al. 2011).
Monitoring the evolution of molecular markers’ allele frequencies during the selection process has been proposed as a tool to identify specific regions of the genome related to trait(s) under selection (Wisser et al. 2008). The increase in frequency of favorable alleles due to selection is detected as shifts of allelic frequencies from their expected values under a random or neutral scenario. This approach has been named “selection mapping.” Historically, a number of studies have proven the principle that phenotypic change can be explained by significant changes in allele frequencies between generations, at loci governing important characters due to selection. Classical studies of this kind in barley were carried out by Allard and collaborators (Jain and Allard 1960; Allard and Jain 1962; Allard et al. 1972; Clegg et al. 1972, 1978; Kahler et al. 1975; Allard 1988), but also by Hockett et al. (1983) and Charlesworth and Charlesworth (1998). In other cereal species, selection mapping has been used as a tool to monitor recurrent selection, as in oat (De Koeyer et al. 2001) and maize (Stuber and Moll 1972; Labate et al. 1999; Coque and Gallais 2006).
This study is a retrospective analysis of an elite cross from the Spanish National Barley Breeding Program. The cross was the most successful cross produced in the breeding program in the past 20 years. Many progenies were selected in the early generations, resulting in a large number of advanced lines, some of which were released as cultivars in recent years in Spain. It is, therefore, a very relevant resource for six-row barley breeding in Mediterranean environments. The progeny from this cross was investigated at two points in the program, before and after conscious selection, through the analysis of allelic frequencies at a number of genetic loci with molecular markers. The objective of this study is to identify genomic regions that may represent selection footprints as a consequence of the breeding process, indicating possible targets for marker-assisted selection in this and other crosses where such regions are segregating. This approach has some similarities with genomic selection (Heffner et al. 2009), but, rather than building on new phenotyping of a training population, it relies on extensive past records and materials of a breeding program to derive conclusions about the breeding value of specific genomic regions. Pozniak et al. (2012) already encouraged the use of breeding records for this purpose in crop plants.
Materials and methods
The Spanish breeding program follows a pedigree scheme, in which F1 plants from biparental crosses are selfed over nine generations. Samples of the F2 generation from each cross are distributed across four breeding centers (Albacete, Lleida, Valladolid and Zaragoza) and are selected at each site for four generations before selections from each site are amalgamated into a joint selection scheme from the F6 onwards. At each generation, the progenies are evaluated for phenotypic traits, and the best are selected and promoted to the next generation, up to the F10. The cross between cultivars Orria and Plaisant was made three times in the breeding program, each at a different year, with different direction of crossing: 93Z074 (made in Zaragoza in 1993, as Plaisant × Orria), 96V738 (made in Valladolid in 1996, as Orria × Plaisant) and 97L058 (made in Lleida in 1997, as Orria × Plaisant). Therefore, there is a certain replication of the selection process, which ensures that line selection is not the result of chance events occurred at a single year or location. Orria is a six-row winter-sown cultivar with a mild vernalization requirement; it needs approximately 2 weeks of cold temperatures for full induction of heading (Fig. S1) and is very productive across most regions of Spain. Plaisant is also a six-row cultivar, but it is a typical winter type that needs a considerable vernalization time to achieve timely induction of flowering (Fig. S1), and it is less productive than Orria.
The crosses were sampled at two generations, before conscious selection (F2) and after 6 cycles of breeders’ selection (F8). It is not possible to retain all material from a breeding program for an indefinite period, and thus, only two of the original F2 populations (93Z074 and 96V738) could be utilized. Forty-one of the 45 advanced lines that reached the F8 generation in the breeding program (total number for the three crosses) were also available for analysis.
Genomic DNA was extracted, from leaf tissue, using the NucleoSpin Plant II kit (Macherey–Nagel, Düren, Germany). SSR genotyping was carried out in denaturing polyacrylamide gels after silver staining, following the protocol of Bassam et al. (1991). Gene-specific markers were evaluated in agarose gels, stained with SYBR Safe (Invitrogen, Carlsbad, CA, USA). A total of 102 plants from the 93Z074 F2 population were harvested for DNA extraction and marker analysis. Twenty-eight polymorphic microsatellite markers (simple sequence repeats, SSRs) distributed throughout the genome, and two markers of flowering time genes, VrnH1 and PpdH1, were chosen to genotype this F2 population. In a second step, 130 plants from the 96V738 F2 population were analyzed with 11 microsatellite markers and a marker for the VrnH1 gene. Previous genotyping had established that Orria and Plaisant both carried the same winter allele at VrnH2 (Loscos et al. 2014), and thus, full winter habit in this population was governed by segregation of the parental alleles at just VrnH1 (details on the control of the vernalization process by these genes are given in Distelfeld et al. 2009). The F8 lines were genotyped using different sets of markers: SSRs, SNPs and flowering genes (Table S1). Not all F8 lines could be genotyped with the whole set of markers. There were some gaps due to different causes. Indeed, 39 lines had been routinely genotyped with 48 random SSR markers in the framework of the breeding program, 28 of them polymorphic in this cross, and the data were incorporated into this study. The rest of the markers could only be assayed on lines for which either DNA or seed was still available in 2012. In addition, markers for two flowering genes relevant for adaptation of barley to Mediterranean regions were genotyped: VrnH1 (polymorphism as in Casao et al. 2011) and PpdH1 (polymorphism as in Turner et al. 2005).
In summary, the data for the F8 lines consist of SSRs and flowering time genes analyzed in the framework of the breeding program, not yet published, and new marker information generated via a genotyping-by-sequencing approach (GBS, Poland and Rife 2012). For this purpose, DNA from the set of 31 F8 lines for which residual seed was available was analyzed with the DArTseq system, provided by the company Diversity Arrays Technology (Kilian et al. 2012). This system combines complexity reduction methods with next-generation sequencing platforms, targeting primarily genic regions (Carling et al. 2015). It produces two types of markers, classical SNP and presence/absence variation, also named SilicoDArTs (http://www.diversityarrays.com/dart-application-dartseq-data-types).
The distribution of allelic frequencies of single markers at the F2 and the F8 was examined by testing deviations from Mendelian expectations for a scenario without selection, using a Chi-squared test, as recommended by Zhan and Xu (2011). The expected frequencies for this test were calculated using two different approaches: one assumed that the expected frequencies that would result from unselected F2 plants would follow a 1:1 ratio (i.e., 50 % of alleles from each parent) and another took into account that we actually have a measure of actual allelic frequencies in the F2 plants, which showed some shifts from the 1:1 ratio. So we decided to take a very conservative stand and used the most extreme allelic frequency found in the F2 s (68:32) as the expected frequency (an approach we will refer to as the worst-case scenario).Footnote 1 For the GBS data, given the high number of markers analyzed, control of the level of false-positives due to multiple testing was achieved by dividing the threshold P value (0.05) by the number of effective tests. This number was calculated with the software Keffective (Moskvina and Schmidt 2008) and resulted in 414 effective tests along the seven chromosomes.
For the SSR and flowering time genes data, multiple testing control was done using a Bonferroni correction, dividing in each case the threshold P value of 0.05 by the number of markers (30 for the F2 cross 93Z074 and the F8 lines, 12 for the F2 cross 96V738).
DArTseq generates two types of data: presence/absence markers (PAVs) and SNPs present in the sequenced fragments. The DArTseq sequences (69 bp each) corresponding to the PAV/SNP markers were assigned a location in the barley physical map (IBSC 2012), by using the Barleymap pipeline (Cantalapiedra et al. 2015, accessible online at http://floresta.eead.csic.es/barleymap), which relies on BLASTN (Altschul et al. 1990) and GMAP (Wu and Watanabe 2005) to perform the alignments. Thresholds of 95 % query coverage and 98 % alignment identity were imposed to declare positive matches against the available datasets of the physical map (ftp://ftpmips.helmholtz-muenchen.de/plants/barley/public_data/, updated 21-11-2012).
Results
Selection history
The proportion of lines derived from the three Orria × Plaisant crosses increased progressively in the breeding program as generations advanced, especially after F6, which corresponds to the stage at which selection began to be concentrated mainly on grain yield. In the first part of the program, up to F6, the relative frequencies of the lines from crosses between Orria and Plaisant tripled, but, in the second part, they increased almost fivefold (Table 1). The proportion of lines kept at F8 from each single cross was variable, but, in all three cases, the frequencies of the selections from this cross compared to whole generation size increased remarkably (Table 1). Out of each cross, there was seed available to carry out GBS analysis of 12, 16 and 3 F8 lines, of crosses 93Z074, 96V738 and 97L058, respectively.
Average grain yield expressed as percentage of the common check cultivars present at each trial was higher for the selections derived from the Orria × Plaisant crosses than the average of selections derived from all other crosses (Table S2). The data for the F8 trials of the last cross (97L058) are not given, as a replacement of two of the checks used in that year prevented comparison with previous data.
Genotypic frequencies in the F2 and F8
The expected allelic ratio at any generation should be 1:1 in the absence of selection or drift, and the expected genotypic ratio in the F2 should be 1:2:1. The observed genetic and genotypic ratios were tested for deviation from their expected values with a Chi-squared goodness-of-fit test (P < 0.05, Bonferroni correction) for each marker (Table 2). The allelic frequencies in the F2 derived from the 93Z074 cross did not depart from expected Mendelian allelic frequencies for 29 markers. Only Bmag0211, on 1H, departed significantly from the expected frequencies, showing an excess of Plaisant alleles. None of the 12 markers analyzed in the 96V738 F2 population presented frequencies departing the 1:1 expected ratio.
The F8 genotypes showed a higher proportion of loci with frequencies departing from the 1:1 allelic ratio expected under no selection. Out of the 30 markers, three (10 %) showed distorted allelic frequencies, and another six showed P values very close to the threshold. The three significant markers showed allelic frequencies skewed toward Orria (Table 2).
Genotyping-by-sequencing of the F8 lines
The subset of 31 F8 lines was genotyped with the DArTseq method of GBS as a part of a larger set of over 300 genotypes. In the whole set, a total of 8,535 tag sequences presented SNP polymorphisms and 15,526 tag sequences were scored as PAVs. Of these, 6,876 and 7,498 tags (for SNP and PAV, respectively) were aligned successfully to the reference sequence using the tool Barleymap (Cantalapiedra et al. 2015), most to single positions. A large proportion, 5,941 SNP and 6,344 PAV, also had a genetic location assigned. From those, 2,122 SNPs and 2,242 PAVs were polymorphic between the parents, Orria and Plaisant. Seven SNPs that presented multiple genetic positions were removed. Moreover, SNPs with over 10 % missing data or heterozygous alleles were also deleted, leaving 1,373 valid SNPs for the analysis. Regarding PAVs, removal of markers with over 10 % missing data left 2,147 suitable for the analysis. Therefore, further analyses were conducted with curated 3,520 markers.
Overall, polymorphic SNPs revealed the presence of 59 % of alleles from parent Orria and only 41 % from Plaisant. The observed frequencies of the SNPs in the 31 lines were compared to expectations under no selection.
There was good coverage of all chromosomes, from a minimum of 339 markers on 4H to a maximum of 795 on 7H (Table 3). The largest gap detected was 10.5 cM wide on 5H (between cM 31.4 and 41.9), probably due to lack of polymorphism between the parents at that region. The plot of the allelic frequencies of 31 F8 lines against genetic (Fig. 1) and physical distance (Fig. S2) revealed a profile that clearly indicated the action of selection. There were 655 markers with higher than expected Orria allele frequencies and 117 for the Plaisant allele, considering the 1:1 expected frequencies ratio. Using the worst-case scenario expected frequencies, these figures change to 252 and 27, respectively.
a Scan of allelic frequencies corresponding to parent Orria in 31 F8 lines of the cross Orria × Plaisant genotyped with DArTseq SNP (red circles) and PAV (blue circles) markers derived from a genotype-by-sequencing assay. All valid SNP and PAV are represented, with genetic positions extracted from the consensus map published by IBSC (2012). b DArTseq markers (SNP or PAV) left after removal of conspicuous double recombinants. At the bottom of the graph, triangles indicate the position of other known markers in the physical map, numbered according to Table 4. Green triangles indicate coincidence of favorable alleles between the two studies, red ones indicate disagreement and orange ones indicate an inconclusive comparison. Selection footprint regions are numbered S1 to S15 and encircled with a black line. The blue (96V738) and red (93Z074) lines represent the percentage of Orria alleles for the lines derived from each of the two main crosses (moving averages of 8 markers). Thresholds: horizontal lines at values 69.4 and 30.6 represent significance thresholds for Chi-squared tests corresponding to expected allelic frequencies of 1:1, P < 0.05 with a correction for the number of effective tests (414). Horizontal lines at values 80.7 and 19.3 correspond to thresholds calculated in the same manner, for worst-case scenario expected frequencies (see text). In the x-axis, breaks between chromosomes are indicated with vertical dashed lines
Even after curation of the results, the scans were not completely clean because the localization of the DArTseq markers on the physical map carries some uncertainty. These inconsistencies could be a consequence of the methods used to estimate physical positions and anchoring of sequences (IBSC 2012) and will be curated as more data become available for analysis. In order to remove obviously misplaced markers, genotypes of the 31 lines were ordered according to Barleymap results. Genetic linkage information was factored in by searching and removing double recombinants in five marker windows. This process was done iteratively per chromosome until a cleaner profile was obtained (approximately 15 iterations per chromosome). A total of 2,372 markers were retained after this process. Some markers with minor local shifts were also removed in the process, but the abundance of markers still allowed a dense and clean allelic frequency scan (Fig. 1b).
SNPs departing significantly from expected segregations indicated the presence of selection footprints at each chromosome. We chose to declare a selection footprint when at least one marker exceeded the threshold, and the profile of the scan of surrounding regions clearly hinted at the presence of a peak. A total of 15 regions were identified following this criterion (labeled as S1–S15 in Fig. 1), for the lower threshold (1:1 expected frequencies). With the worst-case scenario criterion, seven of these regions were still significant (S4–S10, Fig. 1). Eleven of the regions (six toward Orria and five toward Plaisant) were the result of apparent selection at the two main crosses, 93Z074 and 96V738 (Fig. 1), and four QTLs were due to selection in one cross (two toward Orria and two toward Plaisant). The results for cross 97L058 were based on just three lines, a number too low to derive conclusions from allelic frequencies (data not shown). Most of these peaks were rather narrow, either considering physical (Fig. 2S) or recombination distances. There was a remarkable exception at the footprint on 3H, possibly at a centromeric position, which spanned more than half of the physical chromosome. To relate the profiles identified in the F8 lines with the results of allelic distribution and QTL analysis of a RIL population originated from one of the crosses analyzed here (Mansour et al. 2014), markers from the RIL population were positioned in silico on the barley physical map (Table 4). SNP markers and flowering time genes associated with QTL for traits used as selection criteria in the breeding program (grain yield, plant height and thousand kernel weight), or matching regions of skewed allelic frequencies in the cited work, were plotted with the DArTseq markers in the F8 lines (Fig. 1). The three regions that presented high distortion of allelic frequencies in Mansour et al. (2014) also showed the same kind of frequency shift in the F8 lines, and in the same direction (tags 2–7, 14–15 and 17, S1, S8 and S9 in Fig. 1): The position of VrnH1 on 5H matched a region showing an excess of Orria alleles at DArTseq markers, coincident with a clear deviation in the F8 lines for this allele and also with a QTL identified in the RIL population for yield and heading date. BOPA1 SNP markers on 1H (11_10275) and in the long arm of 4H (11_10379) pointed to regions showing distorted segregation that were previously identified in the QTL analysis of the RIL population. In the region of S3, selection was evident for only one of the two main crosses (96V738), and this was also the region of a grain yield QTL with QTL by environment effect in the RIL study (tags 10–12).
The region of S9 also had the strongest grain yield QTL in the RIL study. On the other hand, the positions of the QTLs found in the RIL population matched selection footprints only in some cases. QTLs and selection footprints S2, S3 (2H), S9 (5H) and S11 (6H) were in good agreement. Forty percent of the selection footprints did not have any counterpart in the RIL study (S5, S6, S7, S11, S12 and S14). The chromosome with most selection footprints was 7H (four), but only one of them (S15) may reflect selection for QTLs (plant height) detected in the RIL study (tags 23–24).
We further inspected the genomic regions around each of the selection footprints identified (at the exact genetic position of the peak, and in a 2-cM-wide interval around them, Table S3) to determine their gene content, using the Barleymap Web tool (Cantalapiedra et al. 2015). The total number of gene models sharing the exact genetic positions of the peaks was 1,276 for the IBSC map (2012) and 4,295 for the POPSEQ map (Mascher et al. 2013). The median and maximum number of gene models for the 15 selection footprints were 34 and 631 using the IBSC reference map. These numbers increased to 50 and 2,150 in the case of POPSEQ (Table S3). Although those numbers are high for most of the footprints, making it difficult to identify candidate genes associated with them, some results are worth mentioning. Among the 50 gene models identified in POPSEQ for S1 (chromosome 1H, 54.53 cM), a High Confidence gene model (MLOC_19482) is annotated as a late embryogenesis abundant protein, LEA-3. Similarly, the MADS-box transcription factor VRNH1 (AK360697) lies within the S9 region (chromosome 5H, 125.76) for both IBSC and POPSEQ maps, even though the number of gene models at this peak is high.
Discussion
Selection in breeding programs is carried out by phenotypic evaluation over years, culling the worst progenies according to several traits, and promoting the best to form the next generation. Thus, the plant material finally produced by a breeding program carries an associated history of the selection that acted on their genomes. The expected outcome of this process is an increased proportion of favorable alleles at loci relevant for adaptation to prevailing environmental conditions and stresses, as generations advance.
The phenotypic superiority of the selections from the three Orria–Plaisant crosses was evident by their increasing frequency (as a proportion of the total number of lines of each generation) throughout the program, and by their significantly superior grain yields. Orria was also a parent of some other crosses in the program, but none of the selections were as productive as those from the crosses with Plaisant, so it appears that it is the particular complementarity of these two cultivars that has led to the superior selections. These parents therefore provide an elite genetic background for six-row barley breeding under Mediterranean conditions. For the purpose of this study, our expectation is that this phenotypic superiority should be partially related to the shifts in allelic frequencies observed in the advanced materials (F8 lines).
Analysis of the two F2 populations showed little evidence of allelic frequencies significantly differing from the expected 1:1 ratio, but to a lesser extent than observed in the F8 lines. We have no reason to believe that the allelic frequencies in the F2 of the untested cross were different from the two analyzed. The considerable differences between allelic frequencies at the F2 and F8 of the same cross are, most likely, the result of artificial selection. Karakousis et al. (2003), using microsatellites, found that several SSRs assessed in F2 crosses showed distorted segregation, while others showed the expected 1:2:1 ratio. They explained this result as a consequence of preferential amplification of alleles, resulting in the inability to detect heterozygote’s for some markers.
Selection in the Spanish National Barley Program is for healthy plants with short straw, large grain size and high grain yield, and we expected to find direct responses to selection for genes controlling these traits. There was no conscious selection for the length of the growth cycle or growth habit, and therefore, any changes in genes underlying these traits (like VrnH1) should be due to, drift, indirect selection or natural selection. We had previous knowledge of the presence of several QTLs for agronomic traits in a RIL population derived from one of the crosses under study, 97L058 (Mansour et al. 2014). A RIL population, by definition, should not have been subjected to artificial selection. Nevertheless, that study found three regions that had suffered a severe shift of allelic frequencies, in excess of 3:7 ratios. The most conspicuous region was on 5HL, surrounding VrnH1, favoring the Orria allele. Also, QTLs for grain yield, days to heading and maturity, were detected at this same region. We hypothesized that this was due to the different vernalization requirement induced by the VrnH1 alleles of these two parents, reacting against different winter temperatures at the multiplication fields. A warm winter may have caused a strong selection against progenies with the Plaisant allele at this locus. Some of these progenies may have failed to produce fertile tillers and, hence, may have been removed from the population. In the present study, we have found further evidence of the strong selection pressure affecting this gene under Spanish conditions, though it is not possible to know whether selection occurred at any particular year, or whether it was due to directional selection over the years. In any case, the region containing VrnH1 was clearly selected, once more against the Plaisant allele, during the breeding process. There were two other regions, one at 1H and another at 4H in which the RILs presented high frequencies of Plaisant alleles. These two regions were also found in the present study, with a selection footprint in the same direction as for the RILs but, although some QTLs for traits subjected to selection were found nearby, the overlap was sufficient to declare that the same genes were selected in the two studies. The region on 1H is, however, not far from Fr-H3 (Fisk et al. 2013), a frost tolerance QTL coincident with a grain yield QTL in the RIL population (tag 1 in Fig. 1). Though the position is not the same, the location of Fr-H3 is not absolutely certain, and we cannot discard that it is related to the selection footprint S1. Interestingly, we found a candidate gene for a late embryogenesis abundant protein, LEA3, within that region. LEA3 family members have been previously associated with tolerance to different abiotic stresses, including freezing (NDong et al. 2002; Kosová et al. 2014). Another member of this family, HvA1 (Straub et al. 1994), is located on the long arm of 1H, but it does not correspond to peak S1.
During the development of a RIL population by single seed descent (as was the case for 97L058), lines are discarded only because they do not survive at some generation. Therefore, only those traits that have a strong effect on fitness can result in the selection observed in the RIL population. The occurrence of the same allelic shifts during the development of two completely independent sets of materials—RILs, F8 lines—with different selection history suggests that alleles at genes located in these regions were preferentially selected by the prevailing environments experienced in the studies. In the case of the F8 lines, the effects of the underlying genes on fitness must have affected agronomic value dramatically, and the effects of specific parental alleles were so important as to be selected consistently in the breeding program.
The strong selection footprint close to the centromere on 3H (S4) is intriguing. A very strong selection pressure during the breeding process almost led to fixation for the Orria allele of a large part of this chromosome. We can only speculate about possible reasons for occurrence of this selection footprint. Other authors have reported grain yield, lodging or plant height QTL in that region of 3H (Hayes et al. 1993; von Korff et al. 2008; Rollins et al. 2013), but no QTL was detected in this region in the QTL analysis of agronomic traits in the RIL population (Mansour et al. 2014). Since segregation distortion was not detected on chromosome 3H in the RIL population nor in the two F2 analyzed, it must be concluded that it is the result of artificial selection for traits not directly related to fitness in this population. Some traits with QTLs mapped to this region are spike morphology (Chen et al. 2012) and head shattering (Larson et al. 1996; Kandemir et al. 2000). We do know that head shattering is heavily selected against during the early generations of our breeding program. However, a possible presence of a QTL for this trait in the RIL population could not be confirmed, because the trait was not recorded, and, in any case, it should have had a correlated response to selection on grain yield as well. Another possible reason underlying a strong response to selection is disease resistance. Some disease resistance QTLs have been identified in this region of 3H: net blotch (Cakir et al. 2011), spot blotch (Roy et al. 2010) and scald (Li and Zhou 2011; Hofmann et al. 2013). Although we are not aware of the incidence of diseases during the breeding process, it is customary that breeders remove progenies, which show symptoms of disease, even mild. We ignore if the parents diverge for response to scald, but this explanation would fit quite well the location of the S4 region, if it represented the same QTL as the one identified by Hofmann et al. (2013). The two markers flanking QTL Rrs1Rh4 in that work are located exactly at the peak of the selection footprint.
The lack of complete correspondence between QTLs selected in a previous study and the selection footprints found here is a common feature of all QTL validation studies (Bernardo 2008). Several kind of statistical biases and genotype-by-environment interactions are the most common causes underpinning this phenomenon.
Our results offer strong evidence for the action of selection on allelic frequencies, and this is supported by results from other retrospective studies. Condón et al. (2008) used SSR markers to analyze changes in allelic diversity in a barley breeding program carried out between 1958 and 1998. They found evidence for a reduction in number of alleles at some marker loci. The authors hypothesized that it was the result of linkage of these markers to major loci for disease resistance or malting quality that were presumably under selection during the breeding process. Several authors have indicated changes in allelic frequencies, with a reduction in diversity for modern cultivars (Russell et al. 2000; Karakousis et al. 2003). Similarly, Fu and Somers (2009) using wheat microsatellites reported that allelic reduction occurred in every part of the wheat genome as a consequence of breeding. Various studies of highly variable barley populations have reported changes in genotypic and allelic frequencies between generations due to bulk selection, apparently reflecting shifts for local adaptation. Clegg et al. (1978), studying Composite Cross V (CCV) of barley after 30 generations, and Saghai-Maroof et al. (1994), studying barley Composite Cross II (CCII) after 53 generations, found substantial genetic changes over the different generations. Changes due to recurrent selection programs have also been reported in maize (Stuber et al. 1980; Romay et al. 2012) and oat (De Koeyer et al. 2001).
The novelty of our approach, however, lies in the genome-wide survey of the selected lines, the distinct signature of selection footprints, and in the direct applicability of the findings to continued cultivar development in breeding programs. This has been possible due to the combination of several features, some of which have become recently available for barley: high-throughput genotyping platforms that provide enough density of marker coverage, a reference draft genome and sufficient sample size after several generations of selection. We have been able to identify regions targeted by selection, without further phenotyping, and based on a much reduced set of lines and, hence, at an affordable cost. The number of lines sampled in the F8 is both inappropriate (due to heavy allelic frequency distortions) and insufficient to construct a high confidence map by genetic mapping alone, but, in combination with the reference genome, these results demonstrate that this sample is sufficient to derive meaningful conclusions about the position of selection targets. This information is directly applicable to breeding, through the identification of segments with favorable or unfavorable breeding values associated with particular alleles. Pozniak et al. (2012) already encouraged the use of phenotypic data routinely collected by breeding programs to identify marker–trait associations. Our approach actually does not require collection of phenotypic data, as it just relies on allelic frequencies, but could work in combination with phenotypic data as well. Similar approaches have been attempted in animal breeding. For instance, Wiener et al. (2011) aimed at discovering genomic regions controlling phenotypic traits that differentiated two cattle breeds and found that they could pick up regions with genes that caused only large phenotypic effects. In our case, the close-knit family structure helps to identify selection footprints with higher power. Also, Martinez et al. (2012) were able to find markers associated with traits highly relevant for salmon breeding by just assessing population divergence with molecular markers, taking into account the selection history of their populations.
Recently, genomic selection (GS) is becoming one of the methods of choice for plant breeders (Heffner et al. 2009). Most often, genomic selection relies on phenotyping of newly created training populations to estimate the breeding values of chromosomal segments of relevant germplasm. The approach presented in this study can be complementary to genomic selection. Actually, its outcome can be combined with standard GS to optimize the estimation of genomic breeding values (GEBV) for genomes of selected parents. Breeding programs usually keep large amounts of data from selection trials. If a sufficient number of advanced lines are also kept in storage, the approach that we have followed in this study would also be feasible, producing high value information, fast and cheap, provided there are sufficient genomic resources developed for the particular crop.
Notes
Actually, we used this frequency and its reciprocal, 32:68 to run two different Chi-squared tests, to allow for drift or selection in both directions. We took the test with the highest P value of the two to build the final Chi-squared test result for this “worst-case” scenario.
Abbreviations
- GBS:
-
Genotyping-by-sequencing
- SNP:
-
Single nucleotide polymorphism
- PAV:
-
Presence–absence variation
- QTL:
-
Quantitative trait locus
References
Allard RW (1988) Genetic changes associated with the evolution of adaptedness in cultivated plants and their wild progenitors. J Hered 79:225–238
Allard RW (1996) Genetic basis of the evolution of adaptedness in plants. Euphytica 92:1–11
Allard RW, Jain SK (1962) Population studies in predominantly self-pollinated species. II: Analysis of quantitative genetic changes in a bulk hybrid population of barley. Evolution 16:90–101
Allard RW, Kahler AL, Weir BS (1972) The effect of selection on esterase allozymes in a barley population. Genetics 72:489–503
Altschul SF, Gish W, Miller W, Myers EW, Lipman DJ (1990) Basic local alignment search tool. J Mol Biol 215:403–410
Bassam BJ, Caetano-Anollés G, Greshoff PM (1991) Fast and sensitive silver staining of DNA in polyacrylamide gels. Anal Biochem 196:80–83
Bernardo R (2008) Molecular markers and selection for complex traits in plants: learning from the last 20 years. Crop Sci 48:1649–1664
Cakir M, Gupta S, Li C, Hayden M, Mather DE, Ablett GA, Platz GJ, Broughton S, Chalmers KJ, Loughman R, Jones MGK, Lance RCM (2011) Genetic mapping and QTL analysis of disease resistance traits in the barley population Baudin × AC Metcalfe. Crop Pasture Sci 62:152–161
Cantalapiedra CP, Boudiar R, Casas AM, Igartua E, Contreras-Moreira B (2015) BARLEYMAP: physical and genetic mapping of nucleotide sequences and annotation of surrounding loci in barley. Mol Breed (in press)
Carling J, Heller-Uszynska K, Jaccoud D, Machado A, Hopper C, Xia L, Vippin C, Caig V, Uszynski G, Kilian A (2015) DArT™ and DArTseq™ genome profiling for breeding, pre-breeding and population genetics applications. Contribution P0052, XXIII Plant and Animal Genome, San Diego, CA 10–14 Jan 2015
Casao MC, Igartua E, Karsai I, Bhat PR, Cuadrado N, Gracia MP, Lasa JM, Casas AM (2011) Introgression of an intermediate VRNH1 allele in barley (Hordeum vulgare L.) leads to reduced vernalization requirement without affecting freezing tolerance. Mol Breed 28:475–484
Charlesworth B, Charlesworth D (1998) Some evolutionary consequences of deleterious mutations. Genetica 103:3–19
Chen GD, Li HB, Zheng Z, Wei YM, Zheng YL, McIntyre CL, Zhou MX, Liu CJ (2012) Characterization of a QTL affecting spike morphology on the long arm of chromosome 3H in barley (Hordeum vulgare L.) based on near isogenic lines and a NIL-derived population. Theor Appl Genet 125:1385–1392
Clegg MT, Allard RW, Kahler AL (1972) Is the gene the unit of selection? Evidence from two experimental plant populations. Proc Natl Acad Sci USA 69:2474–2478
Clegg MT, Kahler AL, Allard RW (1978) Estimation of life cycle components of selection in an experimental plant population. Genetics 89:765–792
Condón F, Gustus C, Rasmusson DC, Smith KP (2008) Effect of advanced cycle breeding on genetic diversity in barley breeding germplasm. Crop Sci 48:1027–1036
Coque M, Gallais A (2006) Genomic regions involved in response to grain yield selection at high and low nitrogen fertilization in maize. Theor Appl Genet 112:1205–1220
Danquah EY, Barrett JA (2002) Grain yield in composite cross five of barley: effects of natural selection. J Agric Sci 138:171–176
De Koeyer DL, Phillips RL, Stuthman DD (2001) Allelic shifts and quantitative trait loci in a recurrent selection population of oat. Crop Sci 41:1228–1234
Distelfeld A, Li C, Dubcovsky J (2009) Regulation of flowering in temperate cereals. Curr Opin Plant Biol 12:178–184
Falconer DS, Mackay TFC (1996) Introduction to quantitative genetics, 4th edn. Longman Group, Harlow, Essex 464 pp
Fisk SP, Cuesta-Marcos A, Cistué L, Russell J, Smith KP, Baenziger S, Bedo Z, Corey A, Filichkin T, Karsai I, Waugh R, Hayes PM (2013) FR-H3: a new QTL to assist in the development of fall-sown barley with superior low temperature tolerance. Theor Appl Genet 126:335–347
Fu YB, Somers DJ (2009) Genome-wide reduction of genetic diversity in wheat breeding. Crop Sci 49:161–168
Hayes PM, Lui BH, Knapp SJ, Chen F, Jones B, Blake T, Franckowiak J, Rasmusson D, Sorrells M, Ullrich SE, Wesenberg D, Kleinhofs A (1993) Quantitative trait locus effects and environmental interaction in a sample of North American barley germ plasm. Theor Appl Genet 87:392–401
Heffner EL, Sorrells ME, Jannink JL (2009) Genomic selection for crop improvement. Crop Sci 49:1–12
Hockett EA, Eslick RF, Qualset CO, Dubbs AL, Stewart VR (1983) Effects of natural selection in advanced generations of barley composite cross II. Crop Sci 23:752–756
Hofmann K, Silvar C, Casas AM, Herz M, Büttner B, Gracia MP, Contreras-Moreira B, Wallwork H, Igartua E, Schweizer G (2013) Fine mapping of the Rrs1 resistance locus against scald in two large populations derived from Spanish barley landraces. Theor Appl Genet 126:3091–3102
IBSC (The International Barley Genome Sequencing Consortium) (2012) A physical, genetic and functional sequence assembly of the barley genome. Nature 491:711–716
Jain SK, Allard RW (1960) Population studies in predominantly self-pollinated species. I: Evidence for heterozygote advantage in a closed population of barley. Proc Natl Acad Sci USA 46:1371–1377
Kahler AL, Clegg MT, Allard RW (1975) Evolutionary changes in the mating system of an experimental population of barley (Hordeum vulgare L.). Proc Natl Acad Sci USA 72:943–946
Kandemir N, Kudrna DA, Ullrich SE, Kleinhofs A (2000) Molecular marker assisted genetic analysis of head shattering in six-rowed barley. Theor Appl Genet 101:203–210
Karakousis A, Barr AR, Chalmers KI, Ablett GA, Holton TA, Henry RJ, Lim P, Langridge P (2003) Potential of SSR markers for plant breeding and variety identification in Australian barley germplasm. Aust J Agric Res 54:1197–1210
Kilian A, Wenzl P, Huttner E, Carling J, Xia L, Blois H, Caig V, Heller-Uszynska K, Jaccoud D, Hopper C, Aschenbrenner-Kilian M, Evers M, Peng K, Cayla C, Hok P, Uszynski G (2012) Diversity arrays technology: a generic genome pro filing technology on open platforms. In: Pompanon F, Bonin A (eds) Data production and analysis in population genomics: methods and protocols, methods in molecular biology, vol 888. Springer, New York, pp 67–89
Kosová K, Vitámvás P, Prášil T (2014) Proteomics of stress responses in wheat and barley: search for potential markers of stress tolerance. Front Plant Sci 5:711
Labate JA, Lamkey KR, Lee M, Woodman WL (1999) Temporal changes in allele frequencies in two reciprocally selected maize populations. Theor Appl Genet 99:1166–1178
Larson SR, Kadyrzhanova D, McDonald C, Sorrells M, Blake TK (1996) Evaluation of barley chromosome-3 yield QTLs in a backcross F2 population using STS-PCR. Theor Appl Genet 93:618–625
Li HB, Zhou MX (2011) Quantitative trait loci controlling barley powdery mildew and scald resistances in two different barley doubled haploid populations. Mol Breed 27:479–490
Linhart YB, Grant MC (1996) Evolutionary significance of local genetic differentiation in plants. Annu Rev Ecol Syst 27:237–277
Loscos J, Igartua E, Contreras-Moreira B, Gracia MP, Casas AM (2014) HvFT1 polymorphism and effect—survey of barley germplasm and expression analysis. Front Plant Sci 5:251
Mansour E, Casas AM, Gracia MP, Molina-Cano JL, Moralejo M, Cattivelli L, Thomas WTB, Igartua E (2014) QTL for agronomic traits in an elite barley population for Mediterranean conditions. Mol Breed 33:249–265
Martinez V, Dettleff P, Lopez P, Fernandez G, Jedlicki A, Yañez JM, Davidson WS (2012) Assessing footprints of selection in commercial Atlantic salmon populations using microsatellite data. Anim Genet 44:223–226
Mascher M, Muehlbauer GJ, Rokhsar DS, Chapman J, Schmutz J, Barry K, Muñoz-Amatriaín M, Close TJ, Wise RP, Schulman AH, Himmelbach A, Mayer KFX, Scholz U, Poland JA, Stein N, Waugh R (2013) Anchoring and ordering NGS contig assemblies by population sequencing (POPSEQ). Plant J 76:718–727
Mather DE (2002) Explorations with barley genome maps: quantitative genetics, genomics, and plant breeding. In: Kang MS (Ed) New York. pp 101–108
Moskvina V, Schmidt KM (2008) On multiple-testing correction in genome-wide association studies. Genet Epidemiol 32:567–573
Muñoz-Amatriaín M, Moscou MJ, Bhat PR, Svensson JT, Bartoš J, Suchánková P, Šimková H, Endo TR, Fenton RD, Lonardi S, Castillo AM, Chao S, Cistué L, Cuesta-Marcos A, Forrest KL, Hayden MJ, Hayes PM, Horsley RD, Makoto K, Moody D, Sato K, Vallés MP, Wulff BBH, Muehlbauer GJ, Doležel J, Close TJ (2011) An improved consensus linkage map of barley based on flow-sorted chromosomes and single nucleotide polymorphism markers. Plant Genome-US 4:238–239
NDong C, Danyluk J, Wilson KE, Pocock T, Huner NPA, Sarhan F (2002) Cold-regulated cereal chloroplast late embryogenesis abundant-like proteins. Molecular characterization and functional analyses. Plant Physiol 129:1368–1381
Poland JA, Rife TW (2012) Genotyping-by-sequencing for plant breeding and genetics. Plant Genome-US 5:92–102
Pozniak CJ, Clarke JM, Clarke FR (2012) Potential for detection of marker–trait associations in durum wheat using unbalanced, historical phenotypic datasets. Mol Breed 30:1537–1550
Rae SJ, Macaulay M, Ramsay L, Leigh F, Matthews D, O’Sullivan DM, Donini P, Morris PC, Powell W, Marshall DF, Waugh R, Thomas WTB (2007) Molecular barley breeding. Euphytica 158:295–303
Rollins JA, Drosse B, Mulki MA, Grando S, Baum M, Singh M, Ceccarelli S, von Korff M (2013) Variation at the vernalization genes Vrn-H1 and Vrn-H2 determines growth and yield stability in barley (Hordeum vulgare) grown under dryland conditions in Syria. Theor Appl Genet. doi:10.1007/s00122-013-2173-y
Romay MC, Butrón A, Ordás A, Revilla P, Ordás B (2012) Effect of recurrent selection on the genetic structure of two broad-based Spanish maize populations. Crop Sci 52:1493–1502
Roy JK, Smith KP, Muehlbauer GJ, Chao S, Close TJ, Steffenson BJ (2010) Association mapping of spot blotch resistance in wild barley. Mol Breed 26:243–256
Russell JR, Ellis RP, Thomas WTB, Waugh R, Provan J, Booth A, Lawrence P, Young G, Powell W (2000) A retrospective analysis of spring barley germplasm development from ‘foundation genotypes’ to currently successful cultivars. Mol Breed 6:553–568
Saghai-Maroof MA, Biyashev RM, Yang GP, Zhang Q, Allard RW (1994) Extraordinarily polymorphic microsatellite DNA in barley: species diversity, chromosomal locations, and population dynamics. Proc Natl Acad Sci USA 91:5466–5470
Silvar C, Dhif H, Igartua E, Kopahnke D, Gracia MP, Lasa JM, Ordon F, Casas AM (2010) Identification of quantitative trait loci for resistance to powdery mildew in a Spanish barley landrace. Mol Breed 25:581–592
Straub PF, Shen Q, Ho THD (1994) Structure and promoter analysis of an ABA- and stress-regulated barley gene, HVA1. Plant Mol Biol 26:617–630
Stuber CW, Moll RH (1972) Frequency changes of isozyme alleles in a selection experiment for grain yield in maize (Zea mays L.). Crop Sci 12:337–340
Stuber CW, Moll RH, Goodman MM, Schaffer HE, Weir BS (1980) Allozyme frequency changes associated with selection for increased grain yield in maize (Zea mays L.). Genetics 95:225–236
Stuber CW, Lincoln SE, Wolff DW, Helentjaris T, Lander ES (1992) Identification of genetic factors contributing to heterosis in a hybrid from two elite maize inbred lines using molecular markers. Genetics 132:823–839
Turner A, Beales J, Faure S, Dunford RP, Laurie DA (2005) The pseudo-response regulator Ppd-H1 provides adaptation to photoperiod in barley. Science 310:1031–1034
Varshney RK, Marcel TC, Ramsay L, Russell J, Röder MS, Stein N, Waugh R, Langridge P, Niks RE, Graner A (2007a) A high density barley microsatellite consensus map with 775 SSR loci. Theor Appl Genet 114:1091–1103
Varshney RK, Langridge P, Graner A (2007b) Application of genomics to molecular breeding of wheat and barley. Adv Genet 58:121–155
von Korff M, Grando S, This D, Baum M, Ceccarelli S (2008) Quantitative trait loci (QTL) associated with agronomic performance of barley under drought. Theor Appl Genet 117:653–669
Wiener P, Edriss MA, Williams JL, Waddington D, Law A, Woolliams JA, Gutiérrez-Gil B (2011) Information content in genome-wide scans: concordance between patterns of genetic differentiation and linkage mapping associations. BMC Genom 12:65
Wisser RJ, Murray SC, Kolkman JM, Ceballos H, Nelson RJ (2008) Selection mapping of loci for quantitative disease resistance in a diverse maize population. Genetics 180:583–599
Wisser RJ, Balint-Kurti PJ, Holland JB (2011) A novel genetic framework for studying response to artificial selection. Plant Genet Resour 9(2):281–283
Wu TD, Watanabe CK (2005) GMAP: a genomic mapping and alignment program for mRNA and EST sequences. Bioinformatics 21:1859–1875
Zhan H, Xu S (2011) Generalized linear mixed model for segregation distortion analysis. BMC Genet 12:97
Acknowledgments
This work was supported by the Spanish Ministry of Science and Innovation (MICINN), who funded this work with the scholarship BES-2008-009623 (EM), and the projects AGL2010-21929, RTA03-028-C4, RTA2006-00020-C04 and RTA2009-00006-C04, and by Grant A06 from the Aragon Government. Genotype by sequencing was funded by DGA—Obra Social La Caixa (Grant Number GA-LC-059-2011). WTBT acknowledges funding by the Scottish Government’s Rural and Environment Science and Analytical Services Division.
Author information
Authors and Affiliations
Corresponding author
Electronic supplementary material
Below is the link to the electronic supplementary material.
Rights and permissions
About this article
Cite this article
Igartua, E., Mansour, E., Cantalapiedra, C.P. et al. Selection footprints in barley breeding lines detected by combining genotyping-by-sequencing with reference genome information. Mol Breeding 35, 11 (2015). https://doi.org/10.1007/s11032-015-0194-8
Received:
Accepted:
Published:
DOI: https://doi.org/10.1007/s11032-015-0194-8