Abstract
The retrieval of stable and reliable empirical Green’s functions using ambient seismic noise plays a major role when studying the Earth’s structure at various scales. High-resolution noise correlation functions are obtained in the NW of Iran by processing techniques including dividing the continuously recorded raw data into short (i.e., 10 min) overlapping (i.e., 80%) time windows. We compare four stacking methods (i.e., linear, RMS, RMS ratio, and Nth-root stacking methods) to study robust and stable inter-station empirical Green’s functions. Our results indicate that the new RMS ratio method of stacking would be the optimal method to stack coherent signals. In other words, this method tackles problems including low signal-to-noise ratio (hereafter SNR) value, distortion of wave shape, and phase instability/unstable polarity treatment. In addition to noise correlation functions, we propose another strategy for the computation of the empirical Green’s functions. In this technique, the cross-correlation of scattered coda waves of the calculated noise correlation functions is performed individually. In addition to coda window length, we also investigate another effective parameter, the geometry of various virtual stations to obtain reliable empirical Green’s functions from the scattered coda waves of correlation functions with high SNR. The error of the velocities of Rayleigh wave empirical Green’s functions is on the order of approximately 0.6%, when compared to ambient seismic noise and scattered coda waves for a period band range of 3–10 s.
Similar content being viewed by others
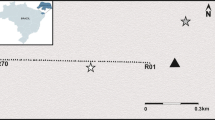
Avoid common mistakes on your manuscript.
1 Introduction
In recent years, the extensive increase of seismic tomographic studies led to the development of a powerful method to study the Earth’s structures, i.e., the ambient seismic noise method. Many theoretical studies (e.g., Weaver and Lobkis 2001; Wapenaar 2004; Snieder 2004; Roux et al. 2005; Gouédard et al. 2008) illustrated that the correlation of a continuous random wavefield passing through two receivers at the same time recording will result in inter-station empirical Green’s functions (hereafter EGFs). Some of the most important features of this method can be summarized as ease of operation, propagation in a wide frequency range, and independency from seismic sources in addition to repeatability (e.g., Shapiro and Campillo 2004). The ambient seismic noise method also plays a major role in recovering subsurface structures especially in aseismic regions where traditional passive earthquake tomography or seismic exploration methods are not applicable for unraveling the Earth’s structure (Shapiro and Campillo 2004; Prieto et al. 2009). Because surface waves are dominant in the ambient seismic noise wavefield, the ambient seismic noise method has been widely used in surface wave studies, such as travel time tomography to produce phase and group velocity maps at different scales (Shapiro et al. 2005; Yang et al. 2007; Stehly et al. 2009; Li et al. 2013; Brandmayr and Vlahovic 2016; Lehujeur et al. 2017). Many researchers have also applied the ambient seismic noise method in various research fields such as detecting different environmental (i.e., petroleum, geothermal, hydrothermal) reservoirs (Mordret et al. 2013; Bakulin et al. 2007; Lehujeur et al. 2015, 2017; Spica et al. 2015), volcano studies (Sens-Schönfelder and Wegler 2006; Brenguier et al. 2008; Obermann et al. 2013; Shomali and Shirzad 2015), landslide monitoring (Renalier et al. 2010; Mainsant et al. 2012), retrieving body waves at various inter-station distances (Poli et al. 2012; Lin et al. 2013; Shirzad and Shomali 2014), and studies of fault planes (Shirzad et al. 2017). Due to the uneven distribution of seismic noise sources, retrieving different lags of EGFs is strongly biased by azimuthal inhomogeneities of the energy flux (Stehly et al. 2006; Pedersen et al. 2007; Froment et al. 2010; Landès et al. 2010). Furthermore, various parameters including quality of data, processing techniques, different stacking algorithms, frequency content of signals, time window length, and overlap percentage influence the quality of the extracted EGF signals (e.g., Seats et al. 2012; Shirzad and Shomali 2015).
Campillo and Paul (2003) indicated that extracting coherent surface wave information would be possible using coda waves generated by earthquakes along large distances within Mexico. In the actual Earth, distribution of sources of the ambient seismic noise is not uniform around station pairs. Therefore, stability and quality of retrieved inter-station EGFs clearly depend on the particular directions that energy flux comes. Scattered coda waves, sampled randomly and repeatedly as parts of wave propagations, are similar to ambient seismic noise. Therefore, scattered coda waves contain valuable information about the propagation properties of the media to solve the azimuthal distribution of energy flux problem. For instance, many researchers (e.g., Spica et al. 2016; Ma and Beroza 2012; Stehly et al. 2008) extracted EGFs from scattered coda wave windows of noise correlation functions (hereafter NCFs).
In this paper, we present how stable and reliable inter-station EGFs (hereafter optimum EGFs) can be extracted in a regional study case using ambient seismic noise and scattered coda waves of the NCFs. Therefore, the effect of various effective parameters such as time window length is first considered, and four stacking methods are then investigated, e.g., linear (hereafter LIN; Bensen et al. 2007), root-mean-square (hereafter RMS), root-mean-square-ratio (hereafter RMS-R), and Nth-root (hereafter NTH; McFadden et al. 1986) stacking methods. Finally, the obtained EGFs from the ambient seismic noise (hereafter C1) are compared with those from the scattered coda waves of the C1 (hereafter C3, i.e., correlation of coda of correlations; Stehly et al. 2008) at similar distances.
2 Data and methods
2.1 Study area and dataset
The Azerbaijan region, NW Iran, is located at the border of Turkey, Armenia and Azerbaijan in the northern part of the Zagros region, Iran. It is also located in the northwestern Alborz Mountains Belt, Iran (Fig. 1a). The study area includes two active volcanoes, Sahand and Sabalan volcanoes, and also some active faults where the catastrophic Ahar–Varzaqan earthquake (Mw 6.5) occurred on Aug. 11, 2012.
a Map shows the study area and stations used (gray triangles). Solid black polygon depicts some important active faults in the Azerbaijan area. The location of the Ahar–Varzaqan earthquake that occurred on Aug. 11, 2012 (Mw 6.5) is denoted by the black star. Histogram in the left bottom shows available data for each station during Dec. 2011 to Dec. 2012. b Top panel shows a 10min period of continuous data including a microearthquake with Nuttli magnitude (Nuttli 1973) MN 2.5, which was recorded at TBZ station on Sep. 28, 2012 (raw data). Middle panel shows one-bit normalization result. Bottom panel illustrates running absolute mean normalization, whereby the waveform was normalized by running absolute mean within a 50-s time window at the center of each sample (Bensen et al. 2007). In the top panel is shown a 40-s window around the microearthquake shown in the two bottom panels
In this study, we processed the vertical component of continuous seismic noise (data) recorded at seven stations with inter-station distances ranging between 47 and 198 km, depicted in Fig. 1a. These stations, as a part of the permanent array of the Iranian Seismological Center (IrSC), are equipped with short period sensors (Kinemetrics SS-1), and the continuous data were recorded with 50 samples per second (sps). The data used in this study was collected over approximately 13 months from December 2011 to December 2012. However, some of the studied stations recorded data for only a few months (e.g., see Fig. 1a, AZR). The data availability for each station is depicted as a histogram in the left bottom panel of Fig. 1a.
2.2 The correlation methods
2.2.1 C1 method
Seats et al. (2012) showed that signal-to-noise ratio (SNR) of inter-station EGFs was enhanced using overlapping and shortening of the time window compared to the classical common low-frequency processing technique outlined by Bensen et al. (2007). Notably, the SNR value was defined as a ratio of the maximum peak of the envelope within expected signal windows to the RMS value in noise windows (Bensen et al. 2007). In this study in order to obtain the optimum shortened time window (hereafter STW) length, continuous raw data were first divided into five STWs: 10, 15, 20, 30, and 60 min. All possible combinations of matched sensor types were then used for further processing in order to avoid instrument response correction (e.g., Cho et al. 2007). After removing the mean and trend, we applied band-pass filtering between 0.1 and 0.33 Hz and then decimating all STW to 10 sps. To suppress the predominance of earthquake signals, human activities, and the existence of non-stationary sources with high amplitudes on calculated signals, we also considered time domain normalization of STW (Bensen et al. 2007; Lin et al. 2008). The results were thus separately investigated for the raw data (without time domain normalization; Cupillard et al. 2011), one-bit normalization (all positive amplitudes = + 1; all negative amplitudes = − 1), and running absolute mean (Bensen et al. 2007) normalization. Figure 1b shows 10 min time windows on Sep. 28, 2012, including raw data (top panel), one-bit (middle panel), and running absolute mean (bottom panel) normalizations in the period band of 3–10 s. We also applied frequency domain whitening normalization for each of the three groups of the time domain normalized dataset, separately. Finally, to obtain NCFs, all prepared STW were correlated with similar normalization techniques (e.g., one-bit, running absolute mean) for all possible station pairs with 80% overlapping length (Seats et al. 2012).
2.2.2 C3 method
In general, the energy flux of ambient seismic noise is non-uniformly distributed (Froment et al. 2010; Weaver et al. 2009), and it is dominated by some particular azimuthal directions. Thus, to reconstruct Rayleigh wave inter-station EGFs, Stehly et al. (2008) suggested that taking a temporary third station, which is termed a virtual station, overcomes these azimuthal energy directions. As a rule of thumb, after preparing single station raw data (Sect. 2.2.1), cross-correlations between both stations and virtual stations were carried out and the results termed NC1Fs. Afterwards, the coda wave of calculated NC1Fs were cross-correlated, which is termed the C3 method, to calculate NC3Fs (Safarkhani and Shirzad 2017; Sheng et al. 2018). It should be noted that the number within NCF hereafter refers to the cross-correlation method.
In this study, we fixed the beginning of the coda wave window (hereafter CWW) equal to two times the Rayleigh wave travel time in NC1Fs. To determine the optimal CWW length, we selected different window lengths of 120, 180, 230, and 280 s for each NC1F (10 min NC1F; see Sect. 3.2). In this study, coda correlation functions were restricted to the pairs recorded at the same time. Similar to the C1 method, EGFs from different CWW lengths were then calculated using two types of time domain normalizations, one-bit and running absolute mean.
2.3 The stacking methods
In this study, extraction of inter-station EGFs based on RMS-R stacking was included according to the following major steps. In the first step, the ratio of RMS values within the signal windows to zero lag (Pedersen et al. (2007); from zero to start of the signal window) was computed for all NCFs for three individual time domain normalization groups. In brief, we calculated this value as RMS-R = RMSexpected_signal_window/RMSzero_lag. Then, individual RMS curves were formed by sorting the calculated RMS-R values. Notably, RMS curves of the corresponding first 7000 NCFs retrieved by three individual time domain normalization groups are depicted in Fig. 2 for station pair TBZ–MRD. The threshold value, which is specified as the number of NCFs used in the stacking procedure, is defined based on the change in gradient (second derivative) of the RMS curve. Although the RMS value of NCFs may extremely differ in the beginning, our investigations indicated that changing gradient of RMS curves is almost similar for all station pairs in the study area which can be explained by the distribution of background energy flux in the study area. In the current study, threshold values were determined to be on the order of 3500 for 10 min time windows (NC1F) in all station pairs. In simple terms, in the RMS-R stacking method, 3500 NC1Fs (for a 10min time window) were selected for further processing which is depicted by different colors in Fig. 2 for the three groups of time domain normalization: raw data (blue), one-bit (black), and running absolute mean (red) normalization. The selection process makes an important distinction between RMS-R stacking and classical type of RMS value stacking (e.g., RMS stacking) method. For investigating NC1F selection, Shirzad and Shomali (2013) showed that the quality of EGFs was enhanced using stacking of non-consecutive signal windows with high RMS values. In a variation of RMS-R stacking procedure, the incoherent NCFs are rejected by two individual conditions. The first condition includes the number of NCF (i.e., 3500 in Fig. 2) with large RMS-R values that correspond to a change in gradient of the RMS curve. The second condition is an enhancement of the SNR values of the calculated signal that ensured the measurement results to agree with one another. In conclusion, less similar non-consecutive NCFs (rejecting by both conditions) are avoided in the RMS-R stacking method, and well-correlated NCFs with the highest RMS-R value within the signal window are exactly used.
RMS curves illustrating the RMS value ratio (RMSexpected_signal_window/RMSzero_lag) of individual NCFs in descending order versus the first 7000 NCFs for three category time domain normalizations. The threshold value (first 3500 NCFs) is depicted by different colors (raw with blue, running absolute mean normalization with red, one-bit with black)
We applied the NTH stacking (Kanasewich et al. 1973; McFadden et al. 1986) method for the selected 3500 NC1Fs. In the NTH stack, the mean of the NTH of each NC1F is raised to the Nth power while preserving the actual sign of each sample. Small coherent signals can be extracted more efficiently by the NTH stacking method than by the classical LIN stacking algorithm (McFadden et al. 1986). In addition, this stacking method is generally a non-linear procedure, which enhanced the SNR of signals at the expense of reduced background noise. In order to suppress incoherent energy, the NTH stacking procedure is preferable to the LIN stacking method (McFadden et al. 1986).
3 Results
3.1 Comparing different stacking methods
The LIN (Bensen et al. 2007), RMS-R, and NTH (McFadden et al. 1986) stacking methods were applied for selected 3500 NC1Fs which are calculated for three individual time domain normalization groups. Retrieved signals using LIN, NTH stacking, and RMS-R stacking methods are depicted in Fig. 3a, b, and c, respectively for station pair TBZ–MRD (inter-station distance ~ 66 km) in the period range of 3–10 s. According to the average group velocity of Rayleigh waves and inter-station distances, the signal window for each inter-station EGF was defined within 20 s around the expected arrival time of the fundamental mode Rayleigh waves (gray columns in Fig. 2) at a period band of 3–10 s. The corresponding RMS value of the calculated signal in stacking processing was, in fact, proportional to enhance SNR value as shown in the bottom panel of Fig. 3a, b, and c (e.g., Shirzad and Shomali 2013). The SNR curves were computed for LIN, NTH and RMS-R stacking in the bottom panel of Fig. 3a, b, and c. Concerning the NTH stacking method, the power (N value) depends on some parameters such as noise level and the SNR of signals (McFadden et al. 1987). Consequently, in this study, we choose N = 3 after testing several values.
The result of different stacking methods using three time domain normalization techniques: a raw data, b one-bit, and (c) running absolute mean normalization for station pair TBZ–MRD (inter-station distance ~ 66 km) in the period band of 3–10 s. For each panel, each signal corresponds to the result which is produced by linear (LIN) stacking of NCFs (light gray), application of Nth-root (NTH) method using LIN stacking with N = 3 (red), and RMS ratio (RMS-R) stacking method (black). Gray shadow columns show the expected signal window used in the calculation of RMS values. Bottom panels show the enhancement of SNR values of calculated signal ensured the measurement results to agree with one another. The horizontal axes of these panels indicate the number of NCFs used in the stacking for three stacking methods (LIN with light gray, NTH with red, RMS-R stacking with black colors)
To retrieve inter-station EGFs in a homogenous medium, we require a closed surface of sources which are surrounded by the receivers (Snieder 2004). All sources located in the Fresnel zone (sources on or near a line that passes through the station pair; stationary sources) affect the definition of the inter-station EGFs (Wapenaar et al. 2010). The adverse effects of non-stationary sources (all sources outside of the Fresnel zone) for retrieving inter-station EGFs are not canceled when the source distribution is incomplete/non-uniform. According to occur more than 800 earthquakes in the study area, and more than 10 large earthquakes around the world at the same time the ambient seismic noise was recorded, some denoising (e.g., Baig et al. 2009) and cutting with strong energies (e.g., Poli et al. 2013; Zigone et al. 2015; Seats et al. 2012) methods may be not appropriate methods to obtain optimum EGFs. Because the non-diffuse earthquake’s coda wave energy could affect directly on retrieved inter-station EGFs. Therefore, in addition to denoising the deterministic signals (e.g., earthquakes), stationary and non-stationary signals should be considered when the distribution of sources would be not uniform. Therefore, the interferometry methods (e.g., cross-correlation of ambient seismic noise) require a stacking process that uses non-consecutive NC1Fs calculated from stationary sources and avoids the non-consecutive NC1Fs calculated from non-stationary sources.
To retrieve optimum EGFs, some studies (e.g., Melo et al. 2013) applied the Eigen vectors and Eigen values using a calculated NC1F matrix based on the singular value decomposition problem. However, the RMS stacking method, which is based on energy within a signal window (energy of stationary sources), was originally developed by Picozzi et al. (2009) for very high frequency data (i.e., 5–14 Hz). Shirzad and Shomali (2013) further extended this method with three main constraints. First, the stacking process is stopped automatically when the number of noise cross-correlation functions (NC1Fs) used in the stacking process becomes greater than a given threshold value. The second constraint is that the measurement results must agree with one another. Finally, RMS stacking was applied for each phase (i.e., surface or body waves) separately, based on a signal window defined around each phase (Shirzad and Shomali 2014, 2015). Although Shirzad and Shomali (2013) obtained inter-station EGFs with high SNR value using these constraints rather than the results of the classical LIN method, the phase of the extracted EGFs was not discussed.
In order to extract inter-station EGFs using the RMS stacking outlined by Shirzad and Shomali (2013), we repeated all EGF retrieval steps including pre-processing (e.g., remove mean and trend, time and frequency domain normalization), cross-correlation, calculating RMS value within the expected signal window of NCFs, sorting calculated RMS values and then forming the RMS curve, defining the threshold value, and stacking. The inter-station EGFs of 10, 15, 20, 30, and 60 min time windows retrieved using the RMS stacking method are shown in Fig. 4 for the TBZ–MRD station pair. In this figure, a comparison of extracted inter-station EGF using RMS stacking (red) and RMS-R stacking (black) methods for similar time window lengths indicates that the polarity of inter-station EGFs gets flipped in different signals obtained using RMS stacking (e.g., 10 and 60 min time windows). In addition, we face incomplete retrieval of EGFs in some time window lengths (e.g., 15 and 30 min time windows) by the RMS stacking method.
3.2 Results in terms of length of correlation
In order to investigate the effect of time window length on stability and reliability of the extracted EGFs using RMS-R stacking, we repeated all EGF retrieval steps, e.g., pre-processing, cross-correlation, and stacking to obtain individual time window EGFs for the 3–10 s period range for different time window lengths, i.e., 10, 15, 20, 30, and 60 min. Figure 5a shows the extracted EGFs of station pair TBZ–MRD calculated using different time window lengths based on one-bit (black) and running absolute mean (red) time domain normalizations. The corresponding SNR increment curves of corresponding retrieved EGF signals, which are calculated by one-bit and running absolute mean normalization, are also depicted in the bottom and upper panels of Fig. 5b, respectively. The SNR increment curve (Fig. 5b) showed that by stacking a similar number of NC1Fs, which are used in the RMS-R stacking, the retrieved EGF signals resulting from 10 min time windows have larger SNR values compared to other time window lengths. Accordingly, the 10min time windows are considered as basis NC1Fs in order to construct CWWs (NC3Fs in C3 method). Although the non-equality number of NCFs for different time windows may have a debate in retrieved EGFs, the number of excited sources will not be the same, if NCFs of time windows are checked with the same number. For example, if the same number of NCFs is used for stacking procedure, thus the number of sources excited for 10 min time window is 6 times less than the sources excited for the 60 min time window. In this study, we assumed that the number of sources is constant during the continuous data recorded for all time windows.
a Results from the C1 method corresponding to station pair TBZ–MRD using different time window lengths (10, 15, 20, 30, and 60 min) in the period band of 3–10 s. EGFs resulted from two time domain normalization techniques: running absolute mean (red) and one-bit (black) normalization. Raw data technique was not shown because of the low SNR value. b SNR increment versus number of NC1Fs for different window lengths in one-bit (bottom panel) and running absolute mean (top panel) normalizations. c The EGFs from the C3 method are depicted for different coda wave window (CWW) lengths (120, 180, 230, 280 s) using one-bit (black) and running absolute mean (red) time domain normalization for station pair SHB–TBZ–MRD (SHB is the virtual station). d SNR curves versus number of coda correlation functions for different CWW lengths in one-bit (bottom panel) and running absolute mean (top panel) normalizations. Gray shadow columns show the expected signal windows
In accordance with the RMS-R stacking, we retrieved inter-station EGFs from calculated NC3Fs. Figure 5c shows the extracted EGFs using the RMS-R stacking method of NC3Fs (C3 or CWWs of the 10 min time window from C1 method) for various time window lengths for the TBZ–MRD station pair with SHB as the virtual station (Sect. 3.3) in the period band of 3–10 s. SNR increment curves of the extracted EGFs with various time window lengths during RMS-R stacking, which correspond to one-bit and running absolute mean normalization, can also be observed in the bottom and upper panels of Fig. 5d, respectively. The optimal CWW length was then selected as the one which produces large SNR values. Furthermore, considering the time window length in the C3 method, it can be seen that the SNR curve for the 180 s CWW length is larger than the other CWW lengths (Fig. 5d). The results of comparing different time window lengths in C1 and C3 methods are illustrated in Table 1.
3.3 Influence of array geometry of various virtual stations on retrieving EGFs using the C3 method
The effects of station geometry used in the C3 method were investigated for the TBZ–MRD station pair. Figure 6a shows the extracted EGFs of station pair TBZ–MRD for various virtual stations (AZR, BST, HRS, SHB, and SRB). The inter-station distances and azimuth between various virtual stations and station pair TBZ–MRD are listed in the Table 2. However, it is more suitable to compare the array geometry when other possible effects stay the same, e.g., data availability. Thus, the cross-correlation operator runs for common time recorded in all stations. It should be noted that the signal shown in the bottom of Fig. 6a depicts the retrieved signal using the C1 method (10 min NC1Fs) from the same available data as used for the C3 results. We also compared the travel time of maximum energy associated with the extracted EGFs using the C3 and C1 methods within the signal window. Figure 6b and c show the overlay of negative and positive lag times for extracted EGFs (upper panels) and the corresponding envelope (bottom panels) within the signal window, respectively. In the ideal case, when the distribution of energy is completely uniform around station pair, all signals (energy) emanated from sources outside the Fresnel zone (non-stationary zone) were canceled out using simply LIN stacking, whereas the non-stationary energy will not be completely canceled when the distribution of energy is non-uniform in the study area and consequently will appear as an artifact in retrieved EGFs. Based on Fig. 6b and c, the relative values in error velocities corresponding to EGFs with virtual stations AZR, BST, HRS, SHB, and SRB and inter-station EGF extracted using the C1 method are approximately 2%, 0.9%, 0.6%, 0.5%, and 0.5% approximately. The error velocities for extracted virtual stations C3 EGFs suggest that the retrieved signals are fairly agreement/fit with C1 EGF signal except for the AZR station may be due to the insufficient data recorded time. Our investigations show that in most virtual station signals, the error velocity is less than approximately 0.6% which is in the range of the dispersion measurement error bars calculated for surface wave tomographic procedure. This error can cause/excite by the different number of non-consecutive NCFs, which are in agreement together in stacking procedure and/or multi-path traveling of surface wave (Capon 1970; Bungum and Capon 1974; Ji et al. 2005; Xia et al. 2018).
a Influence of array geometry on the resulting signals/EGFs from the C3 method for different virtual stations (AZR, BST, HRS, SHB, SRB). Bottom trace depicts EGF from the C1 method for station pair TBZ–MRD (with inter-station distance ~ 66 km) in the period range 3–10 s. Right-hand panels show geometry of the network. Overlay and envelope of EGFs from C1 and C3 are shown for positive (b) and negative (c) lag times. Gray shadow columns show the expected signal windows
4 Discussion
4.1 Optimal stacking method
The main purpose of this study is the extraction of optimal EGFs applied on the IrSC dataset recorded in the Azerbaijan region, NW Iran. In this regard, we investigated the extraction of EGFs using ambient seismic noise for various time window lengths, different time domain normalization techniques, and stacking methods alongside improving the RMS stacking method. Comparing the extracted EGF signals using various stacking approaches indicated that the quality and SNR of retrieved EGF signals using RMS-R stacking are significantly greater (approximately two times) than the SNR of retrieved EGFs using the LIN stacking method. Besides, in comparison with NTH stacking, although the SNR of retrieved EGFs are of lower order, RMS-R stacking can retrieve signals without any sharpening and distortion in the frequency content (shape of waveform; McFadden et al. 1986). We evaluated the correlation coefficients of the retrieved EGF signals with various time domain normalizations. The corresponding correlation coefficients were in the same order; thus, we decided to compare SNR increment curves as a measure to obtain the optimal inter-station EGFs. Inspection of SNR increment curves during the stacking procedure shows that the corresponding stable signals emerge and appear 1.1 times faster for the one-bit time domain normalization pre-processing method than for the running absolute mean normalization pre-processing technique (bottom panel of Figs. 3a, b and 5b, d). Although this result may not be significant for an array with few stations (e.g., the seven stations of this study), it would be appropriate/adequate for an array with many station pairs. High SNR values of EGF signals would be possible using a 10 min STW and 180 s CWW length in the C1 and C3 methods (Fig. 5b, d). Notably, the combination of the inter-station distance and the degree of the heterogeneity in seismic structure (i.e., weak, strong scattering) could affect the STW and CWW length for the C1 and C3 method, respectively.
4.2 The polarity issue
In this study, instead of the RMS stacking method (Shirzad and Shomali 2013), we improved and applied the RMS-R stacking method. The RMS stacking method retrieves inter-station EGFs using a comparison of stationary source energy (RMS value within expected signal window), whereas the RMS-R stacking method considers the ratio of stationary source energy to non-stationary source energy (RMS value within zero lag). As shown in Fig. 4, although the envelopes of the retrieved inter-station EGFs have consistent peak times with both stacking methods, the phases of inter-station EGFs retrieved using RMS stacking are unstable for different time window lengths.
4.3 Comparison of the delay times between C3 functions
Considering different virtual stations, the variety of traveled paths by Rayleigh waves would increase. With regard to surface/Rayleigh waves, multi-pathing due to crustal heterogeneities (Capon 1970; Bungum and Capon 1974; Ji et al. 2005; Xia et al. 2018) may arrive to the virtual stations by different paths at different arrival times. Investigation of the geometry of the virtual station with respect to the other stations used in C3 application indicates that the error of velocities corresponding to extracted EGFs is on the order of 0.6%. Because of small variations in travel time differences, the SHB station was used as a virtual station in the study area. Notably, the SHB station has maximum data availability (the bottom-left panel of Fig. 1a) and is located almost at the center of the study seismic network.
The extracted EGFs of all possible station pairs versus the inter-station distances for the C1 and C3 methods are depicted in Fig. 7, wherein the gray lines indicate velocity range of Rayleigh waves between 1.5 and 2.7 km/s.
5 Conclusions
We retrieved the EGFs of both ambient seismic noise (C1) and scattered coda waves (C3) in the Azerbaijan region (NW of Iran) within the period band of 3–10 s. Our results are summarized below:
-
(1)
Segmenting raw data and also the accuracy of signal window determination are necessary conditions to enhance SNR of extracted EGFs, but not sufficient to retrieve reliable and stable EGFs. In addition to these constraints, the ratio of stationary source energy to non-stationary source energy is a key factor to retrieve stable inter-station EGFs.
-
(2)
Comparing the extracted EGF signals using the RMS-R stacking method and LIN stacking method indicated that the quality and SNR of retrieved EGFs using RMS-R stacking (approximately 24) are significantly greater than the SNR of retrieved EGFs using the LIN stacking method (approximately 12; Fig. 3). Although this method can be used in some cases including little data availability and short temporary deployments data, the data recording duration should be such that at least a few stationary NCFs are existent in stacking procedure.
-
(3)
Geometry investigation of the virtual station with respect to other stations used in the C3 approach indicated that travel time differences for various virtual stations with extracted inter-station EGFs using the C1 method are negligible. Also, the error of velocity differences for various virtual stations is on the order of 0.6%. Thereby, the information of unbiased travel time measurement can result in tomography maps much closer to the real Earth.
-
(4)
The accuracy of estimating arrival times of Rayleigh wave parts of extracted EGFs in this study is improved by optimal processing technique (e.g., RMS-R stacking). Furthermore, in terms of retrieved EGF signal quality to processing time ratio (including single station preparation, cross-correlation for common recorded times, and stacking), the C3 method would be not very higher than the C1 one.
References
Baig AM, Campillo M, Brenguier F (2009) Denoising seismic noise cross correlations. J Geophys Res 114 , B08310. https://doi.org/10.1029/2008JB006085
Bakulin A, Mateeva A, Mehta K, Jorgensen P, Ferrandis J, Shina Herold I, Lopez J (2007) Virtual source applications to imaging and reservoir monitoring. Lead Edge 26:732–740
Bensen GD, Ritzwoller MH, Barmin MP, Levshin AL, Lin F, Moschetti MP, Shapiro NM, Yang Y (2007) Processing seismic ambient noise data to obtain reliable broad-band surface wave dispersion measurements. Geophys J Int 169:1239–1260
Brandmayr E, Vlahovic G (2016) The upper crust of the Eastern Tennessee Seismic Zone: insights from potential fields inversion. Tectonophysics 688:148–156. https://doi.org/10.1016/j.tecto.2016.09.035
Brenguier F, Shapiro NM, Campillo M, Ferrazzini V, Duputel Z, Coutant O, Nercessian A (2008) Towards forecasting volcanic eruptions using seismic noise. Nat Geosci 1:126–130. https://doi.org/10.1038/ngeo104
Bungum H, Capon J (1974) Coda pattern and multipath propagation of Rayleigh waves at NORSAR. Phys Earth Planet Inter 9(2):111–127
Campillo M, Paul A (2003) Long-range correlations in the diffuse seismic coda. Science 299:547–549
Capon J (1970) Analysis of Rayleigh-wave multipath propagation at Lasa. Bull Seismol Soc Am 60(5):1701–1731
Cho KH, Herrmann RB, Ammon CJ, Lee K (2007) Imaging the upper crust of the Korean peninsula by surface-wave tomography. Bull Seismol Soc Am 97:198–207
Cupillard P, Stehly L, Romanowicz B (2011) The one-bit noise correlation: a theory based on the concepts of coherent and incoherent noise. Geophys J Int 184:1397–1414. https://doi.org/10.1111/j.1365-246X.2010.04923.x
Froment B, Campillo M, Roux P, Gouédard P, Verdel A, Weaver RL (2010) Estimation of the effect of nonisotropically distributed energy on the apparent arrival time in correlations. Geophysics 75(5):85–93. https://doi.org/10.1190/1.3483102
Gouédard P, Stehly L, Brenguier F, Campillo M, Colin de Verdière Y, Larose E, Margerin L, Roux P, Sánchez-Sesma FJ, Shapiro NM, Weaver RL (2008) Cross-correlation of random fields: mathematical approach and applications. Geophys Prospect 56:375–393
Ji C, Tsuboi S, Komatitsch D, Tromp J (2005) Rayleigh-wave multipathing along the west coast of North America. Bull Seismol Soc Am 95(6):2115–2124
Kanasewich ER, Alpaslan T, Hemmings CD (1973) Nth-root stack nonlinear multichannel filter. Geophysics 38:327–338
Landès M, Hubans F, Shapiro NM, Paul A, Campillo M (2010) Origin of deep ocean microseisms by using teleseismic body waves. J Geophys Res 115:B05302. https://doi.org/10.1029/2009JB006918
Lehujeur M, Vergne J, Schmittbuhl J, Maggi A (2015) Characterization of ambient seismic noise near a deep geothermal reservoir and implications for interferometric methods: a case study in northern Alsace, France. Geothermal Energy 3. https://doi.org/10.1186/s40517-014-0020-2
Lehujeur M, Vergne J, Maggi A, Schmittbuhl J (2017) Ambient noise tomography with non-uniform noise sources and low aperture networks: case study of deep geothermal reservoirs in northern Alsace, France. Geophys J Int 208(1):193–210
Li Y, Wu Q, Pan J, Zhang F, Yu D (2013) An upper-mantle S-wave velocity model for East Asia from Rayleigh wave tomography. Earth Planet Sci Lett 377:367–377
Lin F-C, Moschetti MP, Ritzwoller MH (2008) Surface wave tomography of the Western United States from ambient seismic noise: Rayleigh and love wave phase velocity maps. Geophys J Int 173:281–298
Lin F-C, Tsai VC, Schmandt B, Duputel Z, Zhan Z (2013) Extracting seismic core phases with array interferometry. Geophys Res Lett 40(6):1049–1053. https://doi.org/10.1002/grl.50237
Ma S, Beroza GC (2012) Ambient-field Green’s functions from asynchronous seismic observations. Seismol Res Lett 39. https://doi.org/10.1029/2011GL050755
Mainsant G, Larose E, Bronnimann C, Jongmans D, Michoud C, Jaboyedoff M (2012) Ambient seismic noise monitoring of a clay landslide: toward failure prediction. J Geophys Res 117. https://doi.org/10.1029/2011JF002159
McFadden PL, Drummond BJ, Kravis S (1986) The Nth-root stack: theory, applications, and examples. Geophysics 51:1879–1892
McFadden PL, Drummond BJ, Kravis S (1987) The Nth-root stack: a cheap and effective processing technique. Explor Geophys 18:135–137
Melo G, Malcolm A, Mikesell D, van Wijk K (2013) Using SVD for improved interferometric Green’s function retrieval. Geophys J Int 194:1596–1612. https://doi.org/10.1093/gji/ggt172
Mordret A, Shapiro NM, Singh SC, Roux P, Montagner J-P, Barkved OI (2013) Azimuthal anisotropy at Valhall: the Helmholtz equation approach. Geophys Res Lett 40:2636–2641
Nuttli OW (1973) Seismic wave attenuation and magnitude relations for eastern North America. J Geophys Res 78:876–885
Obermann A, Planès T, Larose E, Campillo M (2013) Imaging presumptive and corruptive structural and mechanical changes of a volcano with ambient seismic noise. J Geophys Res Solid Earth 118:6285–6294. https://doi.org/10.1002/2013JB010399
Pedersen HA, Krüger F, the SVEKALAPKO Seismic Tomography Working Group (2007) Influence of the seismic noise characteristics on noise correlations in the Baltic shield. Geophys J Int 168:197–210
Picozzi M, Parolai S, Bindi D, Strollo A (2009) Characterization of shallow geology by high-frequency seismic noise tomography. Geophys J Int 176:164–174. https://doi.org/10.1111/j.1365-246X.2008.03966.x
Poli P, Pedersen HA, Campillo M, the POLENET/LAPNET Working Group (2013) Noise directivity and group velocity tomography in a region with small velocity contrasts: the northern Baltic shield. Geophys J Int 192(1):413–424
Poli P, Campillo M, Pedersen H, LAPNET Working Group (2012) Body-wave imaging of Earth’s mantle discontinuities from ambient seismic noise. Science 338:1063–1065. https://doi.org/10.1126/science.1228194
Prieto GA, Lawrence JF, Beroza GC (2009) Anelastic Earth structure from the coherency of the ambient seismic field. J Geophys Res 114. https://doi.org/10.1029/2008JB006067
Renalier F, Jongmans D, Campillo M, Bard P-Y (2010) Shear wave velocity imaging of the Avignonet landslide (France) using ambient noise cross correlation. J Geophys Res 115. https://doi.org/10.1029/2009JF001538
Roux P, Sabra KG, Kuperman WA, Roux A (2005) Ambient noise cross correlation in free space: theoretical approach. Acoust Soc Am 117(1):79–84
Safarkhani M, Shirzad T (2017) Investigation of scattered coda correlation functions from noise correlation functions, in retrieving optimized empirical Green’s functions in Azerbaijan Region, Iran. J Earth Space Phys (in Persian with abstract in English) 43(2):323–337. https://doi.org/10.22059/jesphys.2017.60286
Seats JK, Lawrence JF, Prieto AG (2012) Improved ambient noise correlation functions using Welch’s method. Geophys J Int 188:513–523
Sens-Schönfelder C, Wegler U (2006) Passive image interferometry and seasonal variations of seismic velocities at Merapi Volcano, Indonesia. Geophys Res Lett 33, L21302. https://doi.org/10.1029/2006GL027797
Shapiro NM, Campillo M (2004) Emergence of broadband Rayleigh waves from correlations of the ambient seismic noise. Geophys Res Lett 31, L07614. https://doi.org/10.1029/2004GL019491
Shapiro NM, Campillo M, Stehly L, Ritzwoller MH (2005) High-resolution surface-wave tomography from ambient seismic noise. Science 307:1615–1618
Sheng Y, Nakata N, Beroza GC (2018) On the nature of higher-order ambient seismic field correlations. J Geophys Res Solid Earth 123(9):7969–7982. https://doi.org/10.1029/2018JB015937
Shirzad T, Shomali ZH (2013) Shallow crustal structures of the Tehran basin in Iran resolved by ambient noise tomography. Geophys J Int 196:1162–1176. https://doi.org/10.1093/gji/ggt449
Shirzad T, Shomali ZH (2014) Extracting seismic body and Rayleigh waves from the ambient seismic noise using the rms-stacking method. Seismol Res Lett 86(1):173–180. https://doi.org/10.1785/0220140123
Shirzad T, Shomali ZH (2015) Extracting stable seismic core phases from ambient seismic noise. Bull Seismol Soc Am 106(1):307–312. https://doi.org/10.1785/0120150031
Shirzad T, Shomali ZH, Riahi M-A, Jarrahi M (2017) Near surface radial anisotropy in the Rigan area/SE Iran. Tectonophysics 694:23–34. https://doi.org/10.1016/j.tecto.2016.11.036
Shomali ZH, Shirzad T (2015) Crustal structure of Damavand volcano, Iran, from ambient noise and earthquake tomography. J Seismol 19(1):191–200. https://doi.org/10.1007/s10950-014-9458-8
Snieder R (2004) Extracting the Green’s function from the correlation of coda waves: a derivation based on stationary phase. Phys Rev E 69, 046610. https://doi.org/10.1103/PhysRevE.69.046610
Spica Z, Legrand D, Iglesias A, Walter TR, Heimann S, Dahm T, Froger JL, Rémy D, Bonvalot S, West ME, Pardo MH (2015) Hydrothermal and magmatic reservoirs at Lazufre volcanic area, revealed by high-resolution seismic noise tomography. Earth Planet Sci Lett 421:27–38
Spica Z, Perton M, Calò M, Legrand D, Córdoba-Montiel F, Iglesias A (2016) 3-D shear wave velocity model of Mexico and South US: bridging seismic networks with ambient noise cross-correlations (C1) and correlation of coda of correlations (C3). Geophys J Int 206:1795–1813. https://doi.org/10.1093/gji/ggw240
Stehly L, Campillo M, Shapiro NM (2006) A study of the seismic noise from its long-range correlation properties. J Geophys Res 111, B10306. https://doi.org/10.1029/2005JB004237
Stehly L, Campillo M, Froment B, Weaver RL (2008) Reconstructing Green’s function by correlation of the coda of the correlation (C3) of ambient seismic noise. J Geophys Res 113, B11306. https://doi.org/10.1029/2008JB005693
Stehly L, Fry B, Campillo M, Shapiro NM, Guilbert J, Boschi L, Giardini D (2009) Tomography of the Alpine region from observations of seismic ambient noise. Geophys J Int 178(1):338–350
Wapenaar K (2004) Retrieving the elastodynamic Green’s function of an arbitrary inhomogeneous medium by cross correlation. Phys Rev Lett 93, 254301. https://doi.org/10.1103/PhysRevLett.93.254301
Wapenaar K, Draganov D, Snieder R, Campman X, Verdel A (2010) Tutorial on seismic interferometry: part 1—basic principles and applications. Geophysics 75(5):75A195–75A209
Weaver RL, Lobkis OI (2001) Ultrasonics without a source: thermal fluctuation correlations at MHz frequencies. Phys Rev Lett 87(13), 134301. https://doi.org/10.1103/PhysRevLett.87.134301
Weaver R, Froment B, Campillo M (2009) On the correlation of non-isotropically distributed ballistic scalar diffuse waves. J Acoust Soc Am 126(4):1817–1826
Wessel P, Smith WHF (1998) New, improved version of the generic mapping tools released. Eos Trans AGU 79:579
Xia Y, Ni S, Tape C (2018) Multipathing Rayleigh waves from long-distance noise cross correlation along an ocean-continent boundary (Alaska to California). Geophys Res Lett 45:6051–6060
Yang Y, Ritzwoller MH, Levshin AL, Shapiro NM (2007) Ambient noise Rayleigh wave tomography across Europe. Geophys J Int 168:259–274
Zigone D, Ben-Zion Y, Campillo M, Roux P (2015) Seismic tomography of the Southern California plate boundary region from noise-based Rayleigh and Love waves. Pure Appl Geophys 172:1007–1032. https://doi.org/10.1007/s00024-014-0872-1
Acknowledgments
The digital ambient seismic noise dataset has been collected by the Iranian Seismological Center (IrSC) at the University of Tehran/Iran (http://irsc.ut.ac.ir; not openly available to public; last accessed Feb. 2017). The earthquake waveform used in this study was obtained through the IrSC. All plots were also made using Generic Mapping Tools (GMT) version 4 (Wessel and Smith 1998; www.soest.hawaii.edu/gmt, last accessed May 2019). We would also like to thank the editor and four anonymous reviewers for their constructive comments and useful suggestions.
Funding
This work was supported by the (FAPESP), Sao Paulo, Brazil (grant numbers 2016/20952-4).
Author information
Authors and Affiliations
Corresponding author
Additional information
Publisher’s note
Springer Nature remains neutral with regard to jurisdictional claims in published maps and institutional affiliations.
Rights and permissions
About this article
Cite this article
Safarkhani, M., Shirzad, T. Improving C1 and C3 empirical Green’s functions from ambient seismic noise in NW Iran using RMS ratio stacking method. J Seismol 23, 787–799 (2019). https://doi.org/10.1007/s10950-019-09834-1
Received:
Accepted:
Published:
Issue Date:
DOI: https://doi.org/10.1007/s10950-019-09834-1