Abstract
The study of earthquake swarms and their characteristics can improve our understanding of the transient processes that provoke seismic crises. The spatio-temporal process of the energy release is often linked with changes of statistical properties, and thus, seismicity parameters can help to reveal the underlying mechanism in time and space domains. Here, we study the Torreperogil–Sabiote 2012–2013 seismic series (southern Spain), which was relatively long lasting, and it was composed by more than 2000 events. The largest event was a magnitude 3.9 event which occurred on February 5, 2013. It caused slight damages, but it cannot explain the occurrence of the whole seismic crises which was not a typical mainshock–aftershock sequence. To shed some light on this swarm occurrence, we analyze the change of statistical properties during the evolution of the sequence, in particular, related to the magnitude and interevent time distributions. Furthermore, we fit a modified version of the epidemic type aftershock sequence (ETAS) model in order to investigate changes of the background rates and the trigger potential. Our results indicate that the sequence was driven by an aseismic transient stressing rate and that the system passes after the swarm occurrence to a new forcing regime with more typical tectonic characteristics.
Similar content being viewed by others
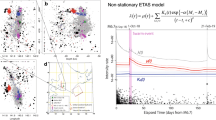
Avoid common mistakes on your manuscript.
1 Introduction
The occurrence of seismic activity in southern Spain is ultimately explained as a consequence of the shortening between the Iberian microplate and the African tectonic plate, which is accommodated over a broad deformation area (Benito and Gaspar-Escribano 2007). Typical mainshock–aftershock sequences and swarm-like seismic series are relatively common in this zone (e.g., Martinez et al. 2005; Rodríguez-Escudero et al. 2014). The particular interest of the Torreperogil–Sabiote 2012–2013 (TS-1213) seismic series lies in the involved significant scientific, social, and media concern.
The seismic series started on October 20, 2012, which lasted for a relatively long period of 8 months, with over 2000 low-magnitude events (−0.1 ≤ M ≤ 3.9). According to the seismic record, this area was considered as a zone of low seismic activity (Cantavella et al. 2013). Some authors (e.g., Pedrera et al. 2013) indicate the presence of basement faults and suggest their activation during the TS-1213 series. The epicentral area was located within the eastern Guadalquivir basin, beneath an elongated ridge known as Loma de Ubeda, between the towns of Torreperogil and Sabiote. A recent study on structural data revealed a previously unknown shear zone including right and left lateral blind faults where their parallel geometry does not promote static triggering (Morales et al. 2015). The shallow and dense distribution of epicenters caused many events to be felt, raising a considerable social concern and a debate about the tectonic or hydro-seismic origin, such as human-made changes in aquifers and reservoirs (Doblas et al. 2014). Morales et al. (2015) discard this idea, associating the initiation of this swarm activity with slow strain release in small but highly fragmented regions under a bending scheme.
Statistical analyses of the seismic series may contribute to gain important insights about their nature because the generation mechanism and the system state changes are often connected to systematic changes of statistical parameters and distributions (e.g., Hainzl and Fischer 2002; Mignan 2012; Schoenball et al. 2015). Especially for cases as southern Spain, where the seismotectonic setting is complex, conclusions can be mainly drawn from statistical analysis of the seismicity patterns.
The main effort of this paper is to investigate the temporal evolution of the seismic series in order to better identify the underlying process. Using a more expanded spatio-temporal overview, we try to discuss this sequence not as an isolated incident, but as a part of a significant growth in the seismic activity in the area. This growth is associated with the occurrence of small clusters since 2010 and within a radius of 30 km to the Torreperogil–Sabiote sequence. We analyze the temporal changes of statistical parameters; in particular, we focus on the b-value and interevent times. We also carry out an epidemic type aftershock sequence (ETAS) modeling of the sequence to unravel the background rate and trigger potential characteristics. Finally, the conclusions of the different statistical observations are brought together and discussed.
2 Data
We analyze the seismic catalog provided by the National Geophysical Institute of Spain (IGN) focusing on a rectangular region centered in the TS-1213 epicentral area within longitude [−3.7, −2.8] and latitude [37.6, 38.4] (box marked in Fig. 1). We start the analysis back in 1980 to explore the study area in a more expanded temporal frame.
The seismic catalog of IGN (M≥1.0) for southern Spain since 1980 is presented in intervals of 10 years in captions a-c. The last temporal interval (d) was chosen until more recent time, end of April 2015. e The spatial distribution of the seismic stations contributing in IGN seismic catalog during the last decade and temporary stations were installed between December 18, 2012 and April 18, 2013. f Zoom into the box-region marked in plots a-e with IGN total data (M≥-0.1) since 2010 to the end of 2014
A relative seismic inactivity is observed from 1980 to 2010. Then, from 2010 up to April 2015, an increase in seismic activity is noted, including the occurrence of the TS-1213 series and other small groups of events to the west and southeast.
The National Seismic Network of IGN, joint with other centers’ stations like the Andalusian Institute of Geophysics (IAG) and conventions of Real Observatory of Armada, Complutense University of Madrid, and German Research Centre for Geosciences (ROA–UCM–GFZ), has localized the seismic activity since its beginning in October 2012. Because of the peculiarity of this series and in order to run a specific study on it, IGN and IAG established additional temporary stations (Fig. 1e) between December 18, 2012, and April 18, 2013, with real-time data transitions (Cantavella et al. 2013).
Apart from the main sequence, four small groups of events are recognizable in time and space while three of them occurred before October 20, 2012. The re-localizations of the main sequence reveal some clustering in the main activity period as well (Peláez et al. 2013; Morales et al. 2015). The occurrence of seismic clusters in the selected frame does not seem usual since diffused seismicity was dominating before in this part of Spain. To explore the potential reasons for this apparent system change, we study in the following sections the statistical properties of this activity. The analyzed data include 2713 events with M ≥−0.1 in the mentioned box region between longitude [−3.7, −2.8] and latitude [37.6, 38.4].
In order to perform a statistical analysis of the seismic series, the first step is to select the subseries which are best suited to characterize the evolution of the plenary incident in time. In this study, we decided to divide the activity into three sub-series in time. The precedent period (phase D1) starts in 2010 and ends just before October 20, 2012, when a sharp increase on the seismic activity indicates the beginning of the main activity phase (phase D2). This lasts until June 30, 2013, when the seismic activity returns to the level of the precedent phase. The subsequent phase (phase D3) lasts until the end of the catalog (Table 1). Figure 3 shows the epicentral locations from 2010.0 to 2015.0, plotting the three phases with different colors. This division provides a suitable term to investigate the changes in statistical properties during and after the sequence. Table 1 provides the details of the time intervals and earthquake numbers of the selected sub-series in Fig. 2. In the following, the statistical properties of the mentioned sub-series are studied (Fig. 3).
Spatial distribution where colors refer to the different temporal intervals: white for pre-activity (Fig. 2) or D1, black for main activity or D2, and gray for post-activity or D3
3 Statistical characterization
The statistical characterization of the TS-1213 series focuses on the time variations of the parameters defining the magnitude–frequency relation (including b-values and magnitude of completeness Mc) and addresses the distribution of interevent times.
3.1 Frequency–Magnitude Distribution
The study of the magnitude frequency distribution is a basic step to characterize any seismic population. Earthquake magnitudes are known to follow generally the Gutenberg–Richter law (Gutenberg and Richter 1956) describing the number of earthquakes with magnitude equal or greater than M as
whereas parameters a and b describe the activity level and the slope of the distribution, respectively. The b-value scatters generally around 1. Experiments of rock samples and observations suggest that the b-value is a “stress meter” where low b-values are indicative of rocks under high stress (Schorlemmer et al. 2005; Scholz 2015). We effectively find a linear relationship between log10(N) and M for magnitudes greater than approximately 1.0 (Fig. 4), which can be fitted by a typical b-value in the order of 1.
However, for b-value estimation, it is essential to firstly determine the magnitude of completeness for the data. For this purpose, we follow the method introduced by Wiemer and Wyss (2000) and apply it to the total activity during 5 years. This was done by calculating the goodness of fit of the Gutenberg–Richter (GR) law to the observed frequency–magnitude distribution as a function of the lower magnitude cutoff. The magnitude Mc at which 95% of the data was modeled by the GR power law is 1.2. For the calculation of a- and b-values of the GR law, we used maximum likelihood estimation for M ≥ Mc.
Applying the same method for detecting potential changes of Mc with time, we select subsequent samples of N events. Considering the lower seismicity in phases D1 and D3, we choose N = 100 events for each sample with an overlap of 10 events. Then, we establish less strict criteria of 90% goodness of fit for data in each sample to be modeled with the GR law.
The result for both D1 and D3 does not oscillate significantly and shows Mc values between 1.2 and 1.3 with σ ∼ 0.1 for 200 bootstrapped samples (Fig. 5). In phase D2 we see a large fluctuation with this sample size. In fact, as during D2 the seismicity rate rises notably, a sample size of 100 covers much shorter time intervals and leads to a less smooth Mc–time curve. On the other hand, establishment of temporary seismic stations between December 18, 2012, and April 18, 2013 (Fig. 1e), enhanced the network capabilities for data detection in quality and quantity. Thus, the capability of detecting smaller magnitude events explains the first drop in Mc values. Then, along with a rise in the occurrence of relatively large magnitudes M ≥ 3.0 since December 2013, the detection ability of the smaller events is likely lowered because of overlapping (and hence inability to spot) of the seismic records of lower-magnitude events occurring immediately after higher-magnitude events, which explains some fluctuations to larger Mc values (Hainzl 2016). However, uncertainties of the Mc values might partially also explain those fluctuations. Increasing the sample size reduces sharp changes but also reduces the temporal resolution. Finally, an Mc value of 1.3 is found to be a reasonable choice for the overall completeness magnitude of the sequence.
Conducting the b-value estimation of events exceeding the time-dependent Mc value (or even a fixed Mc=1.3) results in an evident decrease of the b-value with time. In fact, the result for a sample size of 100 (the same as we used for Mc estimation) indicates b-values of approximately 1.3 in D1 (∼1000 days) followed by a decreasing trend within the high-activity phase from a value over 1.5 to a value of approximately 1.1 in the end and a further decrease toward a value of 0.8 in the D3 phase (Fig. 6). This drop of b-value might result from an increase in stress level that leads to the occurrence of bigger magnitudes. Such process might be the same as the differential stress diminishes at the location of the activity in phase D1 and right before the main sequence where the b-value rises (Scholz 2015). Beside the general b-value decay, larger fluctuations occur on short times which might be related to secondary loading/unloading processes due to stress transfer or pore pressure changes.
Temporal variation of the b-value calculated for sample sizes of 100 events with 10% overlap with three methods: (1) using an Mc that provides 90% goodness of fit for modeling the frequency–magnitude distribution of the data with the GR law (black solid curve) and ±σ (gray solid curves); (2) using an Mc driven from the maximum curvature method (light dashed curve) and ±σ (gray dashed curve); and (3) using a fixed Mc = 1.3 (thick dashed curve). Time interval selection is marked with vertical dashed lines
In Fig. 7, we show the normalized magnitude distribution for each of the three phases. In all cases, the distribution can be well described by the Gutenberg–Richter law with similar slope for D1 and D2, while the slope for D3 is significantly smaller, in agreement with our analysis in moving time windows (Fig. 7).
3.2 Interevent time distribution
For characterizing the temporal occurrence of the events within the seismic sequence, we study the interevent time distribution. Time lag or interevent time τ indicates the time between two consecutive events. Figure 8 visualizes the interevent time distribution versus event index for the 1622 events that occurred in the three phases considered in this study with M ≥ 1.3. Right after October 20, 2012, the interevent time starts to decrease by a factor of almost 102 and remains smaller than 103 in almost the whole duration of the high-activity phase D2.
The interevent time is the most important characteristic of any point process in the time domain and can be quantified by cumulative probability distribution as
where ht(u)du is the probability that the next event after time t occurs between times t + u and t + u + du conditioned on its non-occurrence between times t and t + u. Assuming a zero probability for simultaneous events implies that ht(τ)dτ ≈ λ(t| Ηt)dt with λ(t| Ηt) being the intensity (local event rate) of the process which generally depends on the history Ηt of the preceding events.
In a Poissonian process, the local event rate is independent of the history λ(t), and it becomes a constant value λ in the case of a stationary Poisson process. In this case, the interevent time distribution is
The probability density function of the interevent time follows an exponential distribution:
Here, λ is the long-term average of the event rate. Thus, if the analyzed sequence represents a stationary Poisson process, we would expect to have an exponential distribution of the interevent times. The expected result for the Poisson process is illustrated by the dotted curve in Fig. 9. In comparison, the probability densities of interevent time calculated for the M ≥ 1.3 events in the three phases show no evidence of such exponential tendency.
Tomada (1954) reported a power law distribution of interevent times of the form f(τ) ∝ τ−q, with q = 1 ∼ 2, for some volcanic swarms and aftershock sequences (Utsu et al. 1995). Senshu (1959) interpreted Tomada’s result and showed that for a decaying event rate according to (1/tp), the decay exponent of the interevent time probability density is q = 2 − 1/p.
Densities in Fig. 9 show that during D1 and D3 the probability decays with an almost similar constant power of approximately 0.75, which would relate to a p value of 0.8 in Senshu’s formulation for a single power law decay of the rate. Nevertheless, for limited spatio-temporal windows and superpositions of aftershock sequence and background activity, the interevent time distribution gets more complex (Saichev and Sornette 2007; Lippiello et al. 2012).
This stable power law behavior indicates the similarity of earthquake occurrence in time scale, especially for time lags between 101 and 104 minutes. The observed deviation for small interevent times is likely related to incompleteness, while the bending at large values might be related to finite sample size and observation time. The interevent time distribution for D2 deviates from a stable power law toward a faster decay for time lags bigger than 102 minutes. This is influenced by a higher contribution of events that occurs very closely in time because of a higher degree of clustering.
4 ETAS analysis
One of the most common models for characterizing the clustering of seismicity and understanding the probable source processes is the epidemic type aftershock sequence (ETAS) model, a point process model introduced by Ogata (1988).
This model accounts for activity driven by aseismic processes as well as aftershocks triggered by observed earthquakes. Aftershock occurrences can be well described by the Omori–Utsu law (Utsu et al. 1995) stating that the aftershock rate decays with time t after the mainshock according to
where c and p are constants (see Utsu et al. (1995) for a review). The exponent p is typically in the range 0.8–1.2 and independent of the mainshock magnitude M, whereas K0 is known to depend exponentially on M (Utsu et al. 1995, Hainzl and Marsan 2008). Detailed aftershock studies showed that the delay parameter c is very small, in the order of 1 to several minutes or even less (e.g., Peng et al. 2006; Enescu et al. 2007), while larger estimations often result from incomplete recordings directly after the occurrence of larger earthquakes (Kagan 2004; Hainzl 2016). Note that for single aftershock decay according to the Omori–Utsu law, the probability density function of the interevent times decays with an exponent of 2 − 1/p (see above).
The TS-1213 series is however not dominated by a single mainshock with its aftershocks and consists of several events with similar magnitudes, the highest one in the range between 3.4 and 3.9 (see Fig. 2). Thus, we are dealing with a swarm-like sequence likely attributed to some external aseismic forcing. Aseismic forces contribute to the background seismicity which becomes time-dependent (Hainzl and Ogata 2005), e.g., due to transient creep (such as slow earthquakes) or rapid fluid intrusions (Marsan et al. 2013). In this study, we analyze the temporal behavior of the seismicity using a modification of the ETAS model by Hainzl and Ogata (2005) which provides comprehensive information about time variation of the background rate:
where ti and Mi are the occurrence times and magnitudes of earthquakes. This formulation separates the time-dependent forcing (background) rate μ(t) and the earthquake rate υ(t) related to earthquake–earthquake triggering, where parameters c and p come from the Omori law and K and α are related to the magnitude-dependent aftershock productivity. At any time t since the start of the catalog, the rate of seismicity λ(t) is history-dependent through the term υ(t) which sums the aftershock rate of all the earthquakes that occurred before t with magnitude Mi which is equal or greater than Mc. If the aseismic forcing would be almost constant, then λ(t) could be modeled by aftershock rate υ(t) plus a constant rate μ. But if the background significantly varies with time during the swarm, then a reasonable model fit requires a variant aseismic forcing which should be questioned on its roots.
5 Method
To estimate the model parameters and background rate simultaneously, we apply the algorithm developed by Marsan et al. (2013) and further tested by Hainzl et al. (2013) which is based on the inversion of the temporal ETAS model. This algorithm iteratively estimates the four parameters K , α , c, and p of the ETAS model by maximizing the log-likelihood value inside a time interval Di and then estimating the time-dependent background rate using the plus/minus n-nearest neighbors.
With the estimated μ(t), the ETAS parameters are re-estimated and so on, until the convergence of both parameters and background rates. The smaller the smoothing window n, the larger the degree of freedom of the model would be and the variation of μ(t) would be stronger as well. The optimal value of the smoothing window is determined by the Akaike information criterion, AIC = 2 k − 2ln(L) where k is the number of free model parameters and L is the maximum likelihood value. The computation of the ETAS parameters is carried out considering the three phases of Table 1. An alternative computation for phase D3 is developed excluding the aftershock productivity of events before July 2013 (D3′). This leads to an unrealistic situation in the case of D3′, which assumes no prior high activity. However, it prepares a more conceivable comparison between background seismicity before and after the main sequence.
6 Results and discussion
The Akaike information criterion (AIC) yields n = 3 as optimal smoothing parameters for D1, D3 and D3′, while the optimal value is n = 9 in the case of D2. These small smoothing windows indicate that strong temporal changes of the background rates are necessary to statistically explain the observed data. Thus, transient aseismic processes likely occurred in all three phases which triggered the majority of observed M ≥ 1.3 events. The estimated fraction of events attributed to the background activity is between 60 and 83%. Vice versa, only 17 to 40% of the events are identified as aftershocks. This result is provided in Fig. 6 together with the estimation of the ETAS parameters. The estimated c-values are small and range between 3 and 13 min (0.002 and 0.009 days), while α-values are close to 1 for D1 and D2 which are significantly smaller than those values observed for typical aftershock sequences (Ogata 1992; Hainzl and Ogata 2005). However, smaller α-values have been previously found to be indicative of swarm activity. In contrast, the latest phase has an estimated value of 1.55 which is close to typical tectonic values (Hainzl et al. 2013). Together with the observed b-value decrease in this last phase, this might indicate the change of the activity from swarm-type to mainshock–aftershock-type activity. However, the reason for the significant increase in the Omori p value from 1.15 in D1 to 1.44 in D2 and 1.69 in D3 remains unclear.
It is also noticeable that the result for D3 does not change significantly, if we exclude the aftershock productivity of events before July 2013, as in D3′. Figure 10a shows the estimated time-dependent background rate before and after the main swarm activity in logarithmic scale. Apart from short time excursions, the rate fluctuates around 0.1 events per day in D1 and around 0.3 events per day in the D3 phase. The background rate during D2 is strongly amplified and gradually decays with time approximately according to an exponential function. The fit of the ETAS model is shown in Fig. 10b indicating that the ETAS model is capable to model the swarm activity and predicts only 24 events less than observations.
a The time-dependent background rate before (D1), after (D3), and during the main activity (D2). b The result of ETAS modeling for the cumulative event numbers in D2 is illustrated with a red solid line in comparison to the observed one (black dashed line), while the blue dotted line describes the cumulative number of estimated background events
In order to understand changes in the aftershock productivity (trigger potential), we need to introduce the theoretical relation between the number of aftershocks triggered by a mainshock of magnitude M, studied by Utsu (1971).
In the ETAS formulation, this should be related to\( {\int}_0^{\infty}\mathrm{K}{\mathrm{e}}^{\upalpha \left(\mathrm{M}-{\mathrm{M}}_{\mathrm{c}}\right)}{\left(\mathrm{t}+\mathrm{c}\right)}^{-\mathrm{p}}dt \) for p > 1, that is,
Using ETAS parameters in Table 2, we find A to be 0.030, 0.068, 0.005, and 0.008 for D1, D2, D3, and D3′. Then, we can find the minimum magnitude that will produce at least one aftershock (n = 1) using Eq. (9) in order to compare the productivity more explicitly.
Figure 11 shows the productivity changes during the three phases. The trend varies with the α-value and with the A-value and defines the mainshock magnitude which is related to the specific number of aftershocks. The trend during D1 and specially D2 is slower with a rise in magnitude (α ∼ 1), and among D2, smaller magnitudes are more productive in comparison to the other phases (A = 0.068).
After D2, the capacity of the aftershock production is shown for both cases D3 and D3′. As we have seen in ETAS calculation results, they basically expose very similar parameters and occurrence rates. Such comparison indicates the independence of seismicity rate in this phase from the past. However, Fig. 11 illustrates that if we ignore D2 for ETAS parameter calculations in post-activity phase D3′, the productivity potential after the swarm is more or less likely before the swarm and starts with magnitude ∼3.1, but then the trend gets faster, indicating a more rapid rise in productivity with rise in the magnitude. This is an evidence for the fact that since July 2013, the forcing system is experiencing some changes that cause less swarm characteristics or more elastic effects. But the same minimum magnitude for being a potential parent seems unrealistic if we assume that the system has gone toward a higher rigidness. Including the history for ETAS calculations of the post-activity phase D3, the result gives a more reasonable view of what happens after the main swarm D2. It can be seen that the minimum patented magnitude for having at least one aftershock rises from 3.1 in D1 and D3′ to 3.4 for D3.
7 Summary and conclusions
The Torreperogil–Sabiote 2012–2013 seismic series represents a swarm-like activity with strong clustering in space and time. Earthquakes are known to interact by means of induced dynamic and static stress changes and thus cannot be modeled as independent events. This is clear for classical mainshock–aftershock sequences which can be modeled by the Omori–Utsu law. However, stress interactions occur also during earthquake swarms where no clear mainshock can be identified. Some earthquake swarms might be only the random result of stress interactions where several triggered events have by chance similar large magnitudes. Often, however, earthquake swarms are driven by an additional transient aseismic process, such as fluid intrusions or slow earthquakes. The aseismic process might change not only the background rate but also some other statistical properties of the activity. For a proper analysis of the observed sequence, we thus analyze temporal changes of the statistical properties and apply a modified version of the ETAS model which includes time-dependent background rates.
The result of maximum log-likelihood estimations for the ETAS parameters was derived for small smoothing windows indicating rapid temporal changes in background activity. Such fluctuations in time can be due to rapid evolutions in the forcing rate which switch the system to a higher seismic activity as we observe since October 20, 2012. The high proportion of background events ∼80% for all small clustered earthquakes between 2010 and October 2012 (before the main sequence) suggests a high contribution of transient aseismic process. Along with the occurrence of the main phase of the swarm, the aftershocks’ contribution duplicates from 20 to 40%. But it is still carrying out the minority of the whole population of the events. The resulting μ-value shows some strong fluctuations which might suggest an episodic character of the aseismic forcing. Nevertheless, some fluctuations could also be partially related to missing events in phases of activity (Hainzl 2016).
Our analysis of the sequence shows that the activity is not solely explainable by tectonic loading and earthquake–earthquake triggering and that an additional transient aseismic loading process must have taken place. Decreasing b-values (Fig. 6) during the main seismic activity might be related to an aseismic process which continuously increased the average stress level. Considering that the area is not volcanic, it is likely that fluid movements or slow earthquakes were responsible for the swarm activity in the years 2012–13 in the Torreperogil–Sabiote area. The potential processes were also suggested based on the analysis of the hypocenter distribution and seismotectonic structures by Morales et al. (2015).
Furthermore, we find that the background rate remains elevated after the main swarm activity with decreased b-value and increased α-value of the trigger potential. Altogether, this might indicate a system change to a more critical stress state in this region. The background rate in this period describes 83% of the activity. The result for D3′ is driven independent of the history and shows the same percentage of the background events as for D3. It may be concluded that the behavior of the area after the main sequence is not a continuation of the 2012–2013 swarm but is its consequence.
References
Benito B, Gaspar-Escribano JM (2007) Ground motion characterization in Spain: context, problems and recent developments in seismic hazard assessment. J Seismol 11:433–452
Cantavella JV, Morales J, Martinez-Solares JM (2013) La serie sísmica de la comarca de La Loma (Jaén). Antecedentes, distribución temporal, localización y mecanismo focal, Jaen. Informe del grupo de trabajo interinstitucional sobre la actividad sísmica en la comarca de la loma (Jaen), Ministerio de Fomento, Madrid, pp 25–38
Doblas M., Toubi N, Delas Doblas J, Galindo AJ (2014) The 2012/2014 swarmquake of Jaen, Spain: a working hypothesis involving hydroseismicity associated with the hydrologic cycle and anthropogenic activity. Nat Hazards 73(II): 1223–1261
Enescu B, Mori J, Masatoshi M (2007) Quantifying early aftershock activity of the 2004 mid-Niigata Prefecture earthquake (Mw6.6). J Geophys Res: 112(B4)
Gutenberg B, Richter CF (1956) Earthquake magnitude, intensity, energy and acceleration. B Seismol Soc Am 46:105–145
Hainzl S, Fischer T (2002) Indications for a successively triggered rupture growth underlying the 2000 earthquake swarm in Vogtland/NW Bohemia. J Geophys Res 107(B12)
Hainzl S, Ogata Y (2005) Detecting fluid signals in seismicity data through statistical earthquake modeling. J Geophys Res 110(B5)
Hainzl S, Marsan D (2008) Dependence of the Omori-Utsu law parameters on main shock magnitude: Observations and modeling. J Geophys Res 113(B10)
Hainzl S, Zakharova O, Marsan D (2013) Impact of aseismic transients on the estimation of aftershock productivity parameters. B Seismol Soc Am 10(3):1723–1732
Hainzl S (2016) Rate-dependent incompleteness of earthquake catalogs. Seismol Res Lett 87(2A)
Kagan YY (2004) Short-term properties of earthquake catalogs and models of earthquake source. B Seismol Soc Am 94:1207–1228
Lippiello E, Corral A, Bottiglieri M, Godano C, de Arcangelis L (2012) Scaling behavior of the earthquake intertime distribution: influence of large shocks and time scales in the Omori law. Phys Rev E 86:066119
Marsan D, Prono E, Helmstetter A (2013) Monitoring aseismic forcing in fault zones using earthquake time series. B Seismol Soc Am 103(1):169–179
Martinez MD, Lana X, Posadas AM, Pujades L (2005) Statistical distribution of elapsed times and distances of seismic events: the case of the southern Spain seismic catalogue. Nonlinear Proc Geoph 12:235–244
Mignan A (2012) Seismicity precursors to large earthquakes unified in a stress accumulation framework. Geophys Res Lett 39(21308)
Morales J, Azañón JM, Stich D et al (2015) The 2012-2013 earthquake swarm in the eastern Guadalquivir basin (South Spain): a case of heterogeneous faulting due to oroclinal bending. Gondwana Res 28(4):1566–1578
Ogata Y (1988) Statistical models for earthquake occurrence and residual analysis for point processes. J Am Stat Assoc 83(401):9–27
Ogata Y (1992) Detection of precursory relative quiescence before great earthquakes through a statistical model. J Geophys Res 97: 19, 845–19, 871
Pedrera A, Ruiz-Constán A, Marín-Lechado C, Galindo-Zaldívar J, González A, Peláez JA (2013) Seismic transpressive basement faults and monocline development in a foreland basin (Eastern Guadalquivir, SE Spain). Tectonics 32:1571–1586
Peláez JA, García-Tortosa FJ, Sánchez-Gómez M et al (2013) La serie sísmica de Torreperogil-Sabiote (Jaén). Enseñanza de las Ciencias de la Tierra 21(3):336–338
Peng Z, Vidale JE, Houston H (2006) Anomalous early aftershock decay rate of the 2004 Mw6. 0 Parkfield, California, earthquake. Geophys Res Lett 33(17)
Rodríguez-Escudero E, Martínez-Díaz JJ, Álvarez-Gómez JA et al (2014) Tectonic setting of the recent damaging seismic series in the Southeastern Betic Cordillera, Spain. B Earthq Eng 12:1831–1854
Saichev A, Sornette D (2007) Theory of earthquake recurrence time. J Geophys Res 112(B04313)
Schoenball M, Davatzes NC, Glen JM (2015) Differentiating induced and natural seismicity using space-time-magnitude statistics applied to the Coso Geothermal field. Geophys Res Lett 42:6221–6228
Scholz CH (2015) On the stress dependence of the earthquake b value. Geophys Res Lett 42(5):1399–1402
Schorlemmer D, Wiemer S, Wyss M (2005) Variations in earthquake-size distribution across. Nature 734(22):539–542
Senshu T (1959) On the time interval distribution of aftershocks. J Seismol Soc Jpn 12(4):149–161
Tomada Y (1954) Statistical description of the time interval distribution of earthquakes and on its relations to the distribution of maximum amplitude. Zisin 7(2):155–169
Utsu T (1971) Aftreshocks and earthquake statistics (III). J Fac Sci U Hokkaido, Ser VII, Geophys 3(5):379–441
Utsu T, Ogata Y, Matsu'ura RS (1995) The centenary of the Omori formula for a decay law of aftershock activity. Phys Earth 43:1–33
Wiemer S, Wyss M (2000) Minimum magnitude of completeness in earthquake catalogs: examples from Alaska, the Western United States, and Japan. B Seismol Soc Am 90(4):859–869
Acknowledgments
Two anonymous reviewers provided insightful comments to this paper and they are gratefully thanked. Technical support by J.L.G. Pallero (UPM) and research discussions with S. Cesca (GFZ) are highly appreciated. This work was partly conducted during a research stay of P.Y. at GFZ (Potsdam). It is part of the PhD Project of P.Y. that is carried out in the Earthquake Engineering Research Group of UPM, which it is also acknowledged.
Author information
Authors and Affiliations
Corresponding author
Rights and permissions
About this article
Cite this article
Yazdi, P., Hainzl, S. & Gaspar-Escribano, J.M. Statistical analysis of the 2012–2013 Torreperogil–Sabiote seismic series, Spain. J Seismol 21, 705–717 (2017). https://doi.org/10.1007/s10950-016-9630-4
Received:
Accepted:
Published:
Issue Date:
DOI: https://doi.org/10.1007/s10950-016-9630-4