Abstract
Shanghai is one of the largest metropolitan areas in the world, during the rapid urbanization of the past decades, impervious surface expanded dramatically and became a main factor influencing surface water quality. Thus, exploring the driving forces of impervious surface has great implications in such metropolitan area. In this study, an impervious surface coefficient method (ISC) was used to measure the percentage of total impervious area (PTIA) of Shanghai; regression analysis was conducted to define the relationship between PTIA and three socio-economic factors, population density, unit area gross domestic product, and unit area industrial output at the city and district scale. Results showed that the industrial land use generated the highest ISC value, followed by high-density residential. Strong correlations were showed between PTIA and socio-economic indicators, in which population density was the most significant. Threshold effect was presented that when population density was higher than 15000 per/km2, this relationship would become less significant and PTIA remained stable. Similar effects were found when unit area gross domestic product exceeded 125 million yuan/km2. Scale effect was also discussed that the relationship was more significant at city scale than district. An improved understanding of the threshold effect and scale effect will help guide future urban planning and design new urban ecosystem policies.
Similar content being viewed by others
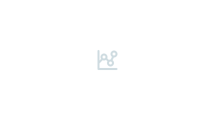
Explore related subjects
Discover the latest articles, news and stories from top researchers in related subjects.Avoid common mistakes on your manuscript.
Introduction
During processes of urban expansion, a large proportion of pervious surfaces transform into impervious (Chaudhuri et al. 2017; Sekertekin et al. 2018). Impervious surfaces are often defined as roads, parking lots, rooftops, etc. (Ma et al. 2018; Xian et al. 2007), and the percentage of total impervious area (PTIA) has been identified as a reliable indicator of environment quality (Xian and Crane 2005; Yan et al. 2019). Relationships between impervious surfaces and related socio-economic driving forces may explain why impervious surfaces expand, and guide urban and environmental planners in designing policies related to PTIA control. Previous researches on impervious surfaces have mainly focused on estimation and mapping techniques, spatial distributions, and relationships between PTIA and environmental indicators (Wu and Murray 2003; Jat et al. 2008; Xian 2008; Yue 2009; Jacobson 2011; Nagy et al. 2012; Uygun and Albek 2015; Hafsi et al. 2016; Tahmina et al. 2018).
However, few studies have discussed on whether there is a linear relationship between PTIA and the socio-economic indicators, and whether the relationship has a scale effect. The threshold and scale effects are essential for planning the future of urban development, particularly for the studies of typical rapid-urbanization megacities. The objective of this study is to quantify the relationship between socio-economic indicators and PTIA to test the capability of socio-economic indicators for predicting the trends of impervious surface expansion, while examining whether this relationship shows threshold and scale effects.
There are three methods that have been employed to estimate and map PTIA: manual extraction, GIS-based model, and impervious surface coefficient method (ISC). The first one, which involves manually digitizing impervious surfaces from aerial photographs or satellite images, is generally the most accurate but cost effective for small areas. However, it is too expensive and time-consuming to employ when examining larger-scale applications (Weng 2012). The second, which involves extracting impervious surface bases via GIS, spectral mixture analysis (SMA), and classic vegetation-impervious surface-soil (V-I-S) models, must be conducted by researchers with professional background in geography and remote sensing, and typically used in specific cases of impervious surface extraction (Rashed et al. 2001; Wu and Murray 2003; Guo and Huang 2013; Henits et al. 2017). The third method involves applying field survey-derived ISC to specific land uses in order to measure PTIA; land uses can typically be divided into industrial, commercial, residential, forest, agriculture, public, road, and water area (Sleavin et al. 2000; Lu and Weng 2006; Barbara et al. 2010; Beck et al. 2016).
Taking Shanghai as a case, we developed an impervious surface coefficient (ISC) set for land uses in this metropolitan area; the PTIA and its spatial distribution were displayed; it is also discussed whether socio-economic indicators such as population density (PD), unit area gross domestic product (UAGDP), and unit area industrial output (UAIO) played as driving forces in impervious surface expansion, and the threshold and scale effects were then displayed. An improved understanding of the relationships and its threshold effects will help guide future planning efforts aimed at urban ecosystem management.
Study area and method
Study area
Shanghai is a metropolitan area composed of 18 districts (nine urban and nine suburban districts) with a total area of 6340.5 km2, making it one of the largest metropolises in the world. In the past three decades, Shanghai has experienced rapid urbanization, growing from a population of 11.46 million in the 1980s to 24.15 million in 2010; the city’s GDP has also increased from RMB 33.7 billion Yuan to 2496.5 billion Yuan. Although the process of rapid urbanization has brought prosperity to the city, it also caused environmental problems. Figure 1 illustrates Shanghai’s location in China and population density histograms of each district.
Data
The imagery data of sampling sites is from ZY-3 satellite and provided by the China National Platform for Common Geospatial Information Services, and the image resolution is 0.6 m, while the land use data of Shanghai were originated from STOP-5 satellite remote sensing image and interpreted by the Key Laboratory of Geographic Information Science of the Education Ministry of East China Normal University; the image resolution is 1.4 m.
It should be stressed that in the land use data of Shanghai, land uses dominated by impervious area, such as residential and industrial, were interpreted as entirely impervious, for example, the permeable surfaces like the green spaces in residential were ignored. Moreover, the green spaces and parks were interpreted as entirely pervious, and the impervious elements like roads and buildings embedded in the pervious areas were interpreted as impervious. Therefore, the land use data is not suitable to measure the impervious surface coefficients in Shanghai.
The data of socio-economic indicators of Shanghai were drawn from Shanghai statistical yearbook (2007) (Table 1), while the PD, UAGDP, and UAIO for all 18 districts were derived from statistical yearbook (2007) of each district. Statistical Yearbook of Shanghai and each district contain economic and social statistical data (e.g., population, GDP, total industrial output, acreage) collected by Shanghai Municipal Bureau of Statistics and China National Bureau of Statistics.
Methods
The imagery data can be used to map the impervious surfaces such as roofs, roads, and parking lots in various land uses (e.g., residential, industrial, and green space). Therefore, this paper combined the land use data with imagery data to assess the impervious surface coefficients of sampling sites in Shanghai (Fig. 2). Based on the land use data, a total of 240 samples were selected for six land uses (50 high-density residential, 50 middle-density residential, 50 low-density residential, 30 public, 30 industrial, and 30 green space) (Fig. 3). With imagery data, the impervious surfaces were interpreted in ArcGIS vectorization module. First, the roof, road, parking lot, and square were mapped for each sampling site, and the ISC for each sampling site was then calculated. Finally, the ISC value of each land use was assigned as an average of sampling sites; furthermore, when mapping the PTIA for Shanghai and its districts/towns, the ISC of each land use would be applied to all corresponding land uses.
Two regression analyses were conducted to define the relationship between PTIA and three socio-economic indicators at the city and district scale, which would display the threshold effect and scale effect. At city scale, this paper takes Shanghai as the research subject and 18 districts or 136 towns as samples. In terms of district scale, the study took nine suburban districts as the research subjects and towns/sub-districts in each district as samples.
It should be noted that the area of each urban district (city center) in Shanghai is too small, and even the total area of urban districts may be smaller than that of any suburb district. Furthermore, the level of urbanization in the urban district is usually higher, and there were no differences between towns and sub-districts in city center. For suburb districts, they cover a larger area and the urbanization level is unevenly distributed in towns and sub-districts. Therefore, the urban districts were no longer subdivided into sub-districts and towns in our analysis, but the suburbs were further subdivided into towns and sub-districts.
Results
Impervious surface coefficients of land uses
Industrial land uses generate the highest ISC with a value of 0.75 and are followed by high-density residential and public areas with ISC values of 0.73 and 0.64, respectively, and green spaces generate the lowest value of 0.33 (Table 2).
Spatial variations of impervious surfaces
Figure 4 illustrates spatial variations of PTIA for Shanghai; it shows that PTIA levels in the city center were higher than those of suburban areas. The average PTIA value for the city center (nine urban districts) was recorded as 64.9% and the average PTIA value for the nine suburban districts was 19.24%. Chongming district, an island isolated from mainland Shanghai, generated the lowest PTIA of 3.69%. Overall, PTIA levels in the city center and in the center of suburban district were found to be higher due to the presence of impervious structures such as buildings, roads, and parking lots. However, PTIA levels were found to be lower in less-developed suburban area and water resource protection area composed of agricultural land and suburban residents.
Relationship between PTIA and population density
Relationships at the city scale
Figure 5 shows regression results for the relationship between PTIA and population density (PD) at the city scale. When a total of 18 districts in Shanghai were examined, strong correlations were found between PTIA and PD (R2 = 0.922). From Fig. 5, we could deduce that when PD increase five times, PTIA of corresponding areas increase by 20%. However, when PD reached 15,000 per/km2, PTIA would remain stable.
In order to verify the above conclusions, 136 towns and sub-districts were also examined; a strong correlation was also found between PTIA and PD (R2 = 0.670). Figure 5 also shows a threshold effect wherein the relationship between PTIA and PD becomes insignificant when PD levels exceed 15,000 per/km2. At the same time, it can be found that the results of the study using 18 districts as samples were significant than those for towns.
Relationships at the district scale
Relationships between PTIA and PD for nine suburban districts were examined. Table 3 shows regression models for the relationship between PTIA and PD in suburban districts. The Jiading, Jinshan, and Minhang districts generated higher R2 values of 0.682, 0.661, and 0.634, respectively, while the Qingpu district showed the lowest R2 with a value of 0.108. Generally, districts with higher PD (> 1000 per/km2) presented better relationship (R2 > 0.5), and districts with lower PD (< 750 per/km2) expressed unclear relationship. An average R2 value of 0.473 was found at the district scale, which is much lower than that found at the city scale. The analyses suggested that the relationship between PTIA and PD is more significant at city scale (the whole Shanghai) than district scale (each suburban district).
Relationships between PTIA and economic variables
Relationships at the city scale
Figure 6 shows regression models of the relationships between PTIA and economic factors at the city scale. When a total of 18 districts in Shanghai were examined, a stronger positive correlation between PTIA and UAGDP (R2 = 0.739) was found than that between PTIA and UAIO (R2 = 0.463). This implies that PTIA was affected more by UAGDP. Figure 6 also shows a threshold effect of the relationship between PTIA and economic indicators. Also, an interesting finding was displayed, namely, when UAGDP increased five times, the PTIA increased by 20%, and this relationship was less significant when UAGDP was higher than 125 million yuan/km2. Similar results were found for the relationship between PTIA and UAIO. Rather, when UAIO was higher than 250 million yuan/km2, PTIA remained stable.
In order to verify the above conclusions, 136 towns and sub-districts were also examined; positive correlations were found between PTIA and economic factors. The relationship between PTIA and UAGDP was calculated as R2 = 0.520, and R2 = 0.355 was found for the relationship between PTIA and UAIO. Thus, UAGDP represents a more significant factor when analyzing relationships between PTIA and economic factors.
Relationships at the district scale
Table 3 presents regression models of the relationship between PTIA and economic factors at the district scale. Positive correlations were observed for the relationships between PTIA and UAGDP (average R2 = 0.392), and PTIA and UAIO (average R2 = 0.257). Minhang district showed the highest UAGDP correlation while Fengxian district generated the highest UAIO correlation (R2 = 0.912). It should be noted that Jiading district generated the lowest UAGDP value while Chongming district generated the lowest UAIO values.
When all regression models for the relationship between PTIA and socio-economic indicators are considered together, relationships between PTIA and PD were superior for all of the socio-economic indicators at the district scale (R2 = 0.473), which was inferior to relationships between PTIA and PD at the city scale (R2 = 0.922). The analyses suggested that PD serves as the most significant factor; additionally, larger scale cases show better relationships.
Discussion
ISC and impervious surface estimations
ISC values for six land uses were compared with previous studies. Several differences were found, and especially in relation to residential, industrial, green spaces, and public land uses.
- (1)
We examined residential areas based on three categories (high, middle, and low density) following Choi and Ball (2002) and found ISC levels of 0.73, 0.55, and 0.49, respectively. Chen et al. (2006) showed ISC values for middle- and high-density residential areas of between 0.55 and 0.95 for Nanjing, another Chinese city, consisting with our results. Carlson (2001) found the values of ISC range from 0.30 to 0.75, echoing with our results. Prisloe et al. (2001) found an ISC value of 0.36 for mixed residential and commercial areas, while the ISC value is 0.12 for suburban residential areas. Sleavin et al. (2000) found ISC values for residential areas ranged from 0.08 to 0.39. However, our average ISC for residential areas are 50–100% higher than those of previous studies (Lu and Weng 2006; French 2009). This is attributable to the fact that percentage of low density residential land in China, a huge population country, is quite lower than those of developed countries.
- (2)
An ISC value of 0.75 was found for industrial areas. Previous studies have found ISC values for such areas range between 0.5 and 0.9 (Sleavin et al. 2000; French 2009; Barbara et al. 2010). Reporting on California, Barbara et al. (2010) found an ISC value of 0.91 for heavy industry areas and an ISC value of 0.81 for light industry areas, exceeding our results by 15%. While American industrial sites include little vegetation and bare soils (Chen et al. 2006), industrial sites in Shanghai include more green spaces in accordance with municipal regulations, thus explaining our lower ISC results for industrial areas.
- (3)
ISC value of green spaces is 0.33 in Shanghai. Chabaeva et al. (2009) found ISC values of 0.13, 0.046, and 0.049 for turf and grass, deciduous forest, and coniferous forest areas, respectively. Prisloe et al. (2001) found ISC values of 0.09 for turf and grass areas and ISC values of 0.045 for forested areas. Green spaces in this study refer to man-made parks and grass areas with few paved surfaces and buildings, while forested areas examined in previous studies were natural with virtually no impervious features, and thus, our results for green spaces are much higher.
- (4)
ISC value of public areas is 0.64 in Shanghai. Barbara et al. (2010) found an ISC value of 0.44 for public/quasi-public areas, while Chen et al. (2006) displayed a value of 0.83 for public areas. These results showed the highest degree of variance of the results for land uses considered, as designations were based on ownership rather than on types of use (Barbara et al. 2010). Hence, ISC values for public areas were more variable.
Driving forces of impervious surface
Several researchers have showed positive correlations between PTIA and socio-economic factors (Dhorde et al. 2012; Ma et al. 2016; Liao et al. 2017; Chen et al. 2018; Li et al. 2018; Cao et al. 2019). Azar et al. (2010) conducted a regression analysis to determine the relationship between impervious surfaces and PD levels at three administrative levels. R2 values of 0.94, 0.81, and 0.85 were found for department (1st level), commune (2nd level), and section scales (3rd level), respectively. The same result was found by Dhorde et al. (2012). Our study finds a similar result that at the city scale, PD levels correlate strongly with PTIA. However, at the district scale, several districts (e.g., Fengxian, Songjiang, and Qingpu) showed very low R2 values (0.10–0.35).
Cao et al. (2019) indicated that the growths of population and gross domestic product (GDP) are regarded as the key drivers for urban expansion associated with an increase of ISC. The same result was showed by Liao et al. (2017) and Li et al. (2018). Chen et al. (2018) thought that tertiary industry product, GDP, secondary industry product, total population, and urban population were driving factors of urban land sprawl in Northeast China from 1990 to 2015.
We also made clear that positive relationships were showed between PTIA and economic forces, and UAGDP may be having a more significant effect than UAIO. As a world-famous developing country, China’s industrialization is the most important driving force of urbanization (Ma 2002; Eng. 2010). As one of the most developed city in China, Shanghai had entered the post-industrialization stage after the 1990s. In this stage, the development of cities no longer depended solely on industry, and technological innovation and service industry have become primary factors leading urbanization. For example, according to our study (Zhao 2008), the percent of industrial land in center Shanghai increased from 4.86% in 1947 to 22.07% in 1989, but decreased to 13.05% in 2006. It can be speculated that the urbanization of Shanghai was dependent on industrialization before 1989, and then the industrial land has transmitted to the suburbs and concentrated in industrial parks. At the same time, the percentage of residential and public land is rising. As the best indicator of a country’s economic, UAGDP shows more significant relationship between PTIA than UAIO.
As to population, when a city was at its preliminary stage, low population density areas have more impervious surface per resident because of roads and non-residential land use. When the city is in the stage of rapid development, the population increases rapidly, the living facilities continue to increase due to the increase of population, and high population density areas have less impervious surface per resident. Thus, the relationship between PD and PTIA is more significant in the case of high population density.
It should be stressed that China’s urban population includes the local population, permanent immigrants, and floating population, and the floating population is the main source of urban population growth. With the continuous development of the economy, labor productivity has increased and labor demand has begun to decrease, which slowed down the increase in the floating population. Furthermore, the inflow of young labor and the outflow of mature labor have formed a cycle in the internal structure of population mobility. With some arbitrary, we hold that floating population had no influence on this research.
In addition, since the actual population of some highly migrated areas is difficult to count, the PTIA can also be used to estimate the actual population. This paper can provide a way of estimating internal migration, for regions that have a high level of unofficial movement to cities.
Threshold effect and scale effect
A clear threshold effect was indicated. When PD levels exceeded 15,000 per/km2, the relationship with PTIA was less significant. Similar results were found when UAGDP exceeded 125 million yuan/km2. Since the city’s land is limited, we argue that processes of impervious surface expansion have limits, and eventually, PTIA must reach the summit and remain stable.
The relationship between PTIA and socio-economic factors was more significant at city scale than district scale, which means that the relationship was more significant on a larger scale. At the same time, the results in the case of 18 districts as samples were more significant than those of 136 towns.
Limitations
The results of this study are limited by the following factors. First, our ISC of land use calculations were less specific, as ISC levels were regarded as 1 for roads and as 0 for agricultural and water areas. Second, only 240 samples were examined and our use of fewer samples may have introduced sampling bias. Third, while our explanations of threshold phenomena and scale effects found are empirical to a degree, more quantitative analyses should be conducted such as the piecewise regression method, such as R software.
Conclusion
Taking Shanghai, a world metropolitan area, as a case, this paper examined coefficients for all land uses, and it is found that industrial land use generates the highest impervious surface coefficient (ISC) with a value of 0.75, followed by high-density residential. A regression analysis was also conducted to define the relationship between PTIA and three socio-economic factors at city scale and district scale. The results suggest that there is a significant positive correlation between PTIA and socio-economic indicators, in which PD was the most significant one. Threshold effect and scale effect were also presented. When PD exceeded 15,000 per/km2, the relationship between PTIA and socio-economic indicators would be less significant. Similar results were found for UAGDP, which exceeded 125 million yuan/km2. Moreover, the relationship became more significant at larger scale. The results of this paper would give suggestions for urban planning and environmental management.
References
Azar, D., Graesser, J., Engstrom, R., Comenetz, J., Leddy, R. M., Schechtman, N. G., & Andrews, T. (2010). Spatial refinement of census population distribution using remotely sensed estimates of impervious surfaces in Haiti. International Journal of Remote Sensing, 31(21), 5635–5655. https://doi.org/10.1080/01431161.2010.496799.
Barbara, W., Katie, Y., et al. (2010). User’s guide for the California impervious surface coefficients. California Environment Protection Agency: United States.
Beck, S. M., Mchale, M. R., & Hess, G. R. (2016). Beyond impervious: urban land-cover pattern variation and implications for watershed management. Environmental Management, 58(1), 15–30. https://doi.org/10.1007/s00267-016-0700-8.
Cao, S., Hu, D., Zhao, W., Mo, Y., & Zhang, Y. (2019). Monitoring changes in the impervious surfaces of urban functional zones using multisource remote sensing data: a case study of Tianjin, china. GIScience & Remote Sensing. https://doi.org/10.1080/15481603.2019.1600110.
Carlson, T. N. (2001). Impervious surface area and residential housing density: a satellite perspective. Geocarto International, 16(1), 15–20. https://doi.org/10.1080/10106040108542178.
Chabaeva, A., Civco, D. L., & Hurd, J. D. (2009). Assessment of impervious surface estimation techniques. Journal of Hydrologic Engineering, 14(4), 377–387. https://doi.org/10.1061/(ASCE)1084-0699(2009)14:4(377.
Chaudhuri, A. S., Singh, P., & Rai, S. C. (2017). Assessment of impervious surface growth in urban environment through remote sensing estimates. Environmental Earth Sciences, 76(15), 541.
Chen, S., Zhang, X. Y., & Peng, L. H. (2006). Impervious surface coverage in urban land use based on high resolution satellite images. Resources Science, 28(2), 41–46.
Chen, L., Ren, C. Y., Zhang, B., Wang, Z. M., & Liu, M. Y. (2018). Quantifying urban land sprawl and its driving forces in Northeast China from 1990 to 2015. Sustainability, 10(1), 188. https://doi.org/10.3390/su10010188.
Choi, K. S., & Ball, J. E. (2002). Parameter estimation for urban runoff modelling. Urban Water,4(1), 31-41. https://doi.org/10.1016/S1462-0758(01)00072-3.
Dhorde, A. A., Dhorde, A., & Joshi, G. (2012). Population calibrated land cover impervious surface coefficients for Upper Bhima basin. International Journal of Geomatics & Geosciences, 4, 7–1047.
Eng, I. (2010). The rise of manufacturing towns: externally driven industrialization and urban development in the pearl river delta of china. International Journal of Urban & Regional Research, 21(4), 554–568. https://doi.org/10.1111/1468-2427.00101.
French, S. P. (2009). Regional impervious surface estimation: an urban heat island application. Journal of Environmental Planning and Management, 52(4), 477–496. https://doi.org/10.1080/09640560902868207.
Guo, H., & Huang, Q. (2013). Spatiotemporal analysis of urban environment based on the vegetation–impervious surface–soil model. Journal of Applied Remote Sensing, 8(1), 084597. https://doi.org/10.1117/1.JRS.8.084597.
Hafsi, R., Ouerdachi, L., Kriker, A. E., & Boutaghane, H. (2016). Assessment of urbanization/impervious effects on water quality in the urban river Annaba (Eastern Algeria) using physicochemical parameters. Water Science & Technology, 74(9), 2051–2059. https://doi.org/10.2166/wst.2016.350.
Henits, L., Mucsi, L., & Liska, C. M. (2017). Monitoring the changes in impervious surface ratio and urban heat island intensity between 1987 and 2011 in Szeged, Hungary. Environmental Monitoring & Assessment, 189(2), 86–13. https://doi.org/10.1007/s10661-017-5779-8.
Jacobson, C. R. (2011). Identification and quantification of the hydrological impacts of imperviousness in urban catchments: a review. Journal of Environmental Management, 92(6), 1438–1448. https://doi.org/10.1016/j.jenvman.2011.01.018.
Jat, M. K., Garg, P. K., & Khare, D. (2008). Monitoring and modelling of urban sprawl using remote sensing and GIS techniques. International Journal of Applied Earth Observation and Geoinformation, 10(1), 26–43. https://doi.org/10.1016/j.jag.2007.04.002.
Li, M., Zang, S., Wu, C., & Na, X. (2018). Spatial and temporal variation of the urban impervious surface and its driving forces in the central city of Harbin. Journal of Geographical Sciences, 28(3), 323–336. https://doi.org/10.1007/s11442-018-1475-z.
Liao, C., Dai, T., Cai, H., & Zhang, W. (2017). Examining the driving factors causing rapid urban expansion in china: an analysis based on globeland30 data. ISPRS International Journal of Geo-Information, 6(9), 264. https://doi.org/10.3390/ijgi6090264.
Lu, D. S., & Weng, Q. H. (2006). Use of impervious surface in urban land-use classification. Remote Sensing of Environmen, 102(1-2), 146–160. https://doi.org/10.1016/j.rse.2006.02.010.
Ma, L. J. C. (2002). Urban transformation in china, 1949-2000: a review and research agenda. Environment & Planning A, 34(9), 1545–1569. https://doi.org/10.1068/a34192.
Ma, Q., He, C. Y., & Wu, J. G. (2016). Behind the rapid expansion of urban impervious surfaces in China: Major influencing factors revealed by a hierarchical multiscale analysis. Land Use Policy, 59, 434–445. https://doi.org/10.1016/j.landusepol.2016.09.012.
Ma, Q., Wu, J., He, C., & Hu, G. (2018). Spatial scaling of urban impervious surfaces across evolving landscapes: from cities to urban regions. Landscape & Urban Planning, 175, 50–61. https://doi.org/10.1016/j.landurbplan.2018.03.010.
Nagy, R. C., Lockaby, B. G., Kalin, L., & Anderson, C. (2012). Effects of urbanization on stream hydrology and water quality: the Florida Gulf Coast. Hydrological Processes, 26(13), 2019–2030. https://doi.org/10.1002/hyp.8336.
Prisloe, S., Lei, Y., & Hurd, J. (2001). Interactive GIS-based impervious surface model. Proceedings of the 2001 ASPRS Annual Convention, St. Louis, MO. CD-ROM. American Society for Photogrammetry & Remote Sensing.
Rashed, T., Weeks, J. R., Gadalla, M. S., & Hill, A. G. (2001). Revealing the anatomy of cities through spectral mixture analysis of multispectral satellite imagery: a case study of the greater Cairo region, Egypt. Geocarto International, 16(4), 7–18. https://doi.org/10.1080/10106040108542210.
Sekertekin, A., Abdikan, S., Marangoz, A. M. (2018) The acquisition of impervious surface area from LANDSAT 8 satellite sensor data using urban indices: a comparative analysis. Environmental Monitoring and Assessment, 190, (7).
Shanghai Statistical Bureau. (2007). Shanghai Statistical Yearbook. Beijing: China Statistics Press
Sleavin, W. J., Civco, D. L., Prisloe, S., Educator, A., Giannotti, L., & Coordinator, N. P. (2000). Measuring impervious surfaces for non-point source pollution modeling. Proceedings Asprs Annual Convention.
Tahmina, A., Philippe, Q., Steven, J. E., & Guido, V. (2018). Impacts of climate and land use changes on flood risk management for the Schijn River, Belgium. Environmental Science & Policy, 89, 163–175. https://doi.org/10.1016/j.envsci.2018.07.002.
Uygun, B. S., & Albek, M. (2015). Determination effects of impervious areas on urban watershed. Environmental Science and Pollution Research, 22(3), 2272–2286. https://doi.org/10.1007/s11356-014-3345-2.
Weng, Q. (2012). Remote sensing of impervious surfaces in the urban areas: requirements, methods, and trends. Remote Sensing of Environment, 117(2), 34–49. https://doi.org/10.1016/j.rse.2011.02.030.
Wu, C. S., & Murray, A. T. (2003). Estimating impervious surface distribution by spectral mixture analysis. Remote Sensing of Environment, 84(4), 493–505. https://doi.org/10.1016/S0034-4257(02)00136-0.
Xian, G. (2008). Satellite remotely-sensed land surface parameters and their climatic effects for three metropolitan regions. Advances in Space Research, 41(11), 1861–1869. https://doi.org/10.1016/j.asr.2007.11.004.
Xian, G., & Crane, M. (2005). Assessments of urban growth in the Tampa Bay watershed using remote sensing data. Remote Sensing of Environment, 97(2), 203–215. https://doi.org/10.1016/j.rse.2005.04.017.
Xian, G., Crane, M., & Su, J. (2007). An analysis of urban development and its environmental impact on the Tampa Bay watershed. Journal of Environmental Management, 85(4), 965–976. https://doi.org/10.1016/j.jenvman.2006.11.012.
Yan, Z. G., Teng, M. J., He, W., Liu, A. Q., Li, Y. R., Wang, P. C. (2019). Impervious surface area is a key predictor for urban plant diversity in a city undergone rapid urbanization. Science of The Total Environment, 650, 335–342. https://doi.org/10.1016/j.scitotenv.2018.09.025.
Yue, W. Z. (2009). Improvement of urban impervious surface estimation in Shanghai using Landsat7 ETM+ data. Chinese Geographical Science, 19(3), 283–290. https://doi.org/10.1007/s11769-009-0283-x.
Zhao, J. (2008). Landscape pattern change and its environmental response across multiple spatial scales in tidal plain
Acknowledgments
We thank the Key Laboratory of Geographic Information Science, Ministry of Education of East China Normal University, for providing land use data of Shanghai.
Funding
The study was funded by the National Natural Science Foundation of China (41101550).
Author information
Authors and Affiliations
Corresponding author
Additional information
Publisher’s note
Springer Nature remains neutral with regard to jurisdictional claims in published maps and institutional affiliations.
Rights and permissions
About this article
Cite this article
Fu, B., Peng, Y., Zhao, J. et al. Driving forces of impervious surface in a world metropolitan area, Shanghai: threshold and scale effect. Environ Monit Assess 191, 771 (2019). https://doi.org/10.1007/s10661-019-7887-0
Received:
Accepted:
Published:
DOI: https://doi.org/10.1007/s10661-019-7887-0