Abstract
The spatial resolution of exposure data has a substantial impact on the accuracy and reliability of seismic risk estimates. While several studies have investigated the influence of the geographical detail of urban exposure data in earthquake loss models, there is also a need to understand its implications at the regional scale. This study investigates the effects of exposure resolution on the European loss model and its influence on the resulting loss estimates by simulating dozens of exposure and site models (630 models) representing a wide range of assumptions related to the geo-resolution of the exposed asset locations and the associated site conditions. Losses are examined in terms of portfolio average annual loss (AAL) and return period losses at national and sub-national levels. The results indicate that neglecting the uncertainty related to asset locations and their associated site conditions within an exposure model can introduce significant bias to the risk results. The results also demonstrate that disaggregating exposure to a grid or weighting/relocating exposure locations and site properties using a density map of the built areas can improve the accuracy of the estimated losses.
Similar content being viewed by others
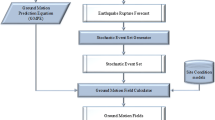
Avoid common mistakes on your manuscript.
1 Introduction
A common issue in earthquake risk models is related to whether the resolution of the exposure data is sufficiently detailed. The exposure information available from public resources is typically aggregated with limited information about the actual spatial distribution of assets (e.g., Dabbeek et al. 2020). Aggregated exposure refers to the case in which buildings in spatially extended region are, for the purposes of characterizing input ground motion, represented by a single location within that region. This implies shifting assets from their original locations, thus altering the local site properties and the distance to the earthquake sources, leading to inaccurate input ground motions and damage estimates. This matter is not independent from the resolution of the site model, which is itself aggregated across a spatial extent (e.g., 30 arc-seconds grid cell) such that a range of site conditions are represented by a single, often uncertain, property such as topographically-inferred 30-m averaged shear-wave velocity, VS30.
Though the practice of aggregating exposure may have its drawbacks in terms of accuracy and/or consistency with the seismic inputs, there are both practical and theoretical considerations that may necessitate it. The overarching consideration is the computational cost of the risk calculations, and the extent to which this impacts the users of the risk model. Running probabilistic loss calculations at a regional scale using high-resolution exposure models requires significant computational resources, in terms of both infrastructure and time. For applications in the insurance industry there is often a need for clients not only to retrieve rapid estimates of losses in the aftermath of an event, but also to run calculations repeatedly in order to explore both the uncertainties in the losses and their sensitivities. The cost of using high-resolution exposure models may be that one is unable to adequately characterize the epistemic uncertainties, which are now often represented by increasingly complex logic trees, or, in the case of stochastic event-based probabilistic loss calculations, be able to run a sufficient number of simulations to capture the tails of the distributions that may be significant for assessing risk in a region (i.e., the low probability, high consequence events). At the same time, even where the locations of the assets are known to a high level of accuracy, there may be many elements in the risk model that are known or modelled at a coarser resolution, such as site properties within a grid cell or geological unit, or the proportion of structural types within an administrative district. In these cases, it may not necessarily be true that the computational cost of using higher resolution exposure yields a greater return in terms of accuracy than that of a more coarsely aggregated model. The critical question, however, and one that we endeavour to address within this analysis, is whether the adoption of coarser scale exposure introduces systematic biases into the loss estimates and, if so, how these manifest and what can be done to mitigate them.
The issue of spatial resolution has been investigated at the urban level in several studies. For example, Bazzurro and Park (2007) found that aggregating portfolios at the zip code level led to an underestimation of recurrent small losses and overestimation of large rare losses. In particular, they observed that the impacts on portfolio loss due to aggregation were highest in the largest zip code regions. Similar conclusions were also obtained in the studies by Scheingraber and Käser (2019) and Bal et al. (2010). The latter study analyzed four levels of spatial resolution (i.e., district, postcode, sub-district and the geocell) and found that the mean damage at the district level (i.e., the lowest resolution) was the most inaccurate. Despite these effects, each of those studies considered spatial resolution to have a minimal influence when calculating portfolio mean loss/damage, due to an averaging effect of the over- and underestimation of the losses. However, these studies also indicated that location uncertainty could lead to inaccurate loss estimates (both in the mean and in the distribution) when larger regions are aggregated to a single location. Therefore, the first aim of this study is to investigate further the effect of exposure resolution beyond the urban scale, which is of particular importance for the national, regional and global studies of seismic risk (Crowley 2014).
A single point within an extensive spatial domain cannot be used to accurately represent the ground shaking hazard (including site conditions) that all assets within that domain might experience. Therefore, when the use of aggregated exposure is necessary, it is fundamental to determine a ground-shaking input that accounts for both the location and local site conditions that are closest to the conditions affecting most buildings in a spatial region (DeBock and Liel 2015). In this context, Bazzurro and Park (2007) experimented with population-based centroids, among other relocation strategies, but did not observe changes in the results compared to using only the geometric centroids. In particular, they found that either the centroids were close by chance or that the line between centroids was parallel to the dominant fault source, and thus the results were potentially influenced by their specific case study. Another challenge is determining the correct location when a region has two or more urban centres separated by large distances. Thus, predicting the best choice of location for administrative-based portfolios is not trivial. Currently, no evidence can be found in the literature on which method should be used to geolocate buildings and assign site properties for aggregated portfolios. Hence, the second aim of this study is to examine several relocation strategies and test them on large scale risk analyses.
The issues arising from low spatial resolution in exposure models have been managed in different ways, including modelling region-specific ground motions (e.g.,Bazzurro and Park 2007; Stafford 2012), and stochastic modelling of location uncertainty (Scheingraber and Käser 2019). However, such approaches require repetitive and extensive hazard calculations; accordingly, they are considered less feasible for large-scale probabilistic risk analyses. Other methods focused on the exposure component and associated soil properties; this includes manipulating data (i.e., relocation—Bazzurro and Park 2007) or refining the data's spatial resolution (i.e., disaggregation—Dabbeek and Silva 2020). More recently, suggestions have been made to spatially represent the exposure using Central Voronoidal Tessellations (CVT) to create exposure models with variable spatial resolutions (Gomez-Zapata et al. 2021; Pittore et al. 2020). This study will focus mainly on portfolio relocation and disaggregation methods as a way to treat the bias in risk arising from low spatial resolution.
In this study, we present a sensitivity analysis that explores the effect of spatial resolution in exposure models (including site conditions) on seismic risk analyses as part of the European Seismic Risk Model's (ESRM20, Crowley et al. 2019) testing activities. This analysis contributes to the overall understanding of the influence of location uncertainties on portfolio losses at the regional level and identifies which modelling strategy results in higher accuracy and has the least computational expense. The sensitivity analysis framework includes 35 countries (see 'Appendix') within the European exposure model (Crowley et al. 2020a) and multiple test cases, exploring different spatial resolutions and strategies for best configuring the site and exposure models. Each test case is used to calculate portfolio loss for specific return periods and the average annual loss (AAL). These results are compared to the benchmark loss, calculated with the 30 arc-seconds resolution exposure model (the highest resolution achievable with the input site characterization model) to identify the method that produces a desirable balance between accuracy and need for resources.
2 Case study: European exposure and site models
The underlying exposure data used in this study has been obtained from the European exposure model developed by Crowley et al. (2020a). Exposure models that describe the spatial distribution of the number, replacement value and occupants within residential, industrial and commercial buildings for 44 countries in Europe have been developed using primarily public census data. The buildings in the exposure models have been grouped into building classes (as a function of parameters that are relevant to define their seismic performance) and classified using the GEM Building Taxonomy (Silva et al. 2021). This exposure model is being used in the European Seismic Risk Model 2020 (ESRM20 – Crowley et al. 2019). In this study, we considered 35 countries (listed in 'Appendix') out of 44 countries in Europe. The excluded cases are relatively small countries that do not allow testing the spatial resolution according to the proposed workflow.
The information within the European exposure models is aggregated by administrative zone with a resolution that varies across countries and occupancies (i.e., residential, commercial and industrial). The maximum available resolution of residential and commercial administrative units is illustrated in Fig. 1. It is possible to observe large differences in the surface areas across countries and occupancy levels. For example, in Portugal, France and Italy the divisions appear quite detailed (mean area of 60 km2), compared to Turkey, Spain and Finland where the areas are considerably larger (mean area of 10,000 km2), particularly for the commercial exposure.
As can be seen in Fig. 1, the highest administrative resolution is dictated by the residential exposure, with all countries having either similar or lower levels of resolution for the commercial exposure. The industrial exposure, not shown in Fig. 1, typically has the highest resolution as it has been developed in most countries on a 30 arc-seconds grid (Sousa et al. 2017). The residential building stock contributes to at least 65% of the total replacement value of the European building stock, and thus we only employed the residential exposure model herein, but it has been aggregated to lower administrative levels, where necessary, to reproduce the resolution seen in the commercial models.
The site model used in the analyses has been developed for ESRM20 and makes use of two proxy datasets: topography and geology. The linear site amplification term within the shallow crustal ground motion model (GMM) adopted as the backbone for the GMM logic tree is implemented here as a direct function of the topographic slope and the surface geological unit, further details of which can be found in Crowley et al. (2020b). The topographic slope is derived using the seamless topography/bathymetry data set produced by the General Bathymetric Chart of the Oceans (GEBCO), while the geological data is taken from the harmonized geological map for Europe. Both data sets are rendered onto a regular 30 arc-seconds grid. In addition to the slope and geology dependent site amplification model used for shallow crustal seismicity, some GMMs derived for other tectonic environments (e.g., stable craton regions, subduction zones, deep seismicity etc.) require the definition of the site properties in terms of the VS30. This too, is inferred from the topographic slope using the relation between slope and VS30 proposed by Wald and Allen (2007) and revised by (Allen and Wald 2009).
3 Sensitivity analysis design
The sensitivity of the losses to the spatial resolution is explored using different sets of exposure (administrative and gridded distributions) with a total of 18 test cases per country. Portfolio losses in terms of the AAL and return period losses are estimated using the event-based risk calculator of the OpenQuake-Engine, an open-source software for seismic hazard and risk assessments (Pagani et al. 2014; Silva et al. 2014). Note that the OQ-engine can also compute the aggregate AAL of the portfolio using the classical PSHA-based calculator; however, the event-based calculator can compute portfolio loss curves (i.e. aggregate losses to the portfolio for a range of return periods) and has been better optimized for large scale calculations such as those undertaken herein. We simulate thirty thousand stochastic catalogues, each with one-year of seismicity, as well as ground motions at the exposure locations for each simulated event, using the ESHM13 (Woessner et al. 2015) source model with the ESHM20 ground-motion models (Kotha et al. 2020; Weatherill et al. 2020; Weatherill and Cotton 2020). The losses for each event are then calculated by combining the simulated ground motions with the exposure model, together with a set of vulnerability functions that represent the probability of loss conditional on the level of ground shaking for each building class in the exposure model (Martins and Silva 2020; Crowley et al. 2021). It is worth noting here that ground motion spatial correlation and building-to-building damage correlation has not been considered in this study. Spatial correlation is less impactful for large-scale risk analysis; nonetheless, the effects become more profound in smaller portfolios, especially in the tails of the loss distribution. Spatial clusters spread over a large region might be strongly or weakly correlated depending on the separating distance. This creates an averaging effect that reduces the impact of spatial correlation in the ground motion residuals in the losses for rare return periods (Silva 2019). The performance of the various exposure datasets is measured with respect to the losses obtained from a benchmark exposure (30 arc-seconds gridded exposure), which is assumed to be the optimum parameterizable resolution at present for regional-scale risk analysis corresponding to the resolution of the input site model.
3.1 Administrative workflows
In the type of exposure considered herein, each administrative unit is presented by a single location and site property. We considered four workflows (wf1, wf2, wf3, wf4) depending on the choice of location and site conditions. These properties are either taken using the geometric centroid of the admin unit or are obtained using 30 arc-seconds grid of the built-up area density grid, interpolated from the 250 × 250 m resolution built-up area density map (Pesaresi et al. 2015). wf1 presents the base model in which the values simply represent both exposure latitude and longitude locations and site properties at the geometric centroid of the admin unit. wf2 uses the geometric centroids for the locations, whereas the site properties are represented by a (built-up area) density weighted-average of all the site conditions in the 30 arc-seconds grid cells covering the admin unit. In wf3, a density weighted-centroid of all the 30 arc-seconds grid cells is used for the locations and the density-weighted average values adopted for the site conditions. Lastly, in wf4 the locations are placed at the maximum built-up area density within the admin unit, whereas the sites remain with a density weighted-average (see Table 1 for a summary of these workflows).
In this process, the aim is to shift locations or sites to where the greatest proportion of the population lives by giving denser cells higher weights and the unoccupied cells a zero weight. These workflows were designed to allow independent testing of the effects of location and site conditions.
Figure 2 provides a comparison between the locations resulting from the use of different weighting methods for the second administrative level in Spain. Significant locational differences can be observed in many regions. In particular, the maximum density and density weighted-average locations seem to match the location of major Spanish cities marked with the cross symbol ( +), though the maximum density method fails to represent regions with more than one major city. However, the latter is true for any method in which a region with several large cities is represented by a single point. Additionally, the geometric centroids often do not match the populated places as expected, as this method depends only on the geometry of the administrative boundary. Figure 3 compares the VS30 values calculated using the geometric centroid location used in wf1 (left) and the density-weighted average property used in wf 2, 3, 4 (right). In general, the geometric centroid method seems to estimate higher VS30 values across Spain, indicating that the choice of weighting can introduce a systematic change in site properties rather than a random one.
3.2 Gridded workflow
The gridded exposure is a regularly spaced grid of points disaggregated from the base model, using the Global Human Settlement Layer (GHSL) 250 × 250 m resolution built-up areas density map (Pesaresi et al. 2015). For this type of exposure (hereafter termed wf5), building locations and site properties correspond to the centre of the grid cell which is considered with six resolutions: 30, 60, 120, 240, 480 and 960 arc-seconds (note that 30 arc-seconds grid cell ranges between 768 m EW and 926.6 m NS at a latitude of 35˚N to 391.6 m EW and 926.6 m NS at a latitude of 65˚N), all of which were downsampled from the 250 × 250 m resolution built-up area density map. It is important to note that the maximum resolution was restricted to 30 arc-seconds in order to manage the computational demand of the risk calculations. On the other hand, the lowest resolution was limited to 960 arc-seconds, a level that is similar to the resolution of some administrative units. Samples of the gridded exposure for Spain are demonstrated in Fig. 4, and a summary of all of the considered test cases is presented in Table 1.
4 Sensitivity analysis results
4.1 Effect of administrative-based exposure on portfolio loss
The first set of analyses focuses on the impact of admin resolution on the cumulative portfolio loss following the most common type of aggregated exposure models (wf1). Figure 5 illustrates the difference in AALs (relative to the results with the benchmark 30 arc-seconds gridded exposure model) for Belgium, Bosnia and Herzegovina, Greece, Italy and Switzerland obtained from administrative divisions 1, 2 and 3. These countries have been selected as they have the highest residential, industrial and commercial exposure resolution (i.e. administrative level 3) within the European exposure model. From this figure, a clear association can be observed between admin resolution and the percentage change in AAL. The largest bias occurs at admin1 (mostly underestimation), followed by the higher resolutions (admin2 and 3). Although the variation in AAL is smallest at admin3, the bias is not negligible in some countries. One of the reasons for which the bias varies for the same admin level is the surface area discrepancies between countries. This discrepancy can be observed in commercial exposure in Fig. 1 between Spain and Turkey, which are presented at the same admin level (admin1). Such large aggregated portfolios are likely to be associated with higher uncertainty in building locations and site conditions. This larger uncertainty is due to the inability of a single point to adequately characterize the variability of site conditions and building locations across such a large surface area.
By manipulating the exposure locations and site properties (see descriptions of wf2, wf3 and wf4), the accuracy of the AAL is expected to improve. The difference in AAL (relative to the benchmark model) obtained from using the different workflows for several European countries (and where the losses have been aggregated to admin1 level) are shown in Fig. 6. In this chart, there are countries for which the weighted workflows perform better than the geometric centroid (e.g., Greece, Portugal, Turkey, Iceland and Bosnia and Herzegovina), and a few countries where the results seem insensitive to the choice of weighting scheme (e.g., Bulgaria and Croatia).
Considering the numerous case studies analyzed herein, we used an index to evaluate the overall performance of these models. The index describes the frequency distribution of performance between the workflows, as reported in Table 2. In other words, the index measures how frequently (i.e., in how many countries) a model ranks as the best, second-best, third-best and worst option. According to this index, the best performing model is wf3 followed by wf2, wf4 and wf1. The highest index value indicates that wf3 (weighted centroids and sites) stands as the best (1st rank = 13) and also as the second-best model (2nd rank = 13). On the other hand, the lowest index value indicates that wf1 (geometric centroids and their site conditions) is the least effective model, even if it ranks as the best model in 7 cases. It should be noted that the meaning of the index is limited if the margin of difference is small (see, for example, Croatia in Fig. 6). An alternative is to measure the sum of absolute changes in AAL for the 35 countries, which are 940%, 830%, 600%, 730%, for each of wf1, 2, 3 and 4. Accordingly, these values confirm that there is a significant margin in the performance.
The administrative workflows were also tested at the sub-national level (admin1, admin2 and admin3). Figure 7 compares the probability density distribution of the regions/zones at admin1, 2 and 3 in terms of AAL change between wf1 and the other workflows. The total number of units per admin level are 670, 1611 and 15,106, respectively. Significant deviations in AAL with respect to the benchmark case can be observed, particularly for admin1 (up to ± 80%). These deviations are mostly skewed to the left, implying a bias towards the underestimation of losses. One reason for this underestimation has to do with basic geography: towns/cities develop mostly in flat(ter) ground (e.g., upland valleys, river plains, coastal areas) that are associated with lower VS30, predominantly Tertiary and Quaternary sediments and deeper basins. On the other hand, the site values at the geometric centroid of a region can correspond to any geomorphological condition. Using the GHSL to weight both the location and properties of the sites skews the sites toward the conditions that favour higher soil amplification. This particular trend has already be seen in the comparison of the Vs30 values assigned to admin2 units for Spain in Fig. 3. Another factor that explains the underestimation of losses is the artificial correlation which increases the probability of smaller losses in the lower tail of the loss curve, as explained in the next section. It is evident that the dispersion in AAL difference is highest in wf1 (σ = 0.39) and the lowest in wf3 (σ = 0.32), as compared with the other workflows. The scores (51, 65, 72, 62 for each workflow, respectively) of the performance index also suggest that wf3 is the best model. Up to admin2, the discrepancies between workflow distributions are still evident, yet a convergence between these methods becomes noticeable at admin3 (bottom row). In other words, when calculations are carried out at an administrative level of a relatively fine resolution, the difference between methods becomes less noticeable. Based on Fig. 7, weighting both the locations and site properties seems equally important.
Besides portfolio AAL, another common measure tested here is portfolio loss for a specific return period. Figure 8 shows the change in loss for different loss return periods (50, 100, 200, 500, 1000, 2000 and 5000 years) for each of the four administrative workflows calculated using the admin1 exposure. Similar to observations by Bazzurro and Park (2007) and Scheingraber and Käser (2019), aggregation tends to underestimate losses for events with shorter return periods (i.e., 50 years) and overestimate losses for longer return periods (i.e., 2000). Using admin1 exposure means all structures within the area are assigned to the same ground motion values; introducing such artificial correlation broadens the uncertainties in the tails of the distribution, meaning higher probabilities of high losses and higher probabilities of lower losses than the case when correlations are weaker or absent. Both the median (black line) and the average value (hollow circles) tend to move in the positive direction with increasing return period, showing a tendency to overestimate loss for the rare loss events. This increasing trend seems to be slower for countries/regions where seismic hazard is relatively low (e.g. Finland, Sweden, Norway), as these data points can be observed in the negative range for the 2000 year event. As for the median and mean values resulting from the different workflows at any given return period, it appears that all workflows have a similar performance.
4.2 Effect of grid-based exposure on portfolio loss
The previous sections demonstrated that coarse administrative exposure could bias portfolio loss. Despite the improvements brought about by relocating exposure and site conditions in wf3, aggregation effects remain a concern, especially for exposure models developed at the first administrative division (e.g. Spain, Turkey, Austria). High resolution gridded exposure models are less susceptible to aggregation effects; however, they usually require substantial computational resources. Therefore, it is essential to identify the optimal disaggregation level to maintain a reasonable balance between accuracy and computational demand. Figure 9 presents a comparison between the grid and administrative-based resolutions for Italy, Switzerland, Albania, Greece, Belgium, and Bosnia and Herzegovina. Admin resolutions (admin1, admin2 and admin3) correspond to wf3 (density weighted-average). The results demonstrate that the 120 and 240 arc-seconds range or the third administrative level generally leads to higher accuracy than the lower resolution grids (480 and 960 arc-seconds) and coarser administrative levels (admin2 and admin1). Also, it can be noticed that the improvements below 120 arc-seconds are minor, suggesting that resolutions smaller than (approximately) 2 × 2 km2 grid are largely insignificant for calculating the AALs at the national scale. Overall, the sum of absolute change in AAL (35 countries) for the 60, 120, 240, 480 and 960 arc-seconds gridded exposure is 35, 48, 140, 185 and 418%, respectively. Note that the low AAL bias for Italy at admin1 resulted here by chance as the underestimated and overestimated losses counterbalance each other.
In terms of the effect of the spatial resolution on specific event losses (i.e., 50, 100, 200, 500 and 1000 years), Fig. 10 illustrates a similar trend to that observed for the AAL (Fig. 7), where the level of bias becomes more evident at lower resolutions (480, 960 and admin 1). The influence of artificial correlation on the losses becomes more evident as the spatial resolution decreases. The frequent loss events (50, 100 years) appear to be more sensitive to spatial resolution. Generally, smaller, more frequent events lead to ground shaking that covers smaller extents and thus, any location shift in the assets can change the level of estimated shaking at the site (and therefore damage and loss) dramatically. Additionally, there is a high sensitivity of small numbers to change. It should be mentioned that this sensitivity might be influenced by the types of structures dominating the portfolio as well. As short period motion attenuates more rapidly than long-period motion, regions with low-rise structures (shorter vibration period) will be more sensitive to this attenuation. This might have an effect at the subnational level, where there can be noticeable changes in construction types, for example, metropolitan regions (with more high-rise structures) versus rural regions (with more low-rise structures).
5 Summary of results
A summary of the impacts of the different exposure models and site models tested in this study on the national and the regional (i.e., subnational) AAL is presented in Fig. 11. The numbers shown indicate the percent absolute difference of AAL with respect to the benchmark case and correspond to the average difference of all countries/administrative units that were analyzed. The lower performance of coarser grids (480 and 960 arc-seconds grids) is evident for both regional and national losses, as the differences in AAL increase from values less than 5% up to 12–27%. A similar outcome can be observed for the three administrative levels (admin1, admin2 and admin3), as calculations carried out at admin3 (highest admin resolution) led to the smallest AAL differences, for both regional (12–16% against 22–31% at admin1) and national (11–14% against 18–27% at admin1) values. The higher performance of wf3 stands out as well, with its AAL differences being the lowest of the four workflows (wf1, wf2, wf3, wf4) for each of the three admin levels at the regional and national levels.
6 Discussion
6.1 Portfolio size, hazard and site property effects on the estimated loss
Previous studies evaluating the effect of spatial resolution on portfolio loss assessment have observed some inaccuracies associated with large urban portfolios. In this study, we explored the dependence of the size of the region, spatial variability of hazard and soil and population distribution on the accuracy of losses. Figure 12 demonstrates the relationship between the area of the administrative divisions in km2 and the corresponding average change in AAL relatively to the gridded 30 arc-seconds model. This figure was generated based on 23,000 regions from admin1, admin2 and admin3. Areas on the x-axis were grouped into several logarithmically-spaced bins. As can be seen, the bias in the AAL increases with the increase in the administrative area. Interestingly, the standard deviation values (named sigma) in Fig. 12 indicate a significant scatter around the average, demonstrating that the bias in equally sized regions can be much higher or lower than the average.
These deviations can be explained in part by the variability of hazard. Figure 13 illustrates the relationship between the hazard coefficient of variation (CoV) and the corresponding average change in AAL with respect to the 30 arc-seconds model. The hazard CoV is calculated based on the 500 years return period hazard map on rock (5 × 5 km grid) and reflects how much the hazard changes within a region. It is possible to observe that the AAL difference is highly sensitive to the hazard CoV. Closer analyses have shown that high variations come mostly from the biggest regions where the hazard has a larger variation in space. This does not imply that there is high variability in hazard for every large region; for example, if the hazard is uniform, the CoV of hazard can be relatively low. This explains why some regions are less sensitive to the choice of location (e.g., geometric centroid vs density weighted-average) despite their large size. For example, Crete island in Greece has a relatively large extent (area of 8336 km2) with relatively uniform hazard levels (ranging from 0.37—0.42 g with 0.02 g CoV—on rock). Consequently, despite the location considered (geometric centroid, density-weighted average centroid and maximum density location—wf2, wf3 and wf4) similar losses were observed.
Similar to what was described for the seismic hazard, site properties change from location to location, which makes specific regions more susceptible to this variability than others. In order to validate this, it is essential to isolate the effects of the hazard, by only considering regions with relatively uniform hazard (i.e., CoV smaller than 0.1) that are large enough to allow sites properties to change (i.e. larger than 500 km2). Figure 14 illustrates the relationship between site effects CoV (where site effects are defined in terms of the Vs30) and the corresponding average change in AAL with respect to the 30 arc-seconds model. The AAL difference seems to increase when the variability of soil is higher. It should be pointed out that, unlike the seismic hazard, soil properties can change abruptly even between neighbouring sites spaced only a few kilometres (or even hundreds of metres) apart; in this sense, the CoV is only indicative in broad terms and cannot fully reflect the actual sensitivity.
6.2 Exposure method and calculation performance
As outlined in the introduction to this work, the main challenge with using high-resolution exposure in probabilistic seismic risk analyses is the high computational cost. To give a sense of the scale of the costs, consider the computational configuration adopted for the current calculations, for which we used a high-performance cluster with 512 GB of RAM and 60–80 threads. This took a total of 48 h to run 35 countries and 1.5 TB of memory to generate ground motion fields using the highest resolution model (30 arc-seconds grids). Generally, the user's needs and the available resources are the main factors that determine the optimal level of resolution. For example, for producing national loss maps without the need to run the model repeatedly, the required time and memory to run it at highest resolution available might be reasonable. However, from a scientific perspective, the tradeoff between the model's detail and accuracy is also essential. For example, the high computational cost of high-resolution models typically prohibits the possibility of running multiple branches of the logic tree, or at least potentially undersampling the number of branches, then the reduction in computational demand is necessary in order to be able to quantify the impact of the epistemic uncertainty on the loss calculations. For an industry use case in which a client may wish to repeatedly run a particular loss analysis or retrieve loss estimates rapidly, a reduction in resolution without losing much of the accuracy can be beneficial. For example, the analysis shows that a reduction in resolution to 240 arc-seconds achieved a 95.5% accuracy level and required 64 times less memory than the 30 arc-seconds model. The time needed to prepare all the input files (exposure and site) is considered minor compared to the time needed for the actual calculations. The exact time depends on the size and the number of administrative regions of the input country. For example, it took about 5 min to prepare the 30 arc-seconds exposure model for Turkey (highest resolution) from admin1 exposure. Note that the run time and memory requirements reported represent the computational hardware, the OpenQuake-Engine version and calculation settings used in this study.
7 Conclusions
This study has investigated the influence of exposure spatial resolution on seismic risk analyses for large building portfolios. Eighteen different methods for modelling exposure and site models were simulated and tested in 35 countries in Europe. Twelve of these methods are based on administrative distributions and consist of three resolutions (admin1, admin2 and admin3), and four workflows to assign buildings locations and site properties: a) geometric centroid and closest site property b) geometric centroid and density weighted-average sites c) density weighted-average location and sites d) maximum density location and density weighted-average sites. The other six methods are grid-based with spatial resolutions of 30, 60, 120, 240, 480, 960 arc-seconds. All the workflows described in this study and more can be readily configured to allow risk modellers to explore these approaches themselves using a free and open set of tools:
-
Exposure Disaggregation ToolFootnote 1 (https://github.com/GEMScienceTools/spatial-disaggregation).
-
Site Preparation Tool (https://gitlab.seismo.ethz.ch/efehr/esrm20_sitemodel).
The sensitivity analysis demonstrated that the spatial resolution of exposure has a significant impact on probabilistic seismic risk at the national and regional scale. For the national AAL, using admin1, admin2 and admin3 exposure with geometric centroid and closest site properties (wf1) lead to an average bias of 27%, 19% and 15%, respectively. The 60, 120, 240, 480, 960 arc-seconds gridded exposure models leads to an average bias per country of 1%, 1.5%, 4.5%, 14%, 27%, respectively. Based on these results, it is worth increasing the resolution to 120 to 240 arc-seconds in order to keep the bias below 5%. However, resolutions higher than 120 arc-seconds did not bring significant improvements and required a relatively large amount of computational resources. At the resolutions higher than that of the national level (i.e., provinces, cities, etc.), the spatial resolution has more significant effect on the AAL. Therefore, lower ranges of the recommended spatial resolutions (i.e. 120 arc-seconds or finer) can be considered when assessing risk at the sub-national level.
The results also demonstrated that it is possible to reduce the bias for administrative-based exposure models by using the density weighted-average location and site properties (wf3). For admin1 level, the AAL bias reduced from 27 to 18%, on average, per country when using this workflow. This method seems to work when there is a high sensitivity to the choice of location caused by the high variability of hazard and soil properties within a region. In most cases, these regions are large enough (i.e., area larger than 500 km2) to allow hazard and site properties to change over space. Hence, the higher the resolution of the admin level, the less likely it is that any weighting method could significantly improve the accuracy of portfolio loss.
Before extrapolating results to other regions, however, it is important to keep in mind the role of site model resolution, ground motion attenuation and seismogenic sources. Although this study explored a handful of strategies for modelling exposure resolution and site properties, there are others to be investigated, some of which are also available in the Site Preparation Tool. For example, the gridded exposure can be improved by weighting site properties within each grid cell or considering variable grids denser around the main faults and where site properties tend to vary. In addition, while this work has focused on regional scale risk studies, further analysis should be undertaken at sub-regional and urban scale in order to understand where a suitable balance can be struck between exposure resolution, uncertainty characterization and computational cost, when more detailed information may be available (e.g., Fayjaloun et al. 2021). Since the effects of data resolution depend strongly on the variation in the hazard (see the discussion), future studies should focus on mitigating the bias at the ground motion level.
This sensitivity analysis is carried on as part of the testing activities of the European Seismic Risk Model (ESRM20, Crowley et al. 2019), initiated within the European Horizon 2020 Project SERA (www.sera-eu.org), and continuing under the umbrella of the European Facilities for Earthquake Hazard and Risk (EFEHR) Consortium (www.efehr.org). The findings will also be of interest to the Global Earthquake Model initiative (GEM) and seismic risk modellers interested in understanding the implications of modelling decisions related to exposure spatial resolution.
Availability of data and material
The following GitLab repository contains the residential exposure data used herein: https://gitlab.seismo.ethz.ch/efehr/esrm20_exposure/-/tree/master/_exposure_models
Notes
Note that the Exposure Disaggregation Tool uses WorldPop population (www.worldpop.org) by default, but it supports other raster datasets including the GHS built-density layer used herein available for download at: https://gitlab.seismo.ethz.ch/efehr/esrm20_exposure/-/tree/master/spatial_disaggregation.
References
Allen TI, Wald DJ (2009) On the use of high-resolution topographic data as a proxy for seismic site conditions (VS30). Bull Seismol Soc Am 99:935–943. https://doi.org/10.1785/0120080255
Bal IE, Bommer JJ, Stafford PJ, Crowley H, Pinho R (2010) The Influence of Geographical Resolution of Urban Exposure Data in an Earthquake Loss Model for Istanbul. Earthq Spectra 26:619–634. https://doi.org/10.1193/1.3459127
Bazzurro P, Park J (2007) The effects of portfolio manipulation on earthquake portfolio loss estimates. In: Proceedings of the 10th international conference on applications of statistics and probability in civil engineering. Tokyo, Japan
Silva V, Brzev S, Scawthorn C, Yepes-Estrada C, Dabbeek J, Crowley H (2021) A building classification system for multi-hazard risk assessment. Int J Disaster Risk Sci (Submitted)
Crowley H (2014) Earthquake risk assessment: present shortcomings and future directions. Geotech Geol Earthq Eng 34:515–532. https://doi.org/10.1007/978-3-319-07118-3_16
Crowley H, Rodrigues D, Silva V, Despotaki V, Martins L, Romão X, Castro J, Pereira N, et al (2019) The European Seismic Risk Model 2020 (ESRM 2020). In: 2nd International Conference on Natural Hazards & Infrastructure (CONHIC 2019). Chania, Greece
Crowley H, Despotaki V, Rodrigues D, Silva V, Toma-Danila D, Riga E, Karatzetzou A, Fotopoulou S et al (2020a) Exposure model for European seismic risk assessment. Earthq Spectra. https://doi.org/10.1177/8755293020919429
Crowley H, Weatherill G, Riga E, Pitilakis K, Roullé A, Tourlière B, Lemoine A, Gracianne Hidalgo C (2020b) D26.4 Methods for estimating site effects in risk assessments. SERA Project Deliverable. Available from: http://www.sera-eu.org/en/Dissemination/deliverables/
Crowley H, Silva V, Martins L, Romão X, Pereira N (2021) Open Models and Software for Assessing the Vulnerability of the European Building Stock. In: 8th International Conference on Computational Methods in Structural Dynamics and Earthquake Engineering (COMPDYN 2021). Athens, Greece
Dabbeek J, Silva V (2020) Modeling the residential building stock in the Middle East for multi-hazard risk assessment. Nat Hazards 100:781–810. https://doi.org/10.1007/s11069-019-03842-7
Dabbeek J, Silva V, Galasso C, Smith A (2020) Probabilistic earthquake and flood loss assessment in the Middle East. Int J Disaster Risk Reduct 49:101662. https://doi.org/10.1016/j.ijdrr.2020.101662
DeBock DJ, Liel AB (2015) A comparative evaluation of probabilistic regional seismic loss assessment methods using scenario case studies. J Earthq Eng 19:905–937. https://doi.org/10.1080/13632469.2015.1015754
Fayjaloun R, Negulescu C, Roullé A, Auclair S, Gehl P, Faravelli M (2021) Sensitivity of earthquake damage estimation to the input data (soil characterization maps and building exposure): case study in the luchon valley. France 11:249. https://doi.org/10.3390/GEOSCIENCES11060249
Gomez-Zapata JC, Brinckmann N, Harig S, Zafrir R, Pittore M, Cotton F, Babeyko A (2021) Variable-resolution building exposure modelling for earthquake and tsunami scenario-based risk assessment An application case in Lima, Peru. Nat Hazards Earth Syst Sci Discuss. https://doi.org/10.5194/NHESS-2021-70
Kotha SR, Weatherill G, Bindi D, Cotton F (2020) A regionally-adaptable ground-motion model for shallow crustal earthquakes in Europe. Bull Earthq Eng 18:4091–4125. https://doi.org/10.1007/s10518-020-00869-1
Martins L, Silva V (2020) Development of a fragility and vulnerability model for global seismic risk analyses. Bull Earthq Eng. https://doi.org/10.1007/s10518-020-00885-1
Pagani M, Monelli D, Weatherill G, Danciu L, Crowley H, Silva V, Henshaw P, Butler L et al (2014) Openquake engine: an open hazard (and risk) software for the global earthquake model. Seismol Res Lett 85:692–702. https://doi.org/10.1785/0220130087
Pesaresi M, Ehrilch D, Florczyk AJ, Freire S, Julea A, Kemper T, Soille P, Syrris V (2015) GHS built-up grid, derived from Landsat, multitemporal (1975, 1990, 2000, 2014). In: Eur. Comm. Jt. Res. Cent. (JRC), . http://data.europa.eu/89h/jrc-ghsl-ghs_built_ldsmt_globe_r2015b. Accessed 24 Apr 2019
Pittore M, Haas M, Silva V (2020) Variable resolution probabilistic modeling of residential exposure and vulnerability for risk applications. Earthq Spectra 36:321–344. https://doi.org/10.1177/8755293020951582
Scheingraber C, Käser MA (2019) The impact of portfolio location uncertainty on probabilistic seismic risk analysis. Risk Anal 39:695–712. https://doi.org/10.1111/risa.13176
Silva V (2019) Uncertainty and correlation in seismic vulnerability functions of building classes. Earthq Spectra 35:1515–1539. https://doi.org/10.1193/013018EQS031M
Silva V, Crowley H, Pagani M, Monelli D, Pinho R (2014) Development of the openquake engine, the global earthquake model’s open-source software for seismic risk assessment. Nat Hazards 72:1409–1427. https://doi.org/10.1007/s11069-013-0618-x
Sousa L, Silva V, Bazzurro P (2017) Using open-access data in the development of exposure data sets of industrial buildings for earthquake risk modeling. Earthq Spectra 33:63–84. https://doi.org/10.1193/020316eqs027m
Stafford PJ (2012) Evaluation of structural performance in the immediate aftermath of an earthquake: a case study of the 2011 Christchurch earthquake. Int J Forensic Eng 1:58. https://doi.org/10.1504/ijfe.2012.047447
Wald DJ, Allen TI (2007) Topographic slope as a proxy for seismic site conditions and amplification. Bull Seismol Soc Am 97:1379–1395. https://doi.org/10.1785/0120060267
Weatherill G, Cotton F (2020) A ground motion logic tree for seismic hazard analysis in the stable cratonic region of Europe: regionalisation, model selection and development of a scaled backbone approach. Bull Earthq Eng 18:6119–6148. https://doi.org/10.1007/s10518-020-00940-x
Weatherill G, Kotha SR, Cotton F (2020) A regionally-adaptable “scaled backbone” ground motion logic tree for shallow seismicity in Europe: application to the 2020 European seismic hazard model. Bull Earthq Eng 18:5087–5117. https://doi.org/10.1007/s10518-020-00899-9
Woessner J, Laurentiu D, Giardini D, Crowley H, Cotton F, Grünthal G, Valensise G, Arvidsson R et al (2015) The 2013 European Seismic Hazard Model: key components and results. Bull Earthq Eng 13:3553–3596. https://doi.org/10.1007/s10518-015-9795-1
Funding
The work presented herein has received funding from the European Union’s Horizon 2020 research and innovation program through the research projects (1) “SERA” Seismology and Earthquake Engineering Research Infrastructure Alliance for Europe, under Grant agreement No. 730900 and (2) “RISE” Real-time Earthquake Risk Reduction for a Resilient Europe, under grant agreement No. 821115.
Author information
Authors and Affiliations
Corresponding author
Ethics declarations
Conflict of interest
Not applicable.
Code availability
All the workflows described in this study can be readily configured to allow risk modellers to explore these approaches themselves using a free and open set of tools: Exposure Disaggregation Tool (https://github.com/GEMScienceTools/spatial-disaggregation) and Site Preparation Tool (https://gitlab.seismo.ethz.ch/efehr/esrm20_sitemodel).
Additional information
Publisher's Note
Springer Nature remains neutral with regard to jurisdictional claims in published maps and institutional affiliations.
Appendix
Appendix
This appendix provides the list of 35 countries and the available administrative level used in the analyses (see Table 3). Note that the highest resolution for each country is given by the highest resolution at which the residential exposure data is originally made available. Lower levels of resolution have been produced by aggregating the data at lower administrative levels using available shapefiles.
Rights and permissions
About this article
Cite this article
Dabbeek, J., Crowley, H., Silva, V. et al. Impact of exposure spatial resolution on seismic loss estimates in regional portfolios. Bull Earthquake Eng 19, 5819–5841 (2021). https://doi.org/10.1007/s10518-021-01194-x
Received:
Accepted:
Published:
Issue Date:
DOI: https://doi.org/10.1007/s10518-021-01194-x