Abstract
Crop yield has been analyzed under different hydrological conditions by many researchers. However, little information is available about the behavior of rice yield for different conditions during its growth. This study investigated the behavior of rice yield under varying hydrological conditions in two regions of India, one rainfed and the other irrigated, for the period 2000–2018. Additionally, it examined how individual, coincidental, and sequential compound extremes, such as rainfall, temperature, and soil moisture, affected rice yield. Four individual, two coincidental, and two sequential compound extremes regression models were developed. These models were designed with yield as a function of individual and compound extremes. Individual extreme models focused on heat and water metrics independently, while compound extremes occurred when heat and water stress coincided or followed each other closely. Linear panel models were used to assess the dependency of rice yield on hydrological parameters. Results indicate that excessive heat negatively affects rice yield, particularly when coupled with low soil moisture. However, excess soil moisture mitigates heat-related damage, highlighting the significance of controlling soil moisture levels. Additionally, coincidental compound extremes pose greater threats to rice yield than sequential ones. The study underscores the importance of considering geographical variations and hydrological variables in understanding crop yield behaviour. Overall, the findings suggest the potential for optimizing soil moisture management to enhance rice yield amidst changing climatic conditions.
Similar content being viewed by others
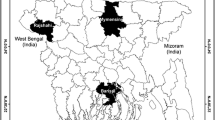
Avoid common mistakes on your manuscript.
1 Introduction
Climate change is anticipated to introduce new trends in the future, with extreme events becoming more frequent and severe (Das et al. 2022; Dash et al. 2007; Praveen et al. 2020). These extremes, such as heat waves and floods, are expected to escalate, particularly in regions like India, which is highly susceptible to such occurrences (Gershunov et al. 2013; Hasan et al. 2023; Meehl et al. 2000; Sienz et al. 2012; Sillmann et al. 2013; Yaduvanshi et al. 2021).The country has been one of the high-risk zones for these occurrences. For the recent 30-year period (1986–2015), temperatures of the warmest day and the coldest night of the year have rised by about 0.63 °C and 0.4 °C, respectively (Krishnan et al. 2020). India has already witnessed notable increases in temperatures and the frequency of heat waves (Raji et al. 2021; Rohini et al. 2016), exacerbating the impact of concurrent hydrological extremes, leading to compound events with significant repercussions on agriculture (Aihaiti et al. 2021; Dash and Maity 2021; Kalyan et al. 2021). These compound extremes are projected to rise under all emission scenarios, underscoring the urgent need to assess their combined impact on crop yield (Das et al. 2022; Lesk et al. 2022).
Understanding the effects of climate extremes on crop yield is crucial for global food security, especially considering India's significant role as the world's second-largest producer and largest exporter of rice (IFPRS-2023). Temperature is a critical factor influencing rice yield, with changes in climate patterns posing significant challenges to crop production (Peng et al. 2004; Pushpalatha et al. 2022; Quang et al. 1995; Saud et al. 2022). Traditional approaches relying on seasonally averaged metrics may overlook the detrimental effects of extreme events occurring on specific days, highlighting the importance of within-season variability analysis (Denmead and Shaw 1960; Kaur et al. 2018; Schmidt et al. 2011). When solely considering individual climate extremes, the analysis overlooks the intricate interplay of various climatic factors impacting crop yield. Focusing solely on singular extremes may result in an incomplete understanding of the true magnitude of climate-induced risks to agricultural productivity. However, by examining coincidental and sequential compound extremes, a more detailed comprehension of climate effects on crop yield emerges. This approach unveils the synergistic relationships among different climatic variables, offering a more accurate assessment of agricultural vulnerabilities and facilitating the formulation of targeted adaptation strategies. Thus, considering compound extremes enhances our ability to safeguard crop yield and ensure food security amidst changing climatic conditions. Additionally, incorporating both rainfall and soil moisture as water metrics is essential for a comprehensive evaluation of their impact on crop yield, further enhancing our ability to develop targeted adaptation strategies and ensure food security in the face of changing climatic conditions (Holzman et al. 2014).
While various statistical and process-based models have been employed to study crop yield behavior, most have focused solely on individual hydrological metrics, leaving a gap in understanding the combined effects of compound extremes (Hamed et al. 2021; Haqiqi et al. 2021; Lal et al. 1999; Ortiz-Bobea et al. 2019; Roberts et al. 2013). This study aims to address this gap by integrating coincidental and sequential compound extremes into the statistical modelling of rice yield, utilizing advanced regression techniques to capture their combined impact. By incorporating data from multiple sources and considering both individual and sequential compound extremes, this research is poised to enhance our understanding of climate change's implications for rice production and inform the development of effective adaptation strategies. Ultimately, the findings from this study can provide valuable insights for policymakers, farmers, and stakeholders involved in agriculture and food security, aiding in the formulation of robust strategies to mitigate the adverse effects of climate change on crop yield and maintain food security in the face of evolving climatic conditions.
2 Materials and methods
2.1 Study area
The research work encompasses six rice-growing states of India, namely West Bengal, Uttar Pradesh, Punjab, Tamil Nadu, Bihar, and Karnataka, as shown in Fig. 1. These states are often referred to as the Rice Bowl of India, and West Bengal is the largest producer of rice in the country (Karamchedu 2023). Rice is cultivated in three different seasons in these states. However, the majority of rice production occurs during the kharif season, where rainfall serves as a primary source of water availability. In West Bengal, Uttar Pradesh, and Bihar, rice is predominantly grown under rainfed conditions. On the other hand, in Punjab, Tamil Nadu, and Karnataka, rice cultivation primarily takes place under irrigated conditions.
2.2 Data used
This study used rainfall, soil moisture, temperature and crop yield data (Table 1). Rainfall and temperature data were obtained from the India Meteorological Department (IMD), Pune, for the period between 2000 and 2018. Rainfall data was available at a resolution of 0.25° × 0.25°, while temperature data was available at a resolution of 1° × 1°. The soil moisture data was collected at a resolution of 0.25° × 0.25° from the Global Land Data Assimilation System (GLDAS). Additionally, the district-wise crop yield data was collected from the Directorate of Economics and Statistics, Govt. of India for 19 years starting from 2000 to 2018. This study period is selected due to the limited availability of consistent crop yield data during past years. To facilitate district-wise analysis, the data was spatially averaged for each district.
2.3 Methodology
This study aimed to analyze the impact of hydrological extremes on crop yield using statistical models. These models build upon the regression models previously developed by Haqiqi et al. (2021). The methodology of this study is illustrated in Fig. 2. Three different types of regression models depicting the response of rice yield to individual and compound extremes were formulated using datasets on temperature, rainfall, and soil moisture. The daily temperature data was used to develop the metric for heat. The metrics of soil moisture were constructed by measuring their deviations from the mean volumetric content. These metrics were then used to formulate regression models to study the impact of climate extremes on rice yield. Additionally, the models were estimated using the panel fixed effect approach, which is a statistical tool for analyzing two-dimensional panel data (in this case time and location). Finally, we conducted a Hausman test (Hausman 1978) to determine the most appropriate model, choosing between random and fixed effects models.
The models are formulated with yield as a function of individual and compound extremes. Individual extreme models are formulated with heat and water metrics without considering their interaction. Compound Extreme are said to occur when heat stress and water stress occur in combination, either on the same day or follow each other within a short period. The compound extremes are categorised into compound coincidental extremes (CCE) and compound sequential extremes (CSE). The compound coincidental extremes occur when two or more hydrological variables reach their respective extreme values on the same day. The compound sequential extremes occur when two or more hydrological variables reach their respective extreme values one after the other within a certain duration. For this study, sequential heat stress with a period of 3 days followed by water stress with a period of 5 consecutive days in the upcoming 7 days as a sequential heat and water compound extreme event is considered. This approach has also been previously followed in many literatures. (Das et al. 2022; Weber et al. 2020).
A preliminary analysis was conducted utilizing rice yield as the dependent variable and temperature as independent variable, as depicted in Fig. 3. Various temperature values were explored to assess the influence of temperature on rice. Ultimately, a threshold temperature of 35 °C was pinpointed, indicating that temperatures exceeding this level will have a detrimental effect on rice growth. The root zone of rice extends up to 100 cm depth from the top of the soil layer, thus the soil moisture stress in this zone can also have a direct impact on crop yield (Sure and Dikshit 2019). Therefore, the soil moisture of the top 100 cm soil layer is considered in this analysis. The threshold for soil moisture range is taken the same as taken by Haqiqi et al. (2021) as the soil moisture values can be controlled in these limits. The metrics for soil moisture for compound extremes are constructed by taking the deviations from the normal level. The normal level is defined by taking the mean soil moisture content for the growing season of the entire study period for the given location. Five different ranges for soil moisture are considered for the compound coincidental and sequential extreme models. The study categorised soil moisture conditions into five classes, which included normal soil moisture condition (soil moisture is within 25 mm above or below the normal levels), surplus soil moisture condition (soil moisture is in between 25 to75 mm above normal levels), extreme surplus soil moisture condition (Soil moisture is 75 mm above normal level), deficit soil moisture condition (Soil moisture is in the range of 25 mm-75 mm below normal level) and extreme deficit soil moisture condition (Soil moisture is 75 mm below normal levels).
2.3.1 Individual Extreme Model (IEM)
The IEM model (Schlenker and Roberts 2009) assumes that the effects of heat and water are cumulative over the growing season and their impacts do not interact with each other. Table 2 presents the major variables considered for studying the impact of individual extremes.
The heat stress for each IEM model is taken by considering the number of instances when the daily maximum temperature (Tmax) exceeds 35 °C in a growing season. For IEM(a), cumulative rainfall (P) for the entire growing season is taken as water metric. For IEM(b), seasonal mean soil moisture content (M) is used as water metric. Again, for IEM(c) the number of days where soil moisture is above normal, i.e., in the surplus range (NDS) and below normal levels, i.e., in the deficit range (NDD) is considered. For IEM(d), cumulative soil moisture above normal (CSMN) and below normal levels (CSMD) is considered. Equation 1–4, represents the models for IEM(a-d), respectively.
where, \({Y}_{it}\) is the crop yield in district i at time \(t\).\(\alpha\), \(\lambda\) are regression parameters, t is the time trend variable, ci is the time-invariant district fixed effect, ∈ is the error term.
2.3.2 Compound Coincidental Extreme Model (CCM)
The CCM is formed following the approach as developed by Haqiqi et al. 2021. The CCM is formulated to focus on the compound metrics with the daily interaction of available water and heat stress. CCM evaluates the conditional marginal impact of compound metrics on rice yield. Table 3 summarizes the major variables for studying the effect of coincidental compound extremes (CCM) on rice yield. CCM(a) studies the marginal impact of heat excess combined with rainfall deficit. The model is defined with metrics of heat excess, i.e., when the maximum daily temperature exceeds 35 °C combined with dry days (daily rainfall is less than 1 mm). Equation (5) represents the model for CCM(a). CCM(b) forces the interaction of heat excess to different combinations of soil moisture defined at 25 mm intervals as deviations from normal. Equation (6) represents the model for CCM(b). It includes heat stress above 35 °C combined with soil moisture in the different zones: normal, surplus, extreme surplus, deficit and extreme deficit.
2.3.3 Compound Sequential Extreme Model (CSM)
The compound sequential extreme model (CSM) studies the impact of sequential extremes on rice yield. Table 4 provides the major variables used in the study of sequential compound extremes. The extreme heat condition has been taken when the temperature is above the threshold temperature (35°C) for a span of three consecutive days followed by respective water metric conditions.
The CSM models are formulated to understand rice yield’s response to the sequential occurrence of heat and water stress. It will also help to understand how the damage due to one variable is affected by the stress of another variable. Equations 7 and 8 represent CSM(a) and CSM(b), respectively.
2.4 Yield response in alternate conditions
The growing season of rice has been defined in three different stages. The first one is the vegetative growth that extends for the first seven weeks. It is followed by the reproductive stage for the next five weeks. The third stage called the ripening stage extends for the last five weeks. The CCM and CSM models are estimated for these three stages to check whether rice yield behaves differently due to the occurrence of compound extremes in the different stages. The study evaluated models for two distinct regions: the rainfed region, where rice cultivation relies on rainfall, and the irrigated regions, where rice is grown under irrigated conditions.
2.5 Model evaluation
To evaluate the models, four performance-based indices namely R2 (Coefficient of determination), adjusted R2, Akaike Information Criterion (AIC) and Bayesian Information Criterion (BIC) have been used. Equations (9 - 12) represents the formulation of these indices.
R2 (Coefficient of determination):
where, \({y}_{i}\) is the observed data, \({f}_{i}\) is the fitted data, \(\widehat{y}\) is the mean of observed data.
Adjusted R square: -
where, \({df}_{e}\) is the degrees of freedom (m – p – 1) of the estimates of the underlying population error variance, and \({df}_{t}\) is the degrees of freedom (m – 1) of the estimates of the population variance of the dependent variable. Here, m is the total sample size and p is the number of predictors.
Akaike information criterion (AIC): -
Bayesian information criterion (BIC): -
where, \(N\) is the number of rows in the dataset, \(LL\) is the log-likelihood of the model, and \(k\) is the number of parameters in the model.
3 Results
The study focused on assessing the impact of individual and compound extremes on rice yield for rainfed and irrigated regions of India using temperature, rainfall, and soil moisture data.
From Fig. 3, it can be seen that the when the temperature occurrence above 35 °C has negative impact on rice yield. Figures 4 and 5 shows the variation in temperature, rainfall and soil moisture data. Figure 4 shows the comparison of the change in year-wise rainfall and soil moisture in the rainfed and irrigated regions. The variation in average rainfall is higher than the variation in average soil moisture. The variation in soil moisture is higher in the rainfed region than in the irrigated region.
In the rainfed region rainfall is moderately correlated to soil moisture. Here the coefficient of correlation is found to be 0.54. In contrast, the coefficient of correlation is found to be 0.15 in the irrigated region. The significance of relating soil moisture with rainfall in the present study lies in understanding how soil moisture levels interact with rainfall patterns to influence crop yield in rainfed and irrigated conditions. It shows that soil moisture in these regions is mostly driven by irrigation. It is important to note that factors like irrigation, floods, waterlogging contributes to the soil moisture, thus in certain years soil moisture can have opposite trend as that of rainfall. The rainfall and soil moisture observe the same variations in the rainfed region. However, in some years like 2006, 2007 and 2008, a contradiction is observed as in these years the trend of soil moisture is found to be opposite of the trend of rainfall. Factors like irrigation, floods, waterlogging contributes to the soil moisture, thus in certain years soil moisture can have opposite trend as that of rainfall. The rainfed region experience on an average 861 mm of rainfall in the growing season whereas the irrigated region experiences an average of only 437 mm of rainfall. The average soil moisture (top 100 cm soil layer) in the rainfed region during this period is 227 mm and in the irrigated region is 258 mm. The rainfed region have high rainfall however, the soil moisture in the irrigated regions is fairly high due to the contribution of irrigation to the soil moisture.
Figure 5 illustrates the bivariate dynamics of daily soil moisture and heat in rainfed regions during the growing season from 2000 to 2018. In June, the distribution indicates lower soil moisture, skewed towards the lower right, suggesting a higher likelihood of compound extremes with high temperature and low soil moisture. It's noticeable that June and July are more susceptible to these compound extremes. Conversely, in August and September, the distributions shift towards the upper left, indicating abundant moisture conditions with fewer hot days. These months exhibit relatively higher soil moisture levels as they are less warm compared to June and July. For the estimation approach of models, the Hausman test revealed that the fixed effect model would perform better for analysing the data in this study. Hence fixed effect model was used for the estimation of models. The regression results of various models for rainfed and irrigated regions are discussed below.
3.1 Individual Extremes Model (IEM)
The regression results for IEM has been presented in Table S1. For rainfed regions, the results show that rice yield is largely dependent on cumulative rainfall throughout the growing season. Rice grows under waterlogged conditions and requires nearly 10 cm of waterlogging during the growing season. The model results indicate that the coefficients for heat stress, i.e., days on which the maximum temperature exceeds 35 °C, are negative and significant for all models. The coefficient for cumulative rainfall was found to be positive, suggesting that rice yield increases with an increase in cumulative rainfall during the growing season. Rice requires high soil moisture conditions throughout the growing season, unlike other crops. Thus, abundant rainfall is always beneficial for rice growth. Similarly, the mean soil moisture coefficient had a positive and significant correlation, and the coefficients for NDS and CSMN conditions were also significant. This result confirms that rice yield requires higher soil moisture throughout its growing season. However, the results for NDD and CSMD were not significant. The significance of the results was checked using the P-Value test. In irrigated region, the effect of heat excess on rice yield was negative but not statistically significant. Soil moisture parameters above normal had a positive impact on rice yield, indicating the benefit of surplus soil moisture. Conversely, the coefficient for soil moisture in the deficit range was negative and insignificant.
3.2 Compound Coincidental Extremes Model (CCM)
Table S2 presents the regression results from CCM (a & b) models for both rainfed and irrigated regions. In the rainfed region, the regression results of CCM(a) indicate that hot and dry days negatively affect rice yield, with coefficients similar to those of events with only heat stress. The regression results of CCM(b) show that the compound heat and soil moisture metrics also impact rice yield. Negative and significant coefficients were found for the compound metric with heat stress and soil moisture conditions around normal or below. However, the coefficients were positive but insignificant for soil moisture in the surplus and extreme surplus zone on hot days, suggesting that surplus water can offset the damage caused by heat. The coefficient of soil moisture in the extreme surplus range was about ten times more efficient in counteracting the damage caused by excess heat than when it was in the surplus range, as evidenced by its coefficient of 0.0165, compared to 0.0015 for the surplus range.
No hot days with extreme soil moisture deficit were observed in irrigated region. Hot days with soil moisture within normal conditions had negative but insignificant coefficients. The coefficient for soil moisture in the surplus range was positive, but in the extreme surplus range, it was negative.
3.3 Compound Sequential Extremes Model (CSM)
Table S3 shows the results of CSM models for both rainfed and irrigated regions. The results indicate that the impact of sequential extremes with soil moisture was less significant than coincidental extremes when the temperature exceeded the heat stress threshold. There was no significant evidence of the direct impact of sequentially compounding extremes on rice yield. Additionally, the damage caused by heat was not counteracted by excess soil moisture on the following days. The regression coefficient for sequentially compounding heat stress and rainfall scarcity was negative and significant (−0.01787400) with a confidence level of 95 percent, indicating that sequential hot and dry days are more damaging to rice yield than simple hot days. In irrigated regions, sequential hot events followed by consecutive extreme surplus or extreme deficit soil moisture days were not observed. Additionally, the coefficient of hot days with different soil moisture conditions in irrigated regions was insignificant.
3.4 CCM in different growing stages
Table S4 shows the regression results of compound coincidental model parameters in different stages of the growing season for rainfed region. Compound hot and dry days have a negative coefficient in all three stages, indicating that rainfall is the dominant factor in determining yield in all three stages. However, this dominance is more pronounced in the third stage. No instances of compound hot days with extreme soil moisture deficit were observed in the second and third stages of rice growth. However, the coefficient of this instance in the first stage was negative and significant. The coefficient of soil moisture deficit in the third stage was also negative and significant, highlighting the damage caused by water scarcity in the final stages of rice cultivation.
3.5 CSM in different growing stages
Table S5 shows the results of CSM models in different stages for the rainfed regions. The influence of sequential extremes in different stages was found to be the same as evident from sequential extreme parameters for the entire season. The coefficients of sequential extremes with heat stress followed by soil moisture in the surplus range in the second and third stages were negative and significant, indicating that the damage due to heat was not counteracted by the following excess water.
Figure 6 illustrates the separate effects of heat and water metrics on rice yields in rainfed areas. The results indicate that rice yield was negatively impacted by an increase in heat stress and a decrease in soil moisture. In most years, an increase in heat stress and a decrease in soil moisture had a negative impact on rice yield. Conversely, in years where soil moisture increased and heat stress decreased, there was a positive effect on rice yield. Notably, in 2012, it was observed that the damage caused by heat was offset by increased soil moisture, resulting in an increase in crop yield.
Figure 7 shows the decomposed effect of heat and water metrics on rice yields in irrigated regions. In these areas, the trend in crop yield was mainly driven by the trend in heat stress, like in the year 2003 when even though the soil moisture decreased crop yield still increased due to the decrease in heat stress.
3.6 Model comparison
The performance indices of models formulated in this study have been shown in Table 5. The performance of models in the rainfed regions was found significantly better than in the irrigated regions. The models are better capable of capturing the variance in rainfed regions. There is not much difference in the predictive capacity of models in the same region.
Among the individual extremes model in the rainfed regions, IEM(d) which uses cumulative soil excess above and below normal levels has the best performance, whereas in the irrigated regions IEM(b) which uses mean soil moisture of the growing season has better performance. The performance of models increases when parameters for different seasons were taken separately. From all the models in rainfed regions, CCM(b) has the best Adjusted R2 and is found to be the best performing. In the Irrigated regions CSM(b) was found to be the best performing model.
4 Discussion
The work aims to study the impact of individual and compound extremes on rice yield in two different regions of India. Previously, researchers have used statistical models to study the impact of individual hydrological extremes on crop yield (Ortiz-Bobea et al. 2019; Roberts et al. 2013) and the impact of compound extremes on crop yield (Haqiqi et al. 2021), but most of these works have been carried out for countries like USA. In India, no such study has been carried out yet. Statistical models have also been used to predict the changes in crop yield due to projected climate change (Lobell and Burke 2010). From the climate change studies, it was evident that the hydrological extremes are expected to multiply both in frequency and intensity in the upcoming future (Bevacqua et al. 2019; Manning et al. 2019; Myhre et al. 2019; Poschlod et al. 2020). Thus, extensive studies are required to study the behaviour of crop yield to the occurrence of these extremes.
The formulation of the yield response function of crops through statistical models provides sufficient evidence of the crop yield response to extremes. The response of crop yield to extreme events such as drought, heat stress, and excess rainfall varies with the type of crop. Therefore, it is important to study the impact of these extremes on different crops to develop appropriate management practices for ensuring food security. Rice is a staple crop in many parts of the world and is particularly important in India, where it is widely grown and consumed. Understanding the response of rice yield to different water and heat metrics is crucial for developing strategies to mitigate the impact of extremes on rice production.
The analysis of the regression coefficients of different water metrics and extreme events on rice yield provides valuable insights into the impact of these factors on crop productivity. The negative and significant coefficient of days with maximum temperature exceeding 35 °C in the rainfed regions indicates that extreme heat has an adverse effect on rice yield. Moreover, past studies suggest that the average rice yield would have been higher if the pre-1960 climatic conditions prevailed during 1960–2007 in India (Auffhammer et al. 2012; Pattanayak and Kumar 2014), highlighting the potential impact of climate change on crop yield. On the other hand, the positive and significant coefficient of IEM (C&D), NDS & CSMN indicates that soil moisture plays a crucial role in determining rice yield, with higher moisture levels leading to higher yields.
In the rainfed region, the coefficient of only hot days obtained from IEM’s are same as that of hot and dry days as obtained from CCM(a). It may be due to the fact that most extremely hot days are mostly accompanied by scanty rainfall (rainfall < 1 mm). The regression results of CCM(b) shows that hot days accompanied by soil moisture in deficit and extreme deficit range adversely affects the rice yield, but lacks sufficient evidence of this conclusion. The coefficients of hot and dry days, as well as surplus and extreme surplus soil moisture, show a positive effect on rice yield. The coefficients of CSM(a) show that sequentially occurring hot and dry days are more dangerous to rice yield than simply dry days as dry days result in lower moisture availability to the crop. However, no evidence of sequentially occurring heat and soil moisture extremes impacting rice yield was found from the CSM(b) models. The coefficient of parameters of CCM(b) in different stages shows that extreme surplus soil moisture on hot days is harmful in the first and second stage but beneficial in the last stage. Thus, highlighting the need for within season analysis of crop yield. These results suggest that extreme heat can be compensated by maintaining soil moisture within the desired range, highlighting the importance of managing soil moisture levels for optimizing crop yield. However, the lack of significant evidence for the impact of sequentially occurring heat and soil moisture extremes on rice yield suggests that further research is needed in this area.
In irrigated regions, the coefficients of hot days are found to be insignificant, likely due to consistent irrigation offsetting the damage caused by hot days. However, the study found that in irrigated regions, extreme surplus soil moisture on hot days has a negative coefficient, which may be due to the already high levels of normal soil moisture in these areas due to more prominent irrigation.
Overall, the study suggests that rainfall and soil moisture are critical factors for rice yield in rainfed regions, while in irrigated regions, the impact of extreme weather events may be offset by consistent irrigation. The results also highlight the importance of considering different combinations of extreme weather events and within-season analysis of crop yield in understanding the impact of climate change on crop production.
To evaluate the performance of models, this study used adjusted R2. Adjusted R2 is a statistical measure that adjusts the R2 value for the number of independent variables included in the model. It takes into account the fact that including more variables in the model can lead to an increase in R2 even if the additional variables are not significant predictors of the dependent variable. Therefore, adjusted R2 is a better measure of model performance than R2 alone, especially in cases where there are many independent variables. AIC (Akaike Information Criterion) and BIC (Bayesian Information Criterion) are also commonly used measures of model performance. They take into account the number of parameters in the model and penalize models with too many parameters. However, they are more suitable for comparing models with the same number of parameters, which is not the case in the present study. In the present study, CCM(b) while modelled for different stages of the growing season is found to be the best performing model for both the rainfed and irrigated regions. However, the predictive capability of the models in the irrigated region is considerably lower compared to the rainfed region. The lower predictive capability of models in the irrigated region compared to the rainfed region may be due to the consistent irrigation in these regions, which may offset the impact of climate variability on rice yield.
The statistical models do have certain limitations like being unable to capture artificial processes such as irrigation, use of fertilizers, and other farm management practices. These limitations reduce the predictive capability of statistical models. The use of a single metric can lead to over or underestimation of the climate change impacts. The results in this study can be further improved by improving the sample size taken in the study which can be done by extending the period of study, if consistent crop yield data is available for the study period. It can also be done by extending the regions under analysis if the crop growth is under similar conditions in the extended region.
The past studies and the future model projections have shown that the shift in rainfall with more intense and frequent spells in some parts of the season will lead complete dryness in other parts. This in turn will result in abrupt transition in soil moisture (Guhathakurta et al. 2015; Menon et al. 2013; Sahana et al. 2015; Sarkar et al. 2015). The increase in temperature will lead to a greater number of hot days. These scenarios will have damaging impact on crop yield as identified by the study. The southern and western parts of India which currently have relatively lower temperatures compared to northern and eastern regions, are likely to show greater sensitivity in rice yields under climate change (Aggarwal and Mall 2002). Hence constant irrigation would be required to avoid the damage due to soil moisture in deficit zone in both the rainfed and irrigated regions.
5 Conclusions
This study analysed the impact of heat and water stress on crop yield using rainfall, temperature and soil moisture data. The available data is used to extract heat and water metrics for the formulation of statistical models. In this study district-wise analysis is done with rice yield being a dependent variable over climatic conditions like rainfall, temperature and soil moisture. The results obtained from the regression analysis indicate the damage to rice yield due to unfavourable climatic conditions. One of the major causes of concern is excess heat that adversely affects rice yield. In this study, it has been found that hot days with soil moisture scarcity have negative impact on the rice yield where the coefficient of hot days is between −0.0020 to −0.0032 but hot days with soil moisture excess have controlled these damages as suggested by positive coefficient of 0.0165. The primary finding of this study is that by controlling soil moisture within the desired limits it is possible to reduce the damage due to excess heat as hot days with soil moisture in extreme surplus range have coefficient of 0.0165 and are able to effectively counter the heat days by more than 10 times than hot days with soil moisture in surplus range which have coefficient of 0.0015. Soil moisture is the only controllable water metric that can be altered through the application of additional water by irrigation, thus further studies on optimal soil moisture can be carried out to efficiently support the rice yield. Further, the study also found that coincidental compound extremes are found to be more damaging to the rice yield than sequential compound extremes. The behaviour of these models changes with the study area due to the different in geographical conditions and the distribution of hydrological variables.
This study demonstrates the effectiveness of statistical models in assessing the impact of coincidental and sequential compound extremes on crop yield within consistent hydrological conditions. The framework offers potential for analyzing future crop yield trends with dependable projections of rainfall, temperature, and soil moisture. Moreover, the methodology outlined here is transferable and can be applied to investigate the yield-response patterns of different crops. Future research endeavours could focus on enhancing the predictive capacity of these models by integrating data-driven approaches such as machine learning and deep learning techniques, along with incorporating additional farm management practices supported by comprehensive data sets. Such advancements hold promise for refining agricultural resilience and adaptation strategies in the face of changing environmental conditions.
Data availability
The data will be made available on request.
Abbreviations
- CCE :
-
Compound coincidental extremes
- CCM :
-
Compound coincidental extremes model
- CSE :
-
Compound sequential extremes
- CSM :
-
Compound sequential extremes model
- CSMD :
-
Cumulative soil moisture below normal levels
- CSMN :
-
Cumulative soil moisture above normal levels
- DS :
-
Different stages
- IEM :
-
Individual extremes model
- M :
-
Seasonal mean soil moisture content
- M 2 :
-
Square of season mean soil moisture content
- NDD :
-
Number of days when soil moisture is below normal levels
- NDS :
-
Number of days when soil moisture is above normal levels
- R :
-
Cumulative growing season rainfall
- R 2 :
-
Square of cumulative growing season rainfall
- T max :
-
Daily maximum temperature
References
Aggarwal PK, Mall RK (2002) Climate change and rice yields in diverse agro-environments of India. II. effect of uncertainties in scenarios and crop models on impact assessment. Clim Chang 52:331–343. https://doi.org/10.1023/A:1013714506779
Aihaiti A, Jiang Z, Zhu L, Li W, You Q (2021) Risk changes of compound temperature and precipitation extremes in China under 1.5 °C and 2 °C global warming. Atmos Res 264:105838. https://doi.org/10.1016/j.atmosres.2021.105838
Auffhammer M, Ramanathan V, Vincent JR (2012) Climate change, the monsoon, and rice yield in India. Clim Chang 111:411–424. https://doi.org/10.1007/s10584-011-0208-4
Bevacqua E, Maraun D, Vousdoukas MI, Voukouvalas E, Vrac M, Mentaschi L, Widmann M (2019) Higher probability of compound flooding from precipitation and storm surge in Europe under anthropogenic climate change. Sci Adv 5:eaaw5531. https://doi.org/10.1126/sciadv.aaw5531
Das J, Manikanta V, Umamahesh NV (2022) Population exposure to compound extreme events in India under different emission and population scenarios. Sci Total Environ 806:150424. https://doi.org/10.1016/j.scitotenv.2021.150424
Dash S, Maity R (2021) Revealing alarming changes in spatial coverage of joint hot and wet extremes across India. Sci Rep 11:18031. https://doi.org/10.1038/s41598-021-97601-z
Dash SK, Jenamani RK, Kalsi SR, Panda SK (2007) Some evidence of climate change in twentieth-century India. Clim Change 85:299–321. https://doi.org/10.1007/s10584-007-9305-9
Denmead OT, Shaw RH (1960) The effects of soil moisture stress at different stages of growth on the development and yield of corn 1. Agron J 52:272–274. https://doi.org/10.2134/agronj1960.00021962005200050010x
Gershunov A, Rajagopalan B, Overpeck J, Guirguis K, Cayan D, Hughes M, Dettinger M, Castro C, Schwartz RE, Anderson M, Ray AJ, Barsugli J, Cavazos T, Alexander M, Dominguez F (2013) Future climate: projected extremes. In: Garfin G, Jardine A, Merideth R, Black M, LeRoy S (eds) Assessment of climate change in the Southwest United States. Island Press/Center for Resource Economics, Washington, pp 126–147. https://doi.org/10.5822/978-1-61091-484-0_7
Guhathakurta P, Rajeevan M, Sikka DR, Tyagi A (2015) Observed changes in southwest monsoon rainfall over India during 1901-2011. Int J Climatol 35:1881–1898. https://doi.org/10.1002/joc.4095
Hamed R, Van Loon AF, Aerts J, Coumou D (2021) Impacts of compound hot–dry extremes on US soybean yields. Earth Syst Dyn 12:1371–1391. https://doi.org/10.5194/esd-12-1371-2021
Haqiqi I, Grogan DS, Hertel TW, Schlenker W (2021) Quantifying the impacts of compound extremes on agriculture. Hydrol Earth Syst Sci 25:551–564. https://doi.org/10.5194/hess-25-551-2021
Hasan N, Pushpalatha R, Manivasagam VS, Arlikatti S, Cibin R (2023) Global sustainable water management: a systematic qualitative review. Water Resour Manag 37:5255–5272. https://doi.org/10.1007/s11269-023-03604-y
Hausman JA (1978) Specification Tests in Econometrics. Econometrica 46:1251–1271. https://doi.org/10.2307/1913827
Holzman ME, Rivas R, Piccolo MC (2014) Estimating soil moisture and the relationship with crop yield using surface temperature and vegetation index. Int J Appl Earth Obs Geoinf 28:181–192. https://doi.org/10.1016/j.jag.2013.12.006
Kalyan A, Ghose DK, Thalagapu R, Guntu RK, Agarwal A, Kurths J, Rathinasamy M (2021) Multiscale spatiotemporal analysis of extreme events in the Gomati River Basin, India. Atmosphere 12:480. https://doi.org/10.3390/atmos12040480
Karamchedu A (2023) Creating the ‘Rice Bowl of India’: examining the political economy of groundwater-led agrarian transformation in dryland India. Environ Plan E Nat Space 25148486231217141. https://doi.org/10.1177/25148486231217141
Kaur G, Nelson K, Motavalli P (2018) Early-season soil waterlogging and N fertilizer sources impacts on corn N uptake and apparent N recovery efficiency. Agronomy 8:102. https://doi.org/10.3390/agronomy8070102
Krishnan R, Sanjay J, Gnanaseelan C, Mujumdar M, Kulkarni A, Chakraborty S (eds) (2020) Assessment of climate change over the Indian region: a report of the Ministry of Earth Sciences (MoES), government of India. Springer Singapore, Singapore. https://doi.org/10.1007/978-981-15-4327-2
Lal M, Singh KK, Srinivasan G, Rathore LS, Naidu D, Tripathi CN (1999) Growth and yield responses of soybean in Madhya Pradesh, India to climate variability and change. Agric For Meteorol 93:53–70. https://doi.org/10.1016/S0168-1923(98)00105-1
Lesk C, Anderson W, Rigden A, Coast O, Jägermeyr J, McDermid S, Davis KF, Konar M (2022) Compound heat and moisture extreme impacts on global crop yields under climate change. Nat Rev Earth Environ 3:872–889. https://doi.org/10.1038/s43017-022-00368-8
Lobell DB, Burke MB (2010) On the use of statistical models to predict crop yield responses to climate change. Agric For Meteorol 150:1443–1452. https://doi.org/10.1016/j.agrformet.2010.07.008
Manning C, Widmann M, Bevacqua E, Van Loon AF, Maraun D, Vrac M (2019) Increased probability of compound long-duration dry and hot events in Europe during summer (1950-2013). Environ Res Lett 14:094006. https://doi.org/10.1088/1748-9326/ab23bf
Meehl GA, Zwiers F, Evans J, Knutson T, Mearns L, Whetton P (2000) Trends in extreme weather and climate events: issues related to modeling extremes in projections of future climate change. Bull Am Meteorol Soc 81:427–436. https://doi.org/10.1175/1520-0477(2000)081%3c0427:TIEWAC%3e2.3.CO;2
Menon A, Levermann A, Schewe J (2013) Enhanced future variability during India's rainy season. Geophys Res Lett 40:3242–3247. https://doi.org/10.1002/grl.50583
Myhre G, Alterskjær K, Stjern CW, Hodnebrog Q, Marelle L, Samset BH, Sillmann J, Schaller N, Fischer E, Schulz M, Stohl A (2019) Frequency of extreme precipitation increases extensively with event rareness under global warming. Sci Rep 9:16063. https://doi.org/10.1038/s41598-019-52277-4
Ortiz-Bobea A, Wang H, Carrillo CM, Ault TR (2019) Unpacking the climatic drivers of US agricultural yields. Environ Res Lett 14:064003. https://doi.org/10.1088/1748-9326/ab1e75
Pattanayak A, Kumar KSK (2014) Weather sensitivity of rice yield: evidence from India. Climate Change Economics 5:1–24. https://doi.org/10.1142/S2010007814500110
Peng S, Huang J, Sheehy JE, Laza RC, Visperas RM, Zhong X, Centeno GS, Khush GS, Cassman KG (2004) Rice yields decline with higher night temperature from global warming. Proc Natl Acad Sci 101:9971–9975. https://doi.org/10.1073/pnas.0403720101
Poschlod B, Zscheischler J, Sillmann J, Wood RR, Ludwig R (2020) Climate change effects on hydrometeorological compound events over southern Norway. Weather and Climate Extremes 28:100253. https://doi.org/10.1016/j.wace.2020.100253
Praveen B, Talukdar S, Shahfahad Mahato S, Mondal J, Sharma P, Islam ARMdT, Rahman A (2020) Analyzing trend and forecasting of rainfall changes in India using non-parametrical and machine learning approaches. Sci Rep 10:10342. https://doi.org/10.1038/s41598-020-67228-7
Pushpalatha R, Santhosh Mithra V, Sunitha S, Goerge J, Nedunchezhiyan M, Mamatha K, Ashok P, Alam S, Saud B, Tarafdar J, Mitra S, Deo C, Velmurugan M, Suja G, Ravi V, Gangadharan B (2022) Impact of climate change on the yield of tropical root and tuber crops vs. rice and potato in India. Food Secur 14:495–508. https://doi.org/10.1007/s12571-021-01226-z
Quang VD, van Hai T, Dufey JE (1995) Effect of temperature on rice growth in nutrient solution and in acid sulphate soils from Vietnam. Plant Soil 177:73–83. https://doi.org/10.1007/BF00010339
Raji P, Shiny R, Byju G (2021) Impact of climate change on the potential geographical suitability of cassava and sweet potato vs. rice and potato in India. Theor Appl Climatol 146:941–960. https://doi.org/10.1007/s00704-021-03763-1
Roberts MJ, Schlenker W, Eyer J (2013) Agronomic weather measures in econometric models of crop yield with implications for climate change. Am J Agric Econ 95:236–243. https://doi.org/10.1093/ajae/aas047
Rohini P, Rajeevan M, Srivastava AK (2016) On the variability and increasing trends of heat waves over India. Sci Rep 6:26153. https://doi.org/10.1038/srep26153
Sahana AS, Ghosh S, Ganguly A, Murtugudde R (2015) Shift in Indian summer monsoon onset during 1976/1977. Environ Res Lett 10:054006. https://doi.org/10.1088/1748-9326/10/5/054006
Sarkar R, Dutta S, Dubey AK (2015) An insight into the runoff generation processes in wet sub-tropics: field evidences from a vegetated hillslope plot. CATENA 128:31–43. https://doi.org/10.1016/j.catena.2015.01.006
Saud S, Wang D, Fahad S, Alharby HF, Bamagoos AA, Mjrashi A, Alabdallah NM, AlZahrani SS, AbdElgawad H, Adnan M, Sayyed RZ, Ali S, Hassan S (2022) Comprehensive impacts of climate change on rice production and adaptive strategies in China. Front Microbiol 13:926059. https://doi.org/10.3389/fmicb.2022.926059
Schlenker W, Roberts MJ (2009) Nonlinear temperature effects indicate severe damages to U.S. crop yields under climate change. Proc Natl Acad Sci 106:15594–15598. https://doi.org/10.1073/pnas.0906865106
Schmidt JP, Sripada RP, Beegle DB, Rotz CA, Hong N (2011) Within-field variability in optimum nitrogen rate for corn linked to soil moisture availability. Soil Sci Soc Am J 75:306–316. https://doi.org/10.2136/sssaj2010.0184
Sienz F, Bothe O, Fraedrich K (2012) Monitoring and quantifying future climate projections of dryness and wetness extremes: SPI bias. Hydrol Earth Syst Sci 16:2143–2157. https://doi.org/10.5194/hess-16-2143-2012
Sillmann J, Kharin VV, Zwiers FW, Zhang X, Bronaugh D (2013) Climate extremes indices in the CMIP5 multimodel ensemble: part 2. Future climate projections. J Geophys Res Atmos 118:2473–2493. https://doi.org/10.1002/jgrd.50188
Sure A, Dikshit O (2019) Estimation of root zone soil moisture using passive microwave remote sensing: a case study for rice and wheat crops for three states in the Indo-Gangetic basin. J Environ Manag 234:75–89. https://doi.org/10.1016/j.jenvman.2018.12.109
Weber T, Bowyer P, Rechid D, Pfeifer S, Raffaele F, Remedio AR, Teichmann C, Jacob D (2020) Analysis of compound climate extremes and exposed population in Africa under two different emission scenarios. Earths Future 8. https://doi.org/10.1029/2019EF001473
Yaduvanshi A, Nkemelang T, Bendapudi R, New M (2021) Temperature and rainfall extremes change under current and future global warming levels across Indian climate zones. Weather Clim Extrem 31:100291. https://doi.org/10.1016/j.wace.2020.100291
Acknowledgements
The authors would like to thank Swati Kumari for her help in data visualisation and Aditi Babanrao Palve and Swagat Patra for their support in data extraction.
Funding
This research did not receive any specific grant from funding agencies in the public, commercial, or not-for-profit sectors.
Author information
Authors and Affiliations
Contributions
Anshuman Mishra: Conceptualization, Methodology, Software, Data Curation, Formal analysis, Writing -Original Draft, Visualization.
Litan Kumar Ray: Conceptualisation, Methodology, Supervision, Writing-Reviewing & Editing, Visualization.
V. Manohar Reddy: Conceptualization, Software, Data Curation, Formal analysis, Visualization.
Corresponding author
Ethics declarations
Competing interests
The authors declare no competing interests.
Additional information
Publisher's Note
Springer Nature remains neutral with regard to jurisdictional claims in published maps and institutional affiliations.
Key points
1. Developed statistical models for analysing the effect of the individual as well as coincidental and sequential compound extremes on rice yield.
2. Sequential hot and dry days are more damaging to rice yields than simply hot days.
3. Temperature above 35 °C are found to be negatively correlating with the rice yield.
4. Rice yield favours abundant soil moisture above normal soil moisture in the growing season.
5. Damage due to extreme heat on rice yield can be controlled by keeping the soil moisture above the normal soil moisture conditions.
Supplementary Information
Below is the link to the electronic supplementary material.
Rights and permissions
Springer Nature or its licensor (e.g. a society or other partner) holds exclusive rights to this article under a publishing agreement with the author(s) or other rightsholder(s); author self-archiving of the accepted manuscript version of this article is solely governed by the terms of such publishing agreement and applicable law.
About this article
Cite this article
Mishra, A., Ray, L.K. & Reddy, V.M. Effects of compound hydro-meteorological extremes on rice yield in different cultivation practices in India. Theor Appl Climatol 155, 4507–4520 (2024). https://doi.org/10.1007/s00704-024-04894-x
Received:
Accepted:
Published:
Issue Date:
DOI: https://doi.org/10.1007/s00704-024-04894-x