Abstract
The motivation behind this paper is to explore the issue of passivity and passification for delayed neural networks with Markov jump parameters. A state feedback control approach with non-uniform sampling period is considered. The interest of this paper lies in the thought about another basic uniqueness wound up being less conservatism than watched Jensen’s inequality and takes totally the relationship between the terms in the Leibniz–Newton formula inside the arrangement of linear matrix inequalities. By utilizing the Lyapunov–Krasovskii functional strategy, a novel delay-dependent passivity criterion is developed with respect to linear matrix inequalities to guarantee the Markov jump delayed neural frameworks to be passive. Passivity and passification problems are tackled by using mode-dependent non-uniform sampled-data control. Using many examples from the literature, it is exhibited that the proposed stabilization theorem is less direct than past results. Finally, the framework is associated with benchmark issue, exhibiting to gather compelling stability criteria for reasonable issues, using the proposed strategy.
Similar content being viewed by others
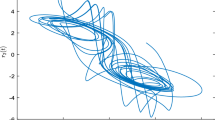
Explore related subjects
Discover the latest articles, news and stories from top researchers in related subjects.Avoid common mistakes on your manuscript.
1 Introduction
In the midst of the earlier decades, a speedy development has been made in the examination of neural networks which have wide applications in various locales, for instance, reproducing moving pictures, signal processing pattern recognition and optimization issues. For the late progress, since time delays cannot be kept up a key separation from and they frequently provoke insecurity of neural networks, it essentially focuses on progressing different sorts of stability conditions of delayed neural frameworks. Thus, the delayed neural frameworks have been widely studied by many authors and a assortment of results have been derived in [1–11]. Before the above examination, there is a crucial errand which is to choose the equilibrium and in addition to analysis stability conditions of the equilibrium point of different made neural frameworks. Recently, many efforts have been paid to study the stability of time delay systems [1–60].
However, a huge segment of the present clarifying transition probabilities in the Markov process has been thought to be absolutely accessible. The ideal assumption transition probabilities certainly limit the use of the standard Markovian jump process theory. Honestly, the likelihood to get the complete learning transition probabilities is defective and the cost is in all probability high. So it is tremendous and vital to further focus more wide Markovian jump process with incomplete transition descriptions [20–30]. Recently, the fault detection problem for a class of Markov jump linear system with partially known transition probabilities was investigated in [23–28]. The proposed systems are more general, which relax the traditional assumption in Markov jump systems that all the transition probabilities must be completely known.
Additionally, passivity and passification issues have been given watchful thought starting late. Passivity theory was at first proposed in the circuit analysis [31] and after that connected with various distinctive structures, including high-order nonlinear systems and electrical framework [32]. It can be extensively associated with play out the stability analysis, onlooker plan, signal processing, fuzzy control, sliding mode control and networked control etc. Over the past few years, increasing attention has been devoted to this topic and a number of important results have been reported, (see [33–40]). Recently, the passivity of straight structures with delays and the passivity of delayed neural networks have been focused by using fitting Lyapunov–Krasovskii functionals [36, 40].
Recently, various control approaches have been adopted to stabilize instable systems. Controls such as impulsive control, \(H_\infty\) control, finite-time control, intermittent control and sampled-data control are adopted by many authors [43–48]. The sampled-data control manages constant framework by data sampling at discrete time based on the pc, sensors, filters and network communication. Henceforth, it is more desirable over use of computerized controllers rather than simple circuits. This definitely lessens the measure of the transmitted data and enhances the control proficiency. In this manner, the sampled-data control innovation has demonstrated increasingly predominance over other control approaches ( [49–51]). The authors [52, 53] considered the non-uniform sampled-data control for stochastic passivity and passification of delayed Markov jump genetic regulatory networks with the help of Lyapunov technique and LMI framework. In spite of the way that the significance of sampled-data management and passivity property have been comprehensively seen, up to now, the sampled-data issues of passivity and passification for delayed neural networks with Markov jump parameters have not yet been represented and stay open.
Motivated by the ahead of time conveyed examination, this paper gets some information about the design of non-uniform sampled-data control for Markov jump delayed neural networks with passive theory. Isolated and the present results, another Markov jump delayed neural frameworks is set up to delineate the non-uniform by utilizing the input delay approach. Some new passivity conditions are displayed in the sorts of linear matrix inequalities (LMIs) by using Lyapunov–Krasovskii functional method. In connection of the grabbed conditions, a further mode-dependent passification controller game-plan rationale is given to guarantee the required execution of the ensuing closed-loop Markov process delayed neural networks.
The credentials of this paper are abbreviated here:
-
1.
Energized by the work [32, 34, 35], the new diagram of passivity and passification for delayed neural networks with Markov jump parameters is initially settled in our work.
-
2.
The fact of the matter is to exploit new methodologies to perform less conservatism than Jensen’s inequality and takes altogether the link between the terms within the Leibniz–Newton formula within the arrangement of linear matrix inequalities (LMIs).
-
3.
Finally, numerical cases are given to show the practicality and the less conservatism of the procured passivity criteria.
Notations: Throughout the paper, \({\mathscr {R}}^{n}\) denotes the n dimensional Euclidean space, and \({\mathscr {R}}^{m\times n}\) is the set of all \(m\times n\) real matrices. The notation * represents the entries implied by symmetry. For symmetric matrices \({\mathscr {X}}\) and \({\mathscr {Y}}\), the notation \({\mathscr {X}}\ge {\mathscr {Y}}\) means that \({\mathscr {X}}-{\mathscr {Y}}\) is positive semi-definite; \({\mathscr {M}}^T\)transpose of the matrix \({\mathscr {M}}\); I denotes the identity matrix with appropriate dimensions. \({\mathcal {E}}\) denotes the expectation operator with respect to some probability measure \(\mathcal {P}\). Let \((\mathcal {Z}, {\mathcal {F}},{\mathcal {P}})\) be a complete probability space which relates to an increasing family \(({\mathcal {F}}_t)_{t>0}\) of \(\sigma\) algebras \(({\mathcal {F}}_t)_{t>0} \subset {\mathcal {F}}\), where \({\mathcal {Z}}\) is the samples space, \({\mathcal {F}}\) is \(\sigma\) algebra of subsets of the sample space and \({\mathcal {P}}\) is the probability measure on \({\mathcal {F}}\). Let \(||f||_2=\,\sqrt{\int _0^\infty ||f||^2{\text {d}}t}, f(t) \in L_2[0,\infty )\), \(\Vert f\Vert\) refers to the Euclidean norm of the function f(t) at the time t. \(L_2[0,\infty )\) is the space of square integrable vectors on \([0,\infty )\). Let \(\{\varrho _t, t\ge 0\}\) be a right-continuous Markov chain on a probability space \(({\mathcal {Z}}, {\mathcal {F}},{\mathcal {P}})\) taking values in a finite state space \({\mathcal {N}}=\,\{1,2,\ldots ,m\}\) with generator \(\Pi =\,\{\pi _{pq}\}\) given by,
Here \(\Delta >0,\lim _{t\rightarrow +\infty }\frac{o(\Delta )}{\Delta }=\,0,\pi _{pq}\ge 0\) is the transition rate from p to q if \(q\ne p\) while \(\pi _{pp}=\,-\sum _{q=\,1,q\ne p}^m\pi _{pq}\) for each mode p. Note that if \(\pi _{pp}=\,0\) for some \(p \in {\mathcal {N}}\), then the pth mode is called “terminal mode” [54]. Furthermore, the transition probabilities matrix \(\Pi\) is denoted as follows:
Furthermore, the move rates or probabilities of the jumping process are thought to be known.
2 Problem statement and preliminaries
Consider the Markov jump delayed neural networks described in a complete probability \(({\mathcal {Z}}, {\mathcal {F}}, {\mathcal {P}})\) which can be depicted by the going with conditions
where \(\theta (t)=\,[\theta _1(t),\theta _2(t),\ldots ,\theta _n(t)]^T\in R^n\) is the neuron state vector. The nonlinear function \(g(\theta (t))=\,[g_1(\theta _1(t)),g_2(\theta _2(t)),\ldots ,g_n(\theta _n(t))]^T\in R^n\) denotes the neuron activation function, \(\tau (t)\) are the time-varying delays, \(\phi (t)\in R^n\) is a vector-valued initial condition function. The control inputs \(u(t)=\,[u_1(t),u_2(t),\ldots ,u_n(t)]^T\in R^n\) satisfy \(u_k(t)\ (k=\,1,2,\ldots ,n)\in L_2[0,\infty )\). \(w(t)=\,[w_1(t),w_2(t),\ldots ,w_n(t)]^T\in R^n\) denote the external disturbances satisfying \(w_k(t)\ (k=\,1,2,\ldots ,n)\in L_2[0,\infty )\). \({\mathscr {C}}(\varrho _t)=\,{\text {diag}}\{c_1,\ldots ,c_n\}>0,\ {\mathscr {W}}_1(\varrho _t),\ {\mathscr {W}}_2(\varrho _t),\ {\mathscr {H}}(\varrho _t)\) are interconnection weight matrices with appropriate dimensions for a altered mode \((\varrho _t)\) and all modes can be identified.
For presentation solace, we mean the Markov methodology \(\{\varrho _t, t\ge 0\}\) by i records, then the Markov jump delayed network system (2) can be changed into the accompanying structure:
Presently, we consider the mode-dependent sampled-data control can be laid out as
where \(u_{d}\) is discrete-time control signals. More precisely, the control signs are addressed with a gathering of sampling times: \({\mathcal {K}}_i\) is the gains of the feedback controller to be resolved later. The time-varying delay \(0\le h(t)=\,t-t_k\) is a piecewise linear function with derivative \({\dot{h}}(t)=\,1\) for \(t\ne t_{k}\), where \(t_k\) denotes the sample-time point and the available for interval \(t_k\le t<t_{k+1}\), \(k\in {\mathbb {N}}\).
Under the control law, the system (3) can be rewritten as follows:
Remark 2.1
The investigation of Markov jump delayed neural networks has gotten much consideration because of their hypothetical significance and potential applications. It merits saying that the vast majority of the investigates fundamentally concentrate on the stability problem delayed neural networks and have accomplished remarkable results [20–30]. In this paper, we analyse the non-uniform sampled-data control plan to tackle the passivity and passification issues of Markov jump delayed neural networks.
Remark 2.2
The input delay approach has been a successful strategy to manage the non-uniform sampling case, which is more confused yet more practical in the applications. By presenting the idea of virtual delay, the examined sampled-data control framework can be changed to the continuous-time system framework with time-varying delays, which is the key thought of this paper.
Before proceeding, the going with assumptions, definitions and lemmas are employed throughout our paper.
Assumption 1
The time-varying delays satisfy
where \(\tau\) and \(\mu\) are known constants.
Assumption 2
[55] The nonlinear function \(g_i(e) (i=\,1,2,\ldots ,n)\) fulfils the part condition
where \(g(0)=\,0\), and \(l_i\) are known real scalars and denote \({\mathcal {L}}=\,{\text {diag}}\{l_1,l_2,\ldots ,l_n\}\).
Definition 2.3
[52] The Markovian jump system (2) is said to be stochastically passive if there exists a positive scalar \(\gamma\) such that, \(\forall \ t_p\ge 0\)
holds, under the zero initial condition, where \(z^T(t)=\,x^T(t)\) and \(\varphi ^T(t)=\,w^T(t)\).
Definition 2.4
[52] Let \(V(\theta (t),\varrho _t,t>0)\) be stochastic positive function, its weak infinitesimal \({\mathscr {L}}\) is defined as
Lemma 2.5
[56] For any constant positive matrix \({\mathscr {T}}\in {\mathcal {R}}^{n\times n}\), scalar \(0\le \tau (t)\le \tau\), and vector function \({{\dot{\theta }}}(t):[-\tau ,0]\rightarrow {\mathcal {R}}^{n}\), it holds that
Lemma 2.6
[57] If there exist symmetric positive-definite matrix \({\mathcal {X}}_{33}>0\) and arbitrary matrices \({\mathcal {X}}_{11}\), \({\mathcal {X}}_{12}\), \({\mathcal {X}}_{13}\), \({\mathcal {X}}_{22}\) and \({\mathcal {X}}_{23}\) such that \([{\mathcal {X}}_{ij}]_{3\times 3} \ge 0\), then we obtain
where \(\varpi (t)=\,[\theta ^T(t)\quad \theta ^T(t-h(t))\quad {\dot{\theta }}^T(s)]^T.\)
3 Main results
In this section, the criteria on the passivity and passification of the system (5) are derived. The following theorem presents a sufficient condition on the stochastic passivity of the system (5).
Theorem 3.1
Under Assumption 2 hold, for given scalars \(\tau ,\ h, \ \gamma >0\), and \(\mu\), the system (5) is stochastically passive if there exist matrices \({\mathscr {P}}_i>0(i=\,1,2,\ldots ,m),\) \({\mathscr {Q}}>0,\ {\mathscr {R}}>0,\ {\mathcal {X}}>0,\ {\mathscr {T}}>0\), positive semi-definite matrices \([{\mathscr {Q}}_{ij}]_{3 \times 3}\ge 0\), diagonal matrix \(\varGamma >0\), and any matrices \({\mathscr {J}},\ {\mathscr {G}}_i\) with appropriate dimensions such that the following LMI hold :
where
Moreover, the mode-dependent gain matrices are given by \({\mathcal {K}}_i=\,{\mathscr {J}}^{-1}{\mathscr {G}}_i.\)
Proof
We consider the following LKF candidate as
where
For each mode i, it can be contemplated that
Applying Lemma 2.6 and the Leibniz–Newton formula for the integral term \(-\int _{t-\tau }^{t}{{\dot{\theta }}}^T(s){\mathscr {Q}}_{33}{{\dot{\theta }}}(s)ds\) the following equation holds:
According to Lemma 2.5, the integral terms in (14) can be rewritten as
Moreover, by Assumption 2, for appropriate dimensional diagonal matrix \(\varGamma\), we have
In addition,
Recollecting the last target to display the nonattendance of passivity property, the following expression is given:
By combining (12)–(20) it can be gotten that
where
Integrating both sides of (21) with respect to t over the time period from 0 to \(t_p\), we have
Therefore, the resulting Markov jump delayed neural networks (5) are stochastically passive by Definition 2.3, which completes the proof.\(\square\)
Remark 3.2
It should be noted that Theorem 3.1 provides a passive theory for Markovian jump delayed neural networks with time-varying delay. The results are expressed in the framework of LMIs, which can be easily verified by the existing powerful tools, such as the LMI toolbox of MATLAB. Moreover, if (10) are feasible, it follows from \(\Xi <0\) that \({\mathscr {G}}\) is nonsingular, and thus, the desired state feedback gain \({\mathcal {K}}\) can be readily obtained.
Remark 3.3
It is highly worth to mentioning here, less conservative criteria is obtained by using Lemma 2.5 in the integral term \(-\int _{t-\tau }^{t}{{\dot{\theta }}}^T(s)[{\mathscr {Q}}-{\mathscr {Q}}_{33}]{{\dot{\theta }}}(s){\text {d}}s\), which results,
Furthermore, if we consider the delayed neural networks in (2) without Markovian jumping parameters and without external disturbances, that is
Then, following a similar idea as in the proof of Theorem 3.1, we obtain the corollary 3.4.
Corollary 3.4
Under Assumption 2, for given scalars \(\tau\) and \(\mu\), the system (24) is asymptotically stable if there exist matrices \({\mathscr {P}}>0,\ {\mathscr {Q}}>0,\ {\mathcal {X}}>0,\ {\mathscr {T}}>0\), positive semi-definite matrices \([{\mathscr {Q}}_{ij}]_{3 \times 3}\ge 0\), diagonal matrix \(\varGamma >0\), and any matrix \({\mathscr {J}}\) with appropriate dimensions such that the following LMI hold:
where
Proof
We consider the LKF candidate as
where
The derivation is similar to that of in Theorem 3.1. so it is omitted here.\(\square\)
Remark 3.5
In Theorems 3.1 and Corollary 3.4, some LMI-based conditions are presented to guarantee asymptotically stability of the delayed neural networks. The established criterion is mode-dependent on the Markovian jump parameters. Set \(\tau =\,\frac{1}{\tau }\) and \(h=\,\frac{1}{h}\), in Theorem 3.1 and Corollary (3.4) with fixed value \(\mu\), the optimal value can be obtained through following optimization procedure:
and
Inequalities (27) and (28) are convex optimization problems and can be obtained efficiently by using the Matlab LMI toolbox.
Remark 3.6
It is very interesting to note that, in this paper, the reduced conservatism is primarily from the construction of the suitable Lyapunov–Krasovskii functional and the use of bounding techniques in integral terms. Secondly, a new integral technique is given to take fully the relationship between the terms in the Leibniz–Newton formula in the frame work of linear matrix inequalities (LMIs) into account, which gives the conservatism in our results.
Remark 3.7
Our approach is based on the Lyapunov–Krasovskii functional method combined with the slack variables and an improved technique of calculation. Comparing the numbers of variables required in Theorem 3.1, Corollary 3.4 of this paper and the results of the literature, we can see that our results lead to less conservative. The comparison results are summarized in Tables 1 and 2. From this tables we can conclude that our approach is less conservative.
Remark 3.8
The reduced conservatism of Theorem 3.1 and Corollary 3.4 benefit by introducing some free weighting matrices to express the relationship among the system matrices and neither the model transformation approach nor any bounding technique are needed to estimate the inner product of the involved crossing terms. It can be easily seen that results of this paper gives better results.
4 Numerical examples
In this section, numerical examples are provided to illustrate the potential benefits and effectiveness of the developed method for delayed neural networks.
Example 4.1
Consider the following delayed neural networks with (\(i=\,1,2\)):
The transition rate matrix with two operation modes is given as
Additionally, we take \({\mathcal {L}}=\,{\mathcal {I}},\ \gamma =\,1.4570,\ \tau =\,0.9\) and \(\mu =\,0.8\). Let sampling time points \(t_k=\,0.1 k,\ k=\,1,2,\ldots ,\) and the sampling period is \(h=\,0.1\). By using MATLAB LMI control toolbox and by solving the LMIs in Theorem 3.1 in our paper we get the following feasible solutions
The controller gains as
This implies all conditions in Theorem 3.1 are fulfilled. By Theorem 3.1, system (5) is asymptotically stable under the given sampled-data control. It is found that the proposed states of Theorem 3.1 are feasible with minimum passivity performance \(\gamma\) for different \(\tau ,\ \mu\) given in Table 1.
Given the time-varying delays within the corresponding ranges and system modes evolutions, by applying the obtained controller, the state responses of the resulting closed-loop Markov jump delayed neural networks (6) can be checked and observed as shown in Figs. 1 and 2 for the given initial condition \(\theta (t)=\,[-0.1,0.1]\). It is clear that the designed controller is feasible and ensures the passivity of the resulting closed-loop system (6) despite the disturbances and the time-varying delays.
State trajectories of the system in Example 4.1
Modes evolution i in Example 4.1
Example 4.2
Consider the delayed neural networks (24) with the following parameters:
It can be verified that the LMI (25) is feasible. For different values of \(\mu\), Table 2 gives out the allowable upper bound \(\tau\) of the time-varying delay. It is clear that the delay-dependent stability result proposed in Corollary 3.4 provides less conservative results than those obtained in [6–8, 10].
Example 4.3
Consider the delayed neural networks (24) with the following parameters:
To compare our results with the existing results in [2, 4, 5], we give different values of \(\mu\). The comparison results are stated in Table 3. From Table 3, it is observed that the results in this paper are less conservative than those in [2, 4, 5].
Example 4.4
Originally, neural networks embody the characteristics of real biological neurons that are connected or functionally related in a nervous system. On the other hand, neural networks can represent not only biological neurons but also other practical systems. One of them is the quadruple-tank process, which is presented in Fig. 3. The quadruple-tank process consists of four interconnected water tanks and two pumps. The inputs are the voltages \((\nu _1\) and \(\nu _2)\) to the two pumps and the outputs are the water levels of Tanks 1 and 2. As shown in Fig. 3, the quadruple-tank process can be expressed clearly using the neural network model. [58–60] proposed the state-space equation of the quadruple-tank process and designed the state feedback controller as follows:
where
For simplicity, it was assumed that \(\tau _1=\tau _2=0\), and \(\tau _3=\tau (t)\).
Here, the control input, u(t), means the amount of water supplied by pumps. Therefore, it is true that u(t) has a threshold value due to the limited area of the hose and the capacity of the pumps. Therefore, it is natural to consider, u(t), as a nonlinear function as follows:
\(f(x(t))=[f_1(x_1(t)),\ldots ,f_4(x_4(t))],\ f_i(x_i(t))=0.1(|x_i(t)+1|-|x_i(t)-1|),\ i=1,\ldots ,4.\)
The quadruple-tank process (29) can be rewritten to the form of system (2)with \(\varrho _t=1\), as follows:
where \({\mathscr {C}}=\,-A_0-A_1,\ {\mathscr {W}}_1=\,B^T_0K,\ {\mathscr {W}}_2=\,B^T_1K,\ f(\cdot )=\,f(\cdot )\) and \(w(t)=\,0\). In addition, \({\mathcal {L}}=\,0.1I,\ \tau =\,0.1\) and \(\mu =\,0.5\) can be obtained from the above system parameters. Figure 4 shows the state trajectories of the system is converges to zero equilibrium point with an initial state \([-0.3, 0.2, 0.5, -0.4]\); hence, it is found that the dynamical behaviour of the quadruple-tank process system (30) is asymptotically stable.
Originally, the water levels of the two lower tanks are the only accessible and usable information in the quadruple-tank process, whereas the water levels of the two upper tanks are not. Although the water in the upper tanks overflow, no action can be taken. Therefore, there is a strong need to know the water level of the upper tanks, which is one of the methods for obtaining the information to design the consider system. Until now, the research of the quadruple-tank process focused on designing the sampled-data controller. Therefore, in the sense of practical applications, it deserves that the quadruple-tank process should be applied to the proposed method.
When the sampling interval \(h=\,0.01\), the control gain matrix, which was calculated by Theorem 3.1 can be expressed as
Schematic representation of the quadruple-tank process. Source: From [59]
5 Conclusion
In this paper, we have made an effort for a novel setup of passivity and passification for delayed neural networks with Markov jump parameters via non-uniform sampled-data control. By building reasonable Lyapunov–Krasovskii functional and using Newton–Leibniz enumerating and delay-dependent passivity performance conditions are derived as LMIs. A mode-dependent controller layout method is displayed to guarantee that the resulting Markov jump delayed neural networks are passive. Numerical results are given to show the value of the proposed method.
References
Zhang Q, Wei X, Jin Xu (2005) Global asymptotic stability analysis of neural networks with time-varying delays. Neural Process Lett 21(1):61–71
Sun J, Liu GP, Chen J, Rees D (2009) Improved stability criteria for neural networks with time-varying delay. Phys Lett A 373:342–348
Hua M, Liu X, Deng F, Fei J (2010) New results on robust exponential stability of uncertain stochastic neural networks with mixed time-varying delays. Neural Process Lett 32(3):219–233
Tian J, Zhong S (2011) Improved delay-dependent stability criterion for neural networks with time-varying delay. Appl Math Comput 217(24):10278–10288
Chen W, Ma Q, Miao G, Zhang Y (2013) Stability analysis of stochastic neural networks with Markovian jump parameters using delay-partitioning approach. Neurocomputing 103:22–28
Ge C, Hu C, Guan X (2014) New delay-dependent stability criteria for neural networks with time-varying delay using delay-decomposition approach. IEEE Trans Neural Netw Learn Syst 25(7):1378–1383
Tian J, Xiong WJ, Xu F (2014) Improved delay-partitioning method to stability analysis for neural networks with discrete and distributed time-varying delays. Appl Math Comput 233:152–164
Zhou XB, Tian JK, Ma HJ, Zhong SM (2014) Improved delay-dependent stability criteria for recurrent neural networks with time-varying delays. Neurocomputing 129:401–408
Arik S (2014) New criteria for global robust stability of delayed neural networks with norm-bounded uncertainties. IEEE Trans Neural Netw Learn Syst 25(6):1045–1052
Zeng HB, He Y, Wu M, Xiao S-P (2015) Stability analysis of generalized neural networks with time-varying delays via a new integral inequality. Neurocomputing 161:148–154
Ahn CK, Shi P, Agarwal RK, Xu J (2016) \(L_\infty\) performance of single and interconnected neural networks with time-varying delay. Inf Sci 346:412–423
Karimi HR, Gao H (2010) New delay-dependent exponential \(H_{\infty }\) synchronization for uncertain neural networks with mixed time delays. IEEE Trans Syst Man Cybern B Cybern 40:173–185
Wang Y, Cao J, Li L (2010) Global robust power-rate stability of delayed genetic regulatory networks with noise perturbations. Cogn Neurodyn 4:81–90
Wang L, Luo Z-P, Yang H-L, Cao J, Li L (2016) Stability of genetic regulatory networks based on switched systems and mixed time-delays. Math Biosci 278:94–99
Cao J, Rakkiyappan R, Maheswari K, Chandrasekar A (2016) Exponential \(H_{\infty }\) filtering analysis for discrete-time switched neural networks with random delays using sojourn probabilities. Sci China Technol Sci 59:387–402
Ahn CK, Shi P, Basin MV (2015) Two-Dimensional dissipative control and filtering for Roesser model. IEEE Trans Automat Contr 60:1745–1759
Ahn CK, Wu L, Shi P (2016) Stochastic stability analysis for 2-D Roesser systems with multiplicative noise. Automatica 69:356–363
Ahn CK, Shi P, Basin MV (2016) Deadbeat dissipative FIR filtering. IEEE Trans Circuits Syst I 63:1210–1221
Ahn CK, Wu L, Shi P (2015) Receding horizon stabilization and disturbance attenuation for neural networks with time-varying delay. IEEE Trans Cybern 45:2680–2692
Syed Ali M (2015) Stability of Markovian jumping recurrent neural networks with discrete and distributed time-varying delays. Neurocomputing 149:1280–1285
Kao Y, Shi L, Xie J, Karimi HR (2015) Global exponential stability of delayed Markovian jump fuzzy cellular neural networks with generally incomplete transition probability. Neural Netw 63:18–30
Syed Ali M, Arik S, Saravanakmuar R (2015) Delay-dependent stability criteria of uncertain Markovian jump neural networks with discrete interval and distributed time-varying delays. Neurocomputing 158:167–173
Karimi HR (2013) Passivity-based output feedback control of Markovian jump systems with discrete and distributed time-varying delays. Int J Syst Sci 44:1290–1300
Karimi HR (2011) Robust delay-dependent \(H_{\infty }\) control of uncertain time-delay systems with mixed neutral, discrete, and distributed time-delays and Markovian switching parameters. IEEE Trans Circuits Syst I Regular Papers 58:1910–1932
Kao YG, Wang CH, Xie J, Karimi HR, Li W (2015) \(H_{\infty }\) sliding mode control for uncertain neutral-type stochastic systems with Markovian jumping parameters. Inf Sci 314:200–211
Li S, Xiang Z, Lin H, Karimi HR (2016) State estimation on positive Markovian jump systems with time-varying delay and uncertain transition probabilities. Inf Sci 369:251–266
Wei Y, Qiu J, Karimi HR, Wang M (2015) Model approximation for two-dimensional Markovian jump systems with state-delays and imperfect mode information. Multidim Syst Sign Process 26:575–579
Kao Y, Wang C, Xie J, Karimi HR (2015) New delay-dependent stability of Markovian jump neutral stochastic systems with general unknown transition rates. Int J Syst Sci 47(11):1–11
Syed Ali M, Gunasekaran N, Zhu Q (2016) State estimation of T-S fuzzy delayed neural networks with Markovian jumping parameters using sampled-data control. Fuzzy Sets Syst. doi:10.1016/j.fss.2016.03.012
Saravanakumar R, Syed Ali M, Ahn CK, Karimi HR, Shi P (2016) Stability of Markovian jump generalized neural networks with interval time-varying delays. IEEE Trans Neural Netw Learn Syst. doi:10.1109/TNNLS.2016.2552491
Belevitch V (1968) Classical network theory. Holden-Day, San Francisco
Luo M, Zhong S (2012) Passivity analysis and passification of uncertain Markovian jump systems with partially known transition rates and mode-dependent interval time-varying delays. Comput Math Appl 63(7):1266–1278
Balasubramaniam P, Nagamani G (2012) Global robust passivity analysis for stochastic fuzzy interval neural networks with time-varying delays. Expert Syst Appl 39(1):732–742
Guo Z, Wang J, Yan Z (2014) Passivity and passification of memristor-based recurrent neural networks with time-varying delays. IEEE Trans Neural Netw Learn Syst 25(11):2099–2109
Rakkiyappan R, Chandrasekar A, Cao J (2015) Passivity and passification of memristor-based recurrent neural networks with additive time-varying delays. IEEE Trans Neural Netw Learn Syst 26:2043–2057
Syed Ali M, Saravanakumar R, Cao J (2016) New passivity criteria for memristor-based neutral-type stochastic BAM neural networks with mixed time-varying delays. Neurocomputing 171:1533–1547
Li Y, Zhong S, Cheng J, Shi K, Ren J (2016) New passivity criteria for uncertain neural networks with time-varying delay. Neurocomputing 171:1003–1012
Li N, Cao J (2015) Passivity and robust synchronisation of switched interval coupled neural networks with time delay. Int J Syst Sci 47:1–10
Rakkiyappan R, Chandrasekar A, Cao J (2015) Passivity and passification of memristor-based recurrent neural networks with additive time-varying delays. IEEE Trans Neural Netw Learn Syst 26:2043–2057
Thuan MV, Trinh H, Hien LV (2016) New inequality-based approach to passivity analysis of neural networks with interval time-varying delay. Neurocomputing 194:301–307
Velmurugan G, Rakkiyappan R, Lakshmanan S (2015) Passivity analysis of memristor-based complex-valued neural networks with time-varying delays. Neural Process Lett 42(3):517–540
Nagamani G, Radhika T (2015) Dissipativity and passivity analysis of Markovian jump neural networks with two additive time-varying delays. Neural Process Lett. doi:10.1007/s11063-015-9482-x
Zhu QX, Rakkiyappan R, Chandrasekar A (2014) Stochastic stability of Markovian jump BAM neural networks with leakage delays and impulse control. Neurocomputing 136:136–151
Peng C, Zhang J (2015) Event-triggered output-feedback \(H_\infty\) control for networked control systems with time-varying sampling. IET Control Theory Appl 9:1384–1391
Cai M, Xiang Z (2015) Adaptive neural finite-time control for a class of switched nonlinear systems. Neurocomputing 155:177–185
Hu A, Cao J, Hu M (2014) Consensus of leader-following multi-agent systems in time-varying networks via intermittent control. Int J Control Autom Syst 12(5):969–976
Cao J, Sivasamy R, Rakkiyappan R (2016) Sampled-data \(H_\infty\) synchronization of chaotic Lur’e systems with time delay. Circuits Syst Signal Process 35:811–835
Hui G, Zhanga H, Wu Z, Wang Y (2014) Control synthesis problem for networked linear sampled-data control systems with band-limited channels. Inf Sci 275:385–399
Wu Z, Shi P, Su H, Chu J (2013) Stochastic synchronization of Markovian jump neural networks with time-varying delay using sampled-data. IEEE Trans Cybern 43:1796–1806
Weng Y, Chao Z (2014) Robust sampled-data \(H_\infty\) output feedback control of active suspension system. Int J Innov Comput Inf Control 10:281–292
Li N, Zhang Y, Hu J, Nie Z (2011) Synchronization for general complex dynamical networks with sampled-data. Neurocomputing 74:805–811
Lu L, Zeng Q, Zhang L, Zhu Y (2014) Passivity and passification for Markov jump genetic regulatory networks with time-varying delays. Neurocomputing 136:321–326
Lu L, Xing Z, He B (2016) Non-uniform sampled-data control for stochastic passivity and passification of Markov jump genetic regulatory networks with time-varying delays. Neurocomputing 171:434–443
Souza de (2004) Mode-independent \(H_\infty\) filters for hybrid Markov linear systems. Proceedings of IEEE international conference on decision and control, pp 947–952
Khalil HK (1996) Nonlinear systems. Prentice Hall, New Jersey
Peng C, Tian YC (2008) Delay-dependent robust stability criteria for uncertain systems with interval time-varying delay. J Comput Appl Math 214:480–494
Liu P (2009) Robust exponential stability for uncertain time-varying delay systems with delay dependence. J Franklin Inst 346:958–968
Huang T, Li C, Duan S, Starzyk JA (2012) Robust exponential stability of uncertain delayed neural networks with stochastic perturbation and impulse effects. IEEE Trans Neural Netw Learn Syst 23:866–875
Johansson KH (2000) The quadruple-tank process: a multivariable laboratory process with an adjustable zero. IEEE Trans Control Syst Technol 8:456–465
Lee TH, Park JH, Kwon OM, Lee SM (2013) Stochastic sampled-data control for state estimation of time-varying delayed neural networks. Neural Netw 46:99–108
Author information
Authors and Affiliations
Corresponding author
Additional information
This work was supported by Department of Science and Technology (DST), under research project No. SR/FTP/MS-039/2011.
Rights and permissions
About this article
Cite this article
Ali, M.S., Gunasekaran, N. & Saravanakumar, R. Design of passivity and passification for delayed neural networks with Markovian jump parameters via non-uniform sampled-data control. Neural Comput & Applic 30, 595–605 (2018). https://doi.org/10.1007/s00521-016-2682-0
Received:
Accepted:
Published:
Issue Date:
DOI: https://doi.org/10.1007/s00521-016-2682-0