Abstract
Ambient air pollution has recently emerged as a major global public health issue, causing a variety of negative health impacts even at the lowest measurable concentrations. This study aims to analyze the spatial distribution of ambient air pollution in Addis Ababa, Ethiopia. The study was based on cross-sectional data collected from 21 selected sites within the period of October 13, 2019 to January 26, 2020, and July 5 to October 29, 2021. The spatial distribution of ambient air pollution was analyzed using spatial autocorrelation (Moran’s I and Geary’s C), and the hotspot areas of ambient air pollution were identified using the Ord and Getis statistics after visualizing via the Moran Scatter Plot. The average concentration of ambient air pollution was modeled against the covariates using a spatial lag model. Moran’s I, and Geary’s C, showed that the spatial distribution of ambient air pollution was globally clustered in the study area. Results revealed that Petros, Tekle Haimanot, and Bob Marley Squares, Legehar, Jamo Mikael, Sholla, Megenagna, African Union traffic signal, Stadium, North and East sampling sites of Akaki Kality's metal welding shade were identified as the hotspot sites of both ambient air pollutants. The results showed that temperature, average wind speed, wind direction, road characteristics, and land use characteristics were statistically significantly associated with the ambient air pollution concentrations. Paying attention to reducing ambient air pollution in pollution hotspot areas is recommended by the government and all concerned bodies.
Similar content being viewed by others
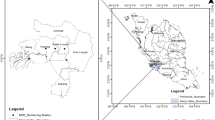
Explore related subjects
Discover the latest articles, news and stories from top researchers in related subjects.Avoid common mistakes on your manuscript.
1 Introduction
Air pollution is the result of emissions of poisonous gas vapors into the air of the environment, which has several negative consequences on health, economy and environment. The major sources of this poison gas fumes include waste burning, coal fire, inefficient modes of transport, industrial, and factory activities (Barreira et al. 2017).
Recently, air pollution is regarded as one of the environmental intimidations to human health through climate change (Whaley and Burns 2021). For instance, nearly 7 million premature deaths are recorded due to air pollution, of which more than 1 million are estimated in the WHO African Region. The criterion considered to indicate the air pollutants are evaluated based on National Ambient Air Quality Standards (NAAQS), which is founded by the United States Environmental Protection Agency (U.S. EPA). These pollutants are carbon monoxide, lead, ground-level ozone, particulate matter, nitrogen dioxide, and sulfur dioxide (Hemming et al. 2006).
According to (Ji et al 2020; Manisalidis et al. 2020) ambient air pollution is becoming one of the major disasters to human health in spreading several airborne diseases. It was also noted that there is an association between air pollutants and respiratory diseases mainly, asthma, wheezing, cough shortness of breath, and chronic obstructive pulmonary.
The spatial distribution of ambient air pollution across the world is varying (Rahmah et al. 2015). For instance, the most vulnerable countries due to ambient air pollution exposure of PM2.5 concentrations were Bangladesh (77.1 µg/m3), Pakistan (59.0 µg/m3), India (51.9 µg/m3), Mongolia (46.6 µg/m3), Afghanistan (46.5 µg/m3), Indonesia (44.4 µg/m3), Bahrain (44.3 µg/m3), Nepal (43.5 µg/m3), Uzbekistan (40.70 µg/m3), and Iraq (40.60 µg/m3) (World Health 2020).
The level of ambient air pollution is increasing in Africa and becoming one of the major environmental and health problems (Njee et al. 2016). Unless an appropriate intervention is taken, it will continue to register more morbidity and mortality (Fisher et al. 2021a, b). The impacts of ambient air pollution certainly contribute to progressively observed epidemiological transformation (Katoto et al. 2019). The growing population and high traffic volume in urban areas also resulted in serious air pollution. The high levels of soot absorption, especially in congested areas, suggest vehicular exhaust emissions as a major source of fine particulates (Njee et al. 2022).
Abera and co-authors indicated that Eastern and Western Sub-Saharan African countries were the most vulnerable African countries (Abera et al. 2020). Along with this, (Fisher et al. 2021a, b) cogently scrutinized the main reasons for the concentration level of particulate matter in Africa include increasing motor vehicles, and climatic and geographic conditions. The particulate matter concentration measured at an urban road site in Nairobi, Kenya, exceeds WHO guidelines (Blake et al. 2018). The study conducted in East African countries suggested that Kampala (Uganda), followed by Addis Ababa (Ethiopia), and Nairobi (Kenya) were the hotspot areas in/with the concentration of PM2.5 and PM10 (Singh et al. 2021). Thus, the spatial distribution of ambient air pollution shows varying levels of intensity in Africa, and Addis Ababa is the second highest vulnerable city in Eastern Africa due to air pollution. Therefore, conducting a study to identify the hotspot areas of ambient air pollution in cities like Addis Ababa with high population density and economic mobility is highly recommended.
The global burden of disease and death associated with air pollution exposure is widely distributed by region; exposure to air pollution is estimated to cause millions of deaths and lost years of healthy life annually (Babatola 2018). The most effective and efficient approach to protecting public health from the adverse effects of outdoor air pollution is to reduce ambient concentrations through emission controls (Chandrappa, R., & Chandra Kulshrestha, U. 2016) to meet the WHO Air Quality Guidelines (Kumie et al. 2021; Whaley and Burns 2021).
According to the (World Health 2020) report the standard concentration values of PM2.5 pollutant were 5 µg/m3 yearly, 15 µg/m3 in 24 h, and of PM10 pollutant was 15 µg/m3 annually, and 45 µg/m3 in 24 h. A study by Evangelpoulos and co-authors shows that 90% of the world's population lived in areas where WHO air quality limits for particulate matter were not being met (Evangelopoulos et al. 2020). The disease burden associated with ambient air pollution among the world's poorest people is highly influenced by demographics, as well as significant disparities in disease burden due to a range of factors (Coates et al. 2021). The distribution of ambient air pollution varies around the world. For instance, in most developing countries, industrial and motor vehicle emissions are the primary sources of pollution. In Low and medium-income countries (LMICs), there is a scarcity of experts and equipment to detect and monitor air pollution levels (World Health 2020). Studies conducted by (Zhou et al. 2020) on the spatial and temporal (Yousefian et al. 2020) on characteristics and influencing factors of particulate matter pollution in China indicate the existence of spatial dependency.
Ambient air pollution is becoming a serious problem in Africa. For instance, in 2019 alone, ambient air pollution was responsible for an estimated 383 out of 419 deaths across Africa. Meanwhile, though air pollution is declining, it still accounts for 60% of all air pollution-related deaths (1.1 million) across Africa (Fisher et al. 2021a, 2021b). However, clearly identifying the hotspot areas of air pollution is important to reduce the risks of air pollution and take an appropriate measure. The burden of air pollution in Ethiopia is becoming one of the serious risks responsible for death and disability (Balidemaj et al. 2021), however clearly identifying the hotspot areas is suggested. According to (Tsegaye et al., 2019), air pollution is also becoming a serious health problem, mainly affecting humans' lives and affecting children and women at large, though its spatial distribution is varying. One of the shortcomings of the studies conducted so far is lack of detail analysis to draw a better inference, from which we noted that identifying the hotspot areas via spatial modelling could contribute positively to making inference. Along this line, a research conducted by (Tefera et al. 2020) assessed the spread of the spatial distribution via descriptive approaches, in which making inference is not advisable. Therefore, to tackle the drawbacks of the existing works, this article is aimed to assess spatial distribution of ambient air pollution and identifying factors associated with it in Addis Ababa, Ethiopia..
1.1 Data and Methodology
1.1.1 Study Area
Addis Ababa is the capital city of Ethiopia and the African Union's administrative center, where there is a high volume of economic mobility, high population density, and industry expansion (Woldesemayat and Genovese 2021). In 2021, the city had an area of 527 square kilometers and a population of 5 Million. In this metropolis, there are 11 sub-cities and 116 woredas (inhabited districts). Geographically, it is located at 90 2'N latitudes and 380 45'E longitudes, in the center of the country. It has an average elevation of 2,400 m above sea level, with the highest altitudes reaching 3,200 m at Entoto Hill to the north. Addis Ababa is thus one of the world's high-altitude capital cities. Moreover, in the city, 21 distinct sites have been identified, primarily Gurara shade and Akaki Kality shade sites, each of which has five sub-sites: the center of the site and 100 m distance from the center site to all four directions (east, west, north, and south) and the other sites are AU signal, Tekle Haimanot square, Bob Marley square, Jamo Mikael square, Petros school, Legehar signal, Sholla signal, Megenagna, Stadium, Kality total, and Maseltegna site. For an easy understanding of the study areas, the specific study areas extracted from the map of Ethiopia are illustrated in Fig. 1.
1.2 Data sources
The say dataset that inspired this study was secondary data collected by Addis Ababa University’s Thematic Research Project team. Average daily concentrations of the air pollutants (PM2.5, and PM10) and some meteorological factors of the selected sites were acquired as secondary data for the current study based on the information gathered by this project. These data were collected from October 13, 2019 to January 26, 2020, and July 5 to October 29, 2021. The Addis Ababa Road Authority provided data on the road characteristics factors. The data were captured in an Excel sheet and exported to R statistical software version 4.1.2. The descriptive analysis including mapping and models were made by using R software and ArcGIS.
1.3 Variables in the study
The dependent variables/outcome measurements in this study were an average daily concentration of PM2.5 and PM10. Depending on the nature of the data, the proposed objectives, and other related works, the predictor variables included were meteorological variables (Elevation, Temperature, Relative humidity, Wind speed, Wind direction), land use characteristics (Land use area), and road characteristics of the selected sites (Road type, width of the road, Neighborhood Road number). In this study, we assessed the spatial distribution of ambient air pollution in Addis Ababa.
1.4 Methodology of the study
1.4.1 Methods of measuring spatial autocorrelation
Spatial weight matrix
There are two types of spatial weight matrices used to model spatial autoregression and to detect spatial autocorrelation. In this study, a distance-based weight matrix was used because the data were not polygon data rather it was point pattern data.
Distance-based weight matrix
Distance is the extent or the amount of space between two locations or coordinates (Suryowati et al. 2018). The non-standardized spatial weight matrix for distance , denoted as \(W(d)\), is defined as:
where \(hypot\left(i,j\right)=\sqrt{{({y}_{i}-\overline{y })}^{2}+{({y}_{j}-\overline{y })}^{2}}\) ,\(d\) is the maximum distance between two locations, \({y}_{i}\;and\;{y}_{j}\) are observations of the dependent variable at location \(i\;and\;j\) respectively and \(\overline{y }\) is the mean value of a dependent variable.
1.5 Test of global spatial autocorrelation
Spatial autocorrelation is an important concept in spatial statistics, and it is used to measure the similarity between nearby observations (Miller HJ 2004). Test for spatial autocorrelation is designed to quantify the extent of clustering and to allow for statistical inference (Chen 2016; Dale & Fortin 2009; Kelejian & Robinson 1992) and Anselin L (1988a). The null hypothesis (under the normality and independence assumptions) (Jarque CM & Bera AK 1980) is given by:
Against the alternative hypothesis of spatial dependence/autocorrelation (\({H}_{1}: \rho \ne 0\)) which is the claim of the interest. To test this hypothesis, we have used Moran’s I Correlation and Geary’s C analysis.
1.6 Moran’s I
Moran’s I correlation coefficient is widely used to identify the spatial association pattern (Schober et al. 2018). To compute the spatial autocorrelation of the ambient air pollution Moran’s I was employed. The value of Global Moran’s I lie in the interval of -1 and 1. This indicates that if this value is significantly less than 0, then there is a negative spatial relationship; if it is greater than zero there is a positive spatial relationship and if it is zero there is no spatial relationship. Whereas Local Moran’s I was used to identify the local spatial pattern and outliers among ambient air pollution of selected sites (Cellmer 2013; Xu et al. 2019). The analysis was conducted using R software 4.1.2 version.
Global Moran’s I is given by:
where \({I }_{g}\) is Global Moran’s I correlation coefficient; \({y}_{i}\) and \({y}_{j}\) respectively represent the daily average concentration of ambient air pollutants of site \(i\) and site \(j\); \(\overline{y }\) is the mean of y; \(n\) is the number of sites; and \({W}_{ij}\) is the elements of row standardized spatial weights between site \(i\) and site \(j\).
1.7 Geary’s C
The global Geary’s C defines value (dis)similarity as the squared difference in values between neighboring observations. For binary weights, Geary (1954) introduced the following coefficient of autocorrelation:
where \(n\), \({W}_{ij},{y}_{i}, {y}_{j}\;and\;\overline{y }\) are denoted as above Eqs. (2), and \(C\) is Geary’s C value.
1.8 Local tests of spatial autocorrelation
Measures of local spatial autocorrelation, such as local Getis and Ord statistics, and the local indicator of spatial association, are used to see if there is a local spatial cluster of high or low values and to identify the regions that contribute the most to the clustering (spatial autocorrelation) (Anselin, 1995). Our concern is frequently focused not only on establishing if the data as a whole exhibits spatial autocorrelation, but also on finding the specific observations that show spatial autocorrelation with their neighbors. The following local measures were employed in this investigation.
1.9 Local Moran’s I
Local Moran's I for each observation, according to Anselin (1995), indicates the level of significant spatial clustering of similar values around that observation. The Local Moran’s I formula is given by:
where variables are as described in Eq. (4) and \({I }_{i}\) is Local Moran’s I correlation coefficient.
1.10 Local Ord and Getis \({{\mathbf{G}}_{\mathbf{i}}}^{\mathbf{*}}\) Statistic
The Ord and Getis \({{\text{G}}_{\text{i}}}^{*}\) test statistic is given by
where \({\text{W}}_{\text{ij}}\), \({\text{y}}_{\text{j}}\), \(\text{n}\) and \(\overline{\text{y} }\) are as described in Eq. (5).
\({\text{W}}^{*}=\sum_{\text{j}=1}^{\text{n}}{{\text{W}}_{\text{ij}}}^{2}\) and \(\text{Sd}\) is denoted as the sample standard deviation of the observations.
1.11 Diagnostics for spatial dependence
After identifying significant spatial dependency using global and local spatial autocorrelation tests (Cliff & Ord K 1972), the next step was to model this spatial autocorrelation using covariates. The diagnostics Moran's I error correlation, Lagrange Multiplier, and Robust Lagrange Multiplier were used to check if the covariates included in the study fully model the spatial dependency.
1.12 The Moran’s I error correlation test
Moran's I can be used to diagnose spatial dependence in the presence of variables. The following is Moran's I test statistic for spatial error dependence in OLS regression residuals:
where \({I }_{\varepsilon }\) is the Moran’s I correlation coefficient of the error term, \(W\) is row standardized spatial weight matrix, \(n\) and \({W}_{ij}\) are denoted as above (Eqs. (4) and (6)), and \(\varepsilon\) is the \(n*1\) vector OLS residuals.
1.13 Lagrange Multiplier Diagnostic for Spatial Dependence
The use of Lagrange Multiplier (LM) diagnostics in OLS specifications is advocated by Anselin and Rey (1991). There are two basic LM diagnostics described in the following subsections. The Lagrange Multiplier is diagnostic for spatial lag dependence and spatial error dependence in the presence of covariates in an OLS model. In regression models, spatial dependence may not simply be reflected in the error. Instead, a spatial lag \(WY\) in the endogenous variable \(Y\) might be used to account for it (Anselin 1988a).
1.14 Lagrange Multiplier Diagnostic for Spatial lag Dependence
Under this this set-up, the regression model reads \(Y= \rho WY+X\beta +\varepsilon\), and the Lagrange Multiplier test of spatial lag dependence under the null hypothesis of no spatial lag dependence (\({H}_{o}: \rho =0\)) versus the alternative hypothesis of (\({H}_{1}: \rho \ne 0)\) is considered.
The Lagrange Multiplier test statistic of spatial lag dependence is given as:
Where \(n\) is the number of observations; \(\varepsilon\) is the vector of OLS residuals; \(tr\) is the matrix trace operator, and \(W\) is the spatial weights matrix for the spatial lagged dependent variable \(Y\) is the value of a predicted variable.
1.15 The Lagrange Multiplier Diagnostic for Spatial Error Dependence
The Lagrange Multiplier diagnostic for spatial error dependence in the presence of covariates in an OLS model is based on the estimation of the regression model
with spatially dependent error term \(\varepsilon\). The Lagrange Multiplier test of spatial error dependence under the null hypothesis of no spatial error dependency (\({H}_{o}: \lambda =0\)) against the alternative hypothesis of (\({H}_{1}: \lambda \ne 0\)).
The Lagrange Multiplier test statistic of spatial error dependence is given as:
where \(W\) is the spatial weights matrix for the spatially lagged error term and the rest notations as in Eq. (10).
1.16 Robust Lagrange Multiplier Diagnostics for Spatial Dependence
The modified Lagrange Multiplier tests are used to diagnose spatial lag and spatial error dependence in OLS specifications as the Robust Lagrange Multiplier (RLM) diagnostics for the OLS model, and are described below.
1.17 The Robust Lagrange Multiplier Diagnostic of the Spatial lag Dependence
Under the null hypothesis of no spatial lag dependency, the OLS regression model satisfies:
The test statistic of RLM for a lag model is
where \({T}^{*}=\left[T+{~}^{T{\left(WX\beta \right)}^{\prime}M\left(WX\beta \right)}\!\left/ \!{~}_{{S}^{2}}\right.\right]\) and other notation as above Eqs. (10 and 11).
1.18 The Robust Lagrange Multiplier Diagnostic of the Spatial error Dependence
Under the null hypothesis of no spatial error dependence given as
The test statistic of the RLM of error dependence is given by
where \({RLM}_{error}\) is the Robust Lagrange Multiplier statistic for error dependence, \(\lambda\) is the spatial dependence of the error term in the OLS regression model, and other notations remain as the above Eqs. (10 and 11).
1.19 Spatial regression model
1.19.1 Spatial regression model specification
The variables were included in the model based on their availability in the secondary data and literature. When there is no prior theoretical foundation for the selected models the indications given by an exploratory analysis of data (Using LISA statistics) can be very useful (Chou, Y.-H. 1997). We consider two types of spatial regression models (Srinivasan, S. 2015) namely the spatial lag model and the spatial error model (Anselin 1988b).
1.19.2 Spatial Lag regression model
A spatial lag regression model is a method for controlling the spatial autocorrelation of a dependent variable when the dependent variable has a spatially dependent relationship (Anselin 1988a, b). The spatial lag model incorporates the influence of unmeasured variables but also stipulates additional effects of neighboring attribute values; e.g., the lagged dependent variable. The spatial lag model is given as follows (Anselin et al. 2008a, 2008b; Cellmer 2014; Loftin & Ward 1983; Zwack et al. 2011)
Or its reduced form is given as
where \(Y\) is \(N*1\) vector of observations of the dependent variable which, in this study, is the average daily concentration of air pollutants in-unit measurement, \(WY\) is an \(N*1\) vector weighted average of a spatial lag of the dependent variables, \(\rho\) is coefficient of spatial lag of the dependent variable, \(X\) is a matrix of observations on the independent variables with \(N*K\) dimension, \(\beta\) is \(K*1\) vector of the coefficients of the independent variables, and \(\varepsilon\) is \(N*1\) vector of random error term which is normally distributed and \(I-\rho W\) is invertible since the weight matrix and identity matrices are non-singular and symmetric matrices.
1.19.3 Spatial error regression model
The spatial error model evaluates the extent to which the clustering of outcome variables not explained by measured independent variables can be accounted for regarding the clustering of the terms. In this sense, it captures the influence of unmeasured independent variables. The spatial model can be expressed in two equations as follows (Anselin et al. 2008a; Loftin & Ward 1983; Zwack et al. 2011).

where \(Y\) is \(N*1\) vector of observation of the dependent variable which is the average daily concentration of air pollutants in-unit measurement, \(W\varepsilon\) is a vector of spatial lag for the nearest values of the error terms, \(\lambda\) is the coefficient of spatial lag of error terms, \(X\) is an \(N*K\) matrix of observation on the independent variables like; meteorological factors and socio-economic factors, \(\beta\) is \(K*1\) vector of regression coefficients of independent variables, and ε is N * 1 vector spatially autocorrelated error term and
is another vector of error terms.
1.19.3.1 Method of parameter estimation
Typically, a model is estimated without incorporating spatial effects, but the results of this estimation (and its residuals) form the starting point for the diagnostics of spatial effects. Consequently, Ordinary least square estimation yields inconsistent and biased estimates, and inference based on this method was flawed. Instead of OLS, other estimation methods must be employed to properly account for the spatial simultaneity in the model. Alternative methods of estimation include Maximum-likelihood and application of instrumental variable estimation for the spatial two-stage, least-square approach (Lesage 2004; Ord 1975)(Loftin & Ward 1983).
1.19.3.2 Model adequacy checking
The assessment of the empirical fit of the estimated model is an important aspect of statistical analysis. In spatial regression analysis, this is slightly complex due to a lack of standard measures, such as R2. If the estimation is based on Maximum likelihood, then the standard R2 will be invalid. Appropriate estimation approaches would be maximized log-likelihood and information-based criterion. The implementation of the information-based criteria of model validity in spatial analysis is straightforward (Akaike 1981; Anselin 1988b). The criteria under this category include:
where \(AIC\;and\;SC\) are Akaike information criteria and the Schwartz criterion, respectively. \(LL\) is log-likelihood, \(K\) is the number of the estimated parameter and \(N\) is the number of observations.
2 Results and Discussions
2.1 Descriptive results of the spatial distribution of PM2.5 and PM10 concentration
The spatial distributions with a descriptive result corresponding to the average daily concentration of PM2.5 and PM10 are given in Figs. 2 and 3 respectively. The observed average daily concentration levels of PM2.5 and PM10 at the Gurara shade sites (Center, East, West, North, and South) were 46.60 µg/m3 to 55.00 µg/m3 for PM2.5 and 88.70 µg/m3 to 90.06 µg/m3 for PM10, The daily average PM2.5 and PM10 concentrations observed were in the range of 71.41 µg/m3 to 78.20 µg/m3 and 90.07 µg/m3 to 97.46 µg/m3 at Petros Square, Akaki North, and Kality total sites respectively, whereas, 55.01 µg/m3 to 71.40 µg/m3 and 97.47 µg/m3 to 103.83 µg/m3 at Akaki kality industrial areas (Center, East, West and North), respectively. The daily average PM2.5 concentration at Bob Marley squares was found in a range of 78.21 µg/m3 to 88.39 µg/m3. The daily average PM2.5 concentration at Tekle Haimanot square, Shola, Megenagna, Legehar, Stadium, and AU signal ranged from 88.40 µg/m3 to 106.72 µg/m3. The average PM10 concentration at Shola, Megenagna, Stadium, AU signal, and Jamo Mikael sites ranged from 103.84 g/m3 to 116.00 g/m3, while the values for Tekle Haimanot, Legehar, and Bob Marley square ranged from 116.01 g/m3 to 120.12 g/m3. The results indicate that the daily average concentration of ambient air pollutants (PM2.5 and PM10) exceeded the WHO standard air quality standards of the air pollutants concentration at all sites considered in the study area.
Table 1 shows the overall average (mean, SD) concentrations of the ambient air pollutants under investigation. Accordingly, PM2.5 and PM10 had mean and standard deviations of (77.72 and 19.54) and (103.99 and 10.34), respectively. This indicates that the overall average concentration of these pollutants exceeded the WHO's daily standard air quality criteria. The averaged updated WHO daily standard air quality criteria values of the PM2.5 and PM10 were 15 µg/m3 and 45 µg/m3, respectively.
2.2 Testing for spatial autocorrelation air pollutants concentration
In this subsection, we present the results of autocorrelation analysis using both global (Moran's I and Geary's C) and local (local Moran's I and local Getis and Ord Gi *) statistics for clustering. The Moran scatter plot is used to show the global test, in which the slope of the regression line corresponds to Moran's I. Local Moran's I and Getis and Ord Gi * statistics were used in the analysis. The result indicates that there is no statistically significant clustering of air pollutants in selected sites, at a significance level of \(=0.05\). Therefore, in the following subsection, the result corresponding to Moran's I and Geary's C test statistics is cogently addressed. Furthermore, a diagnostic test for spatial dependence was employed to ensure consistency.
2.3 Tests of spatial autocorrelation using global Moran’s I and Geary’s C statistics
In this study, interpolation was performed to measure the ambient air pollution concentration at the selected sites. The result shown in Table 2 is used to check whether there is spatial autocorrelation globally.
The results corresponding to the global Moran's I and Geary's C coefficients show that there is a statistically significant positive global spatial autocorrelation at 5% level of significance. The result of the present study also confirms that the spatial distribution of average daily concentrations of PM2.5 and PM10 were clustered in selected sites of Addis Ababa at a 5% level of significance (Table 2). Along with this, under the assumption of normality, the result corresponding to Moran's scatter plot is illustrated to pinpoint the issue of global spatial autocorrelation (Fig. 4).
2.3.1 Moran’s scatter plot of the air pollutants concentration
The visualization of the spatial autocorrelation of PM2.5 is presented using the Moran scatter plot, where the Y-axis is the spatially lagged dependent variable (W_PM2.5) plotted against the X-axis, the dependent variable (PM2.5). Moran's scatter plot reveals that lagged PM2.5 and PM2.5 daily average concentrations have a positive spatial autocorrelation (clustering).
Similarly, the result of spatial autocorrelation of the PM10 with its lagged values (W_PM10) is depicted in Moran's scatter plot. This shows that the PM10 and its lagged value (W_PM10) have a positive spatial association (clustering) based on the result of the Moran scatter plot. The value of the Moran I correlation which is the slope of the Moran scatter plot given in Table 3 of average daily PM2.5 and PM10 concentrations were positive and significant. This also indicates that the nearby sites that have high-high clustering (high value surrounded by high value) and low-low clustering (low value surrounded by low value) are the points clustered in the third and first quadrants of the Moran scatter plot. Very few points were clustered in the second and fourth quadrants, where the outliers of the low values are surrounded by the high values and vice versa for both pollutants (Fig. 4).
2.4 Local Moran’s I of the PM2.5 and PM10 concentration
The results of the global test statistic for spatial dependency presented in Section 4.2.1 show that ambient air pollution from neighboring locations has a strong positive spatial autocorrelation (clustering). Local statistics of spatial autocorrelations were employed in addition to these local statistics to further indicate whether there is a high/low value clustering.
To test the null hypothesis of no local spatial clustering among average concentrations of ambient air pollutants at nearby selected sites, the results of the local Moran's I, local Getis, and local Ord Gi* statistics were taken into consideration. The results show sites where values are physically clustered and where values are vastly different from those of their neighbors (outliers).
Table 3 shows that, in most of the sites there was statistically significant local clustering of PM2.5 and PM10 at a 5% level of significance. PM2.5 and PM10 were found to have statistically significant local spatial dependency (clustering) among several sites.
The results of the local Moran I presented in Table 3 show that, at sites like Gurara shade in all directions from the center, including the central site, Petros Square, Bob Marley Square, Legehar, Tekle Haimanot square, Jamo Mikael, Megenagna, and Stadium, a significant clustering of PM2.5 and daily PM10 average concentration was observed. The average concentration of pollutant PM10 at East and west of Akaki shades sites show strong clustering with a p-value less than a 5% threshold of significance.
Petros square and Tekle Haimanot square are among the sites that have significant negative local spatial autocorrelation (clustering) for both PM2.5 and PM10 average concentration, indicating that low values are surrounded by high values of neighboring/nearest sites or high values are surrounded by the low values of the nearest sites. While all Gurara shade sites, as well as Bob Marley Square, Legehar, Jamo Mikael, Megenagna, and Stadium, have positively significant spatial clustering on both key pollutants, Akaki shade from the center to the north and south directions is positively spatially clustered only for PM2.5 average concentration. AU signal, and Akaki shade from the center to the east and west directions show positive spatial clustering for an average concentration of PM10. The low value of one site is surrounded by low values or the high value of one site is surrounded by the high values of other nearest sites in this positive local spatial autocorrelation.
2.5 Local Ord and Getis statistics for an average concentration of PM2.5 and PM10
Local clustering of high (hotspots) or low (cold spots) average ambient air pollutant concentrations surrounds each site with nearby sites, according to the local Ord's and Getis statistic, \({{G}_{i}}^{*}\). To establish the degree of clustering of ambient air pollution concentrations among the selected sites, the results of \({{G}_{i}}^{*}\) statistic, as well as its p-value, were determined using R software, version 4.1.2. Getis and Ord's criterion was used to adjust the p-values of \({{G}_{i}}^{*}\) statistic for multiple comparisons. The findings of the \({{G}_{i}}^{*}\) statistic are shown in Table 4. The significance of the \({{G}_{i}}^{*}\) statistic was calculated using a 5% significance threshold. The positive value of \({{G}_{i}}^{*}\) statistics shows the spatial clustering of high values (hot spots), whereas the negative value reflects the spatial clustering of low values (cold spots). The clustering of the high or low value of the pollutant's concentrations is depicted in Fig 5. and Fig. 6.
All Gurara shades sites were cold spot clusters of the average concentration of PM2.5 with a 99 percent confidence level, whereas Megenagna, Sholla, Tekle Haimannot, Petros square, Akaki center, Akaki east, Akaki north, and Jamo Mikael are hotspot clusters with a 95 percent confidence level; and Bob Marley square, Legehar, AU signal, and Stadium are hot spot sites with a 99 percent confidence level of the average concentration of the PM2.5 (Fig. 5).
Fig. depicts the result of the average PM10 pollutant concentration distribution. At the 99 percent confidence level, the majority of the areas had a clustering of high values of the average PM10 concentrations. The Akaki kality industrial areas (Akaki East, Akaki West, Akaki North, and Akaki South), Petros Square, Tekle Haimanot Square, AU signal, Jamo Mikael Square, Sholla, and Megenagna were hotspot clusters with a 99 percent confidence level, while the other three sites, Bob Marley square, Legehar, and Stadium, were hotspot clusters at 95 percent confidence level of this ambient air pollutant. The remaining locations had insignificant clustering of the average concentration of PM10.
2.6 Diagnostic for Spatial Dependence
The spatial dependence diagnostics were taken based on the results of the estimation of the OLS regression model of the daily average concentration of PM2.5 and PM10 (Table 4). The daily average concentrations of PM2.5 and PM10 show spatial clustering globally in the study area, as indicated by the results of global and local measures of spatial dependency. The spatial autocorrelation cannot be controlled when using the OLS regression, and the results become biased as the issue of spatial dependency is cast out. To tackle this setback, the spatial autoregressive model is taken into consideration. The model diagnostics are verified using the Lagrange Multiplier test and the Robust Lagrange multiplier test of the dependent variable's lag and the OLS regression model's lag error term. As shown in Table 4, the Moran's I (error) value is 0.0699 with p-value of 0.01644, and Moran's I (error) value is 0.0511 and with the p-value 0.02765 for PM2.5 and PM10 respectively.
This suggests that the spatial error te rms are spatially dependent, which contradicts the standard linear model's assumption. When the SLR and SER models were compared using the model's diagnostic tests, the Lagrange multiplier tests for the SLR model were found to be significant for both ambient air pollutants, as shown in Table 4. As a result, spatial regression was utilized with the premise that spatial correlation structures apply uniformly across the data set, and the diagnostic results indicated that the five tests were used to evaluate the model's spatial dependence.
As noted in Table 4, the spatial lag model is better than the SER model in terms of controlling the influence of close sites' spatial dependency because the LM and Robust LM tests for the Spatial lag model of both pollutants are found significant statistically.
2.7 Results of model specification
After a diagnosis of the spatial dependence (Tobler 1970, 1979), the model specification was made between the spatial lag regression and spatial error regression model. The Akaike Information Criterion (AIC), and log-likelihood analysis were also used to find suitable models (Table 5 and 6), where the results of the model with the smallest value of AIC and highest value of Log-likelihood were appriopriate. The results of the OLS regression model, SLR, and SER models were shown for pollutants vs variables. As indicated in Table 5 and 6, the AIC for the spatial lag model is lower than that of the spatial error regression model, and its log-likelihood is higher than that of the spatial error regression model, which suggests that the spatial lag regression model is suitable. As a result, by integrating the effects of spatial autocorrelation, the spatial lag regression model can be considered as appropriate for modeling the spatial distribution of ambient air pollutants versus covariates considered in this study.
Based on the results presented above, the spatial lag regression model was utilized, as the final model, to model the pollutants. The spatial lag model results of PM2.5 and PM10 are described in detail in the next.
2.8 Results of the spatial lag model for pollutants
In this section, the results of the spatial lag model for both PM2.5 and PM10 are well described based on the selected predictor variables. The result (Table 7) shows that all the predictor variables are found significantly associated with PM2.5 and PM10 concentration except RH, WDE-S, and RTCoble.
3 Discussions
The findings of the study identify the spatial distributions of ambient air pollutants and major driving factors responsible for the spread of ambient air pollutants at the selected sites in Addis Ababa, Ethiopia. The overall daily average concentrations of ambient air pollution were computed from all selected sites in the city, from which we noted that the overall average daily concentration level of air pollutants in Addis Ababa was highly above the air quality guidelines of the daily standard air quality level of the world.
This study identified the spatial distribution of the air pollutants in Addis Ababa among selected sites using spatial autocorrelation and visualizing the spatial clusters based on the Moran scatter plot. The results reveal that there is spatial clustering of the daily average concentration of ambient air pollution. Furthermore, the hotspot areas of the ambient air pollutants concentration were identified using the Getis-Ords statistic for the selected sites. The results show that spatial clustering of hotspot and cold spot areas of the average PM2.5 and PM10 concentration was observed. Among the sites Petros Square, Tekle Haimanot Square, Bob Marley Square, Legehar, Jamo Mikael, Sholla, Megenagna, AU signal, Stadium, Akaki East, and Akaki north were identified as hotspot clusters of daily average concentrations of both ambient air pollutants. Whereas Akaki kality sites (west, and south) were hotspot sites only for the daily average PM10 concentration. Akaki kality site, from the center, was a hotspot cluster only for the daily average concentration of PM2.5.
Following this, efforts were also made to identify the potential predictors that characterizes the spatial distributions of the average concentrations of air pollution in the study area. Therefore, based on the results of the spatial lag model, it was found that the average temperature has a significant effect on the average concentrations of ambient air pollutants. The finding of our study more resembles the work done by (Okimiji et al. 2021) which suggested that the average temperature was the primary meteorological factor that affected the distribution of the concentration of PM2.5. Additionally, The findings of the study are also comparable to the work of (Park and Ko 2021) and (Zhou et al. 2020) and aligns with the study conducted by (Hart et al. 2020). However, the findings achieved in the work of (Hart et al. 2020) aligns with our study. Addressing the issue of spatial dependency in this work is one of the major benefits as compared to the aforementioned related works.
The findings of the study have shown that elevation has a significant negative association with the distributions of the daily average concentrations of the PM2.5 and PM10 pollutants, which is more similar to the work of (Han et al. 2020). This research additionally considered the issue of spatial dependency via the spatial autoregressive model after cogently scrutinizing the global and local spatial clustering.
The relative humidity in this study has no significant effect on the distribution of air pollution and is more consistent with the findings of (Hart et al. 2020). Moreover, the findings of this study showed that relative humidity is negatively associated with a concentration of PM2.5 significantly which is also argued by different previous studies (Li et al. 2017; Park & Ko 2021; Zhou et al. 2020). Furthermore, (Okimiji et al. 2021) also reported that relative humidity was the primary meteorological factor that significantly affects the distribution of PM2.5.
The finding of the present study indicates, that as average wind speed increases the average ambient air pollution concentrations decreases which is consistent with the studies conducted by (Li et al. 2017; Zhou et al. 2020). The result also shows that the average wind speed and the concentration of ambient air pollution have an inverse relation to each other which is inconsistent with (Hart et al. 2020), by supporting the direction of the association between wind speed with average PM2.5 concentration in a positive relationship. The current study findings are in line with the expected association of wind speed with the average concentration of PM2.5 as the high mobility of the air in the atmosphere reduces the concentration of ambient air pollution (Zhou et al. 2020).
The wind direction has a significant association with the distribution of the ambient air pollutants concentration, and is also supported by earlier study findings (Hart et al. 2020; Li et al. 2017; Zhou et al. 2020). The result of the study indicated that land use characteristics have a significant effect on the distribution of ambient air pollutants. The industrial area has a significant effect on the distribution of ambient air pollution which more resembles the result of (Han et al. 2020; Park and Ko 2021). The result of the current study again shows that the mixed land use area has a higher association with daily average concentrations of ambient air pollutants than a commercial area. However, the result of the study (Park and Ko 2021) suggests that there is no significant association between the Mixed land use area and ambient air pollutant concentrations.
The road characteristics such as the number of neighborhood roads, road type, and row size of the road were significantly associated with the distribution of the pollutants. The number of neighborhood roads has a positive significant effect on the distributions of average concentrations of ambient air pollutants regardless of the pollutant type, but not consistent with another study (Park and Ko 2021) which states that the number of neighborhood streets has no significant effect on the distributions of the air pollutants. A road type has a significant association with the distribution average concentrations of PM2.5 and not for the PM10 pollutant. The results of the study reveal row size of the road is significantly negatively associated with the distributions of the average ambient air pollutant concentration regardless of the pollutant type. The distribution of the concentration of the ambient air pollutants is also affected by the spatially lagged dependent values of the neighboring sites as indicated by the coefficients of the spatially lagged value (\(WY\)). This is also consistent with the results of previous study (Park and Ko 2021).
As compared with the aforementioned baselines, this work is better at addressing the spatial distribution of ambient air pollution (PM2.5 and PM10) via incorporating the spatial dependence in terms of the relation of each site with its neighbors and determining the associated factors of the distribution of these ambient air pollutants in Addis Ababa, Ethiopia.
4 Conclusions
This study is aimed to analyze the spatial distribution of ambient air Pollution in Addis Ababa, Ethiopia. The result attained via the global spatial autocorrelation shows the spatial distribution of ambient air pollutants is clustered globally at a 5% level of significance. This is due to the impact of the spatial dependency, which was taken into account via neighboring factors, which has a substantial impact on the distribution of daily ambient air pollution concentrations. The result of the study showed that Petros Square, Tekle Haimanot Square, Bob Marley Square, Legehar, Jamo Mikael, Sholla, Megenagna, AU signal, Stadium, Akaki East, and Akaki North were hotspot sites for both ambient air pollutants, namely PM2.5 and PM10. Whereas the Akaki kality sites (west, and south) were hotspot sites for only daily average PM10 concentration and the Akaki kality site from the center was a hotspot site for the average concentration of PM2.5 only.
This study aimed to also showed that the spatial lag values of both pollutants' concentrations had a considerable impact on the pollutant's concentration distribution at neighboring places. The results of the study also indicated that elevation, row size of the road, average wind speed and temperature show statistically significant negative relationship with the ambient air pollutants (PM2.5 and PM10 concentrations) at a 5% level of significance. The number of neighborhood roads, on the other hand, has a significant positive relationship with the average concentration of ambient air pollutants. Road type was only significantly related to the daily average concentration of PM2.5. Land use characteristics variables and wind direction were significantly related to the daily average concentration of the ambient air pollutants.
As the daily average concentration of ambient air pollution in the study area is relatively greater than the WHO standard, further intervention is required by the government and environmental protection authority jointly to mitigate the spread of air pollution in the study areas. Paying special attention is recommended in the hotspot areas of ambient air pollution concentration in Addis Ababa, Ethiopia. In this regard, policymakers and planners should make good decisions in monitoring air quality in Addis Ababa city. Future works should focus on considering all the potential predictor variables such as population density, morphological building index characteristics, vehicular exhaustive characteristics, etc., to understand the effects of air pollution.
Data availability
All the data are available on request.
References
Abera, A., Mattisson, K., Eriksson, A., Ahlberg, E., Sahilu, G., Mengistie, B., Bayih, A. G., Aseffaa, A., Malmqvist, E., & Isaxon, C. (2020). Air Pollution Measurements and Land-Use Regression in Urban Sub-Saharan Africa Using Low-Cost Sensors—Possibilities and Pitfalls. Atmosphere 11(12). https://doi.org/10.3390/atmos11121357
Akaike H (1981) Likelihood of a model and information criteria. Journal of Econometrics 16(1):3–14. https://doi.org/10.1016/0304-4076(81)90071-3
Anselin L (1988a) Lagrange Multiplier Test Diagnostics for Spatial Dependence and Spatial Heterogeneity. Geogr Anal 20(1):1–17. https://doi.org/10.1111/j.1538-4632.1988.tb00159.x
Anselin L (1988b) Model Validation in Spatial Econometrics: A Review and Evaluation of Alternative Approaches. Int Reg Sci Rev 11(3):279–316. https://doi.org/10.1177/016001768801100307
Anselin L, Gallo JL, Jayet H (2008a) Spatial Panel Econometrics. In: Mátyás L, Sevestre P (eds) The Econometrics of Panel Data: Fundamentals and Recent Developments in Theory and Practice. Springer Berlin Heidelberg, pp 625–660. https://doi.org/10.1007/978-3-540-75892-1_19
Anselin, L., Gallo, J. Le, & Jayet, H. (2008b). Spatial Panel Econometrics BT - The Econometrics of Panel Data: Fundamentals and Recent Developments in Theory and Practice (L. Mátyás & P. Sevestre (eds.); pp. 625–660). Springer Berlin Heidelberg. https://doi.org/10.1007/978-3-540-75892-1_19
Babatola SS (2018) Global burden of diseases attributable to air pollution. J Public Health Africa 9(3):813. https://doi.org/10.4081/jphia.2018.813
Balidemaj, F., Isaxon, C., Abera, A., & Malmqvist, E. (2021). Indoor Air Pollution Exposure of Women in Adama, Ethiopia, and Assessment of Disease Burden Attributable to Risk Factor. Int J Environ Res Public Health 18(18). https://doi.org/10.3390/ijerph18189859
Barreira A, Patierno M, Bautista CR (2017). Impacts of pollution on our health and the planet: The case of coal power plants. UN environment. Perspective 28:1–10
Blake R, Pope F, Gatari M (2018) Airborne particulate matter monitoring in Nairobi, Kenya using calibrated low-cost sensors. EGU General Assembly Conference Abstracts 702
Cellmer R (2014) Use of spatial autocorrelation to build regression models of transaction prices. Real Estate Management and Valuation 21(4):65–74. https://doi.org/10.2478/remav-2013-0038
Cellmer, R. (2013). Use of spatial autocorrelation to build regression models of transaction prices. Real Estate Management and Valuation, 21. https://doi.org/10.2478/remav-2013-0038
Chandrappa, R., & Chandra Kulshrestha, U. (2016). Major Issues of Air Pollution BT - Sustainable Air Pollution Management: Theory and Practice (R. Chandrappa & U. Chandra Kulshrestha (eds.); pp. 1–48). Springer International Publishing. https://doi.org/10.1007/978-3-319-21596-9_1
Chen Y (2016) Spatial Autocorrelation Approaches to Testing Residuals from Least Squares Regression. PLoS One 11(1):e0146865. https://doi.org/10.1371/journal.pone.0146865
Chou Y-H (1997) Exploring spatial analysis in geographic information systems
Cliff A, Ord K (1972) Testing for Spatial Autocorrelation Among Regression Residuals. Geogr Anal 4(3):267–284. https://doi.org/10.1111/j.1538-4632.1972.tb00475.x
Coates MM, Ezzati M, Robles Aguilar G, Kwan GF, Vigo D, Mocumbi AO, Becker AE, Makani J, Hyder AA, Jain Y, Stefan DC, Gupta N, Marx A, Bukhman G (2021) The burden of disease among the world’s poorest billion people: An expert-informed secondary analysis of Global Burden of Disease estimates. PLoS One 16(8):e0253073. https://doi.org/10.1371/journal.pone.0253073
Dale MRT, Fortin M-J (2009) Spatial Autocorrelation and Statistical Tests: Some Solutions. Journal of Agricultural, Biological, and Environmental Statistics 14(2):188–206 (http://www.jstor.org/stable/20696567)
Evangelopoulos D, Perez-Velasco R, Walton H, Gumy S, Williams M, Kelly FJ, Künzli N (2020) The role of burden of disease assessment in tracking progress towards achieving WHO global air quality guidelines. Int J Public Health 65(8):1455–1465. https://doi.org/10.1007/s00038-020-01479-z
Fisher S, Bellinger DC, Cropper ML, Kumar P, Binagwaho A, Koudenoukpo JB, Park Y, Taghian G, Landrigan PJ (2021a) Air pollution and development in Africa: impacts on health, the economy, and human capital. Lancet Planet Health 5(10):e681–e688. https://doi.org/10.1016/S2542-5196(21)00201-1
Fisher S, Bellinger DC, Cropper ML, Kumar P, Binagwaho A, Koudenoukpo JB, Park Y, Taghian G, Landrigan PJ (2021b) Air pollution and development in Africa: impacts on health, the economy, and human capital. Lancet Planet Health 5(10):e681–e688
Han L, Zhao J, Gao Y, Gu Z, Xin K, Zhang J (2020) Spatial Distribution Characteristics of PM2.5 and PM10 in Xi’an City Predicted by Land Use Regression Models. Sustain Cities Soc 61:102329. https://doi.org/10.1016/j.scs.2020.102329
Hart R, Liang L, Dong P (2020) Monitoring, Mapping, and Modeling Spatial-Temporal Patterns of PM(2.5) for Improved Understanding of Air Pollution Dynamics Using Portable Sensing TechnologiesInt J Environ Res. Public Health 17(14):4914. https://doi.org/10.3390/ijerph17144914
Hemming B, Harris A, Davidson C (2006) Environmental Protection Agency (US-EPA). Air Quality Criteria for LeadFinal Report. U.S. Environmental Protection Agency, Washington, DC, EPA/600/R-05/144aF-bF 2006. In, 627-675, Wiley Online Library
Jarque CM, Bera AK (1980) Efficient tests for normality, homoscedasticity, and serial independence of regression residuals. Econ Lett 6(3):255–259. https://doi.org/10.1016/0165-1765(80)90024-5
Ji M, Jiang Y, Han X, Liu L, Xu X, Qiao Z, Sun W (2020) Spatiotemporal Relationships between Air Quality and Multiple Meteorological Parameters in 221 Chinese Cities. Complexity 2020:6829142. https://doi.org/10.1155/2020/6829142
Katoto PDMC, Byamungu L, Brand AS, Mokaya J, Strijdom H, Goswami N, De Boever P, Nawrot TS, Nemery B (2019) Ambient air pollution and health in Sub-Saharan Africa: Current evidence, perspectives and a call to action. Environ Res 173:174–188. https://doi.org/10.1016/j.envres.2019.03.029
Kelejian HH, Robinson DP (1992) Spatial autocorrelation: A new computationally simple test with an application to per capita county police expenditures. Reg Sci Urban Econ 22(3):317–331. https://doi.org/10.1016/0166-0462(92)90032-V
Kumie, A., Worku, A., Tazu, Z., Tefera, W., Asfaw, A., Boja, G., Mekashu, M., Siraw, D., Teferra, S., Zacharias, K., Patz, J., Samet, J., & Berhane, K. (2021). Fine particulate pollution concentration in Addis Ababa exceeds the WHO guideline value: Results of 3 years of continuous monitoring and health impact assessment. Environmental Epidemiology, 5(3). https://journals.lww.com/environepidem/Fulltext/2021/06000/Fine_particulate_pollution_concentration_in_Addis.10.aspx
Lesage, J. (2004). Lecture 1: Maximum likelihood estimation of spatial regression models
Li X, Feng Y, Liang H (2017) The Impact of Meteorological Factors on PM2.5 Variations in Hong Kong. IOP Conf Ser: Earth Environ Sci 78:12003. https://doi.org/10.1088/1755-1315/78/1/012003
Loftin C, Ward SK (1983) A Spatial Autocorrelation Model of the Effects of Population Density on Fertility. Am Sociol Rev 48(1):121–128. https://doi.org/10.2307/2095150
Manisalidis I, Stavropoulou E, Stavropoulos A, Bezirtzoglou E (2020) Environmental and Health Impacts of Air Pollution: a review. Front Public Health 8:505–570. https://doi.org/10.3389/fpubh.2020.00014
Miller HJ (2004) Tobler’s First Law and Spatial Analysis. Ann Assoc Am Geogr 94(2):284–289 http://www.jstor.org/stable/3693985
Njee R, Meliefste K, Malebo H, Hoek G (2016) Spatial Variability of Ambient Air Pollution Concentration in Dar es Salaam. Int J Environ Res Public Health 4:83–90. https://doi.org/10.12691/jephh-4-4-2
Njee RM, Meliefste K, Malebo HM, Hoek G (2022) Spatial Variability of Ambient Air Pollution Concentration in Dar es Salaam. J Environ Res Public Health 4(4):83–90 http://pubs.sciepub.com/
Okimiji, O. P., Techato, K., Simon, J. N., Tope-Ajayi, O. O., Okafor, A. T., Aborisade, M. A., & Phoungthong, K. (2021). Spatial Pattern of Air Pollutant Concentrations and Their Relationship with Meteorological Parameters in Coastal Slum Settlements of Lagos, Southwestern Nigeria. Atmosphere, 12(11). https://doi.org/10.3390/atmos12111426
Ord K (1975) Estimation Methods for Models of Spatial Interaction. J Am Stat Assoc 70(349):120–126. https://doi.org/10.2307/2285387
Park, S., & Ko, D. (2021). Spatial Regression Modeling Approach for Assessing the Spatial Variation of Air Pollutants. Atmosphere 12(6). https://doi.org/10.3390/atmos12060785
Rahmah S, Rahman AA, Norkhadijah SIS, Ismail SNS, Ramli MF, Latif MT, Abidin EZ, Praveena SM (2015) The assessment of ambient air pollution trend in Klang valley, Malaysia. World Environment 5:1–11
Schober P, Boer C, Schwarte LA (2018) Correlation coefficients: appropriate use and interpretation. Anesthesia & Analgesia 126(5):1763–1768. https://journals.lww.com/anesthesia-analgesia/Fulltext/2018/05000/Correlation_Coefficients__Appropriate_Use_and.50.aspx
Singh A, Gatari MJ, Kidane AW, Alemu ZA, Derrick N, Webster MJ, Bartington SE, Thomas GN, Avis W, Pope FD (2021) Air quality assessment in three East African cities using calibrated low-cost sensors with a focus on road-based hotspots. Environ Res Commun 3(7):75007. https://doi.org/10.1088/2515-7620/ac0e0a
Srinivasan, S. (2015). Spatial Regression Models BT - Encyclopedia of GIS (S. Shekhar, H. Xiong, & X. Zhou (eds.); pp. 1–6). Springer International Publishing. https://doi.org/10.1007/978-3-319-23519-6_1294-2
Suryowati K, Bekti RD, Faradila A (2018) A Comparison of Weights Matrices on Computation of Dengue Spatial Autocorrelation. IOP Conf Ser Mater Sci Eng 335:12052. https://doi.org/10.1088/1757-899x/335/1/012052
Tefera, W., Kumie, A., Berhane, K., Gilliland, F., Lai, A., Sricharoenvech, P., Samet, J., Patz, J., & Schauer, J. J. (2020). Chemical Characterization and Seasonality of Ambient Particles (PM2.5) in the City Centre of Addis Ababa. In Int J Environ Res Public Health 17(19). https://doi.org/10.3390/ijerph17196998
Tobler WR (1970) A Computer Movie Simulating Urban Growth in the Detroit Region. Econ Geogr 46(sup1):234–240. https://doi.org/10.2307/143141
Tobler, W. R. (1979). Cellular Geography BT - Philosophy in Geography (S. Gale & G. Olsson (eds.); pp. 379–386). Springer Netherlands. https://doi.org/10.1007/978-94-009-9394-5_18
Tsegaye D, Leta S, Khan MM (2019) Ambient Air Pollution Status of Addis Ababa City; The Case of Selected Roadside. Am J Environ Prot 8(2):39–47. https://doi.org/10.11648/j.ajep.20190802.11
Whaley , N. M., Burns J, (2021). Update of the WHO global air quality guidelines: systematic reviews. (Vol. ( https://www.sciencedirect.com/journal/environment-international/specialissue/10MTC4W8FXJ, accessed 17 June 2021).)
Woldesemayat, E. M., & Genovese, P. V. (2021). Urban Green Space Composition and Configuration in Functional Land Use Areas in Addis Ababa, Ethiopia, and Their Relationship with Urban Form. In Land (Vol. 10, Issue 1). https://doi.org/10.3390/land10010085
World Health, O. (2020). Personal interventions and risk communication on air pollution: summary report of WHO expert consultation, 12–14 February 2019, Geneva, Switzerland. World Health Organization. https://apps.who.int/iris/handle/10665/333781
Xu, W., Tian, Y., Liu, Y., Zhao, B., Liu, Y., & Zhang, X. (2019). Understanding the Spatial-Temporal Patterns and Influential Factors on Air Quality Index: The Case of North China. Int J Environ Res Public Health 16(16). https://doi.org/10.3390/ijerph16162820
Yousefian F, Faridi S, Azimi F, Aghaei M, Shamsipour M, Yaghmaeian K, Hassanvand MS (2020) Temporal variations of ambient air pollutants and meteorological influences on their concentrations in Tehran during 2012–2017. Sci Rep 10(1):292. https://doi.org/10.1038/s41598-019-56578-6
Zhou, H., Yu, Y., Gu, X., Wu, Y., Wang, M., Yue, H., Gao, J., Lei, R., & Ge, X. (2020). Characteristics of Air Pollution and Their Relationship with Meteorological Parameters: Northern Versus Southern Cities of China. Atmosphere 11(3). https://doi.org/10.3390/atmos11030253
Zwack LM, Paciorek CJ, Spengler JD, Levy JI (2011) Modeling spatial patterns of traffic-related air pollutants in complex urban terrain. Environ Health Perspect 119(6):852–859. https://doi.org/10.1289/ehp.1002519
Funding
This work was supported by Addis Ababa University Thematic Research Fund Grant number VPRTT/PY-092/2021. Therefore, the authors thank the Vice President for Research and Technology Transfer Office, Addis Ababa University, Ethiopia, for the financial support through the University’s thematic research program. Daniel also acknowledges Bonga University for sponsoring his MSc study.
Author information
Authors and Affiliations
Contributions
All the authors involved in this research project and in the revision of the entire manuscript.
Corresponding author
Ethics declarations
Informed consent statement
Not applicable (NA).
Institutional review board statement
Not applicable (NA).
Competing interests
The authors declare no competing interests.
Additional information
Publisher's Note
Springer Nature remains neutral with regard to jurisdictional claims in published maps and institutional affiliations.
Appendix
Rights and permissions
Springer Nature or its licensor (e.g. a society or other partner) holds exclusive rights to this article under a publishing agreement with the author(s) or other rightsholder(s); author self-archiving of the accepted manuscript version of this article is solely governed by the terms of such publishing agreement and applicable law.
About this article
Cite this article
Mulgeta, D., Gotu, B., Temesgen, S. et al. Statistical Analysis of Spatial Distribution of Ambient Air Pollution in Addis Ababa, Ethiopia. Stoch Environ Res Risk Assess (2024). https://doi.org/10.1007/s00477-024-02748-6
Accepted:
Published:
DOI: https://doi.org/10.1007/s00477-024-02748-6