Abstract
Previous research has proposed that the approximate number system (ANS) constitutes a building block for later mathematical abilities. Therefore, numerous studies investigated the relationship between ANS acuity and mathematical performance, but results are inconsistent. Properties of the experimental design have been discussed as a potential explanation of these inconsistencies. In the present study, we investigated the influence of set size and presentation duration on the association between non-symbolic magnitude comparison and math performance. Moreover, we focused on strategies reported as an explanation for these inconsistencies. In particular, we employed a non-symbolic magnitude comparison task and asked participants how they solved the task. We observed that set size was a significant moderator of the relationship between non-symbolic magnitude comparison and math performance, whereas presentation duration of the stimuli did not moderate this relationship. This supports the notion that specific design characteristics contribute to the inconsistent results. Moreover, participants reported different strategies including numerosity-based, visual, counting, calculation-based, and subitizing strategies. Frequencies of these strategies differed between different set sizes and presentation durations. However, we found no specific strategy, which alone predicted arithmetic performance, but when considering the frequency of all reported strategies, arithmetic performance could be predicted. Visual strategies made the largest contribution to this prediction. To conclude, the present findings suggest that different design characteristics contribute to the inconsistent findings regarding the relationship between non-symbolic magnitude comparison and mathematical performance by inducing different strategies and additional processes.
Similar content being viewed by others
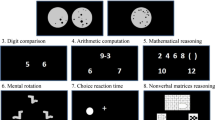
Avoid common mistakes on your manuscript.
Introduction
A dominant view in research on numerical cognition postulates that the foundation of our numerical and arithmetic abilities lies in the evolutionary old approximate number system (ANS; Dehaene, 2001, 2009; Nieder, 2013; Piazza, 2010). The ANS is a cognitive system which is assumed to represent approximately the number of discrete entities in a set (i.e., the numerosity; e.g., Cantlon, Platt, & Brannon, 2009). Due to an overlap between adjacent representations, the representations of numerosities are imprecise, whereby the imprecision of the representation increases the larger the numerosities are (Feigenson, Dehaene, & Spelke, 2004; Lyons, Ansari, & Beilock, 2015; Nieder, Freedman, & Miller, 2002). The overlap between the representations appears to affect behavioral performance of tasks reverting to these representations, like the non-symbolic magnitude comparison task (De Smedt, Noël, Gilmore, & Ansari, 2013; Dehaene, 2009; Halberda, Mazzocco, & Feigenson, 2008). In this task, participants have to judge which of two to-be-compared dots sets is more numerous. Numerous studies have shown that participants’ performance in this task depends on the ratio between the two to-be-compared numerosities (i.e., the ratio effect; e.g., Inglis & Gilmore, 2014; Price, Palmer, Battista, & Ansari, 2012; Soltész, Szucs, & Szucs, 2010). The ratio effect reflects the finding that task performance is better [i.e., higher accuracy, smaller response times (RTs)] when comparing numerosities with a small ratio (e.g., 5 vs. 10 dots; ratio = 0.5) than a large ratio (e.g., 9 vs. 10 dots; ratio = 0.9). This effect is explained by differences in the degree of overlap between the ANS representations, which affect task performance (Cantlon et al., 2009; Nieder, 2011; Nieder et al., 2002). More concretely, the overlap of the representations is larger when the ratio of the to-be-compared numerosities is larger. Moreover, a larger overlap results in a worse performance. Hence, the ratio effect is often referred to as a hallmark of the ANS (e.g., Price et al., 2012) and, moreover, is commonly used as index of the acuity of representations of numerosities (subsequently referred to as ANS representations; for reviews see De Smedt et al., 2013; Dietrich, Huber, & Nuerk, 2015).
ANS representations are thought to be linked to exact number representations. Hence, they are also involved in tasks requiring symbolic math abilities (e.g., Ansari, 2012; Lipton & Spelke, 2005; Noël & Rousselle, 2011; Verguts & Fias, 2004). Evidence for the notion that the ANS underlies symbolic mathematical abilities comes from studies showing a significant relationship between the acuity of the ANS and mathematical performance. In particular, more precise ANS representations were associated with better math performance (Halberda et al., 2008; Libertus, Feigenson, & Halberda, 2011; Mazzocco, Feigenson, & Halberda, 2011). However, there are also numerous studies, which did not find evidence for such a relationship (Mundy & Gilmore, 2009; Soltész et al., 2010; Vanbinst, Ghesquière, & De Smedt, 2012; for a review see De Smedt et al., 2013). These inconsistent results have not been solved yet (Chen & Li, 2014). Nevertheless, these inconsistencies can be partially attributed to differences in measures employed to assess ANS acuity and tasks used to assess mathematical competence as indicated by a recent meta-analysis of Schneider et al. (2016).
Moreover, there are several methodological aspects which can be manipulated in a non-symbolic magnitude comparison task and which differ considerably between studies (for a review see Dietrich et al., 2015a, b). In the following, we will first give an overview of design characteristics influencing the performance in a non-symbolic magnitude comparison task, before we focus on additional cognitive processes or strategies being induced by different aspects of task design and elaborate how these additional processes caused by design characteristics affect the relationship between non-symbolic magnitude comparison and math performance.
Design characteristics affecting performance in a non-symbolic magnitude comparison task
With regard to the construction of the stimuli two aspects are commonly varied: methods to control for visual cues (see e.g., Dietrich et al., 2015a; Gebuis & Reynvoet, 2011) and the concrete number of dots (i.e., the set size, e.g., De Smedt et al., 2013; Dietrich et al., 2015a).
Methods to control for visual cues have been developed to ensure that participants solve the non-symbolic magnitude comparison task based on the numerical magnitude information and not based on visual properties of the stimuli (Piazza et al., 2004; Gebuis & Reynvoet, 2011). Visual properties of the dot sets can be divided into properties of individual items, including dot size (i.e., average diameter of the dots) or sparsity (i.e., average field area—the space within which the dots are drawn—per item), and parameters of the whole set, like total surface area (i.e., sum of surfaces of the individual dots) or convex hull (i.e., smallest area covering all dots e.g., DeWind, Adams, Platt, & Brannon, 2015). As the number of dots is highly related to visual properties of the stimuli, they might affect or even underlie task performance (Gebuis & Reynvoet, 2012; Leibovich & Henik, 2013). To control for this confound, researchers have attempted to match specific visual properties across the to-be-compared sets (e.g., Bartelet, Vaessen, Blomert, & Ansari, 2014; Libertus, Woldorff, & Brannon, 2007) or kept visual properties and numerosity negatively related (Szűcs, Nobes, Devine, Gabriel, & Gebuis, 2013) to ensure that no single visual cue is consistently predictive of numerosity throughout the entire set.
However, several studies found that performance in non-symbolic magnitude comparison tasks depended on the method used to control for visual properties of the dot sets (Clayton, Gilmore, & Inglis, 2015; Smets, Sasanguie, Szücs, & Reynvoet, 2015; Szűcs et al., 2013). It was shown that task performance decreased the more visual parameters were controlled for (Clayton et al., 2015; Smets et al., 2015). Moreover, task performance was worse in incongruent trials (i.e., when visual parameters were negatively correlated with numerosity) than in congruent trials (i.e., when visual parameters were positively correlated with numerosity; Gebuis & Reynvoet, 2012; Gilmore et al., 2013; Szűcs et al., 2013). These findings indicated that the non-symbolic magnitude comparison task does not assess numerosity representations independently from visual cues. Gebuis and Reynvoet (2012) even went a step further and proposed that participants may not extract numerosity information at all. Instead, they suggested performance in the non-symbolic magnitude comparison task to be driven by the integration of multiple visual cues. Moreover, only recently DeWind et al. (2015) developed a model to separate the effect of numerical information and non-numerical, visual information.
Besides visual parameters, it was observed that set size influences task performance (e.g., Clayton & Gilmore, 2015). Clayton and Gilmore (2015) found that task performance declined with increasing set size (i.e., the number of dots in the to-be-compared dot sets). Moreover, set size interacted with the congruency of visual parameters. For example, the congruency effect for convex hull increased as set size increased (Clayton & Gilmore, 2015). Moreover, several studies found that very small dot sets (i.e., up to 3–4) are processed differently than larger numerosities (Cutini, Scatturin, Basso Moro, & Zorzi, 2014; Feigenson et al., 2004; Piazza, 2010; Revkin, Piazza, Izard, Cohen, & Dehaene, 2008). In this range, the number of dots can be recognized very rapidly and accurately; this ability is called subitizing and can be differentiated from the ANS (Feigenson et al., 2004; Kaufman, Lord, Reese, & Volkmann, 1949; Mandler & Shebo, 1982; Piazza, 2010; Revkin et al., 2008; Trick & Pylyshyn, 1994). Furthermore, for very large set sizes, when the dots are arranged too densely, texture-density mechanisms are involved (e.g., Anobile, Turi, Cicchini, & Burr, 2015; Cicchini et al., 2016). Texture-density indicates the number of elements per unit of an area (Durgin, 1995). Evidence for different mechanisms was provided by Anobile, Cicchini, and Burr (2013) who observed that only for low density patterns discrimination performance was in line with ANS theory, whereas for denser stimuli discrimination performance was described better by a model where number is derived as a product of texture-density and area.
While visual controls and set size are aspects to consider when constructing the stimuli, presentation duration is an important aspect when determining the procedure of the task. To prevent participants using counting strategies, the use of short presentation durations has been proposed (e.g., Halberda et al., 2008; Inglis, Attridge, Batchelor, & Gilmore, 2011). However, there is no consensus how short the stimuli have to be presented to rule out counting strategies (see Dietrich et al., 2015a). Moreover, presentation duration of the stimuli varies heavily between studies, ranging from 150 ms (Agrillo, Piffer, & Adriano, 2013; Castronovo & Göbel, 2012) to up to 4000 ms (De Oliveira Ferreira et al., 2012; Lonnemann, Linkersdörfer, Hasselhorn, & Lindberg, 2013). In other studies presentation duration is not restricted; instead the stimuli are presented until participants respond (e.g., Bartelet et al., 2014; Defever, Reynvoet, & Gebuis, 2013). Inglis & Gilmore (2013) showed that presentation duration affected the performance in a non-symbolic magnitude comparison task. More specifically, discrimination performance of participants was more accurate, the longer the presentation duration of the dot sets.
Do design characteristics induce additional cognitive processes or strategies?
Recent studies suggest that depending on the concrete aspects of task design additional cognitive processes are involved in the non-symbolic magnitude comparison task. For example, the congruency between visual properties of the stimuli and numerosity affected the involvement of inhibitory control. More concretely, the processing of incongruent trials, where the information based on numerosity and on visual properties of the stimuli was conflicting, required inhibitory control (Fuhs & McNeil, 2013; Gilmore et al., 2013). Moreover, it was also discussed that depending on the arrangement of the stimuli additional processes might be involved. For example, in case of successive presentation of the to-be-compared dot arrays of an item working memory resources might be required, while in case of an intermixed presentation of dot arrays (e.g., yellow dots within a set of blue dots) spatial resolution processes might be necessary (Price et al., 2012).
Previous research has already shown that participants use strategies flexibly depending on task characteristics in numerosity judgment tasks (Gandini, Lemaire, & Dufau, 2008b; Luwel, Verschaffel, Onghena, & De Corte, 2003a, b). Hence, depending on design characteristics participants might also use different strategies to solve the non-symbolic magnitude comparison task. Although the use of strategies like counting or visual strategies has been discussed frequently in ANS literature, strategies have not been investigated explicitly so far. Thus, in the present experiment we aimed at investigating, which strategies are involved in non-symbolic magnitude comparison and how strategy selection was influenced by the design parameters set size and presentation duration. More concretely, we focused on the use of visual strategies, counting strategies, calculation-based strategies, numerosity-based strategies, and subitizing.
Visual strategies (i.e., considering visual properties of the stimuli in the decision such as density, field area, etc.) were chosen, as it was already argued that the judgments of participants in a non-symbolic magnitude comparison task are rather based on the weighting of multiple visual parameters than on the pure processing of numerosity information (Gebuis & Reynvoet, 2012). Moreover, there are already hints that visual strategies might be influenced by design characteristics. For instance, Clayton and Gilmore (2015) found the congruency effect (for convex hull) to increase the larger the set size was. Congruency effects have been interpreted as indicator for the use of visual strategies (Gebuis & Reynvoet, 2012). Thus, we expected that visual strategies are influenced by set size, whereby a more frequent use of visual strategies was expected for larger set sizes. With regard to influences of presentation duration on the use of visual strategies, it might be argued that visual strategies as rather fast strategies (compared to counting or calculation-based strategies) should be more likely for short presentation durations than for longer presentation duration, where also slower, but more accurate strategies can be used (Luwel & Verschaffel, 2003).
Another strategy, which has been considered in ANS research, involves counting the number of dots in each set (see e.g., Dietrich et al., 2015a, b; Halberda et al., 2008). The use of counting strategies is strongly related to the presentation duration employed in the non-symbolic magnitude comparison task. Restricting the presentation duration to a few 100 ms makes counting strategies impossible. In contrast, presenting the to-be-compared dot sets for several seconds enables to use of counting strategies. Hence, we expected a higher frequency of counting strategies for longer presentation durations. Furthermore, the use of counting strategies could be influenced not only by presentation duration but also by set size, as smaller sets can be counted more easily than larger dot sets making counting strategies more likely to be used in smaller set than in larger sets (Dietrich, Huber, & Nuerk, 2015). Accordingly, there should be an interaction between set size and presentation duration regarding the use of counting strategies. Counting strategies should be most frequent in conditions with small set sizes and long presentation duration.
Research on numerosity estimation indicated that participants also use calculation-based strategies to estimate the number of dots in one set (e.g., Gandini et al., 2008b). For example, the number of dots in a set can be determined by subdividing the dot set into similar subgroups (e.g., there are 4 similar subgroups), estimating the number of dots in one subgroup (e.g., one group consists of 5 dots) and then multiply this number with the number of subgroups (e.g., 5 × 4 = 20 dots; i.e., decomposition strategy). Alternatively, participants may also enumerate a subgroup of dots (e.g., there are 6 dots), estimate the remaining dots based on the numerosity of the subgroup (e.g., there are twice as many dots, thus 12 dots) and add up the respective number of dots (e.g., 6 + 12 = 18 dots; i.e., anchoring strategy; see Gandini et al., 2008b). These strategies reported in the context of numerosity estimation might also be employed in non-symbolic magnitude comparison. Participants might estimate or roughly calculate the number of dots in both sets, respectively or treat one dot set as anchor. With regard to potential influences of set size on the use of calculation-based strategies, research on numerosity estimation has already shown that calculation-based strategies are applied more often for larger numerosities (Gandini et al., 2008b). Hence, we assumed that calculation-based strategies are employed more frequently for large set sizes. Moreover, calculation-based strategies are also more time-consuming strategies and, hence, should benefit from longer presentation duration.
In case participants solve the task using one of these strategies, they may not (need to) rely on their underlying ANS representation. However, in the present study we also considered the possibility that participants relied on the number of elements in the sets, when solving the non-symbolic magnitude comparison task (reflected by numerosity-based strategies). Numerosity-based strategies, which might refer to the involvement of the ANS, have already been described in the context of numerosity estimation, where the task was solved by retrieving the numerosity representation after scanning the stimuli (see benchmark strategy, Gandini, Lemaire, Anton, & Nazarian, 2008a; Gandini et al., 2008b). This kind of strategy was found more often for larger numerosities (Gandini et al., 2008b). Thus, for larger set sizes we expected a higher frequency of numerosity-based strategies. In contrast, strategies referring to the use of subitizing (i.e., grasping the numerosity of a set at first glance) should be reported for numerosities falling in the subitizing range, but should not play a role for larger set sizes. As subitizing is a fast and accurate process, it should not be influenced by presentation duration (Mandler & Shebo, 1982). Finally, we did not only code numerosity-based strategies besides visual, counting, and calculation-based strategies, but also calculated the ratio effect, a commonly used index of ANS acuity (De Smedt et al., 2013; Dietrich, Huber, & Nuerk, 2015).
Design characteristics influencing the relation between non-symbolic magnitude comparison and math performance
The relationship between non-symbolic magnitude comparison and math performance has been studied frequently. However, results are conflicting (Chen & Li, 2014; De Smedt et al., 2013; Fazio, Bailey, Thompson, & Siegler, 2014), which has been attributed to differences across studies with regard to the employed design of the non-symbolic magnitude comparison task (De Smedt et al., 2013) as well as differences in measures of magnitude comparison and math proficiency (Schneider et al., 2016). As outlined above, variations regarding the design characteristics affect not only performance in the non-symbolic magnitude comparison task (and hence estimates of ANS acuity), they can also alter domain-general processes involved in the solution process of the task. Hence, design characteristics may contribute the conflicting findings regarding the relationship between non-symbolic magnitude comparison and math performance (see Clayton & Gilmore, 2015). There is first evidence for a moderating role of design characteristics on this often studied relationship by changing the cognitive processes involved in the non-symbolic magnitude comparison task. Gilmore et al. (2013) found that children’s performance in a calculation test was significantly correlated with their performance in the incongruent trials of a non-symbolic magnitude comparison task, but not with their performance in the congruent trials. When controlling for inhibitory control, the association between the performance in the incongruent trials and calculation skills was no longer significant (see also, Fuhs & McNeil, 2013; but see Keller & Libertus, 2015). These findings suggest that the varying pattern of results for congruent and incongruent trials is caused by the different involvement of inhibitory control, which has been found to be positively related to math abilities (Bull & Scerif, 2001; Espy et al., 2004; St Clair-Thompson & Gathercole, 2006) and is necessary for the solution of the incongruent trials (Camilla Gilmore et al., 2013).
In the present study we additionally investigated how design characteristics can influence the association between non-symbolic magnitude comparison and math performance by changing the processes involved in the solution process of the non-symbolic magnitude comparison task. We thereby expanded previous research by focusing on the strategies participants used to solve the task rather than domain-general processes. As outlined above, the frequency of the strategies employed may vary depending on design characteristics like set size and presentation duration. Hence, the performance in a subgroup of items in the non-symbolic magnitude comparison task may be driven by different strategies (which is similar to the different involvement of inhibitory control in congruent and incongruent trials). Moreover, the strategies reported above involve processes, which have already been found to be related to mathematical performance. First of all, counting strategies or calculation-based strategies themselves represent mathematical abilities. But also visual strategies might be related to mathematical performance, for example via visuospatial abilities, which in turn have been found to be associated with mathematical performance (Assel, Landry, Swank, Smith, & Steelman, 2003; Guay & McDaniel, 1977; Gunderson, Ramirez, Beilock, & Levine, 2012). Thus, strategies might contribute to the inconsistent findings regarding the relationship between non-symbolic magnitude comparison and math performance, as—similar to inhibitory control—they can affect both the performance in a subgroup of items and are related to math performance.
Present study
Taken together, in the present study, we aimed at investigating whether the design characteristics set size and presentation duration influence the association between non-symbolic magnitude comparison and math performance by inducing different solution strategies. To investigate this issue we used a two-step approach. First, we examined whether the design characteristics set size and presentation duration moderate the relationship between non-symbolic magnitude comparison and math performance (i.e., whether the size of the relationship differs depending on the design characteristics used). Second, we focused on the strategies participants reported to solve the non-symbolic magnitude comparison task and how strategy selection was affected by the design characteristics set size and presentation duration. Moreover, we also investigated whether the strategies reported by the participants were also associated with their math performance. In particular, we examined whether the frequency of the reported strategies is related to the performance in an arithmetic task. However, we also considered the currently dominant theory on the relationship between non-symbolic magnitude comparison and math performance, which explains the relationship by the acuity of the ANS representations. To do so, we focused on the ratio effect as a commonly used hallmark of the ANS (e.g., Price et al., 2012).
Method
Participants
Thirty-two adults (21 female, 3 left-handed) participated in the study. They were on average 23.91 years old (SD = 3.63, range = 19–32 years). Informed consent was obtained from all individual participants included in the study. Moreover, all participants received either a financial compensation of 8€ per hour or course credits. The study was approved by the local ethics committee of the Leibniz-Institut für Wissensmedien in Tübingen.
Materials and procedure
Participants completed a non-symbolic magnitude comparison task and an arithmetic task. The order of these two tasks was counterbalanced across participants.
Non-symbolic magnitude comparison task
The non-symbolic magnitude comparison task consisted of four blocks, whereby set size (small versus large) and presentation duration (short vs. long) were systematically varied. All conditions started with a fixation point which was then replaced by two parallel presented dot sets at the x/y coordinates 400/600 and 1200/600 (at a screen resolution of 1600 × 1200 pixels). Fixation cross and dot sets were presented in white against black background. Dot sets differed according to two dimensions, resulting in a 3 (set size: subitizing, small vs. large) × 2 (presentation duration: 150 vs. 4000 ms) within-subjects design. The small set size condition consisted of 40 trials with numerosities ranging from 5 to 15, whereas the large set size condition consisted of 40 items with numerosities ranging from 30 to 70. In each condition five different ratios between to-be-compared dot sets were used (0.5, 0.6, 0.7, 0.8, 0.9) which were distributed equally across all trials. Moreover, we also included ten items with numerosities within the subitizing range (i.e., 1–4 dots), which were presented intermixed with the items of the small set size condition, but were analyzed separately. We included ten items within the subitizing range, because only very few unique combinations of two integers exist at all and presenting items within the subitizing range more than once might have resulted in a learning effect which in turn might have biased effect size estimates.
Each of the four blocks started with five practice trials allowing participants to familiarize with the respective condition. Taken together participants solved 200 items [(4 × 5 practice trials) + (10 subitizing + 40 small set size + 40 large set size) × 2 presentation durations]. The order of the four blocks was counterbalanced across participants. To control for visual properties of the stimuli, the stimuli were created using the Matlab script by Gebuis & Reynvoet (2011). After the presentation of the dot sets a question mark was presented indicating that participants should indicate which of the two presented dot sets was larger by pressing the corresponding left or right response key of a gamepad controller. Following their response participants were asked to report verbally how they solved the task. Participants were not given any prompts or examples of what they could say.
Arithmetic task
To assess participants’ arithmetic performance, we administered the subtest “Rechenzeichen” of the Intelligenz-Struktur-Test 2000R (Amthauer, Brocke, Liepmann, & Beauducel, 2007). In this task, participants have to solve equations by inserting the correct arithmetic operators (i.e., +, −, ·, \(\div\)). For instance, to solve the equation “6 ? 2 ? 3 = 5”, participants have to select the “+” operator for the first calculation step and the “−” operator for the second calculation step. The sum of correctly solved items served as dependent variable.
Coding of strategies
We developed a standardized coding scheme to classify the verbal reports of the participants in the non-symbolic magnitude comparison task. For each item, we registered, whether participant used one of the following solution strategies (coded with 1) or not (coded with 0), whereby multiple responses were possible: (1) numerosity-based strategy, (2) visual strategies, (3) counting strategies, (4) calculation-based strategy, (5) subitizing, or (6) guessing.
Visual strategies were coded whenever participants reported that they considered specific visual properties of the stimuli in their decision. Moreover, it was registered, which visual property of the stimuli was used: size of the individual dots, density of the dot sets, convex hull of the dot sets, and total surface area. Counting strategies were coded whenever participants explicitly stated that they counted the dots. Calculation-based strategies included approximate calculation of the number of the dots, for example using a decomposition strategy or an anchoring strategy. Moreover, numerosity-based strategies were coded, when participants stated that they relied on the number of elements in the sets, when solving the task. We also noted when participants reported to use subitizing, for example, if they mentioned that they grasped already at first glance that a dot set consisted of less than four dots. Furthermore, it was also registered, when participants mentioned that they had simply guessed which set contained more dots (i.e., guessing; for examples of how different verbal reports were coded, see “Appendix”). Verbal reports of all participants were coded by two raters. Cohen’s κ as a measure of interrater reliability ranged from moderate for numerosity-based strategies (κ = 0.50) to almost perfect for visual strategies (κ = 0.94) (Landis & Koch, 1977). Furthermore, all discrepancies were discussed until a consensus was reached. In case, no agreement was reached or the verbal reports of the participants were judged as unclear, the respective item was excluded from the analysis. This affected 0.7% of the items.
We chose to code all strategies reported for each trial, because we cannot be sure whether a particular decision is influenced only by one of the strategies or which one of the reported strategies was the most influential. Moreover, we checked whether the number of strategies reported decreased with the number of trials. Indeed, trial was a significant predictor. However, the slope estimate was very small with a reduction of 0.00044 strategies per trial (over 200 trials 0.089 strategies). Hence, we are confident that the duration of the experiment did not influence our results seriously. Finally, we examined whether talkativeness was a significant predictor of the frequency of strategies. As a measure for talkativeness we used the mean number of words in verbal reports. Then, we ran generalized linear mixed effects models (GLME) with logit as link function and assuming a binomial error distribution for each strategy separately. In the model, talkativeness was entered as a fixed effect and additionally, we included a random intercept for participants as well as items. However, we found that talkativeness was not a significant predictor after correcting for multiple testing (all p > 0.103). Thus, we also did not find evidence for an influence of talkativeness on the frequency of reported strategies.
Analysis
Accuracy
In order to investigate the effect of set size and presentation duration on the association between accuracy in a non-symbolic magnitude comparison task and arithmetic performance, we ran a GLME with logit as link function and assuming a binomial error distribution. We ran a GLME instead of an analysis of variance (ANOVA), as for accuracy data the assumption of homogenous variances of the ANOVA is not met, which can lead to spurious effects (Jaeger, 2008). In our GLME, accuracy was the dependent variable. Furthermore, fixed effects were set size (β1, β2), presentation duration (pres dur, β3), arithmetic performance (β4), ratio between the to-be-compared numerosities (β5), the interaction of set size and presentation duration (β6, β7), the interaction of set size and arithmetic performance (β8, β9), the interaction of presentation duration and arithmetic performance (β10) as well as the interaction of presentation duration, set size and arithmetic performance (β11, β12). We included a random intercept for participants (\(v_{0i}\)) as well as items (\(w_{0j}\)) in order to account for the fact that we included only a sample of participants and items from the population (Baayen, Davidson, & Bates, 2008). Moreover, presentation duration was included as a random slope both for participants (\(v_{1i}\)) and items (\(w_{1j}\)) in order to estimate the effects of presentation duration separately for each participant and item. All categorical variables were effect coded. Ratio and arithmetic performance were centered. Thus, the following GLME was used:
with \(\beta_{0}\) being the intercept, i indicating a specific participant and j a specific item. \({\text{set size}}1\) and \({\text{set size}}2\) were the effect code predictor variables for set size with [1 0] for large set size, [0 1] for small set size, and [−1 −1] for subitizing. \({\text{pres dur}}\) indicated the predictor variable for presentation duration and was coded with 1 for short presentation duration and −1 for long presentation duration. The predictor variable \({\text{ratio}}\) indicated the centered ratio between the two to-be-compared numerosities. Finally, the predictor variable \({\text{arithmetic}}\) indicated the centered scores of the participants in the arithmetic task.
We calculated p values using likelihood ratio tests. GLME were estimated using the R packages lme4 (Bates, Maechler, Bolker, & Walker, 2015) and afex (Singmann, Bolker, & Westfall, 2015). Moreover, we ran post hoc analyses using the R package multcomp (Hothorn, Bretz, & Westfall, 2008). To correct for multiple testing, we adjusted the p values using the Benjamini–Hochberg procedure (Benjamini & Hochberg, 1995).
Response times
Response times (RTs) were defined as the period of time between the end of stimulus presentation and the response. Prior to the analysis of response times (RT) a trimming procedure was conducted. In a first step, we excluded all RTs larger than 10 s resulting in a loss of 0.5% of all RTs. As RTs were strongly skewed to the right, we applied a log-transformation (Ratcliff, 1993) Afterwards, we ran a linear mixed effects model (LME) with log-transformed RT (log RT) as dependent variable and the following fixed effects: set size, presentation duration, arithmetic performance, ratio between the to-be-compared numerosities, the interaction of set size and presentation duration, the interaction of set size and arithmetic performance, the interaction of presentation duration and arithmetic performance as well as the interaction of set size, presentation duration, and arithmetic performance. Moreover, we included a random intercept for participants and items as well as set size, presentation duration and the interaction between set size and presentation duration as a random slope for participants and presentation duration as a random slope for items (i.e., we used the maximal random effects structure, see Barr, Levy, Scheepers, & Tily, 2013). Again all categorical variables were effect coded and the ratio as well as the arithmetic performance was centred. Thus, the following LME was used:
Based on the results of the LME we calculated z standardized residuals for each log RT and excluded log RTs with absolute z standardized residuals deviating more than 3 SD (see Baayen & Milin, 2010 for a similar procedure). This affected again 0.5% of the data. After removing these outliers, we ran the LME again. We calculated p values using Satterthwaite’s approximation for degrees of freedom available via the R package lmerTest (Kuznetsova, Brockhoff, & Christensen, 2015). Again, post hoc tests were calculated using the R package multcomp (Hothorn et al., 2008).
Frequencies of strategies
As for accuracy data, we conducted a GLME for the frequency of reported strategies. We coded whether participants reported a specific strategy or not (1 = strategy reported; 0 = strategy not reported). These binary variables served as dependent variables in the GLMEs. For the three most frequently reported strategies (i.e., numerosity-based strategies, visual strategies, and counting strategies), we included the following fixed effects: set size, presentation duration, arithmetic performance, as well as all possible two-way and three-way interactions. Moreover, we included a random intercept for participants as well as items. Again, we included presentation duration as a random slope both for participants and items. Additionally, all categorical variables were effect coded and the covariate arithmetic performance was centred. Consequently, the following GLME resulted:
For the other two strategies (calculation-based strategies and subitizing) we could not estimate the model including all fixed effects, as these strategies were reported rather rare. Therefore, we only included set size, presentation duration, and the interaction between set size and presentation duration as fixed effects. The random effects were identical to the above model:
Results
Descriptive statistics of task performance (accuracy and RT) for the six conditions of the non-symbolic magnitude comparison task are given in Table 1. Moreover, the average score in the arithmetic test was 14 (SD = 4) ranging from 3 to 20.
In the following results sections, we chose to report higher level interactions first before presenting main effects, as most of our interactions were disordinal which limits the interpretability of main effects.
Accuracy
The results of the GLME for accuracy are given in Table 2. First, we replicated the ratio effect: accuracy decreased with increasing ratio. Moreover, we observed a significant interaction between set size and presentation duration which is depicted in Fig. 1a.
To analyse the interaction between set size and presentation duration, we tested first, whether the effect of presentation duration was present in all set size conditions. Post-hoc tests revealed that accuracy was significantly better for longer presentation durations than for shorter presentation durations in all set size conditions (all p < 0.015).
Next, we investigated whether accuracy of set size conditions differed in the shorter and the longer presentation condition. For the shorter presentation duration, we found that accuracies did not differ significantly between the set size conditions (all p > 0.523). In contrast, for the longer presentation duration we observed that only the conditions large set size and subitizing differed significantly (z = 2.52, p = 0.033). The other two pairwise comparisons were not significant (all p > 0.075). Thus, there was an effect of set size only for the longer presentation duration and therefore, the main effect of set size should not be interpreted.
The significant interaction between set size and arithmetic indicated that the relationship between accuracy in the non-symbolic magnitude comparison task and arithmetic performance differed depending on set size. The estimated slope (as indicator of this relationship) was largest in the condition large set size, followed by the estimated slope in the small set size condition and the slope for the subitizing condition (see Table 2). Note that a positive slope is associated with a positive relationship between accuracy in the non-symbolic magnitude comparison task and arithmetic performance. More specifically, participants with a better arithmetic performance also performed more accurately in the non-symbolic magnitude comparison task (see Fig. 2). The estimated slope for the large set size condition differed significantly only from the slope for the small set size condition (z = 3.89, p < 0.001). All other pairwise comparisons were not significant (p > 0.214).
Furthermore, we observed a significant interaction between presentation duration and arithmetic. The interaction indicated that estimated slopes differed significantly between the presentation durations. However, when testing whether estimated slopes for the shorter and the longer presentation duration were different from zero, we observed that neither of them differed significantly from zero (150 ms: z = 1.87, p = 0.118; 4000 ms: z = −1.18, p = 0.420). Thus, although we observed that estimated slopes differed significantly between the presentation durations, the relationship between accuracy in the non-symbolic magnitude comparison task and arithmetic performance was significant in neither of the two presentation duration conditions.
Response times
The results of the LME for log RT are given in Table 3. Again, we found a significant main effect of ratio, whereby log RTs increased with ratio (log RT = 0.14, SE = 0.01). Similar to the results for accuracy, we observed a significant interaction between set size and presentation duration which is depicted in Fig. 1b. To analyze the interaction, we again tested first, whether the effect of presentation duration was present in all set size conditions. Post-hoc tests revealed that log RT were faster for longer presentation durations than for shorter presentation durations in all set size conditions (all p < 0.001). Next, we investigated whether log RT of set size conditions differed in the shorter and the longer presentation condition. For the shorter presentation duration, we found that log RT did not differ significantly between the set size conditions (all p > 0.318). In contrast, for the longer presentation duration we observed that log RT in the small set size condition differed significantly from the other two conditions (both p < 0.001), whereas the subitizing set size condition did not differ significantly from the large set size condition (z = 1.09, p = 0.849). Thus, there was an effect of set size again only for the longer presentation duration and therefore, the main effect of set size should not be interpreted. No other interactions were significant.
Strategies
Two participants had to be excluded from the strategy analyses, because their responses were not recorded due to technical errors. Descriptive statistics regarding the frequency of the strategies reported by the participants are given in Table 4. Visual strategies were reported most frequently, followed by numerosity-based strategies and counting strategies. In contrast calculation-based strategies and subitizing were rather rare. Importantly, the relative frequencies added up to more than 100% indicating that participants reported (on average) more than one strategy per trial. Moreover, there were large individual differences regarding the strategies reported, as reflected by the minimum and the maximum of the frequencies. In particular, there were participants relying on visual strategies in almost every trial (in 94% of the reported strategies), whereas others mentioned visual strategies in only about one-third of the trials. Moreover, while some participants relied on numerosity-based strategies in about half of the trials, others almost never reported this kind of strategy. Similarly, some participants never reported counting or calculation-based strategies, whereas others used these strategies repeatedly. With regard to visual strategies, participants reported to rely on different visual properties of the stimuli, including dot size (mean frequency = 68%), density (mean frequency = 46%), convex hull (mean frequency = 59%), and total surface area (mean frequency = 6%). Thus, when participants used visual strategies, they on average considered 1.80 visual properties.
In a next step, we investigated separately for each of the reported strategies, whether the frequency of the respective strategies depended on set size and presentation duration and, moreover, whether the frequency of the respective strategies was influenced by participants’ arithmetic performance. The results of the GLMEs are given in Tables 5 and 6.
For the frequency of numerosity-based strategies, we observed a significant interaction between set size and presentation duration, which is illustrated in Fig. 3a. The interaction indicated that for items in the subitizing range, participants reported numerosity-based strategies more often for the short presentation duration condition than for the long presentation condition. However, in the small set size condition, reported frequencies were similar for both presentation duration conditions. In contrast, in the large set size condition, participants reported less numerosity-based strategies in the short presentation duration than in the long presentation duration. As can be seen in Fig. 3a, the main effect of set size should not be interpreted, as there was no consistent pattern. Nevertheless, these results contradicted our expectations that numerosity-based strategies are reported more frequently in settings with large set sizes.
In line with our assumptions we found a significant interaction between set size and presentation duration for the frequency of reported visual strategies (see Fig. 3b. In general, participants reported visual strategies more often in the short presentation duration condition than in the long presentation duration condition. However, the effect was largest in the small set size condition, followed by the subitizing condition, and smallest in the large set size condition. Moreover, there was a significant main effect of set size indicating that visual strategies were reported more frequently in the large set size condition than in the small set size condition or in the subitizing condition (all pairwise comparisons p < 0.001). The main effect of presentation duration indicated that visual strategies were reported significantly more frequently in the shorter presentation duration condition than in the longer presentation durations.
Moreover, in accordance with our hypotheses a significant interaction between set size and presentation duration on the frequency of reported counting strategies was observed (Fig. 3c). For the subitizing condition and the small set size condition a similar pattern was found: counting strategies were significantly more often reported in the long presentation duration condition than in the short presentation duration condition, whereby the effect was larger for the small set size condition than for the subitizing condition. For the large set size condition, almost no counting strategies were reported. The main effect of set size should not be interpreted, as it was only present in the short presentation duration condition. However, the main effect of presentation duration was consistent: counting strategies were reported significantly more frequently in the long presentation duration condition than in the short presentation duration condition.
For calculation-based strategies, we observed a significant main effect of set size. However, the direction of the effect contradicted our assumption based on previous findings regarding strategy selection in numerosity estimation: calculation-based strategies were reported most often in the small set size condition, followed by the subitizing condition and the large set size condition. However, only the frequency of the reported calculation-based strategies in the small and the large set size condition differed significantly (p < 0.001). In line with our predictions, we found a main effect of presentation duration indicating that calculation-based strategies were reported significantly more frequently in the long presentation duration condition than in the short presentation duration condition.
As expected, subitizing strategies were reported most frequently in the subitizing condition, followed by the small set size condition. In the large set size condition, subitizing strategies were not mentioned a single time and hence, log odds and SE could not be estimated accurately. Thus, only the conditions subitizing and small set size differed significantly regarding the frequency of subitizing strategies (p < 0.001).
For none of the strategies reported, we found a link between the frequency of reported strategies and arithmetic performance. In other words, we found no strategy which predicted arithmetic performance alone. However, it might be possible that using a combination of reported strategies would predict arithmetic performance. To investigate this issue, we ran a multivariate analysis based on a support vector machine (SVM) for classifying arithmetic performance using the frequencies of all reported strategies as features (including numerosity-based strategies, visual strategies, counting strategies, calculation-based strategies, and subitizing). To do so, we categorized the arithmetic performance of participants into “good” and “poor” using a median split (Mdn = 14). For running the SVM, we used the software Rapidminer and the library LIBSVM (Chang & Lin, 2011). Moreover, we used a linear kernel to be able to interpret the feature weights regarding their importance (e.g., van den Berg, Reinders, de Ridder, & de Beer, 2015). We optimized the performance of the SVM, by varying the complexity constant using the values 0.003, 0.03, 0.3, 3, 30, 300, 3000, and 30,000. The performance of the SVM was assessed using tenfold cross-validation (James, Witten, Hastie, & Tibshirani, 2013). The best model with a complexity constant of 300 classified 73.33% of the participants correctly. A binomial test revealed that this classification performance was significantly above chance level, p = 0.016. Moreover, our analysis revealed the following feature weights: 491 for numerosity-based strategies, 1654 for visual strategies, 464 for counting strategies, 87 for calculation-based strategies, and 172 for subitizing. As high weights indicate a strong contribution of the respecting feature to the resulting classification, our results suggested that the frequency of visual strategies played the most important role in predicting arithmetic performance. Moreover, the positive sign of the weight indicated that a high frequency of visual strategies was predictive for good arithmetic performance.
Discussion
Recent studies suggested that inconsistent findings regarding the relationship between ANS acuity and mathematical performance can be attributed to aspects of task design. However, the moderating role of such design characteristics has not been investigated systematically so far. Therefore, in the present study, we examined the influence of two often varied design characteristics (i.e., set size and presentation duration) on the relationship between non-symbolic magnitude comparison and arithmetic performance. Moreover, we were interested in how strategy selection was affected by set size and presentation duration and whether the frequency of the reported strategies was associated with math performance.
In line with previous studies, we observed that both set size and presentation duration affected the performance in a non-symbolic magnitude comparison task. In particular, task performance was better (i.e., more accurate and faster) for smaller dot sets and longer presentation durations than for larger dot sets and shorter presentation durations (Clayton & Gilmore, 2015; Inglis & Gilmore, 2013). However, more importantly, our results revealed that set size was indeed a moderator of the relationship between ANS acuity (i.e., accuracy in the non-symbolic magnitude comparison task) and math performance: The relationship was significantly larger for the large set size condition (i.e., 30–70 dots) than for the small set size condition (i.e., 5–15 dots).
In order to get a better understanding of the processes involved in the solution of a non-symbolic magnitude comparison task and to get insights in the mechanisms underlying the moderating effect of set size, we investigated the strategies participants reported to solve the task. Participants reported numerosity-based strategies, visual strategies, counting, calculation-based strategies, subitizing and guessing, whereby visual strategies were reported most often. Visual strategies included different visual properties of the stimuli (i.e., dot size, sparsity, convex hull, or total area) as cues or information guiding the judgments. Interestingly, participants considered on average more than one visual property. Moreover, participants did not rely on only one strategy per trial. Furthermore, there were large individual differences regarding the frequency of the reported strategies. As expected, the frequency of the reported strategies depended on design characteristics. Set size influenced the frequency of all reported strategies, whereas presentation duration affected the frequency of visual strategies, counting, and calculation-based strategies.
Taken together, we observed that numerosity-based strategies were reported most often for small set sizes and least often for set sizes within the subitizing range in the long presentation duration. Moreover, participants reported visual strategies most often for large set sizes independent of presentation duration as well as for small set sizes for short presentation duration, and least often for set sizes within the subitizing range in the long presentation duration. Counting strategies were most often reported in the long presentation duration for set sizes within the subitizing range as well as for small set sizes, whereas they were virtually absent for small set sizes in the short presentation duration condition as well as for large set sizes independent of presentation duration. Calculation-based strategies were reported most often for small set sizes, followed by set sizes within the subitizing range and large set sizes. Moreover, they were reported more frequently in the long presentation duration condition than in the short presentation duration condition. Finally, subitizing strategies were reported most frequently for set sizes within the subitizing range, followed by small set sizes. For large set sizes, subitizing strategies were not mentioned a single time.
Moreover, we were especially interested whether specific strategies also contributed to the relationship between non-symbolic magnitude comparison and arithmetic performance and, whether specific strategies might explain the moderating effect of set size. We observed that in the large set size condition (i.e., in the condition in which the relationship between non-symbolic magnitude comparison and arithmetic was significantly larger) visual strategies were reported predominantly. However, we did not find a significant relation between the frequency of reported visual strategies and arithmetic performance. Instead, arithmetic performance could be predicted when considering a combination of all reported strategies in a SVM. Nevertheless, our analysis revealed that visual strategies made the largest contribution to the classification of participants’ math performance. In the following, we will discuss implications of the present results for research on the relationship of ANS acuity and arithmetic performance. Moreover, we will elaborate on the validity of the non-symbolic magnitude comparison task in assessing ANS acuity.
Moderators of the relationship between non-symbolic magnitude comparison and arithmetic performance
Numerous studies have investigated the relationship between ANS acuity—measured by a non-symbolic magnitude comparison task—and mathematical skills (Bartelet et al., 2014; Brankaer, Ghesquière, & De Smedt, 2014; Gilmore, Attridge, De Smedt, & Inglis, 2014; Gilmore, Attridge, & Inglis, 2011; Halberda et al., 2008; Inglis et al., 2011; Kolkman, Kroesbergen, & Leseman, 2013; Libertus et al., 2011; Lindskog, Winman, Juslin, & Poom, 2013; Price et al., 2012; van Marle, Chu, Li, & Geary, 2014). However, the results are conflicting with each other (Chen & Li, 2014; De Smedt et al., 2013; Dietrich, Huber, & Nuerk, 2015; Fazio et al., 2014). Nevertheless, research on potential moderators of the relationship between ANS acuity and math performance is rather rare. Three recent meta-analyses provided first hints regarding potential moderators, including the index used to assess ANS acuity (Chen & Li, 2014) or the age of the participants (Fazio et al., 2014; Schneider et al., 2016). Additionally, several authors proposed that aspects of task design might be responsible for inconsistencies in empirical findings (Clayton & Gilmore, 2015; De Smedt et al., 2013). In line with this suggestion, two studies indicated that the relationship between non-symbolic magnitude comparison and mathematical performance depended on the congruency between visual properties of the stimuli and numerosity (Fuhs & McNeil, 2013; Camilla Gilmore et al., 2013). This pattern of results was explained by the involvement of inhibitory control in the processing of incongruent trials, as in incongruent trials the information based on visual properties and on the numerosity of the stimuli was conflicting (Fuhs & McNeil, 2013; Camilla Gilmore et al., 2013).
The present findings support the notion that aspects of task design influence the relationship between non-symbolic magnitude comparison and arithmetic, as we identified set size as a moderator of this relationship. In particular, this relationship was more pronounced for larger set sizes than for smaller set sizes. Previous studies differed considerably regarding the set size employed. Some studies used small numerosities in the range of 1–9 dots (Brankaer et al., 2014) and, thus also included the subitizing range. In contrast, others used substantially larger numerosities in the range of 30–100 dots (Guillaume, Nys, Mussolin, & Content, 2013). Hence, our result indicate that set size might—in combination with other factors like the index used to assess ANS acuity, age group, or congruence of the visual properties—contribute to the inconsistencies of the results regarding the relationship between ANS acuity and math performance. In contrast, presentation duration did not influence the relationship between non-symbolic magnitude comparison and arithmetic performance.
Having identified set size as moderator of this relationship leads to the question which processes involved in the solution of a non-symbolic magnitude comparison task might drive this moderating effect. We considered several strategies which might contribute to the varying strength of the relationship between non-symbolic magnitude comparison and arithmetic performance. Importantly, the reported frequency of all considered strategies was influenced by set size. We observed that participants reported primarily visual strategies in trials with larger set size, whereas in the small set size condition also other strategies were applied. In particular, in about 50% of the trials in the small set size condition participants reported counting strategies. Previous research has demonstrated a link between visual-spatial abilities and mathematical abilities (Assel et al., 2003; Guay & McDaniel, 1977; Gunderson et al., 2012; Kurdek & Sinclair, 2001; Mazzocco & Myers, 2003; Reuhkala, 2001). Hence, participants with better visual-spatial abilities might also perform better in the large set size condition, which might explain the observed correlation between non-symbolic magnitude comparison and math performance. Future research is needed to investigate the role of specific visuospatial abilities as potential moderators of the relationship between non-symbolic magnitude comparison and arithmetic. It remains to be clarified which visuospatial abilities are involved in the solution process and how visuospatial abilities are linked to strategy selection and task performance.
In contrast, in the small set size condition in a large percentage of the trials counting abilities were measured. It can be assumed that students are able to count up to 15 (i.e., the maximal number of dots in a set of the small set size condition). Accordingly, this should reduce the variance of participants’ performance in the non-symbolic magnitude comparison task and thereby, also a potential correlation between non-symbolic magnitude comparison and math performance. Thus, the moderator effect of set size might be caused by the differential use of strategies depending on set size and the involvement of additional processes like visual-spatial abilities or counting.
Validity of the non-symbolic magnitude comparison task in assessing ANS acuity
Recently, increasing research interest was paid to the validity of the non-symbolic magnitude comparison task as a measure of ANS acuity. In this context, studies focused especially on non-numerical processes involved in the solution of a non-symbolic magnitude comparison task. Results revealed the involvement of additional processes, like inhibitory control or relying on visual properties of the stimuli instead of numerosity information (Clayton & Gilmore, 2015; Fuhs & McNeil, 2013; Gebuis & Reynvoet, 2012; Szűcs et al., 2013). In line with previous suggestions that participants’ weight visual properties when solving the task (Gebuis & Reynvoet, 2012), the verbal reports collected in the present study revealed a major involvement of visual strategies in solving non-symbolic magnitude comparison tasks. Moreover, our results indicated that participants referred to more than one visual property when making their judgments. This finding supports the claim of Gebuis & Reynvoet (2012) that participants integrate the information from multiple visual properties. Moreover, the findings of the present study suggested that strategies reported in the context of numerosity estimation such as calculation-based strategies or counting (Gandini et al., 2008b), were also applied in non-symbolic magnitude comparison. In sum, previous and present results suggest the involvement of other processes or strategies involving, for instance, visual cues or counting, in non-symbolic magnitude comparison and thus challenge the validity of the non-symbolic magnitude comparison task as a measure of ANS acuity.
Nevertheless, we observed a ratio effect for numerosity in the present study, which is commonly taken as hallmark of the involvement of the ANS (e.g., Price et al., 2012). Moreover, participants also reported numerosity-based strategies in solving the task. These findings support the notion that the task indeed measures ANS representation, at least in a part of the trials. However, when calculating the ratio effect only for the trials in which participants reported to having used only visual strategies, the ratio effect remained significant (p < 0.001). This finding questions the validity of the ratio effect as a measure of the underlying representation (see also Lyons, Nuerk, & Ansari, 2015). Moreover, the question remains why a (numerical) ratio effect is observed, when participants rely solely on visual cues. One possible explanation might be that participants rely on visual cues only in case of congruent trials, where a ratio effect might be also expected for visual cues. To examine this question, we calculated the number of congruent and incongruent trials when participants reported visual cues. However, the number of congruent and incongruent trials was quite similar and hence, cannot explain the ratio effect.
Another possibility might be that participants integrate different visual cues when comparing the numerosity of two dot sets. Evidence for this suggestion comes from the finding that participants considered more than one visual cue. As shown by DeWind et al. (2015), the numerosity of dots can be calculated and, hence, estimated by participants, based on visual properties of the two dots sets: the log of the numerosity of a given dot set is equal to the log of the total surface area divided by the item surface area, or the field area divided by the sparsity.
Of course, participants are probably not aware of this equation. Nevertheless, they might intuitively understand this relationship and draw inferences based on a specific constellation of visual cues. We have found evidence for this suggestion in the present experiment. For example, a participant reported the following strategy: “The dots on the left side were smaller and denser than on the right side, but the area was approximately the same. That is why there have to be more dots on the left side.” Thus, they might indirectly calculate the numerical ratio between the two dot sets based on visual cues, which in turn would explain why a ratio effect is observed, even when participants rely on visual cues. Hence, a significant ratio effect for numerosity might not necessarily indicate that participants relied on the numerosity of the dots. Instead, they might integrate different visual cues to draw conclusions about the numerosity of a dot set and use this information for comparing the dot sets. Taken together, in line with previous findings the present results indicate that several processes and strategies are involved in the solution of a non-symbolic magnitude comparison task. Hence, this task cannot be assumed to assess ANS acuity exclusively and, hence, should not be taken as pure ANS task (see also Szűcs et al., 2013). However, it cannot be ruled out that the task also assesses numerosity-related processes like the acuity of the underlying ANS representations. Future research is needed to unravel the interplay of numerical and non-numerical processes and strategies involved in non-symbolic magnitude comparison and develop possibilities to quantify them.
Methodological constraints
In the present study, participants had to report immediately after each trial how they solved it. Verbal reports have often been used to get insights in the strategies participants use to solve numerical or arithmetic tasks (Gandini et al., 2008; Kirk & Ashcraft, 2001; Robinson, 2001; Seyler, Kirk, & Ashcraft, 2003; Smith-Chant & LeFevre, 2003). Nevertheless, the validity of verbal reports has been discussed regarding two main issues: veridicality and reactivity (Crutcher, 1994; Kirk & Ashcraft, 2001; Seyler et al., 2003).
First, veridicality refers to the issue whether verbal reports reflect the underlying cognitive processes accurately. Processes that rely on short-term memory—like counting or calculation-based strategies—can be reported validly. In contrast, processes that are automatic and, hence, not easily accessible are difficult to be transferred into a verbal report (Kirk & Ashcraft, 2001; Seyler et al., 2003). This might have affected the report of numerosity-based strategies, as the ANS is assumed to be an automatic and intuitive process (Nieder & Dehaene, 2009), which might not be accessible to participants. However, participants reported numerosity-based strategies in 20% of the strategies. Nevertheless, it might be possible that this value underestimates the frequency of numerosity-based strategies in a regular task setting.
Similarly, it might be argued that visual strategies are rather automatic as well. However, these strategies were reported very frequently. On the one hand this can either be interpreted as evidence that automatic strategies are not reported less frequently per se, or on the other hand, that visual strategies are not that automatic. Evidence for the latter argument comes from our results that participants often considered several visual properties and their relation, which is an integration process and, hence, short-term memory might be involved in some way. Another critical issue is that in case two processes occur concurrently, the slower process is reported more frequently than the faster process (Kirk & Ashcraft, 2001; Seyler et al., 2003). However, the present findings contradict this argument, as visual and numerosity-based strategies—which are rather fast—have been reported more often than counting or calculation-based strategies.
Second, reactivity relates to the possibility that mental processes or strategies might differ between settings with and without verbal reports. In a setting requiring verbal reports participants might prefer accuracy over speed (Russo, Johnson, & Stephens, 1989). This might have been the case in the present study, as the mean RT in our experiment was rather slow compared to other studies (e.g., Dietrich, Huber, Moeller, & Klein, 2015a; Halberda, Ly, Wilmer, Naiman, & Germine, 2012; Price et al., 2012). However, recently, we provided evidence that experiments, in which participants prefer accuracy over speed, seem to be better suited at measuring ANS representations (Dietrich et al., 2016). Moreover, the present study as well as many previous studies showed that the performance in a non-symbolic magnitude comparison task depends on certain aspects of task design, which makes it difficult to directly compare task performance across different studies (e.g., Inglis & Gilmore, 2014). Moreover, also for the present setting we replicated previous findings, including the main effect of set size (Clayton & Gilmore, 2015), the main effect of presentation duration (Inglis & Gilmore, 2013), and the ratio effect (Bartelet et al., 2014; Gilmore et al., 2011; Halberda et al., 2008; Soltész et al., 2010). Thus, the results of the present study and hence, also the strategies applied should not be considerably different from studies investigating ANS representations.
Conclusion
The present study extends previous research on moderators of the often reported relationship between non-symbolic magnitude comparison and mathematic abilities. We observed that the design parameter set size moderated this relationship: The association was higher for larger set sizes (here: 30–70 dots) than for smaller set sizes (5–15 dots). This moderating effect of set size might be due to the differential use of strategies depending on set size and related processes like visual-spatial abilities or counting. This finding supports the notion that different design characteristics of the non-symbolic magnitude comparison task contribute to the inconsistent findings regarding the relationship between non-symbolic magnitude comparison and mathematical performance by inducing different strategies and additional processes (see e.g., De Smedt et al., 2013; Feigenson, Libertus, & Halberda, 2013).
Furthermore, our results revealed several strategies in the solution process of non-symbolic magnitude comparison task including numerosity-based strategies, which might reflect ANS like processing. However, also other strategies were reported including visual strategies, counting strategies, calculation-based strategies, and subitizing. This questions the assumption that the non-symbolic magnitude comparison task measures ANS acuity purely. In particular, visual strategies were reported most frequently, whereby participants often reported to rely on more than one visual parameter. These findings are in line with the notion that participants integrate multiple visual parameters when solving the task (see Gebuis & Reynvoet, 2012). Moreover, participants reported on average more than a single strategy per trial. Hence, the present results challenge the validity of the non-symbolic magnitude comparison task in assessing ANS acuity.
Regarding the relationship between the frequency of reported strategies and arithmetic performance, we found that it was not possible to predict arithmetic performance based on a single strategy. However, when considering all reported strategies, arithmetic performance could be predicted. Thus, it seems that not the application of a strategy per se, but the individual composition of strategies seems to be indicative of arithmetic performance and contribute to the relationship between non-symbolic magnitude comparison and arithmetic.
References
Agrillo, C., Piffer, L., & Adriano, A. (2013). Individual differences in non-symbolic numerical abilities predict mathematical achievements but contradict ATOM. Behavioral and Brain Functions, 9(1), 26.
Amthauer, R., Brocke, B., Liepmann, D., & Beauducel, A. (2007). I-S-T 2000 R: Intelligenz-Struktur-Test 2000 R. Göttingen: Hogrefe.
Anobile, G., Cicchini, G. M., & Burr, D. C. (2013). Separate mechanisms for perception of numerosity and density. Psychological Science, 25(1), 265–270. doi:10.1177/0956797613501520.
Anobile, G., Turi, M., Cicchini, G., & Burr, D. (2015). Mechanisms for perception of numerosity or texture-density are governed by crowding-like effects. Journal of Vision, 15, 4.
Ansari, D. (2012). Why the “symbol-grounding problem” for number symbols is still problematic. Current Anthropology, 53(2), 212–213. doi:10.1086/664818.
Assel, M. A., Landry, S. H., Swank, P., Smith, K. E., & Steelman, L. M. (2003). Precursors to mathematical skills: Examining the roles of visual-spatial skills, executive processes, and parenting factors. Applied Developmental Science, 7(1), 27–38. doi:10.1207/S1532480XADS0701_3.
Baayen, R. H., Davidson, D. J., & Bates, D. M. (2008). Mixed-effects modeling with crossed random effects for subjects and items. Journal of Memory and Language, 59(4), 390–412. doi:10.1016/j.jml.2007.12.005.
Baayen, R. H., & Milin, P. (2010). Analyzing reaction times. International Journal of Psychological Research, 3(2), 12–28.
Barr, D. J., Levy, R., Scheepers, C., & Tily, H. J. (2013). Random effects structure for confirmatory hypothesis testing: Keep it maximal. Journal of Memory and Language, 68(3), 255–278. doi:10.1016/j.jml.2012.11.001.
Bartelet, D., Vaessen, A., Blomert, L., & Ansari, D. (2014). What basic number processing measures in kindergarten explain unique variability in first-grade arithmetic proficiency? Journal of Experimental Child Psychology, 117(1), 12–28. doi:10.1016/j.jecp.2013.08.010.
Bates, D., Maechler, M., Bolker, B., & Walker, S. (2015). Fitting linear mixed-effects models unsing lme4. Journal of Statistical Software, 67(1), 1–48. doi:10.18637/jss.v067.i01.
Benjamini, Y., & Hochberg, Y. (1995). Controlling the false discovery rate: A practical and powerful approach to multiple testing. Journal of the Royal Statistical Society. Series B (Methodological), 57(1), 289–300. Retrieved from http://www.jstor.org/stable/2346101.
Brankaer, C., Ghesquière, P., & De Smedt, B. (2014). Children’s mapping between non-symbolic and symbolic numerical magnitudes and its association with timed and untimed tests of mathematics achievement. PLoS One, 9(4), e93565. doi:10.1371/journal.pone.0093565.
Bull, R., & Scerif, G. (2001). Executive functioning as a predictor of children’s mathematics ability: Inhibition, switching, and working memory. Developmental Neuropsychology, 19(3), 273–293.
Cantlon, J. F., Platt, M. L., & Brannon, E. M. (2009). Beyond the number domain. Trends in Cognitive Sciences, 13(2), 83–91. doi:10.1016/j.tics.2008.11.007.
Castronovo, J., & Göbel, S. M. (2012). Impact of high mathematics education on the number sense. PLoS One, 7(4), e33832. doi:10.1371/journal.pone.0033832.
Chang, C.-C., & Lin, C.-J. (2011). LIBSVM: A library for support vector machines. ACM Transactions on Intelligent Systems and Technology, 2(3), 1–27. doi:10.1145/1961189.1961199.
Chen, Q., & Li, J. (2014). Association between individual differences in non-symbolic number acuity and math performance: A meta-analysis. Acta Psychologica, 148, 163–172. doi:10.1016/j.actpsy.2014.01.016.
Cicchini, G. M., Anobile, G., Burr, D. C., Agrillo, C., Bisazza, A., Izard, V., & Tibber, M. S. (2016). Spontaneous perception of numerosity in humans. Nature Communications, 7, 12536. doi:10.1038/ncomms12536.
Clayton, S., & Gilmore, C. (2015). Inhibition in dot comparison tasks. ZDM, 47(5), 759–770. doi:10.1007/s11858-014-0655-2.
Clayton, S., Gilmore, C., & Inglis, M. (2015). Dot comparison stimuli are not all alike: The effect of different visual controls on ANS measurement. Acta Psychologica, 161, 177–184.
Crutcher, R. J. (1994). Telling what we know: The use of verbal report methodologies in psychological research. Psychological Science, 5(5), 241. doi:10.1111/j.1467-9280.1994.tb00619.x.
Cutini, S., Scatturin, P., Basso Moro, S., & Zorzi, M. (2014). Are the neural correlates of subitizing and estimation dissociable? An fNIRS investigation. Neuroimage, 85, 391–399. doi:10.1016/j.neuroimage.2013.08.027.
De Oliveira Ferreira, F., Wood, G., Pinheiro-Chagas, P., Lonnemann, J., Krinzinger, H., Willmes, K., & Haase, V. G. (2012). Explaining school mathematics performance from symbolic and nonsymbolic magnitude processing: Similarities and differences between typical and low-achieving children. Psychology and Neuroscience, 5(1), 37–46.
De Smedt, B., Noël, M.-P., Gilmore, C., & Ansari, D. (2013). How do symbolic and non-symbolic numerical magnitude processing skills relate to individual differences in children’s mathematical skills? A review of evidence from brain and behavior. Trends in Neuroscience and Education, 2(2), 48–55. doi:10.1016/j.tine.2013.06.001.
Defever, E., Reynvoet, B., & Gebuis, T. (2013). Task- and age-dependent effects of visual stimulus properties on children’s explicit numerosity judgments. Journal of Experimental Child Psychology, 116(2), 216–233. doi:10.1016/j.jecp.2013.04.006.
Dehaene, S. (2001). Precis of the number sense. Mind and Language, 16(1), 16–36. doi:10.1111/1468-0017.00154.
Dehaene, S. (2009). Origins of mathematical intuitions. Annals of the New York Academy of Sciences, 1156(1), 232–259. doi:10.1111/j.1749-6632.2009.04469.x.
DeWind, N. K., Adams, G. K., Platt, M. L., & Brannon, E. M. (2015). Modeling the approximate number system to quantify the contribution of visual stimulus features. Cognition, 142, 247–265. doi:10.1016/j.cognition.2015.05.016.
Dietrich, J. F., Huber, S., Klein, E., Willmes, K., Pixner, S., & Moeller, K. (2016). A systematic investigation of accuracy and response time based measures used to index ANS acuity. PLoS One, 11(9), e0163076.
Dietrich, J. F., Huber, S., Moeller, K., & Klein, E. (2015a). The influence of math anxiety on symbolic and non-symbolic magnitude processing. Frontiers in Psychology, 6, 1621. doi:10.3389/fpsyg.2015.01621.
Dietrich, J. F., Huber, S., & Nuerk, H.-C. (2015b). Methodological aspects to be considered when measuring the approximate number system (ANS)—a research review. Frontiers in Psychology, 6, 295. doi:10.3389/fpsyg.2015.00295.
Durgin, F. H. (1995). Texture density adaptation and the perceived numerosity and distribution of texture. Journal of Experimental Psychology: Human Perception and Performance, 21, 149–169.
Espy, K. A., McDiarmid, M. M., Cwik, M. F., Stalets, M. M., Hamby, A., & Stern, T. E. (2004). The contribution of executive functions to emergent mathematic skills in preschool children. Developmental Neuropsychology, 26(1), 465–486.
Fazio, L. K., Bailey, D. H., Thompson, C. A., & Siegler, R. S. (2014). Relations of different types of numerical magnitude representations to each other and to mathematics achievement. Journal of Experimental Child Psychology, 123(1), 53–72. doi:10.1016/j.jecp.2014.01.013.
Feigenson, L., Dehaene, S., & Spelke, E. (2004). Core systems of number. Trends in Cognitive Sciences, 8(7), 307–314. doi:10.1016/j.tics.2004.05.002.
Feigenson, L., Libertus, M. E., & Halberda, J. (2013). Links between the intuitive sense of number and formal mathematics ability. Child Development Perspectives, 7(2), 74–79. doi:10.1111/cdep.12019.
Fuhs, M. W., & McNeil, N. M. (2013). ANS acuity and mathematics ability in preschoolers from low-income homes: Contributions of inhibitory control. Developmental Science, 16(1), 136–148. doi:10.1111/desc.12013.
Gandini, D., Lemaire, P., Anton, J.-L., & Nazarian, B. (2008a). Neural correlates of approximate quantification strategies in young and older adults: An fMRI study. Brain Research, 1246, 144–157. doi:10.1016/j.actpsy.2008.05.009.
Gandini, D., Lemaire, P., & Dufau, S. (2008b). Older and younger adults’ strategies in approximate quantification. Acta Psychologica, 129(1), 175–189. doi:10.1016/j.actpsy.2008.05.009.
Gebuis, T., & Reynvoet, B. (2011). Generating nonsymbolic number stimuli. Behavior Research Methods, 43(4), 981–986. doi:10.3758/s13428-011-0097-5.
Gebuis, T., & Reynvoet, B. (2012). The interplay between nonsymbolic number and its continuous visual properties. Journal of Experimental Psychology: General, 141(4), 642–648. doi:10.1037/a0026218.
Gilmore, C., Attridge, N., Clayton, S., Cragg, L., Johnson, S., Marlow, N., & Inglis, M. (2013). Individual differences in inhibitory control, not non-verbal number acuity, correlate with mathematics achievement. PLoS One, 8(6), 1–9. doi:10.1371/journal.pone.0067374.
Gilmore, C., Attridge, N., De Smedt, B., & Inglis, M. (2014). Measuring the approximate number system in children: Exploring the relationships among different tasks. Learning and Individual Differences, 29, 50–58. doi:10.1016/j.lindif.2013.10.004.
Gilmore, C., Attridge, N., & Inglis, M. (2011). Measuring the approximate number system. The Quarterly Journal of Experimental Psychology, 64(11), 2099–2109. doi:10.1080/17470218.2011.574710.
Guay, R. B., & McDaniel, E. D. (1977). The relationship between mathematics achievement and spatial abilities among elementary school children. Journal for Research in Mathematics Education, 8(3), 211–215. doi:10.2307/748522.
Guillaume, M., Nys, J., Mussolin, C., & Content, A. (2013). Differences in the acuity of the approximate number system in adults: The effect of mathematical ability. Acta Psychologica, 144(3), 506–512. doi:10.1016/j.actpsy.2013.09.001.
Gunderson, E. A., Ramirez, G., Beilock, S. L., & Levine, S. C. (2012). The relation between spatial skill and early number knowledge: The role of the linear number line. Developmental Psychology, 48(5), 1229–1241. doi:10.1037/a0027433.
Halberda, J., Ly, R., Wilmer, J. B., Naiman, D. Q., & Germine, L. (2012). Number sense across the lifespan as revealed by a massive Internet-based sample. Proceedings of the National Academy of Sciences, 109(28), 11116–11120. doi:10.1073/pnas.1200196109.
Halberda, J., Mazzocco, M. M. M., & Feigenson, L. (2008). Individual differences in non-verbal number acuity correlate with maths achievement. Nature, 455, 665–668. doi:10.1038/nature07246.
Hothorn, T., Bretz, F., & Westfall, P. (2008). Simultaneous inference in general parametric models. Biometrical Journal, 50(3), 346–363. doi:10.1002/bimj.200810425.
Inglis, M., Attridge, N., Batchelor, S., & Gilmore, C. (2011). Non-verbal number acuity correlates with symbolic mathematics achievement: But only in children. Psychonomic Bulletin & Review, 18(6), 1222–1229. doi:10.3758/s13423-011-0154-1.
Inglis, M., & Gilmore, C. (2013). Sampling from the mental number line: How are approximate number system representations formed? Cognition, 129(1), 63–69. doi:10.1016/j.cognition.2013.06.003.
Inglis, M., & Gilmore, C. (2014). Indexing the approximate number system. Acta Psychologica, 145, 147–155. doi:10.1016/j.actpsy.2013.11.009.
Jaeger, T. F. (2008). Categorical data analysis: Away from ANOVAs (transformation or not) and towards logit mixed models. Journal of Memory and Language, 59(4), 434–446. doi:10.1016/j.jml.2007.11.007.
James, G., Witten, D., Hastie, T., & Tibshirani, R. (2013). An introduction to statistical learning (Vol. 6). New York: Springer.
Kaufman, E. L., Lord, M. W., Reese, T. W., & Volkmann, J. (1949). The discrimination of visual number. The American Journal of Psychology, 62(4), 498–525. doi:10.2307/1418556.
Keller, L., & Libertus, M. (2015). Inhibitory control may not explain the link between approximation and math abilities in kindergarteners from middle class families. Frontiers in Psychology, 6, 685. doi:10.3389/fpsyg.2015.00685.
Kirk, E. P., & Ashcraft, M. H. (2001). Telling stories: The perils and promise of using verbal reports to study math strategies. Journal of Experimental Psychology. Learning, Memory, and Cognition, 27(1), 157–175. doi:10.1037/0278-7393.27.1.157.
Kolkman, M. E., Kroesbergen, E. H., & Leseman, P. P. M. (2013). Early numerical development and the role of non-symbolic and symbolic skills. Learning and Instruction, 25, 95–103. doi:10.1016/j.learninstruc.2012.12.001.
Kurdek, L. A., & Sinclair, R. J. (2001). Predicting reading and mathematics achievement in fourth-grade children from kindergarten readiness scores. Journal of Educational Psychology, 93(3), 451–455. doi:10.1037/0022-0663.93.3.451.
Kuznetsova, A., Brockhoff, P. B., & Christensen, R. H. (2015). lmerTest: Tests in linear mixed effects models. Retrieved from http://cran.r-project.org/package=lmerTest.
Landis, J. R., & Koch, G. G. (1977). The measurement of observer agreement for categorical data. Biometrics, 33(1), 159–174. doi:10.2307/2529310.
Leibovich, T., & Henik, A. (2013). Magnitude processing in non-symbolic stimuli. Frontiers in Psychology, 4(June), 375. doi:10.3389/fpsyg.2013.00375.
Libertus, M. E., Feigenson, L., & Halberda, J. (2011). Preschool acuity of the approximate number system correlates with school math ability. Developmental Science, 14(6), 1292–1300. doi:10.1111/j.1467-7687.2011.01080.x.
Libertus, M. E., Woldorff, M. G., & Brannon, E. M. (2007). Electrophysiological evidence for notation independence in numerical processing. Behavioral and Brain Functions, 3, 1. doi:10.1186/1744-9081-3-1.
Lindskog, M., Winman, A., Juslin, P., & Poom, L. (2013). Measuring acuity of the approximate number system reliably and validly: The evaluation of an adaptive test procedure. Frontiers in Psychology, 4, 510. doi:10.3389/fpsyg.2013.00510.
Lipton, J. S., & Spelke, E. S. (2005). Preschool children’s mapping of number words to nonsymbolic numerosities. Child Development, 76(5), 978–988. doi:10.1111/j.1467-8624.2005.00891.x.
Lonnemann, J., Linkersdörfer, J., Hasselhorn, M., & Lindberg, S. (2013). Developmental changes in the association between approximate number representations and addition skills in elementary school children. Frontiers in Psychology, 4, 783. doi:10.3389/fpsyg.2013.00783.
Luwel, K., & Verschaffel, L. (2003). Adapting strategy choices to situational factors: The effect of time pressure on children’s numerosity judgement strategies. Psychologica Belgica, 43, 269–295.
Luwel, K., Verschaffel, L., Onghena, P., & De Corte, E. (2003a). Flexibility in strategy use: Adaptation of numerosity judgement strategies to task characteristics. European Journal of Cognitive Psychology, 15(2), 247–266. doi:10.1080/09541440244000139.
Luwel, K., Verschaffel, L., Onghena, P., & De Corte, E. (2003b). Strategic aspects of numerosity judgment: The effect of task characteristics. Experimental Psychology, 50(1), 63–75. doi:10.1026//1618-3169.50.1.63.
Lyons, I. M., Ansari, D., & Beilock, S. L. (2015a). Qualitatively different coding of symbolic and nonsymbolic numbers in the human brain. Human Brain Mapping, 36(2), 475–488. doi:10.1002/hbm.22641.
Lyons, I. M., Nuerk, H.-C., & Ansari, D. (2015b). Rethinking the implications of numerical ratio effects for understanding the development of representational precision and numerical processing across formats. Journal of Experimental Psychology: General, 144(5), 1021–1035. doi:10.1037/xge0000094.
Mandler, G., & Shebo, B. J. (1982). Subitizing: An analysis of its component processes. Journal of Experimental Psychology: General, 111(1), 1–22. doi:10.1037/0096-3445.111.1.1.
Mazzocco, M. M. M., Feigenson, L., & Halberda, J. (2011). Preschoolers’ precision of the approximate number system predicts later school mathematics performance. PLoS One, 6, e23749. doi:10.1371/journal.pone.0023749.
Mazzocco, M. M. M., & Myers, G. F. (2003). Complexities in identifying and defining mathematics learning disability in the primary school-age years. Annals of Dyslexia, 53(1), 218–253. doi:10.1007/s11881-003-0011-7.
Mundy, E., & Gilmore, C. K. (2009). Children’s mapping between symbolic and nonsymbolic representations of number. Journal of Experimental Child Psychology, 103(4), 490–502. doi:10.1016/j.jecp.2009.02.003.
Nieder, A. (2011). The neural code for number. In S. Dehaene & E. M. Brannon (Eds.), Space, time and number in the brain: Searching for the foundations of mathematical thought (pp. 103–118). London: Academic Press.
Nieder, A. (2013). Coding of abstract quantity by “number neurons” of the primate brain. Journal of Comparative Physiology A, 199(1), 1–16.
Nieder, A., & Dehaene, S. (2009). Representation of number in the brain. Annual Review of Neuroscience, 32, 185–208. doi:10.1146/annurev.neuro.051508.135550.
Nieder, A., Freedman, D. J., & Miller, E. K. (2002). Representation of the quantity of visual items in the primate prefrontal cortex. Science, 297(5587), 1708–1711.
Noël, M. P., & Rousselle, L. (2011). Developmental changes in the profiles of dyscalculia: an explanation based on a double exact-and-approximate number representation model. Frontiers in Human Neuroscience, 5, 165. doi:10.3389/fnhum.2011.00165.
Piazza, M. (2010). Neurocognitive start-up tools for symbolic number representations. Trends in Cognitive Sciences, 14(12), 542–551. doi:10.1016/j.tics.2010.09.008.
Piazza, M., Izard, V., Pinel, P., Le Bihan, D., & Dehaene, S. (2004). Tuning curves for approximate numerosity in the human intraparietal sulcus. Neuron 44(3), 547–555
Price, G. R., Palmer, D., Battista, C., & Ansari, D. (2012). Nonsymbolic numerical magnitude comparison: Reliability and validity of different task variants and outcome measures, and their relationship to arithmetic achievement in adults. Acta Psychologica, 140(1), 50–57. doi:10.1016/j.actpsy.2012.02.008.
Ratcliff, R. (1993). Methods for dealing with reaction time outliers. Psychological Bulletin, 114(3), 510–532. doi:10.1037/0033-2909.114.3.510.
Reuhkala, M. (2001). Mathematical skills in ninth-graders: Relationship with visuo-spatial abilities and working memory. Educational Psychology, 21(4), 387–399. doi:10.1080/01443410120090786.
Revkin, S. K., Piazza, M., Izard, V., Cohen, L., & Dehaene, S. (2008). Does subitizing reflect numerical estimation? Psychological Science, 19(6), 607–614. doi:10.1111/j.1467-9280.2008.02130.x.
Robinson, K. M. (2001). The validity of verbal reports in children’s subtraction. Journal of Educational Psychology, 93(1), 211–222. doi:10.1037/0022-0663.93.1.211.
Russo, J. E., Johnson, E. J., & Stephens, D. L. (1989). The validity of verbal protocols. Memory & Cognition, 17(6), 759–769. doi:10.3758/BF03202637.
Schneider, M., Beeres, K., Coban, L., Merz, S., Susan Schmidt, S., Stricker, J., & De Smedt, B. (2016). Associations of non-symbolic and symbolic numerical magnitude processing with mathematical competence: A meta-analysis. Developmental Science. doi:10.1111/desc.12372.
Seyler, D. J., Kirk, E. P., & Ashcraft, M. H. (2003). Elementary Subtraction. Journal of Experimental Psychology: Learning, Memory, and Cognition, 29(6), 1339–1352. doi:10.1037/0278-7393.29.6.1339.
Singmann, H., Bolker, B., & Westfall, J. (2015). afex: Analysis of factorial experiments. Retrieved from http://cran.r-project.org/package=afex.
Smets, K., Sasanguie, D., Szücs, D., & Reynvoet, B. (2015). The effect of different methods to construct non-symbolic stimuli in numerosity estimation and comparison. Journal of Cognitive Psychology, 27(3), 310–325. doi:10.1080/20445911.2014.996568.
Smith-Chant, B. L., & LeFevre, J.-A. (2003). Doing as they are told and telling it like it is: Self-reports in mental arithmetic. Memory and Cognition, 31(4), 516–528. doi:10.3758/BF03196093.
Soltész, F., Szucs, D., & Szucs, L. (2010). Relationships between magnitude representation, counting and memory in 4- to 7-year-old children: a developmental study. Behavioral and Brain Functions: BBF, 6, 13. doi:10.1186/1744-9081-6-13.
St Clair-Thompson, H. L., & Gathercole, S. E. (2006). Executive functions and achievements in school: Shifting, updating, inhibition, and working memory. The Quarterly Journal of Experimental Psychology, 59(4), 745–759. doi:10.1080/17470210500162854.
Szűcs, D., Nobes, A., Devine, A., Gabriel, F. C., & Gebuis, T. (2013). Visual stimulus parameters seriously compromise the measurement of approximate number system acuity and comparative effects between adults and children. Frontiers in Psychology, 4, 444. doi:10.3389/fpsyg.2013.00444.
Trick, L. M., & Pylyshyn, Z. W. (1994). Why are small and large numbers enumerated differently? A limited-capacity preattentive stage in vision. Psychological Review, 101(1), 80–102. doi:10.1037/0033-295X.101.1.80.
van den Berg, B. A., Reinders, M. J. T., de Ridder, D., & de Beer, T. A. P. (2015). Insight into neutral and disease-associated human genetic variants through interpretable predictors. PLoS One, 10(3), 1–17. doi:10.1371/journal.pone.0120729.
van Marle, K., Chu, F. W., Li, Y., & Geary, D. C. (2014). Acuity of the approximate number system and preschoolers’ quantitative development. Developmental Science, 17(4), 492–505. doi:10.1111/desc.12143.
Vanbinst, K., Ghesquière, P., & De Smedt, B. (2012). Numerical magnitude representations and individual differences in children’s arithmetic strategy use. Mind, Brain, and Education, 6(3), 129–136. doi:10.1111/j.1751-228X.2012.01148.x.
Verguts, T., & Fias, W. (2004). Representation of number in animals and humans: A neural model. Journal of Cognitive Neuroscience, 16(9), 1493–1504. doi:10.1162/0898929042568497.
Acknowledgements
The current research was supported by the Leibniz-Competition Fund (SAW) providing funding to Elise Klein, supporting Stefan Huber as well as by the German Research Foundation (DFG) providing funding to Korbinian Moeller (MO 2525/2-1), supporting Julia F. Dietrich as well as the Margarete von-Wrangell Fellowship of the European Social Fonds (ESF) and the Ministry of Science, Research and Arts Baden-Wuerttemberg, supporting Elise Klein. We thank Frauke Griebel and Sarah Weber for their support in coding the verbal reports.
Author information
Authors and Affiliations
Corresponding author
Ethics declarations
Conflict of interest
The authors declare that they have no conflict of interest.
Funding
The current research was supported by the Leibniz-Competition Fund (SAW) providing funding to Elise Klein, supporting Stefan Huber as well as by the German Research Foundation (DFG) providing funding to Korbinian Moeller (MO 2525/2-1), supporting Julia F. Dietrich as well as the Margarete von-Wrangell Fellowship of the European Social Fonds (ESF) and the Ministry of Science, Research and Arts Baden-Wuerttemberg, supporting Elise Klein.
Ethical approval
All procedures performed in studies involving human participants were in accordance with the ethical standards of the institutional and/or national research committee and with the 1964 Helsinki declaration and its later amendments or comparable ethical standards.
Availability of data and material
Data analysed during this study are included in the supplementary information files.
Electronic supplementary material
Below is the link to the electronic supplementary material.
Appendix
Rights and permissions
About this article
Cite this article
Dietrich, J.F., Nuerk, HC., Klein, E. et al. Set size influences the relationship between ANS acuity and math performance: a result of different strategies?. Psychological Research 83, 590–612 (2019). https://doi.org/10.1007/s00426-017-0907-1
Received:
Accepted:
Published:
Issue Date:
DOI: https://doi.org/10.1007/s00426-017-0907-1