Abstract
Purpose
The aim of this study was to develop and validate a nomogram model to evaluate lymph node metastasis (LNM) in patients with rectal cancer (RC).
Methods
A total of 162 patients with RC were included in the study. The MRI reported model, the Radscore model, and the Complex model were constructed using the logistics regression (LR) algorithm. The DeLong test and decision curve analysis (DCA) were used to compare the prediction performance and clinical utility of these models. The nomogram model was constructed to visualize the prediction results of the best model. Model performance was evaluated in the training and validation groups, and the calibration curve and Hosmer–Lemeshow goodness of fit test were used to evaluate the calibration.
Result
All three models constructed by the LR algorithm were good at identifying LNM. The DeLong test and the DCA results showed that the Complex model outperformed the MRI reported model and the Radscore model in relation to their predictive performance and clinical utility. The nomogram of the Complex model had an area under the curve (AUC) of 0.902 (95% confidence interval (CI) 0.848–0.957) in the training group and an AUC of 0.891 (95% CI 0.799–0.983) in the validation group. Meanwhile, the nomogram showed good calibration.
Conclusion
The nomogram model constructed based on T2WI radiomics and MRI reported had good diagnostic efficacies for LNM in patients with RC, and provided a new auxiliary method for accurate and individualized clinical management.
Graphical abstract

Similar content being viewed by others
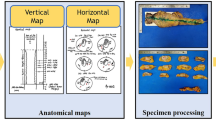
Explore related subjects
Discover the latest articles, news and stories from top researchers in related subjects.Avoid common mistakes on your manuscript.
Introduction
Rectal cancer (RC) is one of the most common malignancies in the digestive system, the Global Cancer Statistics 2020 showed that colorectal cancer (CRC) has a high incidence (10.0%) and mortality (9.4%) [1]. In the past few decades, the incidence of the disease in the United States has generally declined, but has increased in the younger age [2]. The 2016 China Cancer Statistics report showed that both the incidence and mortality of CRC have increased significantly in China [3]. Currently, patients with RC are classified by staging of the tumor/lymph node/metastasis (TNM) system validated by the American Joint Committee on Cancer [4]. Accurate preoperative identification of lymph node metastasis (LNM) is an essential factor for guiding treatment decisions and predicting patient survival [4,5,6,7]. For patients with LNM, surgical resection accompanied by lymph node (LN) dissection is necessary, however, surgical treatment is invasive, expensive, and exhibits inevitable postoperative complications. Postoperative mortality for colorectal and rectal cancer surgery has been reported to be approximately 3–6% [8, 9]. Therefore, in order to reduce or avoid the risk of invasiveness and complications in elective patients, endoscopic resection could be used as another option for patients with early T stage without LNM. It should be noted that the relationship between micrometastases and poor prognosis in patients with node-negative CRC remains controversial. Some immunological studies have shown no association between micrometastases and poor prognosis, while a few studies reported that there was a strong association between them [10]. Patients with LNM have a 5-year survival of 50–68%, with a higher risk of locoregional recurrence. However, for patients without LNM, the 5-year survival increases to 95%, and the risk of loco-regional recurrence is relatively low [11]. Therefore, the prediction of LNs and the accurate assessment of LN state are essential for treatment decision making and prognostic assessments of patients with RC.
Magnetic resonance imaging (MRI) has been recommended by the European Society for Medical Oncology as a part of the standard treatment program for RC [12]. Traditionally, LNs can be evaluated based on their size and changes in internal signal, although due to reactive LN hyperplasia, which can cause changes in internal structures, it can be difficult to identify whether the LN is metastatic or not by observing the change in signal strength alone [13]. In recent years, the application of diffusion weighted imaging (DWI) has greatly improved the qualitative diagnostic accuracy of LNM. The LN detection rate using DWI was about 6% higher than that using conventional T2WI. Seber et al. [14] proved that the apparent diffusion coefficient (ADC) can, to some extent, distinguish between benign and malignant nodes. However, due to the sample size in that study, the choice of b value, ADC value mathematical algorithm model, and the region of interest (ROI), the ADC values have different predictive values for LNM in patients with RC. Other studies have aimed to explore the diagnostic accuracy of LMN in patients with RC by using dynamic contrast-enhanced MRI, magnetic resonance spectroscopy, and blood oxygenation level-dependent MRI [15,16,17]; however, such methods could not achieve a unified consensus and are greatly affected by the scanning parameters and the technology itself. Although some histopathological findings, such as LN infiltration and tumor differentiation, are known to be predictors of LNM, they are only available postoperatively [18].
Radiomics is the process of converting medical images into high-dimensional, exploitable data through high-throughput quantitative feature extraction, followed by data analysis for decision-making support [19]. Radiomics has shown promising prospects in assessing tumor heterogeneity, predicting prognosis, and responding to the tumor microenvironment [20]. Radiomics facilitates the exploration of deep hidden information from medical diagnoses at the macro level to promote precision medicine. Several studies have applied radiomics to study LNM in patients with RC, however, constructing a facilitative model for clinical use in patient management would be significant. The aim of this study was to further confirm the value of radiomic features based on T2WI in predicting LNM in RC patients, and to confirm the complementary role of radiomics in MRI structured reporting assessment of metastatic LN in RC, and to construct a visual and convenient nomogram model.
Materials and methods
Patients
This retrospective study was approved by the ethics review board of The First Affiliated Hospital of Harbin Medical University. A total of 290 consecutive patients with RC who were treated between January 2019 and August 2021 were enrolled in the study. All patients underwent rectal MRI, then surgical resection and postoperative histopathological examination within 1 week. The inclusion criteria were as follows: (1) pathologically confirmed adenocarcinoma < 15 cm from the anal verge, and (2) no history of pelvic surgery. A total of 128 patients were excluded for the following reasons: (1) they underwent neoadjuvant chemoradiotherapy, (2) they had a special histopathological type, including mucinous adenocarcinoma and villotubular adenoma, (3) their MRI scan was not performed or contained poor image quality, and (4) they did not undergo surgery. Ultimately, 162 patients were enrolled in the study. The patients were allocated to a training set (n = 114) and a validation set (n = 48) at a ratio of 7:3 using stratified randomized sampling. The screening procedure for this study is shown in Fig. 1. Baseline prognostic clinical–pathological factors, including age, sex, and TN stage were derived from the patients' electronic medical records. The cohort consisted of 162 patients with RC, including 57 females (35.2%) and 105 males (64.8%), with a mean age of 63.12 ± 9.95 years. A total of 54 patients had LNM in this study.
MRI parameters
MRI scans were performed using a 1.5 T MRI scanner (MAGNETOM Skyra, Siemens Healthcare, Erlangen, Germany) with an 8-channel pelvic phased-array coil. Every patient fasted for 8 h prior to the scan to empty the contents of their intestine. Transversal high-resolution T2W turbo spin echo images were acquired with the following parameters: TR/TE = 4500/110 ms, FOV = 180 × 180 mm2, matrix = 320 × 320, slice thickness = 3 mm, gap = 0 mm, acceleration factor = 3, echo train length = 16, and acquisition time = 4 min 10 s.
Image segmentation and radiomic feature extraction
Tumor segmentation was conducted using the Dr. Wise multimodal scientific research platform (version number: V1.6.2.1, website: keyan.deepwise.com). Region of interest (ROI) delineation was performed by two independent radiologists (reader 1 with 3 years of experience in abdominal imaging, and reader 2 with 8 years in interpreting abdominal MRIs) who were aware of the inclusion criteria for the study, but were blinded to other histopathological findings. All ROIs were segments on the maximum slice in T2WI manually, which contained the chords and burrs surrounding lesions and excluded the fluid in the intestinal lumen. To minimize the impact of different machine parameters on image analysis, image standardization used b spline interpolation sampling techniques for resampling of all MRI images to 2.0 × 2.0 × 2.0 mm3 voxels. A total of 103 high-throughput data features based on feature classes were automatically extracted by the Dr. Wise platform. The process followed image biomarker standardization initiative (IBSI) [21], including 18 first order statistic features, 12 shape-based (2D) features, 22 Gy-level co-occurrence matrix (GLCM), 16 Gy-level run-length matrix (GLRLM), 16 Gy-level size zone matrix (GLSZM), 14 Gy-level dependence matrix (GLDM), and 5 Neighbouring gray tone difference matrix (NGTDM) features.
Manual segmentation may introduce a degree of uncertainty during the determination of tumor ROI. Some features may have less reproducibility when the tumor ROI is manually described by different individuals or at different times [22]. To eliminate the features that were lowly reproducible, reader 1 completed the lesion segmentation for all patients. At 14 days apart, reader 2 randomly selected 20 patients to segment the ROI [23,24,25]. The intraclass correlation coefficient (ICC) was used to assess the inter-observer reproducibility of feature extraction. When the ICC exceeded 0.75, it was considered as having good agreement. The range of the ICC between the two observers was 0.933 ± 0.070. Two features (ClusterShadeGLCM and ClusterProminenceGLCM) were poorly reproducible and were deleted. A total of 101 features were retained.
Radiomics signature building
All features were processed using z-score standardization. The least absolute shrinkage and selection operator (LASSO) method was used to screen the optimal features in the training set. Ten-fold cross-validation was used to compute the optimal lambda. The radiomic signature score (Radscore) was calculated based on the LASSO regression equation.
MRI structured reporting
Images reading and MRI structured reporting writing were performed by two radiologists with 10 years of experience in abdominal radiology diagnosis. The size of tumor lesion, degree of invasion, and number of metastatic LNs were included in the reports, and the diagnostic results were used as the basis for preoperative N staging of RC. Both radiologists observed images independently and were blinded to each other. The diagnostic criteria for LN status included the following: (1) nodal location, (2) the morphological features, such as nodal borders, the short-axis node diameter and an internal signal, (3) whether the evaluated LNs had chemical shift effects(CSE), and (4) restricted diffusion in the DWI sequences (the LNs showed a high signal). If none of the above diagnostic criteria were met, the patient was judged to have no metastatic LNs. LNM was considered when the suspicious LN had restricted diffusion in DWI and had irregular/absent CSE, accompanied with a short-axis node diameter of > 9 mm, rough borders and was located ipsilateral to the primary tumor. When the suspicious LN showed DWI restricted diffusion but with regular CSE, the size, margin, and location were further evaluated. When the short-axis node diameter was increased and the border was not smooth, it was still considered as LNM. However, when not accompanied by such changes, the LN was more likely to be judged as inflammatory LN. Agreement in LN status diagnosis was reached through consultation when the reviewers’ opinions were contradictory. The diagnostic results of the two observers were compared with the histopathological validation. Patients with non-LNM were defined as label 0, and patients with LNM were defined as label 1. The interpretation results were input into the logistic regression (LR) to construct a model named the MRI reported model.
Model establishment and comparison
The model was constructed using LR in the training group. The MRI reported model was based on MRI structured reporting, the Radscore model was based on the Radscore, and the Complex model was based on the MRI structured reporting and the Radscore. Model performance was evaluated using the receiver operating characteristic (ROC) and calculating the area under receiver operating characteristic curve (AUC) values. The Delong test was used to determine whether AUC values were statistically different between the three models. The clinical utility of the prediction models was determined and compared using decision curve analysis (DCA) by quantifying the net benefit to the patient under different threshold probabilities in the queue.
Development and validation of the individualized nomogram
To develop a visually quantitative tool to predict LNM in patients with RC, we developed a nomogram based on the prediction model with the highest AUC value and the clinical utility in the training set. The AUC [95% confidence interval (CI)], sensitivity, specificity, and accuracy of the model were calculated in the training and validation sets. Calibration curves were plotted to assess the calibration of the nomogram by bootstrapping (1000 bootstrap resamples) based on the internal (training set) and external (validation set) validity. The Hosmer–Lemeshow test was used to assess the goodness of fit of the nomogram model.
Statistical analysis
All statistical analyses and model building were performed in the R language (version 3.6.3, http://www.r-project.org). The R package was used to randomize the training and verification groups using “caret.” Clinical data were expressed as \(\overline{x}\) ± s or percentage. An independent samples t-test or the Wilcoxon test was used for continuous variables, and the Fisher’s exact test or χ2 test was used for categorical variables. The ICC analysis was performed using the R software packages “readr” and “irr.” LASSO regression and LR model building was performed using the R package, “glmnet.” ROC curve analysis was performed using the R software package, “pROC.” The nomogram model and the calibration curve were constructed using the R software package, “rms.” The Hosmer–Lemeshow goodness-of-fit test was performed using the R software package, “ResourceSelection.” The DCA curves were plotted using the R software package, “dcurves.” A two-tailed P < 0.05 indicated statistical significance.
Results
Patient characteristics
The cohort was randomly divided into a training cohort (n = 114) and a validation cohort (n = 48) according to 7:3 ratio. The clinical characteristics of the 162 patients in the training and validation cohorts are summarized in Table 1. There were no statistical differences in age (P = 0.335), sex (P = 0.298), T stage (P = 0.945), N stage (P = 1.000), or MRI structured reporting (P = 0.352) between the training and the validation cohorts.
Radiomic feature selection and Radscore building
Of all texture features, 101 features were selected on the basis of the 114 patients in the training cohort using the LASSO LR model. When log(lambda) was − 4.004, the AUC value corresponding to the LASSO model was the highest (Fig. 2A and B), and 12 potential predictors with nonzero coefficients were retained, including SurfaceVolumeRatioShape, MajorAxisLengthShape, MedianHistogram, KurtosisHistogram, EnergyHistogram, Imc2GLCM, SmallAreaHighGrayLevelEmphasisGLSZM, SmallAreaLowGrayLevelEmphasisGLSZM, LargeAreaHighGrayLevelEmphasisGLSZM, GrayLevelNonUniformityNormalizedGLSZM, CoarsenessNGTDM, and GrayLevelNonUniformityNormalizedGLRLM. Then, the Radscore for each patient was calculated according to the following formula:
The LASSO algorithm and tenfold cross-validation were used to extract the optimal subset of radiomic features. A Optimal feature selection according to AUC value. When the value log (lambda) increased to -4.004, the AUC reached the peak corresponding to the optimal number of radiomic features. B LASSO coefficient profiles of the 101 radiomic features. The vertical line was drawn at the value selected by tenfold crossvalidation, where the optimal lambda resulted in 12 nonzero coefficients. LASSO least absolute shrinkage and selection operator, AUC area under receiver operating characteristic curve
In the training cohort (Radscore = − 0.436 vs − 0.891) and the validation cohort (Radscore = − 0.501 vs. − 0.859), the Radscore of RC patients with LNM was significantly higher than that of non-LNM patients (training cohort: P < 0.001; validation cohort: P = 0.003). The Radscores of the two groups are shown as violin plots in Fig. 3A and B.
Violin plot of Radscore for LNM and non-LNM patients in training (A) and validation (B) sets. The thick black line in the middle represents the median. The black line running up and down through the violin diagram represents the range from the smallest non-outlier value to the largest non-outlier value. LNM lymph node metastasis
Performance and clinical utility of the prediction models
The performance of the three models in predicting LNM in patients with RC was evaluated by ROC curves and compared using the DeLong test. The performance of the prediction models to identify LNM is shown in Fig. 4A. The MRI reported model, Radscore model, and Complex model all performed well in discriminating LNM, with AUC values of 0.882, 0.728, and 0.902, respectively. The Delong test showed that the AUC value of the Complex model was significantly higher than that of the MRI reported model (P = 0.001) and Radscore model (P < 0.001), while the MRI reported model had a higher AUC than the Radscore model; however, the difference was not significant (P = 0.159).
ROC curves and DCA of the three prediction models. A ROC curves for the three prediction models in differentiating LNM in the training set. The green line indicates MRI reported model, the blue line indicates Radscore model, the purple line indicates the Complex model. B DCA of the three prediction models in the training set. The Y-axis and the X-axis represent the net benefit and threshold probability respectively. The green line indicates MRI reported model, the blue line indicates Radscore model, the purple line indicates the Complex model, the red oblique line indicates the hypothesis that all patients were LNM, the horizontal brown line represents the hypothesis that all patients were non-LNM. ROC receiver operating characteristic, MRI magnetic resonance imaging, DCA decision curve analysis, Radscore radiomic signature score, LNM lymph node metastasis
Comparisons of the clinical utility of the models were performed using DCA. The results revealed that the Complex model outperformed the MRI reported model and Radscore model in a wide threshold range (Fig. 4B). Therefore, the Complex model was the most reliable clinical management tool for predicting LNM in patients with RC.
Individualized nomogram construction and validation
Considering the Complex model's ability to predict LNM, we developed a nomogram to represent the individual prediction based on the training cohort, and to visualize the prediction results and the proportion of each factor (Fig. 5A). The AUC of the model in the training cohort (n = 114) was 0.902 (95% CI 0.848–0.957), with a sensitivity of 0.798, a specificity of 0.868, and an accuracy of 0.842. The AUC of the model in the validation group (n = 48) was 0.891 (95% CI 0.799–0.983), with a sensitivity of 0.812, a specificity of 0.843, and an accuracy of 0.833. The nomogram exhibited good agreement between the predicted and observed values of the training and validation sets (Fig. 5B and C). The Hosmer–Lemeshow goodness of fit test showed that there was no significant difference between the predicted and observed values in either the training cohort (χ2 = 6.533, P = 0.588) or the validation cohort (χ2 = 9.116, P = 0.333), thus, indicating a good fit. The example of model application was shown in Fig. 6.
Development and performance of a nomogram. A Nomogram based on MRI reported and Radscore. Calibration curves of the nomogram in the training (B) and validation (C) sets. The horizontal axis is the predicted incidence of LNM. The vertical axis is the observed incidence of LNM. The gray line on the diagonal is the reference line, indicating that the predicted value is equal to the actual value and the blue line is the calibration curve. Radscore radiomics signature score, LNM lymph node metastasis
The example of the nomogram model application. A metastatic lymph node (white arrow) in the axial T2-weighted from a 66-year-old male was shown in A. Nomogram model based on MRI structured reporting and Radscore was shown in B. The Radscore calculated based on the LASSO regression equation was -0.492, which corresponded to the point 1 of 57.376 in the nomogram model. The N stage was diagnosed as N1 in the MRI structured reporting, and the corresponding point 2 in the nomogram was 45.813. The two points were added up to get a total point of 103.189, which corresponded to the LNM risk of 0.769. All the calculated scores were indicated by the red long arrow in B. MRI magnetic resonance imaging, Radscore radiomic signature score, LASSO the least absolute shrinkage and selection operator, LNM lymph node metastasis
Discussion
Because the presence of LNM is an important factor in the recurrence of CRC, determining the presence of LNM is important for clinical management and the prediction of survival in patients with CRC [26]. However, the diagnostic efficiency of the TNM staging system remains inadequate in that it cannot fully support the selection of preoperative treatment options [27]. Meanwhile, only adequate intraoperative dissection of 12 LNs can sufficiently confirm the presence of pathological LNM [8], and thus, the determination of the LN status may be inaccurate in patients with inoperable or inadequate LNs. Thus, more reliable quantitative detection of LNM may provide a means of determining the optimal treatment for patients with RC.
Radiomics is a recently developed approach that extracts a massive number of quantitative features from medical images and comprehensively evaluates tumor heterogeneity. Radiomic characteristics (intensity, shape, texture, or wavelet) provide information on the cancer phenotype and tumor microenvironment that is different, but complementary to other relevant data sources [20]. The results of numerous studies have suggested a potential correlation between the radiomic features of primary tumors and LNM [9, 13, 18, 22, 28, 29]. The results of the present study also confirmed that the Radscore constructs based on T2WI differed significantly between the different LN states of RC (P < 0.05). The above results suggested that radiomic features are potential biomarkers for predicting LNM in patients with RC. Such beneficial results thus facilitate the use of radiomics to predict LN status. It is worth noting, however, that the effect of assessing LN status using radiomic features alone was limited, and the model constructed using Radscores alone was good at predicting LNM in patients with RC, with an AUC lower than that of the MRI reported model. Ma et al. compared multiple classifier models for N staging, and the diagnostic efficiency of the random forest classifier was better. However, the AUC was 0.74 [8]. Therefore, we believe that the value of radiomics alone as a marker of LNM needs to be further confirmed.
The assessment of LN status by conventional T2WI is performed based on the changes in size, morphology, and signal intensity of the LN. The diagnostic results are highly subjective and lead to low accuracy and reproducibility. With the development of functional MRI, studies have shown that the DWI detection rate for LNM was higher than 6% for conventional T2WI [30]. Two experienced physicians were added to our study to assess LNM based on a combination of T2WI and DWI. Therefore, the prediction based on the MRI model was good (AUC: 0.882), suggesting that the role of MRI in the detection of LNM is critical. However, the findings do not mean that the imaging model of T2WI + DWI is without drawbacks. Seber et al. reported that the ADC of benign LN was higher than that of malignant nodes, and when the ADC was 0.8 × 10−3 mm2/s, the sensitivity for the diagnostic LNM was 76.4% compared to a specificity of 85.7% and an accuracy of 80.6%. Thus, those data indicated that DWI contributed to the diagnosis of LNM. However, this diagnostic method remained insufficient as the ADC overlaps between non-LNM and LNM, and hence, it could not fully identify benign and malignant LNs [14].
To build a more accurate model, we found that the predictive effect and clinical utility of the Complex model combining the Radscore and MRI structured reporting constructs was improved. We developed and validated a diagnostic and imaging-based nomogram model for the individualized prediction of LNM in preoperative patients with RC, distinguishing LNM from non-LNM in the training and validation groups (AUC: 0.902, AUC: 0.891) with high accuracy (0.842, 0.833). The calibration curve and the goodness of fit test showed good agreement between the predicted and observed values of the model. Based on the LR model, the nomogram model can integrate predictors and assign scores according to the contribution of predictors to the outcome variables (regression coefficient), thus providing a convenient way for clinical prediction of the risk of LNM in patients with RC. This advantage makes the nomogram get more extensive attention and application in the field of cancer research and clinical practice.
The results of previous studies suggest that nomogram models constructed by combining radiomic features with clinical factors or imaging reports were valuable in predicting LNM in RC patients. Huang et al. combined histological features with LN status and the carcinoembryonic antigen levels reported by computed tomography to establish a nomogram model that assessed LNM. The model exhibited better discrimination in the training and validation groups (C-index: 0.736, 0.778) [18]. Another study performed similar evaluations using MRI, where clinical risk factors were combined with high-resolution MRI factors and radiomic features to achieve good results (AUC: 0.90, 0.87) [31].
Many published predictive radiomic models are available to explain the factors associated with disease and treatment, however, such models lack standardized assessments of their performance, reproducibility, and/or clinical utility [32]. Although the current study was retrospective, we standardized the scanning parameters and procedures to ensure uniformity and to avoid selection bias. In addition, the maximum slice was chosen to segment the tumor in this study. Tumor segmentation methods are still inconclusive, with most studies choosing to segment the tumor maximum slice, the height of this method depends on the reader's choice of the maximum slice, and seems to lack an analysis of focal spatial heterogeneity. However, unlike solid organs, the volume of interest from the ROIs of continuous slices may not accurately represent the true shape of the primary lesion [6, 30] due to the growth properties of RC. Therefore, the maximum slice of segmentation may be a more appropriate way of segmentation.
This study also had the following limitations. First, the sample size was not sufficiently large, and thus, the sample size should be expanded to reduce the impact of the data size on the accuracy of the results. The proportion of LNM in the patients in this study was low, resulting in an unbalanced sample size. Second, manual segmentation was used when sketching the ROI. Compared with semi-automatic and automatic segmentation methods, manual ROI segmentation introduces more subjectivity, which will then affect the accuracy of extracting radiomic features. Third, the proportion of LNM in the included patients was also low, which resulted in an unbalanced sample size. Last, our study was conducted based on a single institution without including the test set. To maximize the possibility of model repetition and reproduction by other institutions, the images were resampled, the feature extraction procedure followed that of the IBSI and the poorly reproducible features were excluded by ICC. Although the validation set, which is not involved in building the model, can play a role in testing the effect of the model. However, the best test method is to test the reproducibility and generality of the model through an independent external test set, for which multi-center study is an effective approach.
Conclusion
In conclusion, the nomogram model constructed based on T2WI radiomics and MRI had good diagnostic efficacy for LNM in patients with RC, and provided a new option for precise personalized clinical management.
References
Sung H, Ferlay J, Siegel RL, Laversanne M, Soerjomataram I, et al. Global Cancer Statistics 2020: GLOBOCAN Estimates of Incidence and Mortality Worldwide for 36 Cancers in 185 Countries. CA Cancer J Clin. 2021 May;71(3):209-249. https://doi.org/10.3322/caac.21660.
Chang SH, Patel N, Du M, Liang PS. Trends in Early-onset vs Late-onset Colorectal Cancer Incidence by Race/Ethnicity in the United States Cancer Statistics Database. Clin Gastroenterol Hepatol. 2022 Jun;20(6):e1365-e1377. https://doi.org/10.1016/j.cgh.2021.07.035.
Chen W, Zheng R, Baade PD, Zhang S, Zeng H, et al. Cancer statistics in China, 2015. CA Cancer J Clin. 2016 Mar-Apr;66(2):115-32. https://doi.org/10.3322/caac.21338
Peyravian N, Larki P, Gharib E, Nazemalhosseini-Mojarad E, Anaraki F, et al. The Application of Gene Expression Profiling in Predictions of Occult Lymph Node Metastasis in Colorectal Cancer Patients. Biomedicines. 2018 Mar 2;6(1):27. https://doi.org/10.3390/biomedicines6010027.
Arnold M, Sierra MS, Laversanne M, Soerjomataram I, Jemal A, et al. Global patterns and trends in colorectal cancer incidence and mortality. Gut. 2017 Apr;66(4):683-691. https://doi.org/10.1136/gutjnl-2015-310912.
Beets-Tan RG, Beets GL. MRI for assessing and predicting response to neoadjuvant treatment in rectal cancer. Nat Rev Gastroenterol Hepatol. 2014 Aug;11(8):480-8. https://doi.org/10.1038/nrgastro.2014.41.
Yang Z, Liu Z. The efficacy of 18F-FDG PET/CT-based diagnostic model in the diagnosis of colorectal cancer regional lymph node metastasis. Saudi J Biol Sci. 2020 Mar; 27(3):805-811. https://doi.org/10.1016/j.sjbs.2019.12.017.
Ma X, Shen F, Jia Y, Xia Y, Li Q, et al. MRI-based radiomics of rectal cancer: preoperative assessment of the pathological features. BMC Med Imaging. 2019 Nov 12;19(1):86. https://doi.org/10.1186/s12880-019-0392-7.
Li M, Zhang J, Dan Y, Yao Y, Dai W, et al. A clinical-radiomics nomogram for the preoperative prediction of lymph node metastasis in colorectal cancer. J Transl Med. 2020 Jan 30;18(1):46. https://doi.org/10.1186/s12967-020-02215-0.
Noura S, Yamamoto H, Ohnishi T, Masuda N, Matsumoto T, et al. Comparative detection of lymph node micrometastases of stage II colorectal cancer by reverse transcriptase polymerase chain reaction and immunohistochemistry. J Clin Oncol. 2002 Oct 15;20(20):4232-41. https://doi.org/10.1200/JCO.2002.10.023.
Ishihara S, Kawai K, Tanaka T, Kiyomatsu T, Hata K, et al. Oncological Outcomes of Lateral Pelvic Lymph Node Metastasis in Rectal Cancer Treated With Preoperative Chemoradiotherapy. Dis Colon Rectum. 2017 May;60(5):469-476. https://doi.org/10.1097/DCR.0000000000000752.
Glynne-Jones R, Wyrwicz L, Tiret E, Brown G, Rödel C, et al. ESMO Guidelines Committee. Rectal cancer: ESMO Clinical Practice Guidelines for diagnosis, treatment and follow-up. Ann Oncol. 2017 Jul 1;28(suppl_4):iv22-iv40. https://doi.org/10.1093/annonc/mdx224.
Liu Y, Wan L, Peng W, Zou S, Zheng Z, et al. A magnetic resonance imaging (MRI)-based nomogram for predicting lymph node metastasis in rectal cancer: a node-for-node comparative study of MRI and histopathology. Quant Imaging Med Surg. 2021 Jun;11(6):2586-2597. https://doi.org/10.21037/qims-20-1049.
Seber T, Caglar E, Uylar T, Karaman N, Aktas E, et al. Diagnostic value of diffusion-weighted magnetic resonance imaging: differentiation of benign and malignant lymph nodes in different regions of the body. Clin Imaging. 2015 Sep-Oct;39(5):856-62. https://doi.org/10.1016/j.clinimag.2015.05.006.
Oberholzer K, Menig M, Pohlmann A, Junginger T, Heintz A, et al. Rectal cancer: assessment of response to neoadjuvant chemoradiation by dynamic contrast-enhanced MRI. J Magn Reson Imaging. 2013 Jul;38(1):119-26. https://doi.org/10.1002/jmri.23952.
Kim MJ, Lee SJ, Lee JH, Kim SH, Chun HK, et al. Detection of rectal cancer and response to concurrent chemoradiotherapy by proton magnetic resonance spectroscopy. Magn Reson Imaging. 2012 Jul;30(6):848-53. https://doi.org/10.1016/j.mri.2012.02.013.
Atkin G, Taylor NJ, Daley FM, Stirling JJ, Richman P, et al. Dynamic contrast-enhanced magnetic resonance imaging is a poor measure of rectal cancer angiogenesis. Br J Surg. 2006 Aug;93(8):992-1000. https://doi.org/10.1002/bjs.5352.
Huang YQ, Liang CH, He L, Tian J, Liang CS, et al. Development and Validation of a Radiomics Nomogram for Preoperative Prediction of Lymph Node Metastasis in Colorectal Cancer. J Clin Oncol. 2016 Jun 20;34(18):2157-64. https://doi.org/10.1200/JCO.2015.65.9128.
Horvat N, Bates DDB, Petkovska I. Novel imaging techniques of rectal cancer: what do radiomics and radiogenomics have to offer? A literature review. Abdom Radiol (NY). 2019 Nov;44(11):3764-3774. https://doi.org/10.1007/s00261-019-02042-y.
Lambin P, Leijenaar RTH, Deist TM, Peerlings J, de Jong EEC, et al. Radiomics: the bridge between medical imaging and personalized medicine. Nat Rev Clin Oncol. 2017 Dec;14(12):749-762. https://doi.org/10.1038/nrclinonc.
Zwanenburg A, Vallières M, Abdalah MA, Aerts HJWL, Andrearczyk V, et al. The Image Biomarker Standardization Initiative: Standardized Quantitative Radiomics for High-Throughput Image-based Phenotyping. Radiology. 2020 May;295(2):328-338. https://doi.org/10.1148/radiol.2020191145.
Meng X, Xia W, Xie P, Zhang R, Li W, et al. Preoperative radiomic signature based on multiparametric magnetic resonance imaging for noninvasive evaluation of biological characteristics in rectal cancer. Eur Radiol. 2019 Jun;29(6):3200-3209. https://doi.org/10.1007/s00330-018-5763-x.
Zhao H, Su Y, Wang M, Lyu Z, Xu P, et al. The Machine Learning Model for Distinguishing Pathological Subtypes of Non-Small Cell Lung Cancer. Front Oncol. 2022 May 26;12:875761. https://doi.org/10.3389/fonc.2022.875761.
Ying M, Pan J, Lu G, Zhou S, Fu J, et al. Development and validation of a radiomics-based nomogram for the preoperative prediction of microsatellite instability in colorectal cancer. BMC Cancer. 2022 May 9;22(1):524. https://doi.org/10.1186/s12885-022-09584-3.
Sun Y, Li C, Jin L, Gao P, Zhao W, et al. Radiomics for lung adenocarcinoma manifesting as pure ground-glass nodules: invasive prediction. Eur Radiol. 2020 Jul;30(7):3650-3659. https://doi.org/10.1007/s00330-020-06776-y.
Li F, Hu J, Jiang H, Sun Y. Diagnosis of lymph node metastasis on rectal cancer by PET-CT computer imaging combined with MRI technology. J Infect Public Health. 2020 Sep;13(9):1347-1353. https://doi.org/10.1016/j.jiph.2019.06.026.
Pages F, Mlecnik B, Marliot F, et al. International validation of the consensus Immunoscore for the classification of colon cancer: a prognostic and accuracy study. Lancet. 2018 May 26;391(10135):2128-2139. https://doi.org/10.1016/S0140-6736(18)30789-X.
Liu L, Liu Y, Xu L, Li Z, Lv H, et al. Application of texture analysis based on apparent diffusion coefficient maps in discriminating different stages of rectal cancer. J Magn Reson Imaging. 2017 Jun;45(6):1798-1808. https://doi.org/10.1002/jmri.25460.
Wang D, Zhuang Z, Wu S, Chen J, Fan X, et al. A Dual-Energy CT Radiomics of the Regional Largest Short-Axis Lymph Node Can Improve the Prediction of Lymph Node Metastasis in Patients With Rectal Cancer. Front Oncol. 2022 Jun 7;12:846840. https://doi.org/10.3389/fonc.2022.846840.
Heijnen LA, Lambregts DM, Mondal D, Martens MH, Riedl RG, et al. Diffusion-weighted MR imaging in primary rectal cancer staging demonstrates but does not characterise lymph nodes. Eur Radiol. 2013 Dec;23(12):3354-60. https://doi.org/10.1007/s00330-013-2952-5.
Yang YS, Feng F, Qiu YJ, Zheng GH, Ge YQ, et al. High-resolution MRI-based radiomics analysis to predict lymph node metastasis and tumor deposits respectively in rectal cancer. Abdom Radiol (NY). 2021 Mar;46(3):873-884. https://doi.org/10.1007/s00261-020-02733-x.
Vickers AJ. Prediction models: revolutionary in principle, but do they do more good than harm? J Clin Oncol. 2011 Aug 1;29(22):2951-2. https://doi.org/10.1200/JCO.2011.36.1329.
Funding
The authors did not receive support from any organization for the submitted work.
Author information
Authors and Affiliations
Contributions
All authors contributed to the study conception and design. YS and HZ provided the overall design of the experiments and contributed equally to the experiments. Material preparation, data collection and analysis were performed by LZ, YJ, PX, and ZL. The first draft of the manuscript was written by YS and HZ, and all authors commented on previous versions of the manuscript. PF and PL supervised the study. All authors read and approved the final manuscript.
Corresponding author
Ethics declarations
Conflict of interest
The authors have no competing interests to declare that are relevant to the content of this article.
Ethical approval
This retrospective chart review study involving human participants was in accordance with the ethical standards of the institutional and national research committee and with the 1964 Helsinki Declaration and its later amendments or comparable ethical standards. The study was approved by the First Affiliated Hospital of Harbin Medical University (No. 2021JS44). Due to the retrospective and non-interventional nature of the study, the written informed consent was waived by The First Affiliated Hospital of Harbin Medical University Review Board.
Additional information
Publisher's Note
Springer Nature remains neutral with regard to jurisdictional claims in published maps and institutional affiliations.
The paper has been preprinted at the Research Square. The specific information and link are followed: Yexin Su, Hongyue Zhao, Linhan Zhang et al. A Nomogram Model Developed and Validated for The Evaluation of Lymph Node Metastasis in Patients with Rectal Cancer, 14 February 2022, PREPRINT (Version 1) available at Research Square [https://doi.org/10.21203/rs.3.rs-1297512/v1].
Rights and permissions
Springer Nature or its licensor holds exclusive rights to this article under a publishing agreement with the author(s) or other rightsholder(s); author self-archiving of the accepted manuscript version of this article is solely governed by the terms of such publishing agreement and applicable law.
About this article
Cite this article
Su, Y., Zhao, H., Liu, P. et al. A nomogram model based on MRI and radiomic features developed and validated for the evaluation of lymph node metastasis in patients with rectal cancer. Abdom Radiol 47, 4103–4114 (2022). https://doi.org/10.1007/s00261-022-03672-5
Received:
Revised:
Accepted:
Published:
Issue Date:
DOI: https://doi.org/10.1007/s00261-022-03672-5