Abstract
The performance of the direct random phase approximation (RPA) method based on a Kohn–Sham reference for transition metal chemistry is studied by making comparison to (dispersion-corrected) density functional theory (DFT) and (spin-scaled) Møller–Plesset theory. The recently developed local-pair coupled-cluster method DLPNO-CCSD(T) is used as a benchmark. Emphasis is placed on the study of complete realistic mechanisms and reactions involving large systems. Electronic energies for the mechanism of C–H and C–C bond activation by rhodium fragments are presented as well as for ruthenium-catalyzed olefin metathesis. In addition, the WCCR10 test set, which comprises ten reactions, is revisited, and reaction energies for the reaction of a \(\mu \)-chloride-bridged palladacyclic dimer with phosphane ligands are presented. RPA yields results that are on average within 2–3 kcal/mol of the theoretical benchmark with a maximum deviation of 5 kcal/mol. Of the methods studied, RPA behaves most systematically and is able to provide results of similar accuracy to dispersion-corrected functionals. RPA can thus serve as a complementary method to DFT to obtain insight into transition metal chemistry. Attention is paid to the basis set convergence behavior of RPA as well.
Similar content being viewed by others
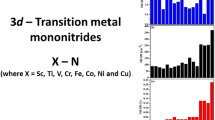
Avoid common mistakes on your manuscript.
1 Introduction
Transition metal elements play a significant role in many catalytic processes. They are, for example, widely used in C–H [1, 2] and C–C [3] bond activation, processes that are of huge importance in homogeneous catalysis [4]. Transition metals can often adapt multiple oxidation states and switch between them with relative ease, making them an integral part of many catalysts. Even though effort is made to find metal-free alternatives, it is clear that transition metals will play a role in the foreseeable future. One of the grand challenges in the field of transition metal catalysis is to find new pathways employing first-row transition metals that are abundantly available rather than the commonly used precious metals, such as palladium and ruthenium. An example is the recent interest in nickel as an alternative to palladium [5,6,7].
Understanding the mechanisms involved in these catalytic processes is of great importance to guide the development of a new generation of catalysts. Computations have become an integral part of many catalytic studies [8, 9]. They provide a unique atomistic picture which can assist in the quest to understand reaction mechanisms and to interpret spectroscopic data. The availability of established quantum chemistry software packages and the low cost of computational resources allow researchers to perform computations as part of a wider experimental study [10].
However, there is always a gap between the theoretical model employed and the chemical reaction as actually performed in the laboratory. By necessity, one has to make approximations to model the catalytic process, such as truncating the catalytic system to manageable size, treating the environment in a simplified manner, or ignoring dynamical effects due to motion of the nuclei. In general, the more accurate the theoretical model used, the more severe the approximations need to be to keep the associated computational cost within bounds. One should therefore be constantly aware of the impact of the approximations made. One consequence is that it is difficult to connect the theoretical results directly to experiment as it is often impossible to know the effect of what is being ignored, making it difficult to draw conclusions based on the computational outcomes [11]. In recent years, the phrase ‘the right answer for the wrong reason’ is being used frequently to point out that methods can coincidentally agree with experiment, but fail to describe the underlying physical system properly [12].
In view of this gap, it is important to understand the performance of a computational model, which motivates the large number of validation or benchmarking studies performed in recent years. Benchmarking, however, cannot be an end in itself. A connection needs to be made to experiment to see if a method can be useful in explaining spectroscopic data or a mechanism. A validation study for a method should thus put the method in the context of other methods and make the comparison to the highest-possible level of theory. In addition, the method should be applied to systems that are as close as possible to realistic and not only to model systems since model systems may not provide a correct picture of the performance of a method. This means that a wide range of systems needs to be included. Finally, if possible, comparison to experimental data should be made.
The latter is hampered in case of transition metal chemistry by a lack of experimental data. Even when data are available, a straightforward comparison is not easy. Solvation effects play a role, as do entropic effects. Both effects need to be accounted for and present their own challenges. Gas-phase data are harder to obtain for transition metal catalysis. Likewise, the theoretical benchmarking is challenging since high-level theoretical results can often be unobtainable due to the large size of realistic catalytic systems. The design of accurate benchmark databases is an area of active development [13].
The recently developed domain-based local-pair natural orbital coupled-cluster singles, doubles and perturbative triples (DLPNO-CCSD(T)) method [14, 15] has led to a breakthrough in obtaining theoretical benchmark values for large systems. Though not as accurate as CCSD(T), the DLPNO-CCSD(T) method provides a good quality benchmark and, due to its lower computational cost, can be applied to systems with over a hundred atoms [9, 12, 16, 17]. (DLPNO-)CCSD(T) is accurate for a range of transition metals when used with careful attention to core correlation, relativistic effects and reference wavefunction [18]. Of particular difficulty for (DLPNO-)CCSD(T) are transition metal systems with significant static or non-dynamical correlation and one may have to resort to multireference or multiconfigurational methods [19,20,21]. Several diagnostics can be used to gauge the amount of multireference character [20].
The literature on benchmark studies is vast (see, for example, Refs. [18, 22,23,24,25,26]). Detailed studies were performed for many reactions, and much work has been devoted to building suitable test sets, but they are often limited to a particular step in the mechanism, such as the predissociation of a Grubbs catalyst [27]. In recent years, more studies emerged that include full reaction mechanisms [9]. It is important to include all steps of a mechanism in a benchmark study as the performance of a method may vary considerably across the mechanism depending on the balance of interactions involved in the species. For example, non-covalent interactions may play a much larger role in transition states than in the reactants or products and only by considering the performance of a method for all species is a good sense of the quality of the method obtained.
The workhorse for computational studies is density functional theory (DFT) [28]. It achieves a good balance between accuracy and efficiency and is able to yield good agreement with experiment [22, 29, 30]. Despite its great success and abundant presence, DFT has several issues when describing transition metal chemistry. With respect to energetics, it shows functional dependence [31] and offers no clear guidance to which functional to choose. Much effort is spent on designing better, all-round functionals. Several promising functionals emerge from the many validation studies performed, such as the dispersion corrected B97-D3(BJ) [32,33,34] functional or functionals of the Minnesota type [35, 36]. However, the vast offering of density functionals available today is bewildering. On the wavefunction side, perturbation methods offer a cost-effective alternative, but cannot be applied to small-gap systems and do not achieve the same accuracy for energies. Higher level theory, such as coupled-cluster methods, is much more accurate but also much more costly. There is thus a need for an efficient complement to DFT that provides accurate results. The random phase approximation (RPA) method for electronic ground state energies provides such an alternative.
Here, we study the performance of the random phase approximation (RPA) [37,38,39,40] for transition metal catalysis. The key question is whether RPA is a good candidate to predict the energy barriers involved in the mechanisms. In recent work, the performance of RPA for transition metal systems was studied [41]. In this study, it was found that RPA provides excellent structures and performs on par with the best DFT functionals while giving improved results over perturbation methods. The energetics studied were either on small model catalysts or on overall reaction energies. Full mechanisms were not included, which is a severe omission, since the quality of a method may differ for each step of a mechanism. For example, the change in electronic structure for a dissociation step involving bond breaking is significantly different from the change in electronic structure for a rearrangement and may be treated with unequal quality by a theoretical model. An important parameter is thus the consistency with which RPA performs across a reaction mechanism.
It is our aim in this study to extend the previous work and give a more complete picture of the performance of RPA for transition metal catalysis. We do this by focusing on large, realistic systems that are part of actual catalytic cycles. We investigate several reaction mechanisms that were previously studied computationally. We also study the performance of RPA for a dispersion-driven reaction. Since RPA includes dispersion from the outset, this provides a good testcase. We revisit the WCCR10 testset which we previously studied, because in the meantime we have obtained a high-quality theoretical benchmark for this set which sheds new light on the quality of RPA. This study is limited to closed-shell systems. This limits issues with multireference character which is often present in open-shell species. We use the DLPNO-CCSD(T) method as the main validation tool in this study. Solvation effects and entropic effects are ignored to make a direct assessment of the quality of the electronic energies of RPA. Where available, we use back-corrected experimental data to compare electronic energies with experiment. It is impossible to be comprehensive in this study. We selected a representative group of chemical reactions which will give a good impression of the performance of RPA. Transition metal catalysis is extremely varied, though, and this study is bound to be incomplete.
In this work, we use the so-called direct RPA method and we calculate the RPA energy from self-consistent Kohn–Sham orbitals. For details on the method, we refer to the literature [37, 38, 40, 42, 43]. The RPA includes dispersion interactions from the outset (in a Casimir–Polder consistent manner); it can be applied to small-gap systems and does not include empirical parameters. Its only dependence is on the choice of functional for the orbitals, but that dependence is small as long as the functional is of the GGA or hybrid type with a small amount of exact exchange [44,45,46]. The RPA implementation used in this work scales as \(N^4\log N\), where N is the system size. Several approaches have been published to reduce the scaling [47,48,49]. For some details on timings for transition metal systems, see Ref. [41].
2 Computational details
The DLPNO-CCSD(T) calculations were performed using the ORCA program system [50, 51]. Well converged SCF orbitals were obtained using the TightSCF setting. The cutoff parameter TCutPairs was set to \(1 \times 10^{-5}\). The RIJCOSX density fitting option was used for the SCF part. All other calculations were performed using the TURBOMOLE program package [52]. The RPA calculations use self-consistent Kohn–Sham orbitals obtained from the Perdew et al. [53] (PBE) functional or, if indicated, from the Tao et al. [54] (TPSS) functional using large integration grids (5) and tight convergence criteria (\(<10^{-7}\) for energy). Density fitting for Coulomb integrals was used for both DFT and RPA calculations. For all correlated single-point energy calculations, the core electrons were frozen using the default frozen core setting in TURBOMOLE (the nearest noble gas configuration). Integration grids of 60 points or more were used for RPA to ensure a sensitivity parameter smaller than \(10^{-5}\). (Spin-scaled) MP2 results were obtained using the ricc2 module in TURBOMOLE [55, 56].
Dunning’s correlation consistent basis sets [57] ((aug-)cc-pVXZ, \(X=\hbox {D, T, Q}\) or 5) were used in addition to the Karlsruhe def2- basis sets [58] (def2-TZVPP and def2-QZVPP). def2-QZVPP is used as the default for RPA calculations. It was shown to be efficient with a basis-set incompleteness error that is small compared to the inherent error of the RPA method [59]. For the coulomb fitting in DFT, the universal auxiliary basis set was used. The def2-QZVPP basis set was used for density fitting in MP2 and RPA. In ORCA, the def2/J auxiliary basis set was used; for correlation calculations the def2-TZVPP/C or def2-QZVPP/C setting were used based depending on the choice of basis set. For transition metals beyond the first row, relativistic effective core potentials were used to model the core electrons. For the Dunning basis sets, defpp-ecp [60] was used and ecp-28 [61] for the Karlsruhe basis sets.
The complete basis set (CBS) limit for RPA was obtained by extrapolation of the RPA correlation energies using the scheme by Helgaker et al. [62, 63]. The exact exchange energy contribution was taken from the calculation with the largest basis set and was assumed to be well converged with respect to basis set size.
3 Results
3.1 C–H and C–C bond activation by rhodium fragments
Evans and Jones [64] used DFT to study the ligand effects on the energetics of C–H and C–CN bond activation on acetonitrile by rhodium fragments. The rhodium fragments, which contain a \(\pi \)-acceptor ligand, first activate the C–H bond, followed by C–C activation. These fragments are one example of the successful activation of C–C bonds by first-row transition metal complexes reported in the past 15 years. Evans and Jones showed that the presence of a \(\sigma \)-donating ligand lowers the barrier for C–CN bond activation compared to \(\pi \)-acceptor ligands which do not show that effect. We use the stationary points on the potential energy surfaces found by Evans and Jones to benchmark RPA and compare its performance to other methods. Solvation effects were shown to be significant for these mechanisms. However, since our main interest is to compare the performance of RPA to other methods, we did not include these effects.
Figures 1, 2, and 3 show an overview of reaction energies for various methods for the C–H and C–C bond activation of acetonitrile by the fragment [CpRh(CNMe)], where Cp stands for C\(_5\)Me\(_5\) and Me for methyl. The species Cp-S3 is the intermediate connecting the C–C activated complex (Cp-S1) with the C–H activated complex (Cp-S5) through the transition states Cp-TS2 and Cp-TS4, respectively.
Relative energies (in kcal/mol) for C–C and C–H bond activation of acetonitrile by [CpRh(CNMe)] for dispersion corrected methods, RPA, and DLPNO-CCSD(T). The D3 dispersion correction was used in conjunction with Becke–Johnson damping (D3(BJ)) [33, 34, 65]. RPA energies were obtained using PBE orbitals. The def2-QZVPP basis set was used for all methods. Energies are relative to complex Cp-S1
Basis set convergence and functional dependence of RPA relative energies (in kcal/mol) for C–C and C–H bond activation of acetonitrile by [CpRh(CNMe)]. DLPNO-CCSD(T) results (obtained using the def2-QZVPP basis set) are included as a reference. The RPA values were obtained using self-consistent PBE and TPSS orbitals as indicated. All energies are relative to complex (Cp-S1). \(\hbox {AV}X\hbox {Z}\) stands for aug-cc-pVXZ, where \(X = \hbox {Q}\) or 5, and QZVPP stands for def2-QZVPP
The methods presented show a wide variety of performance, which is most distinct for Cp-S3 where the overall range is about 15 kcal/mol. This observation highlights the necessity of benchmarking the performance of methods for transition metal chemistry. One would reach very different conclusions depending on the method one chose. Figure 1 shows results for the hybrid functional B3-LYP, the hybrid meta-GGA functional TPSSH and the Minnesota hybrid functional M06-2X. In addition, results are presented for the DLPNO-CCSD(T) method, which has been advocated as good benchmark in lieu of CCSD(T) results. Evans and Jones used B3-LYP in their work. To be consistent with basis sets, the B3-LYP results presented in Fig. 1 were reproduced for this work. The Minnesota functional M06-2X shows similar performance to B3-LYP. Both functionals stabilize the intermediate complex Cp-S3 considerably compared to the other methods. TPSSH, in contrast, yields a higher energy for the Cp-S3 and for Cp-TS4 and is much closer to the DLPNO-CCSD(T) reference. RPA performs quite similar to TPSSH but shows somewhat better agreement with the reference. All methods predict the same qualitative energy landscape, but there are large variations in relative energies. Figure 2 shows results for dispersion corrected functionals using the Grimme-type D3 correction with Becke-Johnson damping (BJ). The dispersion correction has relatively little effect on the energies, but tends to yield higher relative energies and thus better agreement with the DLPNO-CCSD(T) reference. The TPSSH-D3(BJ) functional in particular shows good agreement and is of similar quality to RPA. The largest effect of the dispersion correction is observed for species CP-S3 with a change of relative energy of 2.3 kcal/mol and 2.2 kcal/mol for TPSSH and B3-LYP, respectively.
Also presented are results for RPA, MP2 and spin-component-scaled MP2 (SCS-MP2) [66] (see Fig. 3). Results for scaled opposite-spin MP2 (SOS-MP2) [67] are omitted as they are very similar to SCS-MP2. MP2 does not predict a stable intermediate and has barrierless transitions to both the C–C and C–H activated complex. It thus shows a qualitatively different picture. The SCS-MP2 method is in better agreement with DLPNO-CCSD(T), but also does not show a barrier to the C–H activated complex.
Compared to the DLPNO-CCSD(T) method, all methods underestimate next to all relative energies. Of the presented methods, RPA and TPSSH(-D3(BJ)) give the same qualitative picture as the benchmark and show the smallest deviation, whereas M06-2X deviates most. The large variation in energy values for the different methods is striking. For example, the relative energy for Cp-S5 shows a range of about 4 kcal/mol, whereas for Cp-S3 the range is about 13 kcal/mol.
Finally, Fig. 4 demonstrates that the basis set incompleteness error is small for RPA when using quadruple zeta or quintuple zeta quality basis sets. The deviation from DLPNO-CCSD(T) is therefore not a basis-set issue, but a reflection on the quality of the method. Also, the choice of functional for the Kohn–Sham orbitals that serve as input for RPA has little effect as is evident from the small difference in results obtained based on PBE or TPSS orbitals.
3.2 Ruthenium-catalyzed olefin metathesis
Minenkov et al. [68] provided a complete reaction pathway of ruthenium-catalyzed olefin metathesis of ethyl vinyl ether using DFT and compared their findings to experimental kinetic data obtained by Sanford et al. [69]. Olefin metathesis has become a widely used mechanism to form carbon–carbon bonds, largely due to the development of first- and second-generation Grubbs catalysts [70, 71] and has been subject of much computational research [72]. Complete reaction pathways were presented for seven such catalysts by Minenkov et al. using a range of density functionals. It is evident from their study and from previous work [25, 27, 73] that weak, non-covalent interactions play a significant role in the catalytic cycle, particularly for transition states as they involve weakly interacting fragments. A computational method that accounts for dispersion is thus required to study ruthenium-catalyzed olefin metathesis. Since the amount of dispersion interactions varies across the mechanism, it is important to study the complete mechanism, rather than just one step, to obtain insight in the quality of a method. Here, we compare the performance of RPA for the reaction pathways to density functional theory and wavefunction methods. We only study the mechanism for catalyst 1 from the work of Minenkov and coworkers [68] which is a first-generation Grubbs catalyst (see Fig. 5).
Reaction energies (kcal/mol) for the reaction of the ruthenium precatalyst with EVE for DFT methods and RPA compared to the DLPNO-CCSD(T) method. All values are relative to the precatalyst P. The def2-QZVPP basis set was used for RPA. DLPNO-CCSD(T) results were obtained with the def2-TZVP basis set and DFT results with the def2-TZVPP basis set. RPA results were obtained using PBE orbitals
Reaction energies (kcal/mol) for the reaction of the ruthenium precatalyst with EVE for MP2 methods and RPA compared to the DLPNO-CCSD(T) method. All values are relative to the precatalyst P. The def2-QZVPP basis set was used for RPA and MP2. DLPNO-CCSD(T) results were obtained with the def2-TZVP basis set. RPA results were obtained using PBE orbitals
Figures 6 and 7 show electronic energy differences for the reaction of the ruthenium precatalyst with the olefin ethyl vinyl ether (EVE). All energies are given relative to the energy of the precatalyst (P). The optimized structures obtained by Minenkov and coworkers [68] were used for all calculations. The structures were optimized using the PBE functional which was shown to perform well for transition metal catalysis [25]. The first step of the reaction (P to AC1) is the dissociation of the catalyst in a 14-electron active catalyst and phosphine (\(\hbox {PCy}_{3}\)). The phosphine does not play a further role in the mechanism. The activated catalyst proceeds to bind to EVE to form a \(\pi \)-complex (AC1 to PC1) which in turn will undergo cycloaddition to form metallacyclobutane (PC1 to MCB). Next, the ring in metallacyclobutane opens to yield a styrene \(\pi \)-compound (MCB to PC2) followed by a final step in which the styrene decoordinates and an active complex is left (PC2 to AC2). Each step has its own transition state (TS). Entropic and solvation effects play a significant role in the energy profile. Because they are not included in the results shown in Figs. 6 and 7, some of the steps have transition states that are not energetic maxima, most notably for TS1 which is the transition state for the dissociation of the precatalyst. A small energy barrier was found on the potential energy surface [68]; the free energy barrier on the other hand is very distinct and in the order of 20 kcal/mol. This demonstrates the significance of entropic and solvation effects, which apparently stabilize AC1 to a much larger extent than either P or TS1 [73].
Whether or not the transition states are true transition states for the method studied is not addressed in this work. It may well be that for some methods some steps are barrierless on the potential energy surface; for instance, using B3-LYP no barrier is found for the predissociation step [74]. Since we take the structures from Minenkov and coworkers as our reference point, we include all transition states for all methods.
It is clear from Fig. 6 that a wide range of energy values is observed for the methods studied. For AC1 the range is over 30 kcal/mol which is of the same order of magnitude as the calculated free energy of AC1 relative to P. PBE and B3-LYP yield relative energies that are significantly lower than RPA for all points. The addition of a dispersion correction has a dramatic effect and increases the relative energies by more than a factor of two (see results for B3-LYP-D3) yielding energies that are now significantly higher than RPA for most points. The inclusion of dispersion qualitatively changes the reaction profile, in particular for the activated complex (AC1) which becomes a distinct maximum. The dramatic effect of the dispersion correction directly shows the importance of non-covalent interactions in this reaction.
The dispersion corrected results differ considerably from the RPA results, for some points by more than 10 kcal/mol. A distinct difference is the description of PC1 which for B3-LYP-D3 is higher in energy than TS2, whereas it is lower in energy for RPA and DLPNO-CCSD(T). All species involving EVE (TS2 through PC2) have a much higher relative energy when using B3-LYP-D3. Such a difference will considerably impact calculations based on this reaction profile, such as the calculation of reaction rates. Though it is obvious that weak interactions cannot be neglected for this reaction, there is still a large range of relative energies observed for the methods that include dispersion.
For this reaction, MP2 compares much more favorably to RPA. The general shape of the reaction profiles are the same except for MCB and TS4, where MP2 predicts much lower energies than RPA. Interestingly, in contrast to what was seen in Fig. 3, using SCS-MP2 does not make much of a difference.
As a benchmark, results from DLPNO-CCSD(T) are included in Figs. 6 and 7. At this point, only results obtained with the def2-TZVP basis set are available, but this is not a problem as it was shown by Minenkov et al. that good quality results are obtained at the triple-zeta level [16] and the remaining basis set incompleteness error is thus not expected to change the results significantly. RPA agrees best with the benchmark; it reproduces the energy profile qualitatively and is within 5 kcal/mol of the benchmark for each step in the mechanism. SCS-MP2 improves somewhat upon MP2 and is mostly in good agreement but underestimates the energies of MCB and TS4. B3-LYP and PBE fail to qualitatively describe the first part of the mechanism (predissociation and olefin insertion), whereas the dispersion corrected B3-LYP-D3 method gives a qualitatively correct picture (except for PC1), but yields energies that are too high for many steps.
To give an impression of computational effort involved to obtain the presented results, we compare wall times for the P precatalyst which consists of 120 atoms (see Table 1). DFT with the B3-LYP hybrid functional, which formally scales as \(N^4\) with system size N, is the most efficient with a wall time of 2 hours. RPA scales as \(N^4 \log N\) [75] and is about 5 times more time consuming than B3-LYP for this example. CCSD(T) has a formal scaling of \(N^7\) and is intractable for this problem even with the smaller def2-TZVP basis set. The DLPNO-CCSD(T) method is much more efficient than CCSD(T). It achieves asymptotic linear scaling with system size [76, 77] but has a considerable prefactor resulting, in this case, in a wall time of 42 hours with the smaller def2-TZVP basis set.
3.3 The WCCR10 test set
The WCCR10 set was compiled by Weymuth and Reiher [78] as a ligand dissociation energy database of large cationic transition metal complexes which mimic complexes used in actual catalytic cycles. The set contains 10 reactions using a variety of transition metals and complexes ranging in size from 42 to 174 atoms. Several theoretical methods were compared to dissociation energies obtained from mass spectrometry. We showed that RPA performed reasonably well for this set with a mean deviation of about 7 kcal/mol [41]. Here, we revisit the WCCR10 and rather than comparing to experiment we use the DLPNO-CCSD(T) method as our theoretical benchmark. By doing so, we lose the direct connection to experiment, but also eliminate any error associated with correcting the gas-phase zero kelvin computational results for zero-point vibrational energy and finite-temperature effects. The results are presented in Table 2.
The high-level DLPNO-CCSD(T) method is not in good agreement with experiment. The average absolute deviation is 7.0 kcal/mol and in particular reactions 4 and 6 shows a large discrepancy between experiment and computation (about 18 kcal/mol). This disagreement highlights the challenge in transition metal chemistry to make a good comparison with experiment. Recently, Reiher and coworkers commented extensively on this discrepancy [79]. Two reactions (4 and 9) showed some measure of multireference character as indicated by the \(Z_s(1)\) diagnostic and may therefore not be described well by single-reference methods. However, the remaining eight reactions involve single-configurational complexes according to the same diagnostic. The source for the difference with experiment remains unclear and cannot be accounted for at the moment. Reiher et al. concluded that the agreement of DFT with coupled-cluster data increased significantly upon inclusion of a dispersion correction [79], thereby highlighting the fact that dispersion effects are crucial in describing these reactions.
In light of these observations, it seems to be the best strategy, for the time being, to compare methods to DLPNO-CCSD(T) to assess their potential. Therefore, Table 2 also includes deviations of RPA, PBE0 and MP2 methods from DLPNO-CCSD(T). RPA has a maximum deviation of 3.0 kcal/mol, an average deviation of 0.0 kcal/mol and an average absolute deviation of 1.6 kcal/mol. Compared to DLPNO-CCSD(T), RPA shows excellent performance across the set of reactions, with none of the reactions standing out as yielding qualitatively different results. RPA is thus in good agreement with the DLPNO-CCSD(T) method, whereas both methods show significant deviation with the experimental values. It could be that both single-reference methods fail to capture an essential element necessary to describe this set of reactions or that the comparison to experiment is hampered otherwise. Both PBE0 and MP2 deviate much more from the benchmark. PBE0 underbinds on average by 10.9 kcal/mol, MP2 overbinds on average by 6.8 kcal/mol.
Mean deviations (MD), mean absolute deviations (MAD) and maximum deviations (Max) for the WCCR10 test set compared to DLPNO-CCSD(T) values for various methods. The DLPNO-CCSD(T) results were obtained using the cc-pVQZ(-PP) basis set. RPA results were obtained using self-consistent PBE orbitals and def2-QZVPP basis set. PBE structures taken from Weymuth et al.[78] were used. Results other than RPA were taken from Ref. [78]
Figure 8 compares the performance of several density functionals with and without dispersion correction to DLPNO-CCSD(T). These methods were previously compared to the back-corrected experimental results [41, 78]. The density functionals BP-86, B3-LYP, TPSS and TPSSH perform more or less similarly and yield mean absolute deviations of more than 10 kcal/mol with absolute maximum deviations of up to 30 kcal/mol (for reaction 4). Addition of a Grimme-type dispersion correction reduces the absolute deviation to about 5 kcal/mol and the maximum error to about 10 kcal/mol. The RPA method stands out for its excellent performance and can be concluded to be the method with the best agreement with the theoretical benchmark.
3.4 \(\mu \)-chloride-bridged palladacyclic dimer
Non-covalent interactions often play a large stabilizing role in larger transition metal complexes. The correct inclusion of these weak interactions is a challenge for theoretical chemistry. A representative example of a dispersion-driven transition metal reaction was studied by Hansen et al. [12]. They presented results for a range of density functionals with and without dispersion correction and several wavefunction-based methods for the reaction of a \(\mu \)-chloride-bridged palladacyclic dimer with phosphane ligands (\(\hbox {PR}_3\)) to form monopalladium products. Two ligands were used, namely phenyl or cyclohexyl. The dimer is stabilized by the bridging chlorido ligands forming a labile Cl-Pd bond which is easily quenched by a phosphane ligand (see Fig. 9). The reaction is an example of an elementary ligand coordination reaction. The experimental reaction enthalpy was obtained using isothermal titration calorimetry. Computed reaction energies were compared to theoretically back-corrected experimental reaction energies. The experimental reaction enthalpies have an error of about 1 kcal/mol. The back-corrected 0K gas-phase reaction energies have an associated error of 3 kcal/mol; the increase reflects the uncertainty related to the methods used for the back-correction. Hansen et al. conclude that dispersion-corrected functionals (such as PW6B95-D3(BJ) or B3-LYP-NL) or the DLPNO-CCSD(T) method are able to give energies within 3 kcal/mol of the experimental value. Table 3 gives an overview of the performance of RPA for this system compared to other methods.
The monopalladium complex formed in reaction with \(\hbox {PPh}_{3}\) has stronger \(\pi {-}\pi \) and CH–\(\pi \) dispersion interaction than the complex formed in reaction with \(\hbox {PCy}_{3}\). MP2 fails to distinguish between the two reactions and strongly overestimates the dispersion interactions resulting in a reaction energy that is about 20 kcal/mol too negative. HF, as expected, fails to describe the reaction energies. Addition of the D3 correction gives very good agreement with experiment and is a dramatic demonstration of the importance of the role of weak interactions in this reaction. The recent DLPNO-CCSD(T) method gives good agreement with experiment and is recommended as a reliable benchmark when no experimental results are available. On the DFT side, the best functional is the PW6B95 functional with D3 correction. As is evident from this overview, the best methods that can be applied to such large systems yield an error of about 2–3 kcal/mol and do not reach the so-called chemical accuracy which is defined as errors smaller than 1 kcal/mol. However, for transition metal complexes these are relatively small errors and a big improvement upon what was possible only a few years ago.
The RPA results are also given in Table 3. Results are presented for various basis sets and when extrapolated to the complete basis set limit. Overall, the RPA performance is very good. The parameter-free method is able to correctly predict the relative energies of the two reactions and also gives energies that are in good agreement with experiment. When using the def2-QZVPP basis set, RPA yields results that are very close to experiment. Results vary somewhat with basis set. Using an augmented basis set overestimates the binding energy. Extrapolation to the complete basis set limit gives reaction energies of − 35.8 kcal/mol and − 34.4 kcal/mol, respectively. These RPA binding energies are about 2 kcal/mol larger in magnitude than the DLPNO-CCSD(T) energies, a result that is very similar to what is observed for the WCCR10 set. In comparison to the DFT methods studied, RPA is closest to PW6B95-D3(BJ).
4 Conclusion and discussion
In this work, the performance of the direct RPA method was evaluated for reaction mechanisms relevant in transition metal catalysis and for reaction energies involving large transition metal complexes. Comparison was made to DFT and wavefunction methods, and the DLPNO-CCSD(T) method was used as a theoretical benchmark. Two mechanisms were studied: one where dispersion effects are minor and one where they are very significant. In addition, the WCCR10 test set of reactions was revisited and the dispersion-driven reaction of a palladacyclic dimer was studied.
For all reactions studied, RPA gives results that are closest to DLPNO-CCSD(T) with an average deviation of 2–3 kcal/mol and a maximum deviation of about 5 kcal/mol. For the mechanisms studied, RPA is consistently close to the DLPNO-CCSD(T) method for all steps of the mechanism and is thus able to give a balanced description of the entire reaction mechanism. When revisiting the WCCR10 set, RPA is shown to have the smallest deviation compared to the theoretical benchmark. This is in contrast to the previously published results which made the comparison to experimental results. The discrepancy with experiment remains unresolved. For the large dispersion-driven palladacyclic dimer reaction RPA is able to reproduce the experimentally observed relative energy ordering as well as producing reaction energies that are within 2–3 kcal/mol of the experimental values. In all, we conclude that RPA is able to represent reaction mechanisms including transition metals with an accuracy that is comparable to the best dispersion-corrected functionals. The advantage of RPA is that no choice of functional needs to be made.
Basis sets of at least quadruple zeta quality are required for RPA. From the basis set convergence results presented, we can conclude that the basis set incompleteness error when using quadruple-zeta size basis sets is not dominant. As shown for the palladacyclic dimer, the def2-QZVPP basis set yields results that are in good agreement with the complete basis set limit. As shown in earlier work, [59], the Karlsruhe def2- basis sets of quadruple zeta size strike a good balance between accuracy and efficiency.
MP2 and SCS/SOS-MP2 are often able to represent transition metal chemistry quite well, though the deviation from DLPNO-CCSD(T) is on average larger and less consistent. For the DFT methods, the inclusion of a dispersion correction is crucial. For all functionals studied, the deviation from DLPNO-CCSD(T) was less systematic than for RPA and the size of the error thus depends on which step of the mechanism is studied. Good agreement can be obtained, but depends on the choice of functional.
In conclusion, RPA can serve as an independent method that can be used complementary to dispersion-corrected DFT to obtain a more complete picture of transition metal chemistry.
References
Khan MS, Haque A, Al-Suti MK, Raithby PR (2015) Recent advances in the application of group-10 transition metal based catalysts in C–H activation and functionalization. J Organomet Chem 793:114–133
Davies HML, Morton D (2016) Recent advances in CH functionalization. J Org Chem 81:343–350
Dong G, Cramer N (2016) C–C bond activation. Springer, Berlin
Stang PJ, Diederich F (2008) Metal-catalyzed cross-coupling reactions. Wiley, New York
Ananikov VP (2015) Nickel: the spirited horse of transition metal catalysis. ChemInform 46:1964–1971
Rosen BM, Quasdorf KW, Wilson DA, Zhang N, Resmerita A-M, Garg NK, Percec V (2010) Nickel-catalyzed cross-couplings involving carbon–oxygen bonds. Chem Rev 111:1346–1416
Omer HM, Liu P (2017) Computational study of Ni-catalyzed C–H functionalization: factors that control the competition of oxidative addition and radical pathways. J Am Chem Soc 139:9909–9920
Torrent M, Sola M, Frenking G (2000) Theoretical studies of some transition-metal-mediated reactions of industrial and synthetic importance. Chem Rev 100:439–494
Cheng G-J, Zhang X, Chung LW, Xu L, Wu Y-D (2015) Computational organic chemistry: bridging theory and experiment in establishing the mechanisms of chemical reactions. J Am Chem Soc 137:1706–1725
Pidko EA (2017) Toward the balance between the reductionist and systems approaches in computational catalysis: model versus method accuracy for the description of catalytic systems. ACS Catal 7:4230–4234
Plata RE, Singleton DA (2015) A case study of the mechanism of alcohol-mediated Morita Baylis-Hillman reactions. The importance of experimental observations. J Am Chem Soc 137:3811–3826
Hansen A, Bannwarth C, Grimme S, Petrović P, Werlé C, Djukic J-P (2014) The thermochemistry of London dispersion-driven transition metal reactions: getting the right answer for the right reason. ChemistryOpen 3:177–189
Aoto YA, de Lima Batista AP, Köhn A, de Oliveira-Filho AG (2017) How to arrive at accurate benchmark values for transition metal compounds: Computation or experiment? J Chem Theory Comput 13:5291–5316
Riplinger C, Neese F (2013) An efficient and near linear scaling pair natural orbital based local coupled cluster method. J Chem Phys 138:034106
Riplinger C, Sandhoefer B, Hansen A, Neese F (2013) Natural triple excitations in local coupled cluster calculations with pair natural orbitals. J Chem Phys 139:134101
Minenkov Y, Chermak E, Cavallo L (2015) Accuracy of DLPNO-CCSD (T) method for noncovalent bond dissociation enthalpies from coinage metal cation complexes. J Chem Theory Comput 11:4664–4676
Paulechka E, Kazakov A (2017) Efficient DLPNO-CCSD (T)-based estimation of formation enthalpies for C–, H–, O–, and N-containing closed-shell compounds validated against critically evaluated experimental data. J Phys Chem A 121:4379–4387
Minenkov Y, Chermak E, Cavallo L (2016) Troubles in the systematic prediction of transition metal thermochemistry with contemporary out-of-the-box methods. J Chem Theory Comput 12:1542–1560
Phung QM, Feldt M, Harvey JN, Pierloot K (2018) Toward highly accurate spin state energetics in first-row transition metal complexes: a combined CASPT2/CC approach. J Chem Theory Comput 14:2446–2455
Jiang W, Laury ML, Powell M, Wilson AK (2012) Comparative study of single and double hybrid density functionals for the prediction of 3D transition metal thermochemistry. J Chem Theory Comput 8:4102–4111
Wang J, Manivasagam S, Wilson AK (2015) Multireference character for 4d transition metal-containing molecules. J Chem Theory Comput 11:5865–5872
Cramer CJ, Truhlar DG (2009) Density functional theory for transition metals and transition metal chemistry. Phys Chem Chem Phys 11:10757–10816
Balcells D, Clot E, Eisenstein O (2010) C–H bond activation in transition metal species from a computational perspective. Chem Rev 110:749–823
Harvey JN (2006) On the accuracy of density functional theory in transition metal chemistry. Ann Rep Sect “c” (Phys Chem) 102:203–226
Minenkov Y, Occhipinti G, Heyndrickx W, Jensen VR (2012) The nature of the barrier to phosphane dissociation from Grubbs olefin metathesis catalysts. Eur J Inorg Chem 2012:1507–1516
Chan B, Ball GE (2013) A benchmark Ab initio and DFT study of the structure and binding of methane in the \(\sigma \)-alkane complex CpRe (CO)\(_2\) (CH\(_4\)). J Chem Theory Comput 9:2199–2208
Minenkov Y, Occhipinti G, Jensen VR (2009) Metal–phosphine bond strengths of the transition metals: a challenge for DFT. J Phys Chem A 113:11833–11844
Becke AD (2014) Perspective: fifty years of density-functional theory in chemical physics. J Chem Phys 140:18A301
Rohmann K, Hölscher M, Leitner W (2015) Can contemporary density functional theory predict energy spans in molecular catalysis accurately enough to be applicable for in silico catalyst design? A computational/experimental case study for the ruthenium-catalyzed hydrogenation of olefins. J Am Chem Soc 138:433–443
Qu Z-W, Hansen A, Grimme S (2015) Co–C bond dissociation energies in cobalamin derivatives and dispersion effects: Anomaly or just challenging? J Chem Theory Comput 11:1037–1045
Moltved KA, Kepp KP (2018) Chemical bond energies of 3d transition metals studied by density functional theory. J Chem Theory Comput 14(7):3479–3492
Grimme S (2006) Semiempirical hybrid density functional with perturbative second-order correlation. J Chem Phys 124:034108
Grimme S, Antony J, Ehrlich S, Krieg H (2010) A consistent and accurate ab initio parametrization of density functional dispersion correction (DFT-D) for the 94 elements H-Pu. J Chem Phys 132:154104
Becke AD, Johnson ER (2005) A density-functional model of the dispersion interaction. J Chem Phys 123:154101
Mardirossian N, Head-Gordon M (2016) How accurate are the Minnesota density functionals for noncovalent interactions, isomerization energies, thermochemistry, and barrier heights involving molecules composed of main-group elements? J Chem Theory Comput 12:4303–4325
Zhao Y, Truhlar DG (2011) Applications and validations of the Minnesota density functionals. Chem Phys Lett 502:1–13
Heßelmann A, Görling A (2011) Random-phase approximation correlation methods for molecules and solids. Mol Phys 109:2473–2500
Eshuis H, Bates JE, Furche F (2012) Electron correlation methods based on the random phase approximation. Theor Chem Acc 131:1–18
Ren X, Rinke P, Joas C, Scheffler M (2012) Random-phase approximation and its applications in computational chemistry and materials science. J Mater Sci 47:7447–7471
Chen GP, Voora VK, Agee MM, Balasubramani SG, Furche F (2017) Random-phase approximation methods. Ann Rev Phys Chem 68:421–445
Waitt C, Ferrara NM, Eshuis H (2016) Thermochemistry and geometries for transition-metal chemistry from the random phase approximation. J Chem Theory Comput 12:5350–5360
Mussard B, Rocca D, Jansen G, Ángyán JG (2016) Dielectric matrix formulation of correlation energies in the random phase approximation: inclusion of exchange effects. J Chem Theory Comput 12:2191–2202
Heßelmann A (2017) Non-covalent interactions in quantum chemistry and physics. Elsevier, Amsterdam, pp 65–136
Janesko BG, Henderson TM, Scuseria GE (2009) Long-range-corrected hybrid density functionals including random phase approximation correlation: application to noncovalent interactions. J Chem Phys 131:034110
Olsen T, Thygesen KS (2012) Extending the random-phase approximation for electronic correlation energies: the renormalized adiabatic local density approximation. Phys Rev B 86:081103
Bates J, Mezei P, Csonka G, Sun J, Ruzsinszky A (2016) Reference determinant dependence of the random phase approximation in 3d transition metal chemistry. J Chem Theory Comput 13:100–109
Luenser A, Schurkus HF, Ochsenfeld C (2017) Vanishing-overhead linear-scaling random phase approximation by Cholesky decomposition and an attenuated Coulomb-metric. J Chem Theory Comput 13:1647–1655
Kállay M (2015) Linear-scaling implementation of the direct random-phase approximation. J Chem Phys 142:204105
Kaltak M, Klimeš J, Kresse G (2014) Cubic scaling algorithm for the random phase approximation: self-interstitials and vacancies in Si. Phys Rev B 90:054115
Neese F (2012) The ORCA program system. Wiley Interdiscip Rev Comput Mol Sci 2:73–78
Neese F (2018) Software update: the ORCA program system, version 4.0. Wiley Interdiscip Rev Comput Mol Sci 8:e1327
TURBOMOLE V7.2 (2017) A development of University of Karlsruhe and Forschungszentrum Karlsruhe GmbH, 1989–2007, TURBOMOLE GmbH, since 2007. http://www.turbomole.com. Accessed 2 Aug 2018
Perdew JP, Burke K, Ernzerhof M (1996) Generalized gradient approximation made simple. Phys Rev Lett 77:3865
Tao J, Perdew JP, Staroverov VN, Scuseria GE (2003) Climbing the density functional ladder: nonempirical meta-generalized gradient approximation designed for molecules and solids. Phys Rev Lett 91:146401
Hättig C, Weigend F (2000) CC2 excitation energy calculations on large molecules using the resolution of the identity approximation. J Chem Phys 113:5154–5161
Hättig C (2003) Geometry optimizations with the coupled-cluster model CC2 using the resolution-of-the-identity approximation. J Chem Phys 118:7751–7761
Dunning TH Jr (1989) Gaussian basis sets for use in correlated molecular calculations. I. The atoms boron through neon and hydrogen. J Chem Phys 90:1007–1023
Weigend F, Ahlrichs R (2005) Balanced basis sets of split valence, triple zeta valence and quadruple zeta valence quality for H to Rn: design and assessment of accuracy. Phys Chem Chem Phys 7:3297–3305
Eshuis H, Furche F (2012) Basis set convergence of molecular correlation energy differences within the random phase approximation. J Chem Phys 136:084105
Peterson KA, Figgen D, Dolg M, Stoll H (2007) Energy-consistent relativistic pseudopotentials and correlation consistent basis sets for the 4d elements Y-Pd. J Chem Phys 126:124101
Andrae D, Haeussermann U, Dolg M, Stoll H, Preuss H (1990) Energy-adjusted ab initio pseudopotentials for the second and third row transition elements. Theor Chim Acta 77:123–141
Helgaker T, Klopper W, Koch H, Noga J (1997) Basis-set convergence of correlated calculations on water. J Chem Phys 106:9639–9646
Halkier A, Helgaker T, Jørgensen P, Klopper W, Koch H, Olsen J, Wilson AK (1998) Basis-set convergence in correlated calculations on Ne, N\(_2\), and H\(_2\)O. Chem Phys Lett 286:243–252
Evans ME, Jones WD (2011) Controlling the selectivity for C–H and C–CN bond activation at rhodium: A DFT examination of ligand effects. Organometallics 30:3371–3377
Grimme S, Ehrlich S, Goerigk L (2011) Effect of the damping function in dispersion corrected density functional theory. J Comput Chem 32:1456–1465
Grimme S (2003) Improved second-order Møller–Plesset perturbation theory by separate scaling of parallel-and antiparallel-spin pair correlation energies. J Chem Phys 118:9095–9102
Jung Y, Lochan RC, Dutoi AD, Head-Gordon M (2004) Scaled opposite-spin second order Møller–Plesset correlation energy: an economical electronic structure method. J Chem Phys 121:9793–9802
Minenkov Y, Occhipinti G, Jensen VR (2013) Complete reaction pathway of ruthenium-catalyzed olefin metathesis of ethyl vinyl ether: kinetics and mechanistic insight from DFT. Organometallics 32:2099–2111
Sanford MS, Love JA, Grubbs RH (2001) Mechanism and activity of ruthenium olefin metathesis catalysts. J Am Chem Soc 123:6543–6554
Trnka TM, Grubbs RH (2001) The development of L2X2Ru CHR olefin metathesis catalysts: an organometallic success story. Acc Chem Res 34:18–29
Vougioukalakis GC, Grubbs RH (2009) Ruthenium-based heterocyclic carbene-coordinated olefin metathesis catalysts. Chem Rev 110:1746–1787
Liu P, Taylor BL, Garcia-Lopez J, Houk KN (2015) Computational studies of ruthenium-catalyzed olefin metathesis. Wiley, New York
Minenkov Y, Singstad Å, Occhipinti G, Jensen VR (2012) The accuracy of DFT-optimized geometries of functional transition metal compounds: a validation study of catalysts for olefin metathesis and other reactions in the homogeneous phase. Dalton Trans 41:5526–5541
Tsipis AC, Orpen AG, Harvey JN (2005) Substituent effects and the mechanism of alkene metathesis catalyzed by ruthenium dichloride catalysts. Dalton Trans 17:2849–2858
Eshuis H, Yarkony J, Furche F (2010) Fast computation of molecular random phase approximation correlation energies using resolution of the identity and imaginary frequency integration. J Chem Phys 132:234114
Rolik Z, Szegedy L, Ladjánszki I, Ladóczki B, Kállay M (2013) An efficient linear-scaling CCSD (T) method based on local natural orbitals. J Chem Phys 139:094105
Guo Y, Riplinger C, Becker U, Liakos DG, Minenkov Y, Cavallo L, Neese F (2018) Communication: an improved linear scaling perturbative triples correction for the domain based local pair-natural orbital based singles and doubles coupled cluster method [DLPNO-CCSD (T)]. J Chem Phys 148:011101
Weymuth T, Couzijn EP, Chen P, Reiher M (2014) New benchmark set of transition-metal coordination reactions for the assessment of density functionals. J Chem Theory Comput 10:3092–3103
Husch T, Freitag L, Reiher M (2018) Calculation of ligand dissociation energies in large transition-metal complexes. J Chem Theory Comput 14:2456–2468
Acknowledgements
This work was supported by the National Science Foundation (Grants CHE-1464960 and CNS-1625636).
Author information
Authors and Affiliations
Corresponding author
Additional information
Published as part of the special collection of articles In Memoriam of János Ángyán.
Rights and permissions
About this article
Cite this article
Chedid, J., Ferrara, N.M. & Eshuis, H. Describing transition metal homogeneous catalysis using the random phase approximation. Theor Chem Acc 137, 158 (2018). https://doi.org/10.1007/s00214-018-2369-y
Received:
Accepted:
Published:
DOI: https://doi.org/10.1007/s00214-018-2369-y