Abstract
Satellite remote sensing technologies are currently tested and suggested as a tool in REDD+ (MRV, Measurement Reporting, and Verification). SAR (Synthetic Aperture Radar) has got an extensive application in the estimation of biomass due to its all-weather capabilities. L band radar signals penetrate the canopy more efficiently when compared to C band. Scientific biomass study using SAR has not been conducted in Para in spite of extensive field datasets being freely available under CMS (Carbon Monitoring System) project. This study aims in using various polarization combinations like HH + HV, HH − HV, HH + HV/HH − HV and vegetation index such as NDVI from the optical data. ALOS-PALSAR and Landsat 7 data acquired over Paragominas in Brazil, where field samples were collected in the form of transects. Regression analysis was performed using backscatter coefficients and field collected Above Ground Biomass (AGB). Semi-empirical model was developed to model AGB using various polarization combinations and NDVI as predictor variables. Combination gave higher R2 value of 0.657 for biomass prediction. Multiple linear regression using NDVI and HH + HV as variables yielded R2 of 0.73 during calibration and 0.363 during validation. There is future scope to use other vegetation indices such as RVI, EVI, etc., along with increased number of samples, which may yield more robust models with acceptable level of accuracy for practical application.
Access provided by Autonomous University of Puebla. Download conference paper PDF
Similar content being viewed by others
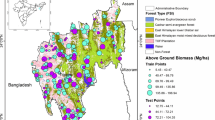
Keywords
1 Introduction
Earlier, collection of biophysical parameters for the forest inventory was tiring and time-consuming task. Comprehension of global C cycle is important in estimation of global terrestrial biomass to eliminate uncertainty [6]. Advent of remote sensing has made it possible to estimate above ground biomass by retrieving information from images acquired by satellites. Synthetic Aperture Radar (SAR) acquisitions are independent of cloud cover, weather and light conditions. SAR images are acquired using both airborne and space-borne platforms in various wavelength bands such as X, C, L, and P. Another Important advantage of using radar image is its penetration capability. L band radar signals penetrate the canopy more efficiently when compared to C band. And L band has performed considerably well in retrieving biomass when compared to C Band [3, 7, 9]. Moreover, SAR plays an important role in forest observation [15]. It was demonstrated that the sensitivity of SAR polarimetry is depending on the structure, density, and tree elements (i.e., trunk/stem, branches, and leaves) of the forests. Although there have been many biomass estimation studies conducted all over the world, there are limited studies done in estimating biomass using synergy of Optical and SAR images. Deforestation has cleared about 15% of the extensive forest on the Brazilian Amazon frontier. In response to the potential climatic effects of deforestation, policy makers have suggested reductions in emissions through deforestation and forest degradation and enhanced forest carbon stocks (REDD+) [14]. Application of different SAR and optical techniques in estimation of biomass will help in understanding the forest management ecosystem [10]. Combination of optical and SAR data has exhibited increase in accuracy level of biomass estimation [5]. This study was done using freely available datasets, as ALOS-2 datasets are expensive to procure.
The sensitivity of backscatter and saturation depends on site conditions and structure of forest [8, 11]. The frequency of SAR is directly proportional to the depth of wave penetration, which means that shorter wavelength can only penetrate the forest for a few centimeters, while longer wavelength can penetrate deeper and sometimes can interact with forest floor [8]. In addition to this, weather conditions may also influence dielectric properties of vegetation and soil surface [6]. The scattering and attenuation of signal depend on stand features, frequency, incidence angle, etc. InSAR is one more emerging technique to estimate biomass [13]. The present study aims to establish a relationship between ABG using SAR backscatter and NDVI as input variables and generate biomass maps of higher accuracy. In addition, freely available field and satellite data are used in this study.
2 Study Area
The study area is located in Paragominas, Brazil (Fig. 1). The study area is bound by extent coordinates of 48°54′15.607″ W, 3°35′15.339″ S to 48°19′43.994″ W, 3°43′24.833″ S. The dominant vegetation in this region is humid forest with predominantly oxisols perenefólia and ultisols soils. Paragominas was a large agricultural and timber center of the country, with its exploitation started in the 70s and expansion in the 80s. During the period 1989–1990, it is estimated that 67,845 ha were explored for wood intended to supply the 238 sawmills operating in the region. Paragominas was once considered the largest timber source of Brazil [4, 14].
3 Data Collection
3.1 Satellite Data
ALOS PALSAR data was downloaded from ASF’s (Alaska Satellite Facility) Data portal for June month in 2010. To avoid any disturbance in backscatter due to soil moisture, dry season of year was chosen for the analysis. Data was acquired in Fine Beam Dual Polarization mode. HH and HV were the two polarization channels in which data was acquired. Data has spatial resolution of 20 m and was acquired at an incidence angle of 34.3°. ALOS PALSAR mission was decommissioned in the year 2011. As there was no suitable data available in 2011, 2010 data was used. Landsat 7 LaSRC (Landsat Surface Reflectance Corrected) Level 2 products were procured by ordering through USGS earth explorer website. Landsat 7 has spatial resolution of 30 m × 30 m.
3.2 Field Data
This data set provides measurements for diameter at breast height (DBH), commercial tree height, and total tree height for forest inventories taken at the Fazenda Cauaxi and the Fazenda Nova Neonita, Paragominas municipality, Para, Brazil. Also included for each tree are the common, family, and scientific name, coordinates, canopy position, and for dead trees the decomposition status. These biophysical measurements were made at Fazenda Cauaxi during 2012 and 2014 and at the Fazenda Nova Neonita during 2013. The data were collected under the project Sustainable Landscapes, a project supported by the United States Agency for International Development (USAID) and US Department of State [4]. Forest inventory surveys were conducted at the Fazenda Nova Neonita and Fazenda Cauaxi in the Paragominas municipality, Para, Brazil (). Total area inventoried at the Fazenda Cauaxi was 22 ha: 22 plots of 10,000 m2. Plot sizes were 20 × 500 m with a 2 × 500-m subplot within a plot. Measurements were made at the Fazenda Cauaxi from 2012/01/27 to 2012/03/26 and also from 2014/02/18 to 2014/04/25. Commercial and total height measurements were only made in 2012. Trees with diameter at breast height (DBH) equal to or greater than 35 cm were accounted for and measured within the plot area. Total Height (m) was measured on live trees and standing dead trees using a clinometer and tape as the height to the highest point of the tree crown (Fig. 2).
4 Methodology
4.1 Image Preprocessing
Level 1.5 product of ALOS was downloaded through ASF’s data portal. Image is a 16 bit datatype with DN’s (Digital numbers) varying from 0 to 65,535. SNAP software was made use of to process the image and the software automatically calibrates the ALOS image for Normalized Radar Cross Section (NRCS) using the following equation:
Fine beam dual pol data has spatial resolution of 20 m. Speckle filtering was done using refined lee filter to eliminate speckles in the image. Image was upscaled to 100 m × 100 m or 1 ha resolution as it is standard practice to measure the biomass in tons/ha units [2]. Layover and shadow effects on the image were reduced using SRTM 1 arc second Digital Elevation Model (DEM). This is done because if terrain slope is facing the sensor then returning signals get stronger relatively to the other side of the slope. Landsat 7 LaSRC products were used to generate NDVI maps using ArcMap 10.1 software. Landsat 7 products had gaps due to error in the detectors and were gap filled using IDL code in ENVI software. NDVI maps generated were also upscaled to a pixel area of 1 ha (Fig. 3).
4.2 Field AGB Calculation
Field AGB was calculated using generalized allometric equations for the study area [1]. AGB depends upon species of tree and can be estimated using allometric equations which are either species specific or generalized within the given area. As there are no species-specific allometric equations, generalized equations have been utilized to calculate the field AGB. Allometric equations take DBH (cm) and Height (m) as input variables and give AGB in tons/ha. The equation used for this study is as follows:
4.3 Plot Planning to Extract Pixel Values
As we can see in Fig. 2, sampling of trees has been done in the form of transect which makes it difficult to extract pixel values for a 500 m long transect. To avoid the same, each transect was manually divided into 3 sample plots of size 90 m × 20 m, 30 m × 20 m and 60 m × 20 m resulting in plots of area 1800 m2, 600 m2, 1200 m2, respectively. Generally, random sampling techniques are adopted to fix the plot size which are generally square in shape. As such square plots were unavailable, in this case randomly sampled plots were created to avoid bias in results. Finally, this resulted in 64 plots for the regression modeling of backscatter versus field AGB. In which 44 plots were chosen for modeling and 20 for validation. Plots covering the upscaled imagery were noted and values were extracted for NDVI, HH and HV images.
5 Results and Discussion
5.1 AGB Modeling Using Optical Data
Nonlinear regression was performed between field calculated AGB and NDVI. Logarithmic fit gave most appropriate results while also giving suitable R2 values. NDVI was used as a predictor variable for modeling. Equation of the following form was used. Coefficient of determination and RMSE were used as performance indicators for the model
R2 of 0.298 was obtained which is considerably low and is exhibiting poor relation with field calculated AGB.
5.2 AGB Modeling Using SAR Data
Generally, the regression function between field AGB and SAR backscatter (σ°) in dB is known to be nonlinear. It can be represented by logarithmic, exponential, and power functions until it reaches saturation. As the curve starts to reach this threshold value of AGB backscatter starts to saturate and becomes nearly constant beyond this AGB point [11, 12]. Coefficient of determination was used as performance indicator for the model. R2 value of 0.604 and 0.345 was obtained for HH and HV polarization channels, respectively. HH backscatter is outperforming HV in estimation of biomass. Apart from this, other polarization combinations were also tested for biomass estimation. In this study, the following polarization combinations were tested (1) HH + HV (2) HH − HV (3) (HH − HV)/(HH + HV) are used (Figs. 4 and 5).
HV backscatter showcased better R2 when compared to HH backscatter with value of 0.5592. Also, HH + HV polarization combination exhibited reasonably good (R2 = 0.6574) relation. (HH − HV) and (HH + HV/HH − HV) combinations had lower relation with AGB.
5.3 AGB Modeling by Combining Optical and SAR Data
Multiple linear regression was carried out in order to generate higher accuracy biomass maps using polarization combinations and NDVI as predictor variables with AGB as a response variable. HH backscatter was considered because it was showing better relation with field AGB. HH backscatter along with NDVI showed increase in R2 to 0.663 with RMSE of 31.01 t/ha.
Equation 4 was used to calculate predicted biomass. Model validation was done using linear regression between predicted and field AGB which resulted in R2 of 0.3625 (Figs. 6 and 7).
6 Conclusion
Synergy of optical and SAR data with different polarization combinations in estimation of biomass was assessed. HV backscatter has better sensitivity (R2 = 0.559) for AGB than HH backscatter (R2 = 0.437) which agrees with earlier research. HH + HV yielded R2 of 0.6574 which was outperforming all other backscatter combinations. Empirical model was developed to generate biomass maps and R2 of 0.732 was obtained during calibration and 0.363 during validation (RMSE = 43.18 t/ha). However, this result was generated without excluding any outliers so as to avoid bias due to small number of samples. Also, Model generated is site specific and depends on structure of tree and weather of surrounding area. Tropical trees have higher density when compared to other species, resulting in attenuation of SAR signals. There is future scope to try other vegetation indices like RVI, EVI, etc., along with various polarization combinations. It is expected that with larger number of sample plots, modeling, and validation statistics become more robust [2]. Figure 8 depicts final biomass map generated using MLR equation.
References
Araujo TM, Higuchi N, de Carvalho Junior JA (1999) Comparison of formulae for biomass content determination in a tropical rain forest site in the state of para, Brazil. Forest Ecol Manag 117(1–3):43–52
Baig S, Qazi WA, Akhtar AM, Waqar MM, Ammar A, Gilani H, Mehmood SA (2017) Above ground biomass estimation of Dalbergia sissoo forest plantation from dual-polarized ALOS-2 PALSAR data. Can J Remote Sens 43(3):297–308
Castel T, Beaudoin A, Stach N, Stussi N, Le Toan T, Durand P (2001) Sensitivity of space-borne SAR data to forest parameters over sloping terrain. Theory experiment. Int J Remote Sens 22(12):2351–2376
dos Santos M, Pinage E, Longo M, Spinelli-Araujo L, Keller M (2015) Characterized edge effect with the use of lidar data in a degraded forest land-scape in the municipality of paragominas (pa). In: Proceedings of the XVII Brazilian symposium on remote sensing-SBSR, Joao Pessoa, Brazil, vol 25
Goh J, Miettinen J, Chia AS, Chew PT, Liew SC (2014) Biomass estimation in humid tropical forest using a combination of ALOS PALSAR and spot 5 satellite imagery. Asian J Geoinform 13:4
Hamdan O, Aziz HK, Rahman KA (2011) Remotely sensed l-band SAR data for tropical forest biomass estimation. J Trop Forest Sci 318–327
Harrell PA, Kasischke ES, Bourgeau-Chavez LL, Haney EM, Christensen NL Jr (1997) Evaluation of approaches to estimating aboveground biomass in southern pine forests using SIR-C data. Remote Sens Environ 59(2):223–233
Imhoff ML (1995) A theoretical analysis of the effect of forest structure on synthetic aperture radar backscatter and the remote sensing of biomass. IEEE Trans Geosci Remote Sens 33(2):341–352
Kellndorfer JM, Dobson MC, Vona JD, Clutter M (2003) Toward precision forestry: plot-level parameter retrieval for slash pine plantations with JPL airsar. IEEE Trans Geosci Remote Sens 41(7):1571–1582
Kumar KK, Nagai M, Witayangkurn A, Kritiyutanant K, Nakamura S et al (2016) Above ground biomass assessment from combined optical and SAR remote sensing data in Surat Thani Province, Thailand. J. Geogr. Inf. Syst. 8:506
Lucas R, Armston J, Fairfax R, Fensham R, Accad A, Carreiras J, Kelley J, Bunting P, Clewley D, Bray S et al (2010) An evaluation of the ALOS PALSAR L-band backscatter above ground biomass relationship Queensland, Australia: impacts of surface moisture condition and vegetation structure. IEEE J Sel Top Appl Earth Obs Remote Sens 3(4):576–593
Mitchard ET, Saatchi SS, Woodhouse IH, Nangendo G, Ribeiro N, Williams M, Ryan CM, Lewis SL, Feldpausch T, Meir P (2009) Using satellite radar backscatter to predict above-ground woody biomass: a consistent relationship across four different African landscapes. Geophys Res Lett 36:23
Tanase MA, Panciera R, Lowell K, Tian S, Hacker JM, Walker JP (2014) Airborne multi-temporal l-band polarimetric SAR data for biomass estimation in semi-arid forests. Remote Sens Environ 145:93–104
Verissimo A, Barreto P, Mattos M, Tarifa R, Uhl C (1992) Logging impacts and prospects for sustainable forest management in an old Amazonian frontier: the case of Paragominas. Forest Ecol Manag 55(1–4):169–199
Wang C, Niu Z, Gu X, Guo Z, Cong P (2005) Tropical forest plantation biomass estimation using RADARSAT-SAR and TM data of South China. In: MIPPR 2005: SAR and multispectral image processing, vol 6043. International Society for Optics and Photonics, p 60432Q
Acknowledgements
The author would like to thank ASF and ORNL DAAC for providing ALOS PALSAR dataset, Forest inventory datasets (CMS project), respectively.
Author information
Authors and Affiliations
Corresponding author
Editor information
Editors and Affiliations
Rights and permissions
Copyright information
© 2020 Springer Nature Singapore Pte Ltd.
About this paper
Cite this paper
Huggannavar, V., Shetty, A. (2020). Biomass Estimation Using Synergy of ALOS-PALSAR and Landsat Data in Tropical Forests of Brazil. In: Ghosh, J., da Silva, I. (eds) Applications of Geomatics in Civil Engineering. Lecture Notes in Civil Engineering , vol 33. Springer, Singapore. https://doi.org/10.1007/978-981-13-7067-0_48
Download citation
DOI: https://doi.org/10.1007/978-981-13-7067-0_48
Published:
Publisher Name: Springer, Singapore
Print ISBN: 978-981-13-7066-3
Online ISBN: 978-981-13-7067-0
eBook Packages: EngineeringEngineering (R0)