Abstract
This paper evaluates the relative performances of academic departments of The NorthCap University, Gurgaon, for the academic year 2014–2015. The study is carried out with two inputs, namely number of academic staff and number of non-academic staff, and four outputs, namely total enrolled students, total pass students, students placed for jobs, and research index. Data envelopment analysis (DEA)-based dual CCR model is used for the efficiency evaluation. The results show that out of 7 academic departments, four are technically efficient with average efficiency score 0.899. The rest three inefficient departments are operating on increasing returns to scale.
Access provided by CONRICYT-eBooks. Download conference paper PDF
Similar content being viewed by others
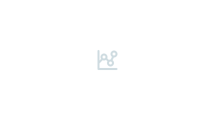
Keywords
1 Introduction
Education and research is the main contributor of the overall development of any nation. There are several government and private institutions in India for the scientific and technical education which works for the development of the nation. There are several names in the list of best government institutions. In counterpart of the government higher education, there are also several private institutions which also provide the scientific and technical education. The first name in the list of private institution/university in NCR region comes into insight is The NorthCap University (previously known as ITM University Gurgaon).
The NorthCap University was founded in 1996. Initially, it was known as Institute of Technology and Management. In the year 2009–2010, the institute gained its status as an autonomous university as ITM university. In August 2015, it has been renamed as The NorthCap University (NCU).
This paper measures the relative efficiencies of academic departments of NCU based on multiple input and multiple output criteria. The second objective of the paper is to measure the comparative efficiency of the departments, and the third objective is to identify the efficient and inefficient performers.
There are two approaches to measure the efficiencies of DMUs. One is parametric, and the other is nonparametric. In parametric approaches, there are two methods, namely ordinary least square method and the stochastic frontier method, in which functional form is required, while data envelopment analysis (DEA) and free disposal hull are nonparametric approaches which do not require any functional form. The most frequently and most suitable technique for measuring the performance of non-profit organizations is DEA. Therefore, this paper applies DEA-based methodology for the performance measurement of the departments. It is, on the whole, appropriate technique for the researcher in investigating the efficiency of DMUs that exchange multiple inputs into multiple outputs.
In recent years, DEA has become very popular among researchers for measuring the performance of DMUs. DEA is extensively used in several areas including education, banking, health, drugs and pharmaceutical industries across the world to measure the efficiencies. Some recent studies are reviewed here.
Tyagi et al. [1] use DEA-based CCR and BCC models to measure the efficiencies of 19 academic departments of IIT Roorkee (India). Rayeni and Saljooghi [2] used DEA to determine the relative efficiency scores of academic departments of a university and set benchmarks for the departments. Aziz et al. [3] use DEA to measure the relative efficiency of 22 academic departments of a public university in Malaysia. Aristovnik [4] used DEA to measure the relative efficiency in public education expenditures in the EU and OECD countries. Barra and Zotti [5] used DEA to assess technical efficiency in a big public university. Puri and Yadav [6] measure the efficiencies of 27 public and 22 private sector banks for the year 2009–2010 using DEA-based CCR and BCC models. Mogha et al. [7] assess the technical and scale efficiencies of 50 private sector hospitals in India for the period 2004–05 to 2009–10 using CRS- and VRS-based DEA models. Mahajan et al. [8] estimate technical efficiencies, slacks, and input/output targets of 50 large Indian pharmaceutical firms for the year 2010–11. Mogha et al. [9] used DEA-based CCR model to assess the efficiency of 36 public sector hospitals of Uttarakhand, state of India, for the calendar year 2011.
The rest of the paper is organized as follows: Sect. 2 contains methodology; Sect. 3 describes the research design, input/output variables, DEA model used in the study and data; Sect. 4 gives efficiency results followed by the conclusion in the last.
2 Methodology
The application procedure of DEA analysis includes three stages: The first stage is to select the DMU(s) departments (in this case). Then, the input and output variables are to be selected for the efficiency analysis. Finally, model is selected to analyze the data. The DEA model is described later in the paper.
2.1 Selection of DMUs
We have selected all seven departments of NCU for the efficiency evaluation, since all the departments have similar objective, i.e., academic orientation (teaching and research), and therefore similar in structure, funded by the same governing body, and thus could be taken for the DEA study.
2.2 Selection of the Inputs and Outputs
As per the availability of data, cross-sectional analysis is based on data of 7 departments for the academic year 2014–2015. The descriptions of used variables are given below.
2.2.1 Academic Staff
Academic staff is a mandatory factor for any academic institution for teaching and research purpose. This includes professors, associate professors and assistant professors, lecturers, and research scholars. These are the main employees of the institution to create enrollments by convincing the students from schools and also to create the research outputs. Thus, the selection of this variable as an input variable is appropriate in this study.
2.2.2 Non-academic Staff
Each institute/university has its construction, staff rooms, and laboratories for each department. So, for non-academic work like laboratory attendant and other file handling work, each department has particular manpower. So, this factor can also be used as an input variable for the efficiency evaluation.
2.2.3 Total Enrolled Students
There are three programs: undergraduate (UG), postgraduate (PG), and doctoral program (Ph.D.) in each of the department of the university. For making a variable of the total enrolled students, we assign weights to the number of students of all the levels. By this, we get a new variable “total enrolled students” as a new variable:
Since all the undergraduate students in first-year courses are taught by the APS department faculty members, in this study, the number of students in APS department is taken as the number of students of B.Sc., M.Sc., and the total first year of UG students. This is used as an output variable.
2.2.4 Total Passed Students
In this, we include the number of graduate and postgraduate degrees conferred. Students have to pass in all the subjects of their concern department. By adding number of undergraduate, postgraduate and doctoral degree awarded, a new output variable “total passed students” is formed.
2.2.5 Total Students Placed for Jobs
This variable includes the number of the students get offer from any company or organization (either they joined or not). This variable is used as an output variable.
2.2.6 Research Index
This is the main activity in the growth of an academic department or any institution. This index is formed with the combination of research papers published in journals and conferences with proceedings, presented in the conferences and research projects carried out by the department. About the quality of research, there are some contradictions between the researchers. In this context, the appropriate term for the variable is “research index.” Thus:
2.3 Selection of the Model
Firstly, DEA was developed in [10] by Charnes et al. which was extended in [11] by Banker, Charnes, and Cooper. These models evaluate the performance based on the criteria of multiple inputs and outputs. There are two types of orientations in DEA literature. One is input-oriented measure which aims to minimize inputs with existing level of outputs, and other is output-oriented measure which aims to maximize outputs with existing levels of inputs.
In DEA applications, where the non-flexible inputs are used, the output orientation is more suitable. If the outputs variables are fixed by the organizations, an input orientation is used (Ramanathan [12]).
In the present study, two inputs, namely academic staff and non-academic staff are used. In most of the cases, these variables are unbendable for a year. Also, the variables such as “progress” and “research index” are also not decided at advance bases. In some of the cases, total enrollment can be fixed by the organization. So, the output orientation is proper for the current analysis.
In this study, dual CCR model, also known as CCR envelopment model, is used and is given by:
where \( s_{rk}^{ + } \) denotes the slack of the kth DMU in the rth output, \( s_{ik}^{ - } \) denotes the slack of the kth DMU in the ith input, and \( \lambda_{jk} \)’s are the dual variables. \( \theta_{k} \) is the (proportional) decrease functional concurrently to all inputs and results in a radial movement torwards to the efficiency frontier. This is properly identified as CCR envelopment model. The explanation of the outcome of the envelopment model (1) can be summarized as:
The DMUk is fully efficient if
-
(a)
Optimal value of \( \theta \) is 1.
-
(b)
All the slack variables becomes zero.
The DMUk is weakly efficient if
-
(a)
Optimal value of \( \theta \) is 1.
-
(b)
\( s_{rk}^{ + *} \ne 0\quad {\text{and}}/{\text{or}}\;\;s_{ik}^{ - *} \ne 0 \) for some r and i in some alternate optima.
The nonzero value of slacks and/or the optimal value of \( \theta \) less than 1 identify the sources and sum of any inefficiency that may be present in the DMU. If the optimal value \( \lambda_{jk}^{*} \) of \( \lambda_{jk} \) is nonzero, then the jth DMU represents the reference set (peer) of the kth DMU and the value for these reference set elements is the coefficients used to construct the benchmark. The reference set can be defined as the collection of DMUs used to construct the virtual DMU as benchmark which shows about increment in outputs and decrement in inputs to make the kth DMU efficient. The results of the study are calculated by using DEA Solver software [13].
3 Data
Table 1 demonstrates the explanatory statistics of the data collected from different sources. The data for input variables are taken from HR admin office of the university. Total enrolled student’s undergraduate, postgraduate, and doctoral records are taken from annual report and VC report of NCU (2014–2015). The data about the number of students got offer for job (anywhere) are taken from controller of records (COR).
Number of research papers published in journals and conference of the department and research project in number of taken by the department all are taken from newsletters of NCU from July 2014 to June 2015. The collected data of the departments for all the factors are given in Table 1.
4 Findings
The efficiency score (OTE) for 7 academic departments of NCU is estimated for year 2014–2015. The results are calculated on the basis of output orientation. The efficiency scores calculated by CCR output-oriented model along with reference set, peer count, slacks, and targets are discussed.
4.1 Overall Technical Efficiency (OTE)
DEA calculates the efficiency of academic departments that create a production leading edge. The calculated efficiency scores indicates that departments whose efficiency is equal to 1 are on the efficiency leading edge under CRS assumption and those having efficiency score less than 1 are inefficient compared to the departments on the efficiency frontier. Table 2 evinces that out of 7 academic departments, only 4 are overall technically efficient and form the efficiency frontier. The remaining 3 departments are inefficient. The efficient departments APS, CSE and IT, ECE and EE, and management form the “reference sets” for the departments which are not efficient. Figure 1 shows the regression line of OTE.
4.2 Slacks and Targets
For inefficient departments, DEA calculate slack in inputs and outputs and set targets so that they can be efficient. These targets can be calculated by the relations given below:
For outputs:
For inputs:
where \( \overline{y}_{rk} (r = 1,2,3,4) \) and \( \overline{x}_{ik} (i = 1,2,3) \) are the output and input targets, respectively, for the kth DMU; \( y_{rk} \) and \( x_{ik} \) are the actual rth output and ith input, respectively, of the kth DMU; \( \phi_{k}^{*} \) is the optimal efficiency score of the kth DMU; \( s_{ik}^{ - *} \) and \( s_{rk}^{ + *} \) are the optimal input and output slacks of the kth DMU for (i = 1, 2, 3) and (r = 1, 2, 3, 4). The optimal input and output slacks for inefficient DMUs are given in Table 3.
Table 4 represents the input and output target values for the departments, which are not efficient, along with percentage diminution in inputs and expansion in outputs. The results expose that on average a department has no considerable scope to shrink inputs, but it has a significant scope to expand outputs compared to the best performing department. On average 59.61% of total enrolled students, 22.09% total passed students, 149.46% of students placed for jobs and 49.00% research index should be increased with 16.75% reduction in non-academic staff and full utilization of academic staff, if all the inefficient departments run at the efficient level.
5 Conclusion
In this paper, we measure the relative efficiencies of 7 academic departments of The NorthCap University, Gurgaon (India), using the DEA-based dual CCR model. The study finds that out of total 7 departments, only 4 (57.14%) departments are found to be efficient as they scored the maximum level of efficiency. Average overall technical efficiency of the departments is found to be 89.90%, which indicates that on average, the inefficient departments are not using their potential by 10.10%; that is, these departments have the scope of producing more outputs with lesser inputs than their existing levels. The target setting results show that only one input have some scope to reduce but all the outputs have significant scope to augment. The results also suggests that on average, 59.61% of total enrolled students, 22.09% total passed students, 149.46% of students placed for jobs and 49.00% research index should be increased with 16.75% reduction in non-academic staff if all the inefficient departments operate at the level of efficient departments.
References
Tyagi, P., Yadav, S.P., Singh, S.P.: Relative performance of academic departments using DEA with sensitivity analysis. Eval. Prog. Plan. 32, 168–177 (2009)
Rayeni, M.M., Saljooghi, F.H.: Benchmarking in academic departments using data envelopment analysis. Am. J. Appl. Sci., Iran 11, 1464–1469 (2010)
Aziz, N.A.A., Janor, R.M., Mahadi, R.: Comparative departmental efficiency analysis within a university: a DEA Approach. In: 6th International Conference on University Learning and Teaching, vol. 22, pp. 212–219 (2012)
Aristovnik, A.: Relative efficiency of education expenditures in eastern Europe: a non-parametric approach. J. Knowl. Manage., Econ. Inform. Technol, Slovenia 3, 1–13 (2013)
Barra, C., Zotti, R.: Measuring efficiency in higher education: An empirical study using a bootstrapped Data envelopment analysis. Int. J. Adv. Econ. Res. 21, 11–33 (2016)
Puri, J., Yadav, S.P.: Performance evaluation of public and private sector banks in India using DEA approach. Int. J. Oper. Res. 18, 91–121 (2013)
Mogha, S.K., Yadav, S.P., Singh, S.P.: Estimating technical and scale efficiencies of private hospitals using a non-parametric approach: case of India. Int. J. Oper. Res. 20, 21–40 (2014)
Mahajan, V., Nauriyal, D.K., Singh, S.P.: Technical efficiency analysis of the Indian drug and pharmaceutical industry: a non-Parametric approach. Benchmarking: Int. J. 21, 734–755 (2014)
Mogha, S.K., Yadav, S.P., Singh, S.P.: Estimating technical efficiency of public sector hospitals of Uttarakhand (India). Int. J. Oper. Res. 25, 371–399 (2016)
Charnes, A., Cooper, W.W., Rhodes, E.: Measuring the efficiency of decision-making units. Eur. J. Oper. Res. 2, 429–441 (1978)
Charnes, A., Cooper, W.W.: Programming with linear fractional functional. Naval Res. Logistics Q. 9, 181–186 (1962)
Ramanathan, R.: An Introduction to Data Envelopment Analysis. Sage Publication India Pvt. Ltd., New Delhi (2003)
Avkiran, N.K.: Investigating technical and scale efficiencies of Australian Universities through data envelopment analysis. Socio. Econ. Plan. Sci. 35, 57–80 (1999)
Acknowledgements
The present study is just for research purpose. The data used in the study are not for commercial purpose. The data are collected from different sources of NCU with the permission of vice-chancellor of the university.
Author information
Authors and Affiliations
Corresponding author
Editor information
Editors and Affiliations
Rights and permissions
Copyright information
© 2018 Springer Nature Singapore Pte Ltd.
About this paper
Cite this paper
Mogha, S.K., Kumar, A., Kumar, A., Kunroo, M.H. (2018). Performance Measurement of Academic Departments: Case of a Private Institution. In: Pant, M., Ray, K., Sharma, T., Rawat, S., Bandyopadhyay, A. (eds) Soft Computing: Theories and Applications. Advances in Intelligent Systems and Computing, vol 584. Springer, Singapore. https://doi.org/10.1007/978-981-10-5699-4_36
Download citation
DOI: https://doi.org/10.1007/978-981-10-5699-4_36
Published:
Publisher Name: Springer, Singapore
Print ISBN: 978-981-10-5698-7
Online ISBN: 978-981-10-5699-4
eBook Packages: EngineeringEngineering (R0)