Abstract
The magnification mechanism of human settlement is called urbanization, involving various other activities such as population transition, resource consumption, etc. leading to various growing patterns of urban augmentation. The assessment of such spatial pattern is crucial in developing a sustainable urban agglomeration. Night-time light (NTL) data is an important machination for such assessment and detailed monitoring. In this paper, DMSP-OLS (the Defence Meteorological Satellite Program/Operational Linescan Program) datasets have been used to assess the urban straggle of Australian Capital Territory (ACT) and to delineate the urban extent from 1992 to 2012, at an interval of 3 years. DMSP-OLS has the unique capability to detect synthetic lights from cities, towns, industrial sites, ports, etc. Moreover, using ARC GIS calligraphies, 20 random points were selected from the extracted area of interest (AOI). Pixels values of those 20 random points are derived from the given time series dataset (1992–2012). A regression value was extracted from each year by using a second-order polynomial equation. A polynomial regression model is also constructed by taking the regression values and the time series as the two variables, respectively. Urban light index (ULI) is also constructed for analysing the progression of urbanization in ACT from 1992 to 2012 with the help of a derived formula. Furthermore, a unit circle buffer zone having a radius of 20 km is established by taking the centre of each built-up zone as the focal point of the constructed buffer, to compare easily the rate of expansion of urbanization. The present paper indicates the growing potential of the DMSP-OLS night-time satellite data to define the urban light space information, which truly describes the attributes of urban sprawl, and to delineate the evolution of urban morphology and urban extension.
Access provided by Autonomous University of Puebla. Download chapter PDF
Similar content being viewed by others
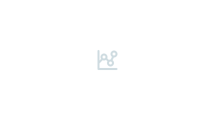
Keywords
1 Introduction
The congenital nature of man as a societal visceral directly or indirectly has led to the development of urbanization. With the plodding increase in the concreteness of people living in urban zones than in rural, this increase of people leads to progression of towns and cities making urban to sprawl. Urban sprawling causes an outward migration from urban to low-density areas. Metamorphosis of agrarian economy to an industrial and transition of natural ecological land cover simulated land use environment (Han et al. 2009; Feng et al. 2002; Elvidge et al. 1999; Li 2004). The synchronized process associated with population statistical transition inducing force on nature and biological cycle, aiding to anthropogenic activities, resource consumption and economic dynamics (Milesi et al. 2003; Ma et al. 2012). Therefore, the measurement of urbanization progress can be deliberated from only with the values of demographic transition (Zhang et al. 2013; Pandey et al. 2013; Letu et al. 2012; He et al. 2006; Lo 2010). Exploration of the potencies influencing urban enlargement and its impact on environment is crucial for scrutinizing ecological urban augmentation (He et al. 2012). Estimation and monitoring of the transition of urban agglomeration over the years is crucial for the development of a sustainable metropolitan planning (Cinzano et al. 2001; Fujita et al. 1999; Small 2003). Constituting of a very small segment of the facade of the earth, urban escalation exerts colossal influence on the other interrelated activities such as mass movements, mass compactness and exploitation of recourses (Small 2005; Sutton et al. 2001). The structural formation of the spatial allocation and the occurrence of the large-scale urban netting have imperative allegation ranging from socio-economic development to ecological urban augmentation to evolution of urban fabrication (Elvidge et al. 2007; Imhoff et al. 1997). Assessment of worldwide urban extent through corporeal supervision is challenging. Currently, night-time dataset for the measurement of the large-scale lighted extension is being employed (Liu et al. 2012; Shu et al. 2011).
The night-time satellite imagery is an important contrivance in analysing the urban straggle and delineating urban extent. Synthetic lights of towns, cities, manufacturing locations and anthropological actions during night-time can be detected using the satellite images of night-time data from the Defence Meteorological Satellite Program/Operational Linescan Program (DMSP/OLS) (Schneider et al. 2010; Elvidge et al. 1999), and VNIR (visible near infrared, 0.4–1.1 μm) and TIR (thermal infrared, 10.5–12.6 μm) are two spectral bands of DMSP with radiometric resolution of 6 bit and 8 bit, respectively (Owen 1998; Elvidge et al. 1997). In a spatial resolution of 2.7 km and a swath width of 3000 km, OLS covers a wider extent of the surface of the earth (Miller et al. 2009). Although previously used for cloud observation (Henderson et al. 2003; Huang et al. 2016), DMSP-OLS images are currently being used extensively for urban morphology assessment due to its ability to detect low light during night without moonlight (Letu et al. 2012; Ma et al. 2012). Delineate global dispersal of metropolitan extents through stable satellite imaging, with the help of DMSP/OLS in a precise and economical manner. The spatial resolution of 30 arcsecond of the DMSP/OLS continues to provide night-time data on urban morphology at a national and global measure, but the coarse resolution debilitates the accurate extraction of the built-up areas (Liu et al. 2015; Li et al. 2016; Lu et al. 2008). The dataset of DMSP-OLS can be arranged into three types: the mutated radiance, the stable NTL and the standardized DN (digital number) values (Welch and Zupko 1980). Among them, the stable NTL dataset is used for assessment of urban morphology (Lo 2002; Ma et al. 2012).The acquired dataset from DMSP-OLS utilized to map the proportion of urban extension, urban nucleus and the progression of urbanization. The major challenged faced while using DMSP-OLS dataset is to acquire quantitative association between urbanization factors and the pixel brightness of the dataset in different types of urban magnification mechanism (Sutton 2003; Ting Ma et al. 2012).
However, the saturated pixel values restrict the possibilities to differentiate between interurban discrepancies (Mertes et al. 2015). Previous studies have revealed inconsistency between the actual lighted zones and the geographical periphery of the urban areas (Su et al. 2015). Sometimes the global synthetic lighted zones aligned to as the advanced built-up area allude due to blooming effecting (Small et al. 2005). The abrasive radiometric and spatial resolution of the DMSP/OLS also detecting scattered low-lighted zones, large amount formation of mixels in OLS satellite imagery and geo-positional errors are introduced during the processing stage; these are some of the constraints of DMSP-OLS which results in blooming effects, yielding erroneous information of synthetic lights of site which in actual does not restrain any energy resource. So, the frequently identified lighted area of threshold 89% was proposed by (Imhoff et al. 1997) to reduce the effects of blooming and to establish a consistent relationship between substantial lighted zones and the perimeter of the urban extent, thus shrinking the lighted zones (Small et al. 2005). But fixation of a threshold evades other minor frequency lights, thus reducing the information content in the dataset (Small et al. 2005). Moreover, DMSP-OLS dataset cannot be applied directly due to unavailability of on-broad calibration producing erroneous DN (digital number) values caused by varied orientation and displacement of sensor and atmospheric and radiometric condition (Li et al. 2014).
NPP-VIIRS, another regional night-time amalgamated data, is also used to the exact statistics of urban morphology chronicling synthetic light at night, having a spatial resolution of 15 arcsecond which is equal to about 500 m and a radiometric resolution of 14 bit (Elvidge et al. 2007; Kaifang Shi et al. 2014). Such high spatial and radiometric resolutions of NPP-VIIRS dataset have the potential to stipulate better product of information. To evaluate urban agglomeration, urban extension and socio-demographic pattern at a variety of global scales such as demographic transition, power consumption and conservational modification, the NPP-VIIRS dataset is a powerful tool for such assessment. However, there are still some constraints in NPP-VIIRS dataset such as area dependency on referenced data affecting the result of urban area extractions; the inability to remove the noise created from fires, gas flares and volcanic eruptions from recent NPP-VIIRS dataset and the eminence of the dataset however requires to be enhanced (Kaifang Shi et al. 2014). A debatable competition is arising between the application of the dataset of DMSP-OLS and the NPP-VIIRS. So, a comparative analysis of the accuracy quality of the dataset of NPP-VIIRS with that of DMSP-OLS will provide better result in scrutinizing the urban sprawl and extraction of the built-up area and their application in future research but being in the toddler stage, VIIRS night-time images are not able to fulfil the demands of the long duration time sequence requirements of analyst and also involves a sophisticated data processing (Dewan and Yamaguchi 2009). However other remote sensing data such as QuickBird, IKONOS and SPOT images are expensive and need intricate data processing along with miscellaneous spectral differentiation, and inadequate spatial and radiometric resolution causes satellite like Landsat TM less capable to detect urban expansion and urban dynamics.
This paper aims to appraise the capabilities of NTL dataset to identify and delineate the urban morphology, urban augmentation and growth of suburban zones over the years of the Australian Capital Territory, using night-time satellite imagery from DMSP-OLS dataset from 1992 to 2012 at an interval of 3 years, for evaluation of the Australian Capital Territory growing pattern of urban sprawl and urban extension which is critical for sustainable development relative to ecological urban planning, management and decision-making for future urban development projects.
2 Study Area
The Australian Capital Territory (ACT) embraces a population of 410,300 out of 24 billion and a population density of 179.96 per km2 (ABS 2017). ACT is located on the south-western side of the country, with geographical extension of 35°18′29″S latitude and 149°7′28″E longitude. Surrounded by the Australia’s capital city Canberra on the north, the Namadgi National Park on the south constitutes about 40% of the territory (Linsie Tan 2017), the Goulburn-Cooma railway line in the east and in the west by the Cotton River. Canberra is a sprawling city with heavily concentrated economic activities of the Australian Capital Territory allied with rapidly increasing population associated with steady employment. The urban morphology of Canberra as a planned city was initially designed by a major American architect Walter Burley Griffin (Wigmore 1971). The transportation network follows a prototype of wheel and spoke. The nucleus of the city’s morphology is arranged as a 90° angle, with two directional axes, one stretching along Lake Burley Griffin and the other stretching from north-eastern Anzac Parade to the foot of the Mount Ainslie. Settlement hierarchy of the urban areas of Canberra is organized in a chronological order of districts, city hubs, assembly hubs, native fringes and rural community (Fig. 10.1).
3 Materials and Methodology
3.1 Spatial Analysis: Estimation of Detected Area Under Threshold Frequency
The lighted zone and their frequency distributions size are detected through frequency threshold to quantify the light detected area and the adjoining light polygons and the regularity of centroid for every dataset, and the frequency of dataset is from 1992 to 2012, which is booked at diverse edge. Using ArcGIS calligraphies, we planned the numeral of sunlit polygons and their extent and outside for both onsets at 10% breaks among 0% and 90% symmetry. The longitude and latitude tones were then bestowed to the centroids of each concerning lighted magnitude. For enumerating the communication amid size and regularity of recognition, we calculated the finding incidence at the centroid of each polygon at a different interval. This was finished by coalescing the inventive light incidence datasets with the case comprehending the longitude and latitude directs of the centroids of the attached bright polygons. By the system, every uncovering regularity value agreeing with connecting lighted area is dispensed to the area centroid. In several cases, people find undeviating distance which is more instinctual than expanse, and we generally embody the size of each irregularly fashioned neighbouring lighted area as comparable circular span defined.
3.2 Urban Built-Up Area Extraction
Constrution of artificially lighted urban areas provides the radiance values for corresponding DN values which surrounds the different values. In other different revisions, a non-set assessment was regularly assistance to division of urban areas on DMSP-OLS documents (Imhoff et al. 1997; He et al. 2006; Liu et al. 2012; Small et al. 2005). Larger than the different threshold values which is considered as the building part of the different urban areas and the corresponding pixel values is the inception value for that particular areas. With the otherwise use of DMSP-OLS data overhead and in lieu of this, the NPP-VIIRS data can be used which is the best suited method as used to extract the onset value of urban modelling. The optimization of different threshold values for urban mapping causing extraction of night-time light data is also a provident of government statistical data (He et al. 2006). The dawn rate that created slightest difference among image-derived assessment and arithmetic figures was scheduled as the foundation for assessment of night-time light-based urban mapping. The high-accuracy implementations based on this method are the more accurate based on the DMSP-OLS data night-time light data-based urban mapping (Shu et al. 2011). This revision includes the adoption of a comparable tactic to decide the peak beginning threshold value of residential urban area abstraction for every one different city from two types of nocturnal graceful NTL-based data images. However, the main objectives to find different diplomatic process are the following: Firstly, the extraction of different types of night-time light images for every municipal (city) was mined from the different international data cliques by using a façade polygon of the managerial periphery. Secondly, an onset of the tiniest or radiance value was used to divide the images hooked on built-up non-urban area.
The extracted area and the different statistical data which gives an absolute value grouping of the differences between the threshold values of different statistical data were chronicled. The recapitulation of different threshold values such a process increases the optimum value of night-time light data and reaches the extreme pixel value of the image. The difference in the maximum and minimum value of the NTL-based data provides the threshold value for the built-up extraction of the urban areas.
3.3 Inter-calibration of the Night-Time Light Dataset
For the different dramatic mapping picture of urbanization through large city light monitoring, the use of night-time images of the earth shows visible light secretions which have become a great interest in recent times (Small et al. 2011). The night-time light data do not quantify land shelter unswervingly, but most of the non-urbanized places which have light during night-time, considering various features, has been shown a many interrelated with the populace density (Lo 2001). Nevertheless, but the DMSP-OLS night-time light series dataset is not being able to rightly be used for studying suburbanization due to the absenteeism of on-board setting in the DMSP-OLS dataset (Miller et al. 2009; Liu et al. 2012). The data used in this paper (1992–2012), which is obtained from different NTL satellites contains no firm inter-calibration. Direct utilization of NTL data cannot be done due to quotation the undercurrents of worldwide and local built-up urban increase because of the deficiency of comparability and endurance revenue of NTL data. To dominate the inconsistencies and instruct confusability to the NTL dataset, the NTL data needs to be inter-calibrated which is indispensable to strict use. The stability and various comparisons to these data of NTL time series data, which we take from 1992 to 2012 for DMSP-OLS data, are monitored by a statistical data formulation of second-order regression algometry that improves data solidity (Elvidge et al. 2009).
In this study, we have created 20 random points in each year data of regional area within a unit loop so that every dataset can cover a unique and equally distributed data. The total random points taken are 20, and a sample version of this pictorial view is given below in the figure (Fig. 10.2).
The DN (digital number) values from each random point are extracted using extract values from point calibration. And the different sets of a DN value from every year data (1992–2012) are extracted. The random points which extracted the values were then sampled, and by using the second-order polynomial equation, the regression value from each set is extracted. These illustration data of Australian Capital Territory (ACT) were extracted from each year data (1992–2012) (ACT, urban area and semiurban). In the process of swotting the night-time light series dataset, it has initiated that the data from the satellite −2003 (F152003) apprehended with extreme numeral of stricken pixels in the region of Australian Capital Territory. From the year 2003, the main urban area begins to encompass in the form of different sides. The extracted various DN values of the raster image are shown in Table 10.1.
The data inter-calibration of DMSP-OLS satellite was steered using the regression formula of second-order polynomial regression (In Eq. 10.1), relaying on capricious as the reference image and self-regulating inconstant as images to be calibrated.
The DN values which were extracted from 20 random points were inter-calibrated by using Eq. 10.1, where the reliant flexible values are used as the reference data to use the regression equation. The typical restrictions a, b and c were appraised with conventional slightest tetragonal relapse equation for specific NTL image dataset (Pandey et al. 2013) (Fig. 10.3).
The core-centred model of urban indices in two different circles shows that the centred area is how much expanded accordingly with the evaluation of time. The second image correlates the random points to extract the DN values, where DN 53–63 belongs in core mid area, 41–52 belongs to second circle and 0–40 belongs to last circle. This is how about the random points show their significance in this core research.
3.4 Statistical Data for Measuring ULI
In this research, NTL time series study period (1992–2012) was separated into eight periods (1992–2012) with a hiatus of 3 years. F10 for 1992, F12 for 1995 and F16 for 2006 were particularly selected due to elevated coefficients than the erstwhile substitute satellite of F14 and F15 which was used for the year 1998, 2000 and 2003, respectively (Table 10.2). Demographic transition indicator like urbanization, economical population, economic growth, integration of industrial progression in the national gross domestic product (GDP) and built-up area are obtained from the Australian Capital Territory (ACT) (1992, 1995, 1998, 2003) (ABS 2017).
3.5 Frequency of Calibration Data
The change in lighted space is the number of adjoining lights for changed recognition regularity inceptions. Increasing threshold from 14% to 20%, the total lighted area monotonically regulates, but the number of adjoining lights primarily intensifies as large cities splinter from the data of DMSP-OLS. As inception value of light increases supplementary on the number of lights moderates as greater quantities of slighter or less frequently area are detected, the data of night-time lights are hampered. The following figures of different years which comprised two graphical representations—before calibrated and after calibrated—give the intensification of urbanization increase in the different years (1992–2012). A frequency threshold of 14% value resulting in the detection of real urbanization from the NTL time series data gives the individual pixel value for the DN ranges in the dataset 1992–2012. Threshold of 10% exploits the numerical value of lights in the 1994–2012 dataset (Fig. 10.4).
3.6 Inter-calibrated Resulted Urban Light Index (ULI)
In this study paper, the different versions of DMSP/OLS NTL data series were used where version 4 data of different satellites, viz., F10, F12, F14, F15, F16 and F18, is used as the coverage of the data is 149°E and 35°S. The bit of DMSP/OLS data is 6 bit, and the range of digital number for each pixel is 0–63. The concentration of the area’s light unswervingly signposts assessment of this value. The zero value of DN in each pixel is from the non-light area. Pixel with 63 DN value is an inundation pixel, and much blizzards are instituted in the fundamental built-up urban area (Elvidge et al. 1997; Letu et al. 2012). The grid data of the night-time light data images accounts the intensity value of the DN and latitudinal magnitude evidence of ACT. The different light areas which are growing or increasing are redirected in association with the marginal areas that are promising (Imhoff et al. 1997). This partakes in the consequence for the broadening of small reimbursements and intensifying the borders of large cities which are expanding and can be validated by NTL data. Therefore, these attributes are accustomed to paradigm expanse sunlit catalogues. The shrewdness formula for urban light index is (Yi et al. 2014):
The ULI indicates the urban light index, whereas DNirefers to the calibrated DN value from Eq. 10.1. sumC is the count of total DN values in a unit area. By Eq. 10.2, the value of urban light index for the different data taken from 1992 to 2012 is calculated, and finally the main outcome of urban sprawl map is determined with a final generalized value.
4 Results and Discussion
4.1 Light Detection Threshold Information Retrieval
The light data series of DMSP/OLS NTL have several important properties with some percentage threshold value and their discrepancy in size occurrence disseminations. The minimum inception seen in the 1992 dataset is the value of 14% and which increases as on 2000-year dataset that provides a useful blue-pencilled to exploit the number of the individual lights thus corresponding to diminution of insignificant different lights with the lessening of latitudinal data completed magnitude over around large cities of ACT. The size occurrence dispersals of the threshold value increase from the 14% value to the 20% over the sharp intensification in the value of threshold where a tiny value of light can distribute the DN value which perseveres to intensification of the threshold value. Another appearance of the tributary mode suggests more areas observed at lower than 14% to 20% of the peak value of threshold value of NTL data; interstitial prospering among reimbursements of the ACT moderately grows than individual small light areas. The maintenance of this allegation of the centroid of NTL data occurrences dissemination. As they show that the dissemination of specific decorations, which mountaintops around 14% beginning and dewdrops accelerating at lower thresholds (Welch 1980). The data observation of different years (1992–2012) recommends that furthermost of the lighted DN value (pixel value) within the threshold range of 14–20% belongs to light blooming on the sideline of the grander reimbursements. The areas which are distinguished in the regression value R2 < 50% corresponds the total area which threshold value belongs within the 14–20% for the dataset 1992–2012 correspondingly (Small 2002). The stratum of 14% threshold value which significantly reduces the up-and-coming threshold DN ranges in many different tempering small settlements also. To retrieve the extreme information from the NTL time series data, a minimum of 14% threshold value for data collecting is used for significant guesstimate of ACT size. Comparable threshold value during 1992–2012 dataset occurs at 17% exposure in this study research for total lighted area and 14% for the non-lighted area.
4.2 Urban Light Indexation (ULI) of NTL Time Series Data
The hardness of urban area level extraction from any data is not easy based on an administrative area because of the great transformations whichever in spatial extent or populace of the dataset. The minimization of this problem levels the study of need of DMSP/OLS dataset which is thoroughly discussed in this paper. In the comparison of the different scale expansions of the urban areas with the total data, a process of fixing the whole data by a filtering through the ULI (urban light index) gives the distinct urbanization boundary from the entire data. Here the study area which comprises of 20 random points and the extracted DN values enhances the urban centre, and to show the entire urbanization, we use a perfect buffer zone for the whole random points that can retreat the entire DN value extraction easily. The given following figures show the increase in ULI value from the different datasets (1992–2012) in Australian Capital Territory (Fig. 10.5).
As the most developed region in the northern Australian Capital Territory (ACT), the Canberra region shows a centre of urbanization which is increasing in time extension. The increasing evolution of the urbanization, the population and economy is the main factor for the growing activities of the ACT in the Canberra region. The system for the northern Australian Capital Territory is integrating towards a wide range in the year from 2000 to 2012 in comparison with the year from 1992 to 2000. The main axis of growing in the southern ACT region is Canberra of ACT. The other cities including Canberra are Acton, Parkes, Melba, Hall, etc. The demography of the ACT shows the actual integration of urbanization in comparison to the population increase with different respective years.
5 Discussion
The suburbanization development parades palpable terrestrial altitudinal powers. DMSP/OLS dark light data was converted for efficient expansion inquiry than the rest daytime satellite data for the succeeding two explanations: the first one is the altitudinal tenacity of DMSP/OLS NTL data is 1–1.2 km, and anthropological domestic expanses are essential for provincial suburbanization inquiry; on the other hand, NTL data is alongside a dusky family, and the night light data metaphors confiscate the troubles of terminated evidence on city surface settings in afternoon metaphors so they can thoughtfully internment the framework of the conurbations, which is advantageous for confiscating urban astrophysical suggestion. The present paper utilizes ULI which is instituted to scrutinize along with quantitative estimation of the speedy and method of suburbanization by incorporating DMSP/OLS NTL Data from the period from 1992 to 2012. The municipal well-lit concentration is incapable to replicate the agglomeration flat of a city’s inhabitants and pecuniary doings, whereas dissimilarity in Metropolitan Dark Space records the trace of municipal space enlargement. For associating levels of suburbanization in unlike cities under the identical average development of unit circle assessment were recognized in this broadside to painstakingly investigate the suburbanization process in ACT in gently years (1992–2012). Substantial variations are found in reverted cities and populous without slanting comparability predisposed by influences as antiquity, position and policy implementation. Hence, suburbanization research is of vital significance in order to ascertain the progression and advancement of urbanizations. Unit circle model up to certain hefty magnitude eradicates the deficiency of urbanization exploration with the directorial area as the appraisal unit. This is prototypically relevant for estimating research of expansion levels midst cities indoors as a province. This urban light index (ULI) is gauged to study the recompenses of weaknesses and the attainability of studies on urbanization. Results showed wider application of ULI in monetary needles, extent of built-up areas in cities and urban inhabitants.
Arrangement of urban light space as times gone by of expansion which effectively imitate the detached landscapes of town space fruition. There is rectilinear bearing among the urban inhabitants, monetary movement, built-up cover and ULI linearity (the correlation coefficients with 70–75%). This study embryonically utilized urban light space for the first time. In agreement with diverse supplies in urban light concentration, the urban light space is classified into central urban area (CUA) (DN 57–63), urban fluorescence space (DN 15–25), suburban changeover zone (SCZ) (DN 45–57) and outlying area (OA) (DN 25–45). From the four light spaces observed built-up types, time-based three-dimensional growth patterns from the time-based and longitudinal dissimilarity of the ACT are given due to consideration of the present. Outcomes derived by analysing built-up cover and ULI are closely associated in course of urbanization with spatial pattern of cities and their geographical distribution.
References
Australian Bureau of Statistics (ABS) (2017) Population projections, Australia, 2016 (base) to 2101. ABS cat. no. 3222.0. Canberra: ABS
Cinzano P, Falchi F, Elvidge CD, Baugh KE (2001) The artificial sky brightness in Europe derived from DMSP satellite data. Preserving the astronomical sky, pp 95–102
Dewan AM, Yamaguchi Y (2009) Land use and land cover change in Greater Dhaka, Bangladesh: using remote sensing to promote sustainable urbanization. Appl Geogr 29:390–401
Elvidge CD, Baugh KE, Kihn EA, Kroehl HW, Davis ER (1997) Mapping city lights with nighttime data from the DMSP operational linescan system. Photogramm Eng Remote Sens 63(6):727–734
Elvidge CD, Baugh KE, Dietz JB, Bland T, Sutton PC, Kroehl HW (1999) Radiance calibration of Dmsp-Ols low-light imaging data of human settlements. Remote Sens Environ 68(1):77–88
Elvidge CD, Tuttle BT, Sutton PC, Baugh KE, Howard AT, Milesi C, Bhaduri B, Nemani R (2007) Global distribution and density of constructed impervious surfaces. Sensors 7(9):1962–1979
Elvidge C, Ziskin D, Baugh K, Tuttle B, Ghosh T, Pack D, Erwin E, Zhizhin M (2009) A fifteen year record of global natural gas flaring derived from satellite data. Energies 2:595–622
Feng Y, Kugler J, Zak PJ (2002) Population growth, urbanisation and the role of government in China: a political economic model of demographic change. Urban Stud 39:2329–2343
Fujita M, Krugman P, Mori T (1999) On the evolution of hierarchical urban systems. Eur Econ Rev 43(2):209–251
Han J, Hayashi Y, Cao X, Imura H (2009) Application of an integrated system dynamics and cellular automata model for urban growth assessment: a case study of Shanghai, China. Landsc Urban Plan 91:133–141
He CY, Shi PJ, Li JG, Chen J, Pan YZ, Li J, Zhuo L, Toshiaki I (2006) Restoring urbanization process in China in the 1990s by using non-radiance calibrated DMSP/OLS nighttime light imagery and statistical data. Chin Sci Bull 51:1614–1620
He CY, Ma Q, Li T, Yang Y, Liu ZF (2012) Spatiotemporal dynamics of electric power consumption in Chinese Mainland from 1995 to 2008 modeled using DMSP/OLS stable nighttime lights data. J Geogr Sci 22:125–136
Henderson M, Yeh ET, Gong P, Elvidge C, Baugh K (2003) Validation of urban boundaries derived from global night-time satellite imagery. Int J Remote Sens 24(3):595–609
Huang X, Schneider A, Friedl MA (2016) Mapping sub-pixel urban expansion in China using Modis and Dmsp/Ols nighttime lights. Remote Sens Environ 175:92–108
Imhoff ML, Lawrence WT, Stutzer DC, Elvidge CD (1997) A technique for using composite DMSP/OLS ‘City Lights’ satellite data to map urban area. Remote Sens Environ 61:361–370
Letu H, Hara M, Tana G, Nishio F (2012) A saturated light correction method for Dmsp/Ols nighttime satellite imagery. IEEE Trans Geosci Remote Sens 50(2):389–396
Li SM (2004) Population migration and urbanization in China: a comparative analysis of the 1990 population census and the 1995 national one percent sample population survey. Int Migr Rev 38:655–685
Li Q, Linlin L, Weng Q, Xie Y, Guo H (2016) Monitoring urban dynamics in the Southeast U.S.A. using time-series Dmsp/Ols nightlight imagery. Remote Sens 8(7):578
Liu ZF, He CY, Zhang QF, Huang QX, Yang Y (2012) Extracting the dynamics of urban expansion in China using DMSP-OLS nighttime light data from 1992 to 2008. Landsc Urban Plan 106:62–72
Liu X, Guohua H, Bin A, Xia L, Shi Q (2015) A normalized urban areas composite index (Nuaci) based on combination of Dmsp-Ols and Modis for mapping impervious surface area. Remote Sens 7(12):17168–17189
Lo CP (2001) Modelling the population of China using DMSP operational line scan system nighttime data. Photogramm Eng Remote Sens 67:1037–1047
Lo CP (2002) Urban indicators of China from radiance-calibrated digital DMSP-OLS nighttime images. Ann Assoc Am Geogr 92:225–240
Lo CP (2010) Urban indicators of China from radiance-calibrated digital DMSP-OLS nighttime images. Ann Assoc Am Geogr 92(2):225–240
Lu D, Tian H, Zhou G, Ge H (2008) Regional mapping of human settlements in southeastern China with multisensor remotely sensed data. Remote Sens Environ 112(9):3668–3679
Ma T, Zhou C, Pei T, Haynie S, Fan J (2012) Quantitative estimation of urbanization dynamics using time series of Dmsp/Ols nighttime light data: a comparative case study from China’s cities. Remote Sens Environ 124:99–107
Mertes CM, Schneider A, Sulla-Menashe D, Tatem AJ, Tan B (2015) Detecting change in urban areas at continental scales with Modis data. Remote Sens Environ 158(0):331–347
Milesi C, Elvidge CD, Nemani RR, Running SW (2003) Assessing the impact of urban land development on net primary productivity in the southeastern United States. Remote Sens Environ 86:401–410
Miller JD, Knapp EE, Key CH, Skinner CN, Isbell CJ, Creasy RM, Sherlock JW (2009) Calibration and validation of the Relative differenced Normalized Burn Ratio (RdNBR) to three measures of fire severity in the Sierra Nevada and Klamath Mountains, California, USA. Remote Sens Environ 113:645–656
Owen TW (1998) Using DMSP-OLS light frequency data to categorize urban environments associated with US climate observing stations. Int J Remote Sens 19:3451–3456
Pandey B, Joshi PK, Seto KC (2013) Monitoring urbanization dynamics in India using DMSP/OLS night time lights and SPOT-VGT data. Int J Appl Earth Obs Geoinf 23:49–61
Runkui L, Zhipeng L, Wenju G, Wenjun D, Qun X, Xianfeng S (2014) Diurnal, seasonal, and spatial variation of PM2.5 in Beijing. Sci Bull 60(3):387–395
Schneider A, Friedl MA, Potere D (2010) Mapping global urban areas using Modis 500-M data: new methods and datasets based on ‘urban ecoregions’. Remote Sens Environ 114(8):1733–1746
Shi K, Huang C, Yu B, Yin B, Huang Y, Wu J (2014) Evaluation of NPP-VIIRS night-time light composite data for extracting built-up urban areas. Remote Sens Lett 5(4):358–366
Shu S, Yu B, Wu J, Liu H (2011) Methods for deriving urban built-up area using night- light data: assessment and application. Remote Sens Technol Appl 26:169–176
Small C (2002) A global analysis of urban reflectance. In: D.M.a.F.S.-E.C. Jurgens (ed) Proceedings of the third international symposium on remote sensing of urban areas. Istanbul, Turkey
Small, C. (2003). High spatial resolution spectral mixture analysis of urban reflectance. Remote Sens Environ, 88(1–2), 170–186
Small C (2005) A global analysis of urban reflectance. Int J Remote Sens 26(4):661–681
Small C, Pozzi F, Elvidge CD (2005) Spatial analysis of global urban extent from DMSPOLS night lights. Remote Sens Environ 96:277–291
Small C, Elvidge CD, Balk D, Montgomery M (2011) Spatial scaling of stable night lights. Remote Sens Environ 115:269–280
Su Y, Chen X, Wang C, Zhang H, Liao J, Yuyao Y, Wang C (2015) A new method for extracting built-up urban areas using Dmsp-Ols nighttime stable lights: a case study in the Pearl River Delta, Southern China. Gisci Remote Sens 52(2):218–238
Sutton PC (2003) A scale-adjusted measure of “Urban sprawl” using nighttime satellite imagery. Remote Sens Environ 86:353–369
Sutton P, Roberts D, Elvidge C, Baugh K (2001) Census from Heaven: an estimate of the global human population using night-time satellite imagery. Int J Remote Sens 22(16):3061–3076
Tan L (2017) Australian states and territories: New South Wales, 2017, Geography & Environment/Geo/Env: Countries/Continents/Geo/Env: CC – Australia/Oceani. ISBN 9780994624710
Welch R (1980) Monitoring urban-population and energy-utilization patterns from satellite data. Remote Sens Environ 9(1):1–9
Welch R, Zupko S (1980) Urbanized area energy-utilization patterns from Dmsp data. Photogramm Eng Remote Sens 46(2):201–207
Wigmore L (1971) Canberra: history of Australia’s national capital. Dalton Publishing Company. ISBN 0-909906-06-8
Yi K, Tani H, Li Q, Zhang J, Guo M, Bao Y, Wang X, Li J (2014) Mapping and evaluating the urbanization process in Northeast China using DMSP/OLS nighttime light data. Sensors 14(2):3207–3226
Zhang J, Zhu T, Kipen H, Wang G, Huang W, Rich D et al (2013) Cardiorespiratory biomarker responses in healthy young adults to drastic air quality changes surrounding the 2008 Beijing Olympics. Res Rep Health Eff Inst 174:5–174
Author information
Authors and Affiliations
Editor information
Editors and Affiliations
Rights and permissions
Copyright information
© 2019 Springer Nature Switzerland AG
About this chapter
Cite this chapter
Elvidge, C.D., Kalita, H., Choudhury, U., Rehman, S., Tripathy, B.R., Kumar, P. (2019). Inter-calibration and Urban Light Index of DMSP-OLS Night-Time Data for Evaluating the Urbanization Process in Australian Capital Territory. In: Kumar, P., Rani, M., Chandra Pandey , P., Sajjad, H., Chaudhary, B. (eds) Applications and Challenges of Geospatial Technology. Springer, Cham. https://doi.org/10.1007/978-3-319-99882-4_10
Download citation
DOI: https://doi.org/10.1007/978-3-319-99882-4_10
Published:
Publisher Name: Springer, Cham
Print ISBN: 978-3-319-99881-7
Online ISBN: 978-3-319-99882-4
eBook Packages: Earth and Environmental ScienceEarth and Environmental Science (R0)