Abstract
Accurate assessment of physical activity (PA) is important to study the associations between PA and health outcomes, to evaluate the effectiveness of interventions and to derive public health recommendations. Despite limitations, accelerometry-based methods generate the best available measures for epidemiological research involving a large number of children and adults. In this chapter, we review the most important methodological issues pertaining to the use of accelerometers to assess the overall volume of PA. We stress the importance of recording and keeping the raw data whenever possible. We review the validation studies using accelerometry to determine energy expenditure and calibration studies that attempt to derive thresholds (“cut-offs”) for differentiating between activity intensity categories. Conceptual and measurement issues due to the use of different thresholds are reviewed, as well as the temporal resolution issues such as sampling rate and epoch length. Different wear time detection algorithms and inclusion criteria are reviewed as well as options in data reduction (deriving meaningful variables from accelerometer data). We present an R package automatising most of the steps in accelerometer data analysis. The chapter concludes with some insights into the future of accelerometry given the wearable revolution and logistical considerations in using accelerometers in large field studies.
On behalf of the IDEFICS and I.Family consortia
Access provided by Autonomous University of Puebla. Download chapter PDF
Similar content being viewed by others
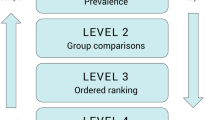
Keywords
These keywords were added by machine and not by the authors. This process is experimental and the keywords may be updated as the learning algorithm improves.
7.1 Introduction
7.1.1 Physical Activity and Health
Physical activity (PA) can be defined as any bodily movement produced by skeletal muscles that result in energy expenditure (EE) (Caspersen et al. 1985). PA is related to all-cause mortality (Lee and Skerrett 2001); we therefore need objective methods of PA assessment to elucidate the dose–response relationship between PA and health outcomes. Evidence of the detrimental effects of a sedentary lifestyle on the health of children is constantly growing (Dencker and Anderson 2008). For example, an association between inactivity and childhood obesity is now generally accepted (Miller et al. 2004; Ekblom et al. 2004; Trost et al. 2001). On the one hand, obese children and adolescents are prone to significant short- and long-term health problems such as cardiovascular diseases, hyperlipidaemia, hypertension, glucose intolerance, type 2 diabetes, psychiatric disorders, and orthopaedic complications (Miller et al. 2004; Reilly et al. 2003) and have an increased risk of developing adult obesity (Whitaker et al. 1997; Mossberg 1989). On the other hand, increasing PA can counter the adverse effects of childhood obesity such as reducing visceral fat (Byrd-Williams et al. 2010). The effects of PA are not confined to the risks associated with obesity and overweight: the direct and indirect effects include bone health, muscular fitness, mood disorders, and cognitive ageing (Miles 2007; de Vet and Verkooijen 2018).
7.1.2 Physical Activity Guidelines
In view of alarming levels of sedentariness and physical inactivity, several PA guidelines and recommendations have been worked out. In the global recommendations compiled by the expert group appointed by the World Health Organization (WHO), low level of PA is mentioned as fourth leading risk factor of mortality (World Health Organization 2010), preceded by high blood pressure, tobacco use, and high blood glucose and followed by overweight and obesity. It is noteworthy that PA is often mentioned as an effective strategy of prevention regarding the other leading causes of mortality (e.g. hypertension: Whelton et al. 2002; overweight and obesity: Tremblay et al. 2005; Jakicic and Otto 2005; Ortega et al. 2007). The WHO guidelines recommend for children and young people:
-
(a)
Accumulating at least 60 min daily moderate-to-vigorous physical activity (MVPA), whereas more than that amount would provide additional health benefit;
-
(b)
Most of the activity should be aerobic, some of it should be of vigorous intensity, and bone- and muscle-strengthening activities should be done at least three times a week.
Several national guidelines [e.g. US (Barlow and Expert Committee 2007), UK (Bull and Expert working groups 2010), and Estonia (Pitsi et al. 2017)] recommend reducing sedentary (sitting) time. For example, the American Academy of Pediatrics (Barlow and Expert Committee 2007) has recommended that television and video time should be restrained to a maximum of 2 h per day for the prevention of paediatric overweight and obesity and resultant comorbidities. There is emerging evidence that the health effects of sedentariness go beyond those of lack of PA (Zhou and Owen 2017).
The promotion of PA among children is important for its direct health effects but also because of its potential to instil lifelong behaviour patterns that, if maintained into adulthood, will result in a more active and physically fit adult population (Sallis and Patrick 1994; Twisk et al. 1997). This notion is dependent on the assumption that PA is tracked from childhood to adulthood; there are not many long-term tracking studies using objective methods (the short-term studies in children and adolescents have typically found moderate tracking, e.g. Rääsk et al. 2015a), whereas moderate to high stability of PA is found using parental and self-reports over several decades (Telama et al. 2014).
A second indirect effect of PA in childhood is the development of both physical fitness (Ortega et al. 2015) and fundamental movement skills (Lubans et al. 2010; Barnett et al. 2009) that are important on their own right, and also as enabling a wider choice of PA later in life.
7.1.3 Importance of Physical Activity Assessment
The current focus on accurate assessment of PA levels is essential for the determination of the dose–response relationship between PA and health outcomes (Wareham and Rennie 1998). For example, valid and reliable measures of PA are necessary for studies designed to (1) determine the association between PA and health outcomes; (2) document the frequency and distribution of PA in defined populations; (3) determine the level of PA required to influence specific health parameters; (4) identify the psychosocial and environmental factors that influence PA behaviour; and (5) evaluate the effectiveness of health promotion programmes to increase habitual PA in individuals group or communities (Wareham and Rennie 1998; Trost 2007).
Questionnaires have been widely used in PA assessment due to their affordability and ease of administration. Depending on the type of the study, a questionnaire may be the only feasible option. The differences between questionnaires and objective methods are not confined to the former being less accurate—using questionnaires, one should consider that the information is based on self-perception and memory, and is always multidimensional, reflecting not only the behaviour of interest but also concerns like self-concept and self-presentation. Therefore:
-
(a)
Questionnaire assessments of PA may be subject to misclassification, to a different degree in different respondents (Gorber and Tremblay 2016).
-
(b)
Respondents may be particularly poor at estimating the volume or frequency of a behavioural category that is artificial from their point of view (e.g. light-intensity activity or, in general, any unstructured activity), but they may be quite reliable in estimating the frequency of personally meaningful behaviours or other relevant facts (e.g. the frequency of walking from home to school or the distance from school to home) (Rääsk et al. 2015a, 2017).
-
(c)
Questionnaire assessments may not be sensitive to change or may change differently from the behavioural change; for example, Rääsk et al. (2015a) found a considerable decline in objective PA in adolescents over 2 years, but no change in self-reports and only a very small decline in parent reports.
Consequently, questionnaires can be highly useful in initial stages of research and in assessing certain types of questions, but their use is highly problematic when one intends to estimate quantitative relationships, for example dose–response relationships between PA and health outcomes, or the effect of an intervention. Caution must be exerted when treating questionnaire data as quantitative, even if they are expressed in terms of physical units such as minutes of MVPA. Potentially, questionnaires can complement accelerometry-based assessment in several ways, e.g. regarding the types of activities that the participant has performed.
PA is a complex and multidimensional phenomenon; in every study, one must make some choices as to which parameters to assess. From a public health point of view, one of the most important parameters is the total volume of PA. This can be expressed (a) as daily or weekly energy expenditure in physical units, (b) in arbitrary units, e.g. “counts” per minute (CPM), (c) in natural units, e.g. daily steps, (d) in units referring to time in intensity categories, e.g. daily minutes of MVPA. In addition to the MVPA, the importance of light activity is being emphasised in more and more studies (Powell et al. 2011; Levine 2004). More complex variables that can be of public health interest are breaks in sedentary activities (Healy et al. 2008; Bailey and Locke 2015) and “bouts” of either MVPA or light activity—that is, periods where a certain intensity level has persisted for at least a certain amount of time (Mark and Janssen 2009). These variables can be easily derived from accelerometer output and are directly related to PA recommendations.
Some more specific health-relevant aspects of PA may be more difficult to assess with accelerometers. For example, for the fundamental movement skills to develop in an optimal way, it is important that the child has a reasonable amount of practice at a range of different activities (e.g. throwing, balancing, jumping, running). These skills could be tested directly and assessed via self-report or proxy reports, but using just one sensor on the waist it is probably impossible to figure out how often the sensor bearer has engaged in the act of throwing something. The same logic applies to bone- and muscle-strengthening exercises, as well as balance exercises: at least, using only one sensor, it is probably impossible to tell whether these have been performed in recommended amounts. The motivation, enjoyment, or interest in PA may play a role in the lifelong continuity of PA behaviours, but are, again, difficult to assess with accelerometry-based devices. Depending on the research question, it may thus be of crucial importance to complement accelerometry-based data by assessments of fitness and skills, and/or self- or proxy reports of motivation, enjoyment, and context.
Not all aspects of PA that are relevant to any given health outcome are fully known. For example, research into the chronobiology of PA has only recently started, but there are data showing that a fragmented rhythm of daily PA is associated with lower cardiorespiratory fitness and higher metabolic risk (Garaulet et al. 2017). This example is one among the many showing that the public health relevance of the data collected in large-scale studies using accelerometry-based PA assessment is not limited to a few summary variables that are typically used. It is thus of crucial importance to find ways to keep the data in their original form, not just the summary variables.
The IDEFICS study is one of the largest European studies on childhood obesity and includes eleven countries and more than 16,000 children aged between 2 and 9.9 years (Bammann et al. 2006; Ahrens et al. 2011). One of the aims of the IDEFICS study was to investigate the primary factors leading to childhood obesity by assessing the lifestyle patterns of children within the European Union in order to develop suitable interventions aimed at countering the obesity epidemic. A number of novel interventions were utilised within the IDEFICS study in order to improve health awareness and encourage healthy eating and physical activity in children. The IDEFICS study is the first large-scale study to assess PA objectively (i.e. accelerometry) among preschool and primary school children (Ahrens et al. 2011).
The I.Family study continued the IDEFICS study with a focus on the familial, social, and physical environment to assess the determinants of eating behaviour and food choice and its impact on health outcomes (Ahrens et al. 2017).
7.2 Accelerometry-Based Activity Monitoring
An activity monitor must be able to detect motion, transform the motion information into some usable units, and store this information over a period of time. A simple mechanical pedometer (or passometer in earlier use) may contain a pendulum that would move back and forth with every step and some mechanism to make a gear wheel advance by one position with every movement of the pendulum, etc. Mechanical pedometers can detect steps with some accuracy but are, by definition, restricted by the concept of step (thus ignoring the intensity and speed dimensions); in addition, pedometers are not good at detecting slow-paced movement (Martin et al. 2012). Accelerometry-based monitoring provides a reasonable compromise between validity and feasibility (see the review by Esliger and Tremblay 2007). More affordable methods such as diaries and pedometers have lower validity; methods with higher validity such as direct observation, calorimetry, and doubly labelled water (DLW) tend to be costly and more burdensome to the participants. In comparing accelerometry with the latter methods with better validity, one must notice that accelerometry-based monitors offer an excellent temporal resolution (differently from DLW) and, at the same time, good ecological validity (participants can carry the equipment with them for weeks and, in principle, years, with no major interference for their daily life—differently from both observation and calorimetry).
The term accelerometer means, in strict sense, only the sensor, which is a crucial but not the only component of the activity monitor. Today, most of the activity monitors contain a capacitive “microelectromechanical systems” (MEMS) or a piezoelectric sensor (John and Freedson 2012). MEMS sensor is used, for example, in the devices used in the IDEFICS and I.Family studies: ActiGraph models GT1M and GT3X, ActiTrainer, as well as the 3DNX that was used in the IDEFICS validation study (Bammann et al. 2011; Ojiambo et al. 2012; Horner et al. 2011). A piezoelectric sensor is used, for example, in Actical, as well as older models from ActiGraph such as AM 7164 (John and Freedson 2012). As to our knowledge, there is yet no systematic comparison of the advantages and disadvantages of different sensor types in activity monitors. ActiGraph’s decision to change the sensor type seems to have been motivated by cost efficiency and feasibility, whereas the claim is that the later and the earlier models yield comparable results (John and Freedson 2012). There are studies comparing devices with different sensors: for example, Fudge et al. (2007) have compared sensors of a different type (piezoelectric sensor in CSA 7164 vs. MEMS sensor in GT1M and GT3X), but these comparisons are confounded with differences in firmware.
Studies of technical variability support the notion that MEMS sensor used in GT3X is reliable in estimating PA in the frequencies that are common to most types of human daily activities (Santos-Lozano et al. 2012).
Bouten et al. (1997) have reviewed studies on human body acceleration and concluded that a sensor with amplitude range of about −6 to + 6 G and with frequency range of about 20–100 Hz would be sufficient if the sensor is placed at waist level. One cannot but notice that several older devices, including GT1X, fall short of this requirement; however, it is unclear whether this causes a serious bias in the estimates of daily activity volume.
The activity monitoring device must also contain a unit for filtering and pre-storage processing of the signal. The pre-processing may be done at the hardware level (as in ActiGraph AM 7164), in updatable firmware within the device (as in GT1M), or in the computer when downloading the data (as in GT3X+). Given the memory limitations in the earlier (pre-GT3X) models, storing the raw data over a period of several days was not possible, so the data must have been processed within the device and stored as values aggregated within an “epoch” (this term is used in the literature to denote the time interval of measurement; typical values of an epoch are between 1 and 60 s). Temporary malfunctioning at this level may result in implausible and impossible values (Rich et al. 2013) which must be corrected before analysis. Another set of issues is related to the band-pass filter included in ActiGraph firmware: the signal outside the range of 0.25–2.5 G is markedly attenuated by the software (John and Freedson 2012). This is likely to be the reason for the “plateau” seen in GT1M output when the device is worn while running: the output (counts per minute) rises linearly until a certain speed (9–10 km/h) and then either declines or continues to rise at a much gentler slope (Fudge et al. 2007). Other researchers (possibly using different versions of firmware) have even noticed a decrease in counts with increasing running speed; as a consequence, running at 18 km/h might look identical to running at 8 km/h in the data (John et al. 2010). Indeed, Chen et al. (2012) saw a plateau effect in post-filtered activity in GT3X and in the 60 s integrated data (“counts”), but not in the original pre-filtered data. Due to firmware features, thus, the ActiGraph monitors may underestimate the intensity of vigorous activity. This is, however, unlikely to influence the estimates of the duration of vigorous activity, and it is probably not very influential for estimating the total daily volume of PA, as running faster than 9 or 10 km/h is, regrettably, a rare and short-lived event for most participants in epidemiological studies. Nevertheless, as the reason for the band-pass filter is to exclude the movement frequencies unlikely to occur in humans, it remains to be determined whether a better trade-off can be achieved (i.e. better representation of high-speed running, but not at the expense of including more non-humanly possible movement).
The memory capacity of the ActiGraph devices has been constantly rising: from 64 kB in AM 7164 to 1 MB in GT1M to 512 MB (possibly more) in GT3X+. The recording time will depend on both memory and temporal resolution: choosing a shorter epoch in older models means that the memory is filled up faster.
Note that the recording capacity of the device also depends on the battery capacity. As the battery time tends to shorten with use, it is advisable to check the batteries regularly and charge them occasionally even when the devices are not in use (e.g. between the fieldwork periods).
The sensor, processing unit, memory, and the battery comprise the functional parts of an activity monitor. These are, however, not the only parts that can influence the results. The casing is important to protect the inner workings of the device from the external forces, but one should also consider the aesthetic component: participants are to carry this with them for at least several days. In large studies, the casing is often the first component to show the signs of wear. The belt to attach the device on waist has some important features to pay attention to: (1) ease of use (can it be used to attach the device firmly in an easy way?) and (2) washability.
Nowadays, on the one hand, there is consensus that raw measurements (in physical units) should be used whenever possible, (1) to minimise dependence on proprietary algorithms and filters, (2) to allow maximal comparability between different devices, and (3) to record data at maximal temporal resolution. On the other hand, a large number of studies have used older-generation devices where raw recordings are not available due to memory restrictions. Thus, ActiGraph counts have become a de facto standard of comparison. Some authors have argued for converting the counts back to physical units, but it is only possible to do it approximately (John and Freedson 2012)—so the comparability achieved with this step may be an illusion. The potential benefits that using raw data may once bring have so far remained largely just that: potential benefits. There is no convincing evidence, for example, that using high-resolution data would bring about a qualitative jump in the accuracy of estimation of daily energy expenditure or the gross volume of PA. It is likely that high-resolution data may allow better accuracy at activity recognition, but even this has its limits with a single sensor whose exact location regarding body parameters is not known. Applications with more sensors with more exact placement, however, are not likely to be feasible in large epidemiological studies.
For the time being, thus, while we recognise the need of recording and storing the data at maximum available resolution and in physical units, we restrict the following discussion to the “counts” output of ActiGraph devices. This is admittedly a temporary solution but is relevant as long as the older generation of activity monitors (not capable of recording at high resolution) or data thereof is used.
7.3 Practical and Methodological Issues in Accelerometry-Based PA Assessment
7.3.1 Issues in Choice of the Device
When the IDEFICS study started in 2006, the question of choosing an activity monitor was essentially that of a choice between brands: hypothetically, is ActiX better than ActiY? The decision to use ActiGraph’s GT1M and ActiTrainer devices in the study was based on the widespread use of these monitors and lower costs compared to other devices in the same category. Since then, the focus has slowly but steadily moved towards establishing non-proprietary algorithms and metrics working on raw acceleration data (John and Freedson 2012; van Hees et al. 2013): with this approach, the brands are to be decomposed into functional units such as sensor, memory, and algorithms. From this perspective, a clear and simple recommendation is to select a device that allows recording and downloading raw data in physical units (see, e.g. van Hees et al. 2013; de Almeida et al. 2018; van Hees 2018 on the analysis of such data).
Using physical units and recording raw data are essential for interbrand comparability, for being able to separate sensor-related and algorithmic components of PA assessment and for being able to use any algorithms derived in future. Whether the raw data provide a higher level of precision is open to debate, and the conclusion will probably depend on which parameter of PA one intends to estimate.
Besides openness and validity, one also needs to pay attention to feasibility and affordability. When planning the IDEFICS study, there was a remarkable price difference between activity monitors with uniaxial and three-axial recording. We therefore investigated the question whether the other spatial axes besides vertical add anything worthwhile to the assessment of general volume of PA. In the validation study with doubly labelled water (Ojiambo et al. 2012), we compared two devices: GT1M (only longitudinal/vertical axis) and 3DNX (three axes: longitudinal, mediolateral, and anterior–posterior) and found that while all three axes were correlated with PA energy expenditure (PAEE), only vertical axis contributed unique predictive variance. In terms of variance explained, using the vertical axis alone was better than a combination of all three axes (vector length). The main reason for this result is probably that the movements along the vertical axis are the most laborious because they go, half of the time, in exactly opposite direction to that of gravity. A study by Howe et al. (2009) reached the same conclusion using a different device (RT3): vector magnitude counts were no more predictive of EE than vertical counts (unfortunately, the other axes besides vertical were not analysed separately). In a recent study using GT3X, Chomistek et al. (2017) found the triaxial composite to have a higher correlation with DLW-derived daily and PA energy expenditure than the vertical counts alone. The difference was, however, small (unadjusted correlations between total EE and counts were 0.59 versus 0.55 for women, and 0.58 versus 0.54 for men). It is unfortunate that the raw files were not preserved in that study, so it is not possible to check whether the result depends on proprietary algorithms used to derive counts (e.g. the band-pass filter discussed above; cf. John and Freedson 2012) or would remain the same with open-source algorithms (e.g. van Hees 2018). The main conclusion of our DLW study, however, remains unaltered: the vertical axis counts are the most important in predicting daily EE, with the other axes adding, if anything, only a small fraction of variance. This should not be taken as a recommendation to use uniaxial sensors if there is a choice: on the contrary, more information is generally preferable to less. However, when one is interested in predicting the gross volume of PA, it should not be taken for granted that the “vector magnitude” (vector length) metric should be preferred to the use of vertical axis alone.
7.3.2 Validity and Calibration
In this section, we are mostly interested in methods to estimate the total volume of PA, as well as the time spent in different levels of intensity of activity. Consequently, two types of validation studies are relevant: (a) validating the accelerometer output against doubly labelled water and (b) studies of classifying activities into intensity categories, typically using indirect calorimetry as a criterion. The DLW enables EE to be accurately measured in free-living conditions, which makes it the method of choice in validating the total volume of PA.
The studies relating accelerometer output to DLW have been of variable quality (Plasqui et al. 2013) and have yielded varying results (Sardinha and Júdice 2017); the zero-order correlation of accelerometer output and energy expenditure is, at best, moderate. Below, we would like to point out two studies that have used ActiGraph devices and a similar age group to that of the IDEFICS study.
Butte et al. (2014) validated two complex multivariate prediction models (cross-sectional time series and multivariate adaptive regression splines, described in Zakeri et al. (2013) in more detail) including biometric variables, accelerometer counts, and heart rate (HR) as predictors and achieved good accuracy in prediction (root-mean-square error [RMSE] = 105 kcal/day); the accuracy was slightly lower without including HR (RMSE = 116 kcal/day). Due to the choice of modelling strategy, however, it is not easy to find out the independent contribution of accelerometer counts to the prediction. In addition, even though this strategy may optimise predictive accuracy, it is less optimal for understanding the functional relationships in question.
In the IDEFICS validation study (Ojiambo et al. 2012), we found, using multiple linear regressions, that body mass alone predicted 71% of the variance in daily energy expenditure (EE); vertical counts from an ActiGraph device (ActiTrainer, compatible with GT1M but capable of recording HR as well) added another 11 percentage points. The prediction formula was:
The RMSE for this model was 0.49 MJ/day (approximately 117 kcal), thus not very different from that found by Butte and colleagues using a more complex model (note, however, that this is a biased comparison as Butte’s study included separate model development and testing steps).
The other device included in the study, 3DNX, was equally predictive of the EE: the model R2 increased by 10 percentage points when vertical counts of 3DNX were added to body mass:
where CPMvertical represents the vertical (longitudinal) axis counts of the 3DNX device. The other two axes, however, were not significant when added to the model and had, in fact, negative regression coefficients:
EE = 0.61127 + 0.16328 × weight + 0.01662 × CPMvertical
– 0.00391 × CPManteroposterior – 0.00593 × CPMmediolateral.
It is interesting to note that not only did the counts from anterioposterior and mediolateral axis not reach statistical significance in the model (the p-value for mediolateral axis was 0.066), but their corresponding regression coefficients were negative. It would be premature to offer a substantive interpretation to the sign of these coefficients, but it does seem to indicate that the lack of prediction was not due to insufficient statistical power.
We are far from claiming that our study represents the optimal strategy in predicting DLW from accelerometer output. On the contrary, the relationship between PA, body parameters, and EE is unlikely to be confined to linear main effects. Using allometric modelling as suggested by Carter et al. (2008) may be a better strategy from a substantive point of view, even if the predictive advantage is modest.
One should also note that the percentages of explained variance depend on both the variance in body parameters and the diversity of lifestyles in the population under study. For example, Ojiambo et al. (2013) studied a highly active group, children and adolescents in Kenya, and found that accelerometer counts (average counts per minute) explained about 12% of the variance in daily energy expenditure when added to the model after body weight. Interestingly, time spent in light-intensity activity contributed another 12%. Tentatively, this may mean that time spent in low-intensity activities is an independent predictor of EE, regardless of the intensity of these activities. To see this effect in data, however, one needs a population where there is a large variance in the amount of light-intensity activities: a large share of light PA in Ojiambo’s study was likely to involve going to school and back; the distance between home and school varied from 0.8 to 13.4 km. As another example, Horner et al. (2013) studied a highly active military population (mean PAEE 6.4 MJ/day (SD = 2.3 MJ/day), which is even more than the 5.7 MJ/day (SD = 3.0 MJ/day) in Ojiambo’s very highly active population, and found the largest share of total EE to be explained by body parameters, with accelerometer counts adding only 4%. Superficially, one might think that in a highly active population, the contribution of PA in EE should be higher. This seeming paradox is explained by the highly structured nature of military activities, offering little room for individual choice over the intensity or duration of an activity.
We have heretofore discussed validity as a problem of prediction of variance. In the research using DLW as a criterion, there is little data on validity in the absolute sense, including possible bias in estimating EE from a regression-based model like the ones described above. This would probably depend on not just the device being validated, but also the anthropometric parameters, as well the level and patterning of PA in the population under question. However, predicting the absolute value of EE is rarely a primary objective (among other reasons, because PA recommendations are not expressed in the units of EE); there is more reason to demand accuracy and lack of bias in the estimation of time spent in different categories of activity intensity. For example, to estimate whether a child conforms to the recommendation of at least 60-min daily MVPA, one would need an unbiased estimate of daily minutes of MVPA. To obtain such measures, one needs to calibrate the accelerometer output against some other measure. There are several ways of doing it, but the most common are (1) developing a regression formula relating accelerometer counts to EE and using this formula to find cut-points for successive intensity categories (e.g. Freedson et al. 2005; Treuth et al. 2004; Puyau et al. 2002; Mattocks et al. 2007; Pate et al. 2006) and (2) finding an optimal trade-off between sensitivity and specificity when using accelerometer counts to predict the intensity category of an activity (e.g. Sirard et al. 2005; Evenson et al. 2008; van Cauwenberghe et al. 2010). Butte et al. (2014) implemented a combination of these strategies by using EE via oxygen uptake as a criterion but sensitivity/specificity analysis via receiver operating characteristic (ROC) curves as an analytical approach. In some cases, there is a confusion about which criterion has been actually used. For example, Hislop et al. (2012) have cited the Evenson et al. (2008) study as being based on oxygen uptake (VO2) as a criterion; in fact, \( {\dot{\text{V}}}{\text{O}}_{2} \) was only used to describe the activities and calculating maximum \( {\dot{\text{V}}}{\text{O}}_{2} \), but cut-points were developed based on pre-classified activities. One might thus say that \( {\dot{\text{V}}}{\text{O}}_{2} \) was only indirectly used as validation criterion, as it was used to demonstrate the validity of classification of activity intensities, but it was not used in data analysis to develop cut-points.
Migueles et al. (2017) offer a comprehensive overview of ActiGraph cut-points for all age groups and metrics; a subset (restricted to vertical counts and younger age groups) is shown in Table 7.1. In studies comparing different sets of cut-points, there is no clear winner. The second strategy is specifically targeted for maximising accuracy and minimising bias; thus, it is not surprising that it has worked out best in a comparison using these criteria. Namely, Trost et al. (2011) have concluded that “Of the five sets of cut points examined, only the EV cut points provided acceptable classification accuracy for all four levels of physical activity intensity”. With this in mind, the Evenson cut-points were applied in the IDEFICS study. It is interesting to compare it with two more recent calibration studies by Butte et al. (2014) and van Cauwenberghe et al. (2010). First of all, the cut-points differentiating between light and moderate activity are all at approximately 2200 counts per minute (CPM) (range 2120–2336 CPM, see Table 7.1), differing from each other by no more than 10%. This is roughly consistent with several other studies (Martinez-Gomez et al. 2011), even though some studies suggest a higher threshold of about 3000 CPM (Guinhouya et al. 2011). The thresholds for vigorous activity range from 3520 to 4450 CPM, the largest difference being thus about 20%. The picture is drastically different with the threshold between sedentary and light activity: 100 CPM (25 counts per 15 s) as estimated by Evenson et al. (2008), 240 CPM as estimated by Butte et al. (2014), and 1492 CPM (373 counts per 15 s) as estimated by van Cauwenberghe et al. (2010)—a 1500% difference between the lowest and the highest estimate. The latter cut-point is not uniquely high, being similar to that obtained by Sirard et al. (2005) in a calibration study with direct observation as criterion.
How can one reconcile this degree of divergence? One can first notice that some activities would certainly be misclassified by van Cauwenberghe et al.’s criterion: for example, slow walking would almost certainly produce less than 373 ActiGraph counts per 15 s: the average was 295 in Evenson et al.’s (2008) study for walking at 3.2 km/h, while the mean VO2 was 12.7 ml/kg/min. However, the mean counts for sedentary play were 447.9, that is, more than for slow walking (265.3). The relationship between counts (acceleration measured at waist level) and energy expenditure is thus dependent on activity type, particularly at a low-intensity spectrum. Sedentary free play may involve frequent upper-body movements that cause acceleration at the site of the sensor but do not involve displacement of the whole-body mass like in walking. Maintaining a single threshold for all activities may thus result in misclassifying some of the sedentary activities (e.g. active play) as light or, alternatively, misclassifying some of the light activities (e.g. slow walking) as sedentary. To avoid both of these sins at the same time, one might consider an algorithm similar to Crouter and Bassett’s (2008) or Crouter et al.’s (2012) branched regression approach—applying different formulae depending on the coefficient of variation (CV) of the counts or using different thresholds depending on the position of the body, which can be estimated by the “inclinometer” function included in some more recent devices such as GT3X. However, the accuracy of classification based on CV or inclinometer is far from perfect, which introduces an additional source of error.
In addition, one should take into account that the threshold estimates depend on the design of the study. In Evenson et al.’s (2008) study, for instance, there was a noticeable gap between sedentary and light activities: the average output from the accelerometer was 6.6 counts per 15 s for the highest averaging sedentary activity, whereas the average was 294.7 for the lowest averaging light activity (walking at 3.2 km/h). If this middle range were filled with sedentary and light activities of various grades of intensity, the discrimination would become a more difficult task, and the estimated threshold might shift up or down, depending on how the tasks are selected. Using measured EE values instead of task indicators (as in Butte et al. 2014) may be a partial solution to this problem.
In conclusion, cut-points are not arbitrary criteria, but neither do they represent the absolute truth. Because the relationship between EE and accelerometer output differs across activities, the statistically optimal set of thresholds could be derived in a calibration study with a representative sample of activities. The representative activities would, however, be different across individuals.
Some authors discourage the use of cut-points, arguing that average acceleration (preferably measured in physical units) per time unit is a better summary of the data set and a better predictor of EE than time in intensity categories. This is only partly justified as the estimates of time in intensity categories contain at least two sources of error that are not present when using average acceleration: (1) uncertainty of the threshold estimates and (2) ignoring the differences of intensity within categories; i.e. light activity at 1.51 MET (metabolic equivalent of task) is treated as equal to light activity at 2.99 MET, whereas the first is almost sedentary and the second is almost moderate. These difficulties may be alleviated by converting counts to EE units (METs, calories, or joules) using some of the approximate formulae in the literature or using MET·minutes or count·minutes instead of simple minutes as a statistic. One would need, however, additional calibration studies (or reanalyses of existing studies) to do this in the optimal way, as most of the calibration formulae are based on linear equations, and some have implausible implications. For example, Treuth formula (Treuth et al. 2004) for predicting METs from counts has 2.1 as intercept and a positive slope for counts: that is, the result can never be less than 2.1 METs. In other words, the formula can never predict sedentariness (MET < 1.5), even for 0 counts. In addition, it is possible that the optimal cut-points may depend on the anthropometric parameters such as height or weight.
It is thus clear that using cut-points for activity intensity categories does not only allow a finer description of activity: it also introduces additional sources of error to the estimates. Selecting the best threshold is not always possible as we do not yet have complete information; as a minimum precaution, it is therefore important to recognise that the choice of thresholds may make a difference with huge clinical and biological implications (Pate et al. 2006; Vanhelst et al. 2014a). However, it is an oversimplification to consider using cut-points an ad hoc solution to the imperfections in our data collection procedures that we will eventually get rid of. Indexing of PA as time in intensity categories has a number of shortcomings from a measurement theoretical point of view; however, such summaries are meaningful from a physiological and practical point of view, e.g. when justifying health recommendations or planning interventions. More fine-grained summaries may be useful for more specialised purposes, but one needs to take into account that finer distinctions tend to be less reliable.
Finally, it is a common practice to adjust cut-points when using a different epoch from that of the calibration study. For example, a calibration study found that 25 counts per 15 s can be used to differentiate light activities from sedentariness; using 60 s epoch, the threshold would thus be 100 CPM. This is a potential source of error: the optimal cut-point would probably be slightly different if 60 s epoch was used in the calibration study. There are, however, no studies making this direct comparison; meanwhile, one may conjecture that the difference is likely to be small. It is important, however, to use the exact, unrounded multipliers when adjusting the cut-points. For example, the methods section of one study (Gabriel et al. 2010) contained this sentence: “For classification of 10 s epochs, NHANES threshold values were multiplied by a factor of 0.17 (i.e., 10 s/60 s)”. The value 0.17 is 2% higher than the true value (1/6); hopefully, the authors had used the true value instead of the rounded value, but this is not clear from the text. For comparing studies using different sets of cut-offs and if original raw data are not available, one can use equating based on linear models (e.g. Brazendale et al. 2016).
7.3.3 Accelerometer Placement
Following the general principle that more data are better than less, one should try using as many sensors as possible; in addition, according to the law of diminishing returns, each additional sensor is likely to give less extra information than the previous one. The possibilities are limited by budget, logistics, and burden on participants; all these considerations typically lead to using a single sensor in moderate to large cohort studies.
If a single sensor is used, it should ideally be placed as close to the mass centre of the body as possible (Rosenberger et al. 2013), which means approximately at the hip level. Wearing the device at the back would probably minimise the distance from the mass centre, but placement at left or right side may be more convenient, and the difference in distance is not large. The hip location, being close to the centre of gravity of the body, correlates well with EE associated with movement against gravitational resistance which is a major component of EE as corroborated by the studies referred above, where the vertical axis was the best predictive of EE (Ojiambo et al. 2012; Howe et al. 2009; Chomistek et al. 2017). The right side of the body may be preferred for convenience as most people are right-handed (Corder et al. 2008). Ward et al. (2005) reported that it made very little difference if the accelerometer was worn on the right or left side.
At high ambulatory speeds (>9 km/h), ankle placement may supersede hip (Kyröläinen et al. 2001). This is partly due to biomechanical shifts in the transition from walking to running leading to altered joint kinematics. This is, however, a secondary consideration when assessing children’s overall volume of PA, which only infrequently includes such speeds. Ankle placement may be useful for capturing fast running and biking, and it also probably captures sedentary fidgeting. Thus, it would be most useful in combination with another sensor at the hip level and perhaps as a way of detecting the posture. Moreover, considering the “plateau” effect in ActiGraph devices (and possibly other devices using band-pass filter), one would recommend using either raw signal or other devices when fidelity in capturing locomotion at high speeds is important.
Wrist placement can be more convenient than hip: typically, watches are worn on wrist. As a corollary, this means that if the device does not include a watch, it should be worn on the watch-less hand, which is usually the right hand; so, placement on non-dominant wrist, which seems to be preferable (Sirichana et al. 2017), may be inconvenient for right-handed watch-wearers. Other researchers (Dieu et al. 2017) have found little difference between dominant and non-dominant hand, but there is evidence (Sirichana et al. 2017) that the difference is there for low-intensity activities.
With both wrist and hip placement, one must consider that the correlation between acceleration and EE may be different for different activities. As a thought experiment, imagine giving a pedometer to three persons. Person A is instructed to collect 1000 steps in walking (wearing the device at waist), whereas person B’s task is to hold the device in hand and collect the same number of “steps” while sitting. Person C is instructed to walk while holding the device in his right hand and to raise the hand up above his head with every second step while lowering it at the next step. Which one of them used the most energy? Who covered more distance, A or C?
Now imagine that for yet another person, D, the pedometer is hidden inside a 5 kg dumb-bell; otherwise, he is instructed to walk and move hand in synchrony with person C. He will expend considerably more energy in this process, but chances are that the final step counts will be identical.
On theoretical grounds, one would say that for capturing the overall volume of PA, the wrist placement is suboptimal because in addition to the energy costly counter-gravity movements of the whole body it reflects the less energy costly hand-only movements. These components cannot be disentangled without additional information. Accordingly, several studies have found considerably higher correlations between oxygen uptake and hip-placed sensor as compared to wrist sensor (Rosenberger et al. 2013; Swartz et al. 2000). In one study, however, similar correlations were found (Phillips et al. 2013; r = 0.90 versus 0.97); this result may be partly explained by a small number of activities included in the study and a different metric that was used (average within-individual r’s, which do not adequately reflect the predictability of EE from counts without individual calibration). It thus seems likely that both sites can be used to estimate the overall volume of PA, but site-specific calibration has to be undertaken. For instance, Shiroma et al. (2016) have found that ActiGraph GT3X counts from wrist are up to 5 times higher than for hip, and their variance is higher.
Other sites that could be worth considering include chest (wearing the device in the form of neck pendant, Zhang et al. 2016), shoe (Lin et al. 2016), and ear (Manohar et al. 2009). Several multiple sensor systems have been used, e.g. Wockets (Rosenberger et al. 2013). Studies using multiple sensors have brought about only a modest increase in the predictability of EE from accelerometer data: for example, in one study (Montoye et al. 2016), predictions based on three sites (wrist, thigh, ankle) had slightly higher correlations with EE than the hip accelerometer (r = 0.79 versus 0.72). This is in concordance with the view that counter-gravity movements of the whole body (best captured by a vertical sensor placed near the mass centre of the body) are the major component of EE.
In the second decade of the twenty-first century, it would be difficult not to notice that many people are, most of the time, carrying a cell phone containing an accelerometer sensor. Besides their primary application of rotating the screen orientation when necessary, these sensors are used in PA applications and have been used in research. For example, Manohar et al. (2011) have successfully used iPhone (fixed at back) in PA assessment. In addition to being a success, this study illustrates two difficulties with using cell phone sensors: (1) for reliable PA assessment, the placement of the sensor has to be known (or devised); (2) people use a large number of different devices with considerable variability in accelerometer sensors.
In conclusion, for measuring the overall activity level, the best site for the sensor is as close to the mass centre of the body as possible; hip is the most often used site; other sites (e.g. back at waist level) may be as informative, but each site needs specific calibration. Other sites add little information that would be directly relevant to EE but can be highly useful for activity recognition. In this regard, only crude distinctions, e.g. distinguishing bipedal locomotion (walking, jogging, and running), from other activities might be possible with a single sensor at hip.
7.3.4 Temporal Resolution, Epochs, and Bouts
The question of temporal resolution consists of at least four subquestions: sampling rate of the sensor, sampling rate of the recording, sampling rate used in the analysis, and, finally, minimal length of an episode that one considers to be meaningful or important. These questions are interrelated.
The sampling rate of the sensors is typically in the range of about 20 Hz to about 100 Hz in the commercially available activity monitors. This is probably enough to register the volume of everyday activities (Chen et al. 2012); however, if one is interested in the question of intensity of the load to weight-bearing bones (which ultimately has an impact on bone density), then one may need much higher frequencies, up to 1000 Hz (Kitamura et al. 2009).
Not all of the activity monitors allow storing the original unprocessed measurements. In these cases, the pre-processed and integrated signal is stored, using arbitrary units (counts) and in pre-specified time units (epochs). The length of the epoch depends on the memory capacity of the device and the desired period of observation and, to a lesser extent, on the battery capacity. Within these limits, it is always advisable to select the lowest possible epoch length for recording. Some monitors (such as the newer ActiGraph devices) offer the possibility to download raw data and, at the same time, a pre-processed file with counts using an epoch set by the user. The most useful option is to do both and set the lowest possible epoch value for the file with counts. First, processing the raw data files is time consuming and still more complicated than processing the count data files. One might thus want to do the preliminary or even all of the analyses using the count data. Even in this case, however, it is important to keep the raw measurements (e.g. the .gt3x files in the case of GT3X) as this is the only way to ensure independence from proprietary algorithms. Second, even if one has decided to use, say, 60 s epoch in the analysis, these data can be easily derived from a 1 s epoch file, whereas it is (at least, for the time being) more tedious to derive it from the raw data. In the absence of raw data, it is not possible to go back from a longer epoch (say, 60 s) to a shorter (say, 1 s) one. That is, one can always aggregate if one has the unaggregated data, but, in the absence of time machine, it is not possible to disaggregate the data that are already aggregated. There is a further consideration in choosing the epoch: for comparability with other studies, it is sensible to use epochs that are submultiples of both 60 and 15 (i.e. 1 and 5) as these can be reintegrated to the most commonly used epochs.
If the previous questions have been successfully solved, one has a choice regarding the epoch to use in further analyses. This choice affects some of the summary variables derived from the data (e.g. wear time; time in intensity categories) but not others (e.g. average counts per minute; total counts). There is no simple answer to the question of an optimal epoch, but one should think of biological relevance. For example, consider a single 30 ms (ms) long period of vigorous activity in the middle of 10 min of sedentariness. Does this data point reflect the activity of the organism? In other words, can one say that during a 10-min period, the organism was otherwise sedentary, but vigorously active for 30 ms? This would probably not make sense. But what about 100 ms? 500 ms? 1 s? It seems reasonable to assume that within a few seconds one can do something vigorous, for instance jump, or lift a heavy dumb-bell; it is, however, difficult to imagine any activity that is much faster. Thus, an analytical epoch much lower than “a few seconds” does probably not make sense.
There are a number of studies showing that the choice of epoch has an impact on the estimates of time in intensity categories (Ojiambo et al. 2011; Edwardson and Gorely 2010; McClain et al. 2008). Gabriel et al. (2010) have shown that MVPA estimates had a comparable correlation with self-reported MVPA at 10 and 60 s epochs, but the correlations with meaningful outcome variables (e.g. body weight, body mass index (BMI), fat, and lean mass) were stronger at 60 s epoch. The generalisability of these results is unknown, but in any case this should not be taken as an argument for recording at a higher (60 s) epoch. Rather, one should make a difference between an analytical epoch and the minimum required length of PA episodes to be relevant to health. The latter issue also comes under the name of “bouts”. Gabriel et al.’s (2010) results could mean that the MVPA episodes with shorter duration than 1 min are irrelevant for health. However, integrating the data into longer epochs is not an optimal solution to this question; rather, one should make a difference between very short episodes (<60 s), and not so short (≥60 s) episodes, and compare their respective contributions to the prediction. This is a potential complement to Mark and Janssen’s (2009) result that short (5–9 min) and medium-to-long (≥10 min) bouts of MVPA are incrementally protective of overweight. In future studies, it is recommendable to keep the effects of epoch and bouts analytically separate and analyse different lengths of bouts while keeping the lowest possible epoch.
7.3.5 Wear Time Detection
Wear time detection is of crucial importance if the device cannot be worn continuously for the whole period of observation: for example, in the IDEFICS study, the participants were instructed to remove the accelerometer at night and for certain activities (e.g. taking a shower). With waterproof devices worn on wrist, it is feasible to have an uninterrupted period of observation, but this gives rise to another problem: distinguishing sleep time from wake time.
It has been argued that wear time validation can be considerably more exact using raw data as compared to time-integrated counts: high-resolution data are sensitive to the very small movements which almost invariably occur when the device is worn but can be “integrated out” when the count units are calculated. To our knowledge, no direct comparison between wear time validation methods used in raw and time-integrated data has been made so far. Van Hees et al. (2011) describe a wear time detection algorithm that can be used with wrist-worn accelerometer using raw data. Zhou et al. (2015) describe a method to improve the accuracy of this method by combining it with data from an integrated sensor of body temperature.
In time-integrated data, there are three commonly used algorithms:
-
(1)
Consecutive zeroes method: Janssen et al. (2015) compared different versions of this rule and found ≥20 min to be the best criterion.
-
(2)
Troiano et al. (2008): Non-wear was defined by an interval of at least 60 consecutive minutes of zero activity intensity counts, with allowance for 1–2 min of counts between 0 and 100.
-
(3)
Choi et al. (2011a) method: “1-min time intervals with consecutive zero counts for at least 90 min time window (w1), allowing a short time interval with nonzero counts lasting up to 2 min (allowance interval) if no counts are detected during both the 30 min (window 2) of upstream and downstream from that interval; any nonzero counts except the allowed short interval are considered as wearing”.
Choi et al. (2012) have compared the last two methods and found the latter to be more accurate, and both perform better at vector length metric than on vertical counts.
The choice of a wear time validation algorithm is most influential on the estimates of sedentary time; the other parameters estimated from data (time in intensity categories; average acceleration or counts per time unit) are only influenced if expressed as proportions of wear time.
Another occasionally used method for detecting non-wear time is using diaries (e.g. Ottevaere et al. 2011); this method shares many problems with other self-report methods but could provide useful information if high compliance is ensured and if concordant with the recorded activity data. Our experience in the IDEFICS study is that activity diary is the component with the poorest compliance; one cannot be sure whether all non-wear events are recorded; in addition, the degree of retrospectiveness of the diaries is unknown (i.e. whether the times were recorded right after the event, or in the evening, or reconstructed right before returning the diary to the survey centre). These are among the reasons why algorithmic methods are preferred in most studies. However, there are occasions where significant PA occurs, but the activity monitors cannot be worn for technical, convenience-related, or aesthetic reasons: for example, swimming, dance competitions, or different contact sports. In these cases, using activity diaries to impute missing data can be a viable strategy to avoid underestimating the true PA levels (de Meester et al. 2011).
In estimating the amount of time that needs to be observed, the calendar day is most often used as a unit. This is one of the natural units by which human activities are organised (in addition to weeks, months, and years), but from a data analytical point of view the period between 00:00 and 24:00 is no different from any other 24-h period. This means, in principle, that incomplete days could be used in analyses if the missing time could be imputed from another day. However, this would make it more difficult to analyse, for example, the effects of weekdays, so keeping day as a natural unit of analysis has some obvious advantages.
There is a lot of discussion in the literature about (a) the valid day issue: the number of hours of wearing time in a day that is needed in order to keep that day in the analyses and (b) the issue of minimum number of valid days required to consider the recording representative of a participant’s usual levels of PA.
There is no universal answer to these questions: it depends on the organisation of daily activities in the population under study. In a hypothetical population where there are consistent individual differences in activity levels but no within-individual differences between hours in a day or days in a year, 1 h of recording would be sufficient to characterise any individual’s level of activity. In real populations, however, these premises do not hold: individuals’ activity levels fluctuate, and days and hours differ widely from each other. For an empirically based answer on minimum recording time, one would thus need to observe a population for a considerable period (say, a few years) to get a truly representative estimate of all kinds of different fluctuations in PA that may occur and their regularity or irregularity (e.g. seasonal variation may be different depending on the weather in a particular year; so at least a few years of observation are needed for a true picture of seasonal variation). If this becomes too difficult for participants, one could sample random hours, days, or weeks from a year. Based on this data set, one could derive a sampling algorithm to minimise the necessary recording time to get a credible estimate of a person’s yearly activity. To our knowledge, such a study has not yet been carried out, but it is not unrealistic given today’s technical capabilities.
In (temporary) lack of long-term studies, shorter ones have been used to predict the reliability of data, presuming that the future resembles the past. For example, Trost et al. (2000) studied youth in different age groups and found, using Spearman–Brown prophecy formula, that to achieve a reliability (measured as intraclass correlation, ICC) of 0.90, one would need to observe 11 days in first to third-graders, but 20 days in tenth to twelfth graders. In contrast, Vanhelst et al. (2014b), using a slightly different method, found that any combination of a weekday and a weekend day was sufficient to explain >90% of the variance in PA in obese youth (aged 7–18 years). Ojiambo et al. (2011) found 1-day ICCs of 0.32, 0.33, and 0.35 of average counts per minute, sedentary time, and MVPA, respectively, which in combination with the Spearman–Brown formula predicted that about 5 days would be needed to achieve a reliability of 0.7, 7 to 9 days for an ICC of 0.8, and 17–19 days for an ICC of 0.9. Such studies can, however, only serve as a rough guide, for several reasons.
-
(1)
Intraclass correlations are based on random effects models and assume interchangeability of observations (Shrout and Fleiss 1979). However, consecutive days are not independent of each other, which means one would need a longer study to estimate the level of autocorrelation, and they are not randomly selected. Randomly selected 3 days from a year might give us an unbiased estimate of a person’s yearly activity, but in a typical study these would be three consecutive days, which is highly improbable to happen by chance (the probability is about 2.07 × 10−8).
-
(2)
Under specific conditions, ICCs may underestimate the true reliability (Hallgren 2012); the amount to which this happens in PA studies is unknown—to ask this question, one should first make sure that the assumption of interchangeability holds.
-
(3)
With the exception of the Vanhelst et al. (2014b) study, the difference between weekdays and weekends is often ignored, and a general recommendation regarding the number of days is given.
-
(4)
ICC, as typically applied in PA studies, considers only one source of reliable variance (“true” levels of PA that are different between individuals) whereas there are many (e.g. there is an interaction between time of the day and person: some people are more active in the morning and some in the afternoon). This situation could be more adequately modelled by generalisability theory (Brennan 2001) or similar methods.
-
(5)
Using the day as the unit of analysis seems natural but is not without problems. A single day of measurement seems likely to reflect, to a certain degree, the habitual activity of people, but its reliability cannot be computed using ICC without subdividing it, say, by hours. Dividing a day by hours, however, is not a full solution because (a) it is an arbitrary division which needs to be justified against alternatives such as half-hours or “academic hours” (45 min); (b) in this case, one would need to consider additional components of variance—time of day and person by time-of-day interaction, e.g. in the generalisability theory framework (Brennan 2001); and (c) more fine-grained divisions mean more units of observation from each person, possibly leading to higher estimates of reliability of the composite; however, reliability estimates should not depend on the arbitrary selection of unit of analysis.
-
(6)
Most severely, the ICC as ratio of the between-individual variance to the total variance is a group-based and sample-dependent measure of reliability. In other words, according to this model, the accuracy of a single measurement occasion depends on how different the other people are from the person being measured. A larger ICC could be achieved by increasing the between-individual variance of the sample, for example including a few more sedentary individuals and competing athletes. To find a small ICC, it suffices to find a highly homogenous sample. This is counter-intuitive: a seeming change in reliability of a measurement has occurred without anything having been changed in the measurement itself. It would thus be desirable to develop a way to assess the accuracy of PA measures that would not depend on individual differences.
Against this background, one cannot fully rely on the ICC-based estimates as a guide. This information should be complemented by common sense, former studies, and knowledge of the target population. In addition, the desired level of reliability is not fixed: higher reliability is needed for individual diagnosis and in smaller samples. In population-based studies, one has to simultaneously manage two risks: (a) poor representativeness of individual measurements due to a too short period of measurement and (b) poor representativeness of the sample due to low compliance in PA measurements. Because protocol adherence tends to decrease with days of wear (Trost 2007; Corder et al. 2008), a compromise between feasibility and accuracy is necessary.
Seasonality of PA is a question that may need more attention: for example, Rääsk et al. (2015b) found a correlation of 0.19 between minutes of MVPA and time of measurement (indexed as absolute difference in days between winter solstice and the first day of accelerometry). This is a small correlation, but it results in a considerable mean difference when measurements from different seasons are compared. There are other studies of seasonality of PA (e.g. Kolle et al. 2009), but a consensus has not yet found of how and to which extent the seasonality must be taken into account.
It is common knowledge that people’s PA differs by time of day, and there are usually differences between weekdays and weekends. From this, the minimum requirement for PA recording is one full weekday and one full weekend day. Recoding more weekdays and, if possible, a whole week, is desirable to capture the between-day differences that are likely to occur; longer periods are even better but may reduce compliance. Repeating the assessment at a different time of the year would probably do more for representativeness than prolonging an assessment by the same number of days.
In the IDEFICS study, the initial decision was to record at least 3 days of activity for each child, including one weekend day, whereas it strived for a recording time of 1 week in the I.Family study. This decision was based on the number of activity monitors available in each centre, the time available for fieldwork and the time needed to set up the devices including downloading the data, charging the batteries, and setting the device up for next recording. In some centres, more devices were available, and longer recording times were thus possible. For deriving reference values for the whole sample (Konstabel et al. 2014), it was decided to use the inclusion criterion of at least one weekend day and one weekday (with at least 8 h of wear time)—this way, 7684 children could be included (only 5047 when two weekdays were required instead of one—that is, the sample size would have been about 35% less).
Ideally, the recording period should cover a whole week or all different days of the week. In many studies, this is not possible to achieve, given common difficulties such as the number of available devices, the study period, or the desired sample size. A simple way to compactly present PA variables in these cases is to use a weighing scheme that is proportional to the number of weekdays and weekend days in a week: either a weighted sum (5 × WD + 2 × WE) or a weighted average (5 × WD + 2 × WE)/7, where WD is the average value in weekdays and WE is the average value in weekend days (Konstabel et al. 2014; Ortega et al. 2013). Note that the result applies to the typical week, not to the average week: for that purpose, one should consider holidays, school breaks, vacations, illness days, and the like; the corrected proportion of non-business days could be used, but one cannot assume that all non-business days are like typical weekend days—consequently, this additional correction could do more harm than good.
7.3.6 Aspects of Data Reduction
Ideally, participants in a study comply with the study protocol. In the IDEFICS study, this meant putting on the accelerometer the first thing in the morning and taking it off every night right before going to sleep; removing it only for taking shower and swimming (or other events which may do harm to the device); and recording all non-wear events in the activity diary. However, protocols are not completely observed and there will be differences in wear time for unknown reasons. Several ways to correct or adjust for these differences have been used, the simplest of them being controlling for wear time (either for number of valid days or average daily wear time; Ortega et al. 2013) or expressing time in activity intensity categories as percentage of wear time (Rääsk et al. 2015a). We have also used a hybrid method: to find adjusted MVPA, the daily MVPA was first expressed as a fraction of wear time and then multiplied by average wear time in minutes (Konstabel et al. 2014). The rationale for this was that the outcome in minutes is easier to communicate and understand than a fraction, especially if that fraction is, unfortunately, close to zero. Additionally, wear times were different across sexes and age groups, thus adjusting provided a simple way of factoring wear time out of the group comparisons. This form of “adjusted minutes” (of, say, MVPA) would, however, presume that the activity at non-wear times is, on the average, as intensive as the activity at the time when the device was worn. There are two complementary ways to minimise the impact of such assumptions: (a) trying to achieve good compliance and (b) imputing the periods of missing data either using closest-in-time activity form the same day or another day from the same individual or using activity diaries. In some special cases, one could consider using group averages from the same period in imputing. Conceptually, this might make sense in groups sharing a common daily schedule of activities, e.g. the military (Horner et al. 2013) or, possibly, students at school time.
Minutes in activity categories are useful summary statistics for ease of communication and understandability. They have, however, a disadvantage of ignoring the within-category differences: activities at 1.51 MET and 2.99 MET are, for example, treated equally as light, even though there is an almost twofold difference in intensity. For some purposes (e.g. public health recommendations—and, consequently, studies validating such recommendations), ignoring the within-category variance is probably inevitable, or if one believes that the error becomes too large, the solution might be to create an additional category. However, there are questions (e.g. the relative contribution of activities at different intensity levels to the energy balance) where ignoring the within-category differences is simply not possible. For these purposes, one could decompose the total daily counts into intensity categories. Using formulae from the literature (Alhassan et al. 2012), one can transform these decomposed counts into EE units (METs or kcal or kcal/kg) or EE-per-time units (e.g. MET∙minutes). For example, Rääsk et al. (2015a) have found longitudinal decreases in both light activity and MVPA in a study of adolescent boys. In addition, Konstabel et al. (2017) showed that in terms of EE, the decreases in light activity mattered more.
7.4 Software for Data Analysis
At the time when data collection in the IDEFICS study was finished, the standard way to analyse GT1M data was a combination of manual pre-processing in spreadsheet software (e.g. deleting manually the long sequences of counts where the participant either appears not to be wearing the device or has written in the activity diary that the device was not being worn), then using the macros or spreadsheet functions to compute summary statistics and then copying these summary statistics into another table for final analysis. In small samples, this does not result in too extensive workload and may even lead to important insights into the data, as everything is checked by the human eye. For a large epidemiological study, this method is obviously unpractical and could lead to human errors. There were also a number of software programs available, such as MAHUffe, MeterPlus, and Kinesoft, which allowed some laborious steps of pre-processing to be automatised. We needed, however, a fully customisable system which would be well integrated with a good statistical platform. Therefore, we decided to write a program using the software R (Ihaka and Gentleman 1996; R Core Team 2017). To date, this has evolved into an R add-on package “accelerate” (Konstabel 2018; for access see also Sect. 7.7) which provides a comprehensive set of functions to process the ActiGraph (7164, GT1M, and GT3X count data) and 3DNX data. The previous versions of the package have been used in analysing data from several projects (e.g. Rääsk et al. 2017; Garaulet et al. 2017; Ortega et al. 2013).
7.4.1 Design Choices
R was chosen as a platform because of its widespread use, integration of a statistical environment with programmability, availability of a large number of statistical and data analysis methods as add-on packages, integration with literate programming tools, and extensibility. The main goals of the package are comprehensiveness and ease of use which means inclusion of all commonly used methods and tasks in a simple workflow. The package was designed to be modular and easily extensible. Each subtask is programmed as a separate function; the user can change each part separately without the need of changing the whole program (e.g. new functions can be added for new data file formats or new analytical methods). For extensibility and robustness, the number of dependencies was kept at minimum. Finally, as accelerometer data files can be quite large, sparing use of memory had to be kept in mind. For example, data files are processed sequentially rather than loading them all into the memory and, instead of storing a timestamp with each data point, the timestamp is reconstructed when necessary, given the start time and time interval between the observations (“epoch”).
7.4.2 Reading in and Pre-processing the Data
The first layer in the accelerate package is functions to read in the data files in various formats. There are three separate functions to read in plain text file formats from ActiGraph: read.actigraph.dat (for “dat” files), read.actigraph.csv (for “csv” files), and read.csa (for “dat” files from older models such as CSA 7164). These plain text formats store date and time information in local formats (e.g. 02/06/2018, 06.02.2018, and 2018-02-06 all refer to the same date, 6 February 2018); the functions thus contain algorithms to automatically try to interpret such information in a meaningful way (e.g. taking into account that downloading the data is a later event than initialising the device and that there are no more than 12 months in a year). Alternatively, one can set the date format to be used in an extra argument named dateformat . The newer “agd” file format used by ActiGraph, as well as the 3DNX device (read in respectively by read.agd and read.3dnx ), stores dates in unambiguous format, so reading in is more straightforward. Finally, the read.actigraph function accepts any of the ActiGraph file formats (except for the older CSA format) and chooses the appropriate function to use based on file extension (e.g. “agd” files are redirected to read.agd ).
All the reading-in functions accept a get.id argument which refers to a function to derive an identification number (ID) of the participant based on the file name or any information within the file. Two such functions are provided, getID which uses all digits in the file name as an ID and getNAME which deletes the file extension and any digits and uses the rest as an ID. An appropriate function should be chosen or written based on the conventions of the study.
The data are stored as a list of two components, X, a data frame containing the data, and HD , a vector containing all other relevant information such as start date/time of recording, the epoch, serial number of the device, ID code of the participant, file name.
By default, the reading-in functions do some pre-processing, such as filtering out the faulty data (e.g. negative counts) and detecting the wear and non-wear times (the latter are replaced by missing data codes). This behaviour is controlled by two arguments, .filter, and preprocess ; to suppress all pre-processing, one can set both of these to identity.
Two algorithms of wear time detection are available: the delete.zeros function implementing the “consecutive zeros” algorithm (detecting the periods with at least N minutes of consecutive zeros, where N is, by default, 20) and the wmChoi function implementing the algorithm proposed by Choi and colleagues (2011a) adapted with slight changes from the PhysicalActivity package (Choi et al. 2011b).
For sequential processing of many files, the package includes a function process.folder that can be adapted to various tasks that involve applying similar rules to every file. This mechanism is used in read.actigraph.folder which reads in, pre-processes, and summarises all ActiGraph files in a folder (reintegrating every file to a common epoch to avoid treating files with different epochs in the same way), producing a data frame where each day is represented by a row and participants are identified by different values in the ID column. The mechanism can be easily adapted to different sets of rules and different tasks, such as producing a feedback sheet to every participant and possibly sending it via e-mail. The function takes care of errors when reading in or summarising the files: instead of quitting and giving an error message, the messages are recorded and saved for later inspection. By default, process.folder does timing in all cases, so it is possible to estimate how much time each operation might take, even retrospectively.
For example, to read in and summarise all over 2000 Hungarian files from the IDEFICS baseline survey (T0), the following code was used:
> HT0 <- read.actigraph.folder( ′′ Hungary_T0 ′′ )
This can take some time, so for estimating when the processing will be complete one might try the argument LIST to read in just a few files first:
> HT0 <- read.actigraph.folder( ′′ Hungary_T0 ′′ , LIST = 1:5)
> attr(HT0, ′′ timing ′′ )
7.4.3 Summarising the Data
Suppose we have read in a file using …
> x <- read.actigraph( ′′ sampledata/1036.dat ′′ )
We can now summarise the data by some time unit. Usually, this would be days, but in some cases shorter periods such as hours or half-hours can be useful. The code uses R’s built-in function cut.POSIXt to divide the time sequence, so specifications like “30 min” or “hours” or “2 days” are all valid. Thus, the first line in the following code segment summarises x by days and the other one by 30 min segments:
> summary(x, ′′ DSTday ′′ ) # the default
> summary(x, ′′ 30 min ′′ ) # each row summarises a 30 min period
One could use just “days” in the first line, but “ DSTday ” takes care of the daylight saving time; due to this, in many countries, one day in a year will only have 23 h, while another has as many as 25.
The summary outputs a data frame with some information about each time period in the file. First, the file name, subject’s ID, epoch, model, and serial number of the device are included; these data are, obviously, identical for all rows based on the same file. The next three variables can be different for each row in the summary: Period (the start of the time period, as a date/time variable with class ), Length (nominal length of the period, including non-wear time), and POSIXct Wkdy (day of the week, coded as integers from 0 to 6, starting from Sunday). The following variables depend on what has been recorded in the data file and the functions one chooses using the argument STATS to summary . The CNTSTATS function outputs some of the most common statistics used for counts: valid time ( val.time ), total counts ( tot.counts ), average counts per minute ( avg.cpm ), and time in intensity categories. One or more sets of cut-points can be specified using the cutoffs argument; both minutes in intensity category and the corresponding proportion of valid time are computed. The CNTSTATS2 function uses the cut-points in a slightly different way. In addition to minutes in each intensity category, it outputs the sum of counts in each intensity category, which can be converted to quasi EE units (Konstabel et al. 2017), as discussed in Sect. 7.3.6. If the file contains information on steps or heart rate, this can be analysed using STEPSTATS and HRSTATS . Finally, HRSTATS2 combines count and heart rate data to find median heart rate in each category of activity intensity (an analysis by Ojiambo et al. 2012).
As an example, the following line will compute the count and step statistics by each day in category x, using Evenson cut-points; the results will be expressed in a compact way; i.e. errors in the data file (e.g. negative counts) are not included in the table, and time in intensity categories is expressed only as minutes (instead of minutes and a fraction of valid time):
> summary(x, STATS = c(CNTSTATS, STEPSTATS), cutoffs = Cutoffs.Evenson, short = TRUE)
The data can be plotted using the generic plot function. The plot.acc method will plot counts as lines, using time as the x-axis. Figure 7.1 is produced by the following code:
> plot(x, cutoffs = Cutoffs.Evenson) # Figure 7.1
Another way to visualise the daily PA profile is to partition the time periods into activity categories in the order of increasing intensity (see Fig. 7.2). This can be done with aciPlot:
Activity profile in a single day (“Day 4” in Fig. 7.1) by 30-min segments
> aciPlot(x, select = Wkdy %in% 5) # Figure 7.2
Finally, the function summarize is intended to summarise the data at the next level with one row per participant. This can be applied to a summary of a single file [such as the output summary(x)] or of a number of files (such as the output of read.actigraph.folder ). The two main arguments to summarize are: condition (an expression using variables in the output of summary, such as val.time > 8*60) and no.days (number of valid time periods—typically days—that a participant must have in order to be included). Another version, summarize2 , treats weekdays and weekend days differently: the reqdays argument can be set as a named vector, such as c(wd = 5, we = 2) to require at least five valid weekdays and two weekend days for a participant’s data to be included.
7.5 Future Perspectives in Accelerometry
Accelerometry is among the best ways to measure PA, but it has inherent limitations: for example, static efforts and weight carrying seem unlikely to be captured, especially with a single sensor. In addition, the relationship between movement and EE may be different in different types of activity (e.g. biking as compared to running). There seem to be two ways forward for more accurate prediction of EE. First, while portable \( {\dot{\text{V}}}{\text{O}}_{2} \) systems do already exist, they are not convenient for carrying along during several days of ordinary life. The advancement of technology may provide even better solutions that, if not affordable for large-scale testing, could provide a breakthrough in calibrating and validating the accelerometry-based monitors. Secondly, activity recognition algorithms could be developed based on either the advancements of Crouter and Bassett’s (2008) two-regression model, multiple accelerometer sensors or a combination of different sensors. While the “black box” modelling strategy (e.g. using artificial neural networks) is guaranteed to give a result, joining the efforts of biomechanics and metabolic physiology is more likely to advance our substantive understanding of the nature of PA energy expenditure at different activities.
As many contemporary devices allow downloading the raw data, and software for analysing such data is becoming easily accessible (van Hees 2018), using raw data should be considered especially in the cases where counts-based methods of analysis are known to be suboptimal as, e.g. in wear time detection as well as at the low and high end of the intensity spectrum. Combining different sensors (e.g. temperature or light sensors) could be an alternative way of wear time detection.
There is a large number of sensors that can be used in combination with accelerometry and probably an even larger field of unexplored or underexplored possibilities. For example, altimeters (pressure sensors) could be used to classify inclined locomotion (e.g. walking upstairs) to enhance the accuracy in EE estimation (Yang and Hsu 2010).
For understanding and intervening on PA, it has to be treated as a meaningful human activity rather than just physical movement. For that purpose, linking the accelerometer data with information on mood and behaviour (e.g. diaries, experience sampling, or electronically activated recording), physiological reactions, and context [e.g. geographical location from geographic information systems (GIS)] is crucial. GIS, in particular, offers a large array of possibilities to relate PA to what is changeable in the environment (Buck et al. 2015a, b).
7.6 Assessing Physical Activity in the Field
Besides technology and algorithms, the data quality also depends on human factors and fieldwork logistics. The first, crucial, task is to make sure that the person who wore a device is identifiable. In the IDEFICS study, this was done in slightly different ways in different survey centres, depending on the local conditions. In one participating country, for example, it was necessary to label the devices with child’s name. This was especially important in the younger, kindergarten-aged group, who, in the workdays, had organised nap time from approximately 1 pm to 3 pm. Typically, the teacher would collect the devices before the nap and distribute them again after it; this would not be possible without the labels. In school-aged children, in contrast, the labelling was more of a precaution, as the devices were typically not removed during the day. Nevertheless, the labels were occasionally lost, and the devices were uncoupled from the participants for other reasons. For example, it happened that several siblings from the same family were wearing the activity monitors at the same time, or classmates inadvertently (or perhaps advertently) swapped their devices. In such cases, if the swapping happened on the first day or right before returning the devices, the data could be used, in case the bearers of the devices were identified by parents’ or teachers’ reports, comparing activity diaries with the recorded data or other information. In a few cases, the device was returned but was not worn or contained mostly missing data because the child either could not or forgot to wear it or was ill at the time. If logistically possible, the child was then offered a new opportunity, and the latter file was used in analyses.
There are some reports on reactivity on wearing accelerometers (Dössegger et al. 2014), that is, higher than usual activity on the first day of measurement, which thereafter returns to the presumably usual level. The IDEFICS study was not planned for assessing reactivity. For example, the first day of wearing was not chosen at random but determined based on logistics and device availability; however, indirect assessment of reactivity might be possible. We did try to minimise reactivity by emphasising in the instructions that the participants should continue with their usual activities. The problem is, of course, that the reactivity might not always be intentional or even conscious: by being given an activity monitor, participants are inevitably reminded about physical activity, and this may be enough to prompt an increase in activity. In addition, reactivity due to the personnel at schools or kindergartens may have a longer lasting effect and be even more difficult to control.
The motivation and compliance of the participants are an important factor in data quality. Feedback on PA has potential to increase motivation; quick and fully automatic ways of creating feedback were not available to us at the baseline survey of the IDEFICS study, but by the end of the study period we had developed a rudimentary script, which is included in a simplified form in the accelerate package ( do.feedback) . However, providing feedback (as well as other ways of boosting interest in PA assessment) could increase reactivity. One might also want to consider the potentially adverse effect of negative feedback. Thus, the “too little PA” message should be framed in a positive and encouraging way.
There are logistic issues that may do harm to motivation even if the participant is otherwise interested in PA assessment. One of these is the fear of losing or damaging the device. There is a trade-off involved in eliminating this fear completely because for obvious reasons we do not want the participants to lose or damage the devices. Another issue is that of aesthetics and hygiene. First, the devices tend to look less and less new with every user, up to a point when one must do something about it; second, in study logistics, one must plan sufficient time for washing and drying the belts sufficiently often.
When downloading the data, it is useful to regularly check the files, for example, making a plot similar to Fig. 7.1. If the data do not look like human activity, one should investigate the reason. The malfunctioning hardware may need to be repaired or removed from use; temporary problems at download time are unlikely but can be repaired by downloading the data anew (which is impossible if the device is already set up to record new data); incompatibilities with new versions of firmware may be resolved by using other (newer or older) versions of download software; finally, during the IDEFICS study, several data files with firmware-related problems were successfully repaired in collaboration with the device manufacturer. It is useful to become aware of these problems as early as possible; thus, having a look at the files right after downloading is probably a good idea.
7.7 Provision of Instruments and Standard Operating Procedures to Third Parties
All standard operating procedures (SOPs) described in this chapter are provided by the General Survey Manuals that can be accessed on the following website: www.leibniz-bips.de/ifhs after registration. The R package “accelerate” can also be downloaded from this website.
Each third partner using the SOPs provided in this chapter is kindly requested to cite this chapter as follows:
Konstabel K, Chopra S, Ojiambo R, Muñiz-Pardos B, Pitsiladis Y, on behalf of the IDEFICS and I.Family consortia. Accelerometry-based physical activity assessment for children and adolescents. In: Bammann K, Lissner L, Pigeot I, Ahrens W, editors. Instruments for health surveys in children and adolescents. Cham: Springer Nature Switzerland; 2019. pp. 135–173.
References
Ahrens W, Bammann K, Siani A, Buchecker K, De Henauw S, Iacoviello L, et al. IDEFICS consortium. The IDEFICS cohort: design, characteristics and participation in the baseline survey. Int J Obes (Lond). 2011;35(Suppl 1):S3–15.
Ahrens W, Siani A, Adan R, De Henauw S, Eiben G, Gwozdz W, et al. I. Family consortium. Cohort profile: the transition from childhood to adolescence in European children—how I.Family extends the IDEFICS cohort. Int J Epidemiol. 2017;46(5):1394–5j.
Alhassan S, Lyden K, Howe C, Kozey Keadle S, Nwaokelemeh O, Freedson PS. Accuracy of accelerometer regression models in predicting energy expenditure and METs in children and youth. Pediatr Exerc Sci. 2012;24(4):519–36.
Bailey DP, Locke CD. Breaking up prolonged sitting with light-intensity walking improves postprandial glycemia, but breaking up sitting with standing does not. J Sci Med Sport. 2015;18:294–8.
Bammann K, Sioen I, Huybrechts I, Casajús J, Vicente-Rodríguez G, Cuthill R, et al. IDEFICS consortium. The IDEFICS validation study on field methods for assessing physical activity and body composition in children: design and data collection. Int J Obes (Lond). 2011;35(Suppl 1):S79–87.
Bammann K, Peplies J, Sjöström M, Lissner L, De Henauw S, Galli C, et al. IDEFICS consortium. Assessment of diet, physical activity and biological, social and environmental factors in a multi-centre European project on diet- and lifestyle-related disorders in children (IDEFICS). J Pub Health. 2006;14(5):279–89.
Barlow SE, Expert Committee. Expert committee recommendations regarding the prevention, assessment, and treatment of child and adolescent overweight and obesity: summary report. Pediatrics. 2007;120(Suppl 4):S164–92.
Barnett LM, van Beurden E, Morgan PJ, Brooks LO, Beard JR. Childhood motor skill proficiency as a predictor of adolescent physical activity. J Adoles Health. 2009;44(3):252–9.
Bouten CV, Koekkoek KT, Verduin M, Kodde R, Janssen JD. A triaxial accelerometer and portable data processing unit for the assessment of daily physical activity. IEEE Trans Biomed Eng. 1997;44(3):136–47.
Brazendale K, Beets MW, Bornstein DB, Moore JB, Pate RR, Weaver RG, et al. Equating accelerometer estimates among youth: the Rosetta Stone 2. J Sci Med Sport. 2016;19(3):242–9.
Brennan RL. Generalizability theory. New York: Springer; 2001.
Buck C, Kneib T, Tkaczick T, Konstabel K, Pigeot I. Assessing opportunities for physical activity in the built environment of children: interrelation between kernel density and neighborhood scale. Int J Health Geogr. 2015a;22:14–35.
Buck C, Tkaczick T, Pitsiladis Y, De Bourdehaudhuij I, Reisch L, Ahrens W, et al. Objective measures of the built environment and physical activity in children: from walkability to moveability. J Urban Health. 2015b;92(1):24–38.
Bull FC, Expert working groups. Physical activity guidelines in the U.K.: review and recommendations. School of Sport, Exercise and Health Sciences, Loughborough University. 2010. https://www.gov.uk/government/uploads/system/uploads/attachment_data/file/213743/dh_128255.pdf. Accessed 8 Feb 2018.
Butte NF, Wong WW, Lee JS, Adolph AL, Puyau MR, Zakeri IF. Prediction of energy expenditure and physical activity in preschoolers. Med Sci Sports Exerc. 2014;46(6):1216–26.
Byrd-Williams CE, Belcher BR, Spruijt-Metz D, Davis JN, Ventura EE, Kelly L, et al. Increased physical activity and reduced adiposity in overweight Hispanic adolescents. Med Sci Sports Exerc. 2010;42:478–84.
Carter J, Wilkinson D, Blacker S, Rayson M, Bilzon J, Izard R, et al. An investigation of a novel three-dimensional activity monitor to predict free-living energy expenditure. J Sports Sci. 2008;26(6):553–61.
Caspersen CJ, Powell KE, Chistenson GM. Physical activity, exercise, and physical fitness: definitions and distinctions for health-related research. Public Health Rep. 1985;100:126–31.
Chen KY, Janz KF, Zhu W, Brychta RJ. Redefining the roles of sensors in objective physical activity monitoring. Med Sci Sports Exerc. 2012;44:S13–23.
Choi L, Liu Z, Matthews CE, Buchowski MS. Validation of accelerometer wear and nonwear time classification algorithm. Med Sci Sports Exerc. 2011a;43(2):357–64.
Choi L, Liu Z, Matthews CE, Buchowski MS. Physical activity: process physical activity accelerometer data. R package version 0.1–1. 2011b. https://CRAN.R-project.org/package=PhysicalActivity. Accessed 3 May 2018.
Choi L, Ward SC, Schnelle JF, Buchowski MS. Assessment of wear/nonwear time classification algorithms for triaxial accelerometer. Med Sci Sports Exerc. 2012;44(10):2009–16.
Chomistek AK, Yuan C, Matthews CE, Troiano RP, Bowles HR, Rood J, et al. Physical activity assessment with the ActiGraph GT3X and doubly labeled water. Med Sci Sports Exerc. 2017;49(9):1935–44.
Corder K, Ekelund U, Steele RM, Wareham NJ, Søren Brage S. Assessment of physical activity in adolescents. J Appl Physiol. 2008;105:977–87.
Crouter SE, Bassett DR Jr. A new 2-regression model for the Actical accelerometer. Br J Sports Med. 2008;42(3):217–24.
Crouter SE, Horton M, Bassett DR. Use of a 2-regression model for estimating energy expenditure in children. Med Sci Sports Exerc. 2012;44(6):1177–85.
de Almeida Mendes M, da Silva ICM, Ramires VV, Reichert FF, Martins RC, Tomasi E. Calibration of raw accelerometer data to measure physical activity: a systematic review. Gait Posture. 2018;61:98–110.
de Meester F, De Bourdeaudhuij I, Deforche B, Ottevaere C, Cardon G. Measuring physical activity using accelerometry in 13–15-year-old adolescents: the importance of including non-wear activities. Public Health Nutr. 2011;14(12):2124–33.
de Vet E, Verkooijen KT. Self-control and physical activity. Disentangling the pathways to health. In: de Ridder D, Adriaanse M, Fujita K, editors. The Routledge international handbook of self-control in health and well-being. London: Routledge; 2018. p. 276–87.
Dencker M, Andersen LB. Health-related aspects of objectively measured daily PA in children. J Sports Med. 2008;28:133–44.
Dieu O, Mikulovic J, Fardy PS, Bui-Xuan G, Béghin L, Vanhelst J. Physical activity using wrist-worn accelerometers: comparison of dominant and non-dominant wrist. Clin Physiol Funct Imaging. 2017;37(5):525–9.
Dössegger A, Ruch N, Jimmy G, Braun-Fahrländer C, Mäder U, Hänggi J, et al. Reactivity to accelerometer measurement of children and adolescents. Med Sci Sports Exerc. 2014;46(6):1140–6.
Edwardson CL, Gorely T. Epoch length and its effect on physical activity intensity. Med Sci Sports Exerc. 2010;42(5):928–34.
Ekblom O, Oddsson K, Ekblom B. Prevalence and regional differences in overweight in 2001 and trends in BMI distribution in Swedish children from 1987 to 2001. Scan J Public Health. 2004;32:257–63.
Esliger DW, Tremblay MS. Physical activity and inactivity profiling: the next generation. Appl Physiol Nutr Metab. 2007;32:195–207.
Evenson KR, Cattellier D, Gill K, Ondrak K, McMurray RG. Calibration of two objective measures of physical activity for children. J Sports Sci. 2008;26:1557–65.
Freedson P, Pober D, Janz KF. Calibration of accelerometer output for children. Med Sci Sports Exerc. 2005;37:523–30.
Fudge BW, Wilson J, Easton C, Irwin L, Clark J, Haddow O, et al. Estimation of oxygen uptake during fast running using accelerometry and heart rate. Med Sci Sports Exerc. 2007;39:192–8.
Gabriel KP, McClain JJ, Schmid KK, Storti KL, High RR, Underwood DA, et al. Issues in accelerometer methodology: the role of epoch length on estimates of physical activity and relationships with health outcomes in overweight, post-menopausal women. Int J Beh Nutr Phy Activ. 2010;7:53.
Garaulet M, Martinez-Nicolas A, Ruiz JR, Konstabel K, Labayen I, González-Gross M, et al. HELENA study group. Fragmentation of daily rhythms associates with obesity and cardiorespiratory fitness in adolescents: the HELENA study. Clin Nutr. 2017;36(6):1558–66.
Gorber SC, Tremblay MS. Self-report and direct measures of health: bias and implications. In: Shephard RJ, Tudor-Locke C, editors. The objective monitoring of physical activity: contributions of accelerometry to epidemiology, exercise science and rehabilitation. New York: Springer; 2016. p. 369–76.
Guinhouya BC, Hubert H, Zitouni D. Need for unbiased computation of the moderate-intensity physical activity of youth in epidemiologic studies. Am J Prev Med. 2011;41(1):e1–2.
Hallgren KA. Computing inter-rater reliability for observational data: an overview and tutorial. Tutor Quant Methods Psychol. 2012;8(1):23–34.
Healy GN, Dunstan DW, Salmon J, Cerin E, Shaw JE, Zimmet PZ, et al. Breaks in sedentary time—beneficial associations with metabolic risk. Diabetes Care. 2008;31:661–6.
Hislop JF, Bulley C, Mercer TH, Reilly JJ. Comparison of accelerometry cut points for physical activity and sedentary behavior in preschool children: a validation study. Pediatr Exerc Sci. 2012;24(4):563–76.
Horner F, Bilzon JL, Rayson M, Blacker S, Richmond V, Carter J, et al. Development of an accelerometer-based multivariate model to predict free-living energy expenditure in a large military cohort. J Sports Sci. 2013;31(4):354–60.
Horner FE, Rayson MP, Bilzon JLJ. Reliability and validity of the 3DNX accelerometer during mechanical and human treadmill exercise testing. Int J Obes (Lond). 2011;35(Suppl 1):S88–97.
Howe CA, Staudenmayer JW, Freedson PS. Accelerometer prediction of energy expenditure: vector magnitude versus vertical axis. Med Sci Sports Exerc. 2009;41(12):2199–206.
Ihaka R, Gentleman R. R: A language for data analysis and graphics. J Comput Graph Stat. 1996;5:299–314.
Jakicic JM, Otto DA. Physical activity considerations for the treatment and prevention of obesity. Am J Clin Nutr. 2005;82(Suppl 1):226S–9S.
Janssen X, Basterfield L, Parkinson KN, Pearce MS, Reilly JK, Adamson AJ, et al. Gateshead millennium study core team. Objective measurement of sedentary behavior: impact of non-wear time rules on changes in sedentary time. BMC Public Health. 2015;23:504.
John D, Freedson P. Actigraph and actical physical activity monitors: a peek under the hood. Med Sci Sports Exerc. 2012;44:S86–9.
John D, Tyo B, Bassett DR. Comparison of four ActiGraph accelerometers during walking and running. Med Sci Sports Exerc. 2010;42(2):368–74.
Kitamura K, Nemoto T, Sato N, Chen W. Development of a new accelerometer-based physical activity-monitoring system using a high-frequency sampling rate. Biol Sci Space. 2009;23:77–83.
Kolle E, Steene-Johannessen J, Andersen LB, Anderssen SA. Seasonal variation in objectively assessed physical activity among children and adolescents in Norway: a cross-sectional study. Int J Behav Nutr Phys Act. 2009;6:36.
Konstabel K, Mäestu J, Rääsk T, Lätt E, Jürimäe J. Decline in light-intensity activity is a major component of the longitudinal decline in physical activity in adolescent boys. Acta Paediatr. 2017;106(Suppl 470):24.
Konstabel K, Veidebaum T, Verbestel V, Moreno LA, Bammann K, Tornaritis M, et al. IDEFICS consortium. Objectively measured physical activity in European children: the IDEFICS study. Int J Obes (Lond). 2014;38(Suppl 2):S135–43.
Konstabel K. accelerate: an R package for accelerometry data analysis version 1.0.1. 2018. https://osf.io/s42a3/.
Kyröläinen H, Belli A, Komi PV. Biomechanical factors affecting running economy. Med Sci Sports Exerc. 2001;33:1330–7.
Lee IM, Skerrett PJ. Physical activity and all-cause mortality: what is the dose-response relation? Med Sci Sports Exerc. 2001;33:459–71.
Levine JA. Non-exercise activity thermogenesis (NEAT). Nutr Rev. 2004;62:S82–97.
Lin SY, Lai YC, Hsia CC, Su PF, Chang CH. Validation of energy expenditure prediction modelling using real-time shoe-based motion detectors. IEEE Trans Biomed Eng. 2016;64:2152–62.
Lubans DR, Morgan PJ, Cliff DP, Barnett LM, Okely AD. Fundamental movement skills in children and adolescents. Review of associated health benefits. Sports Med. 2010;40(12):1019–35.
Manohar C, McCrady S, Pavlidis IT, Levine JA. An accelerometer-based earpiece to monitor and quantify physical activity. J Phys Act Health. 2009;6(6):781–9.
Manohar CU, McCrady SK, Fujiki Y, Pavlidis IT, Levine JA. Evaluation of the accuracy of a triaxial accelerometer embedded into a cell phone platform for measuring physical activity. J Obes Weight Loss Ther. 2011;1(106):3309.
Mark AE, Janssen I. Influence of bouts of physical activity on overweight in youth. Am J Prev Med. 2009;36(5):416–21.
Martin JB, Krč KM, Mitchell EA, Eng JJ, Noble JW. Pedometer accuracy in slow walking older adults. Int J Ther Rehabil. 2012;19(7):387–93.
Martinez-Gomez D, Ruiz JR, Ortega FB. Author response. Am J Prev Med. 2011;41(1):e2–3.
Mattocks C, Leary S, Ness A, Deere K, Saunders J, Tilling K, et al. Calibration of an accelerometer during free-living activities in children. Int J Pediatr Obes. 2007;2:218–26.
McClain JJ, Abraham TL, Brusseau TA Jr, Tudor-Locke C. Epoch length and accelerometer outputs in children: comparison to direct observation. Med Sci Sports Exerc. 2008;40(12):2080–7.
Migueles JH, Cadenas-Sanchez C, Ekelund U, Delisle Nyström C, Mora-Gonzalez J, Löf M, et al. Accelerometer data collection and processing criteria to assess physical activity and other outcomes: a systematic review and practical considerations. Sports Med. 2017;47(9):1821–45.
Miles L. Physical activity and health. Nutr Bull. 2007;32:314–63.
Miller J, Rosenbloom A, Silverstein J. Childhood obesity. J Clin Endocrinol Metab. 2004;89:4211–8.
Montoye AHK, Dong B, Biswas S, Pfeiffer KA. Validation of a wireless accelerometer network for energy expenditure measurement. J Sports Sci. 2016;34(21):2130–9.
Mossberg H. 40-year follow-up of overweight children. Lancet. 1989;26:491–3.
Ojiambo R, Cuthill R, Budd H, Konstabel K, Casajus JA, Gonzalez-Agüero A, et al. IDEFICS consortium. Impact of methodological decisions on accelerometer outcome variables in young children. Int J Obes (Lond). 2011;35(Suppl 1):S98–103.
Ojiambo R, Gibson AR, Konstabel K, Lieberman DE, Speakman JR, Reilly JJ, et al. Free-living physical activity and energy expenditure of rural children and adolescents in the Nandi region of Kenya. Ann Hum Biol. 2013;40(4):318–23.
Ojiambo RM, Konstabel K, Veidebaum T, Reilly JJ, Verbestel V, Casajús JA, et al. IDEFICS consortium. Validity of hip-mounted uniaxial accelerometry with heart-rate monitoring versus triaxial accelerometry in the assessment of free-living energy expenditure in young children: the IDEFICS validation study. J Appl Physiol. 2012;113(10):1530–6.
Ortega FB, Cadenas-Sánchez C, Sánchez-Delgado G, Mora-González J, Martínez-Téllez B, Artero EG, et al. Systematic review and proposal of a field-based physical fitness-test battery in preschool children: the PREFIT battery. Sports Med. 2015;45(4):533–55.
Ortega FB, Konstabel K, Pasquali E, Ruiz JR, Hurtig-Wennlöf A, Mäestu J, et al. Objectively measured physical activity and sedentary time during childhood, adolescence and young adulthood: a cohort study. PLoS ONE. 2013;8(4):e60871.
Ortega FB, Ruiz JR, Sjöström M. Physical activity, overweight and central adiposity in Swedish children and adolescents: the European Adolescents Heart Study. Int J Behav Nutr Phys Act. 2007;4:61.
Ottevaere C, Huybrechts I, De Meester F, De Bourdeaudhuij I, Cuenca-Garcia M, De Henauw S. The use of accelerometry in adolescents and its implementation with non-wear time activity diaries in free-living conditions. J Sports Sci. 2011;29(1):103–13.
Pate RR, Almeida MJ, McIver KL, Pfeiffer KA, Dowda M. Validation and calibration of an accelerometer in preschool children. Obes (Silver Spring). 2006;14(11):2000–6.
Phillips LRS, Parfitt G, Rowlands AV. Calibration of the GENEA accelerometer for assessment of physical activity intensity in children. J Sci Med Sport. 2013;16:124–8.
Pitsi T, Zilmer M, Vaask S, Ehala-Aleksejev K, Kuu S, Löhmus K, et al. Eesti toitumis- ja liikumissoovitused 2015 (Estonian guidelines on nutrition and physical activity). Tallinn: Tervise Arengu Instituut. 2017. https://intra.tai.ee//images/prints/documents/149019033869_eesti%20toitumis-%20ja%20liikumissoovitused.pdf. Assessed 2 Feb 2018.
Plasqui G, Bonomi AG, Westerterp KR. Daily physical activity assessment with accelerometers: new insights and validation studies. Obes Rev. 2013;14(6):451–62.
Powell KE, Paluch AE, Blair SN. Physical activity for health: What kind? How much? How intense? On top of what? Annu Rev Public Health. 2011;32:349–65.
Puyau MR, Adolph AL, Vohra FA, Butte NF. Validation and calibration of physical activity monitors in children. Obes Res. 2002;10(3):150–7.
R Core Team. R. A language and environment for statistical computing. Vienna, Austria: R Foundation for Statistical Computing. 2017. https://www.R-project.org/. Accessed 3 May 2018.
Rääsk T, Konstabel K, Mäestu J, Lätt E, Jürimäe T, Jürimäe J. Tracking of physical activity in pubertal boys with different BMI over two-year period. J Sports Sci. 2015a;33:1649–57.
Rääsk T, Lätt E, Jürimäe T, Mäestu J, Jürimäe J, Konstabel K. Association of subjective ratings to objectively assessed physical activity in pubertal boys with differing BMI. Percept Mot Skills. 2015b;121(1):245–59.
Rääsk T, Mäestu J, Lätt E, Jürimäe J, Jürimäe T, Vainik U, et al. Comparison of IPAQ-SF and two other physical activity questionnaires with accelerometer in adolescent boys. PLoS ONE. 2017;12(1):e0169527.
Reilly JJ, Methven E, McDowell Z. Health consequences of obesity. Arch Dis Child. 2003;88:748–52.
Rich C, Geraci M, Griffiths L, Sera F, Dezateux C, Cortina-Borja M. Quality control methods in accelerometer data processing. PLoS ONE. 2013;8(6):e67206.
Rosenberger ME, Haskell WL, Albinali F, Mota S, Nawyn J, Intille S. Estimating activity and sedentary behavior from an accelerometer on the hip or wrist. Med Sci Sports Exerc. 2013;45(5):964–75.
Sallis JF, Patrick K. Physical activity guidelines for adolescents: consensus statement. Pediatric Exerc Sci. 1994;6:302–14.
Santos-Lozano A, Marín PJ, Torres-Luque G, Ruiz JR, Lucía A, Garatachea N. Technical variability of the GT3X accelerometer. Med Eng Phys. 2012;34(6):787–90.
Sardinha LB, Júdice PB. Usefulness of motion sensors to estimate energy expenditure in children and adults: a narrative review of studies using DLW. Eur J Clin Nutr. 2017;71(8):1026.
Shiroma EJ, Schepps MA, Harezlak J, Chen KY, Matthews CE, Koster A, et al. Daily physical activity patterns from hip- and wrist-worn accelerometers. Physiol Meas. 2016;37(10):1852–61.
Shrout PE, Fleiss JL. Intraclass correlations: uses in assessing rater reliability. Psychol Bull. 1979;86(2):420–8.
Sirard JR, Trost SG, Pfeiffer KA, Dowda M, Pate RR. Calibration and evaluation of an objective measure of physical activity in preschool children. J Phys Act Health. 2005;2(3):345–57.
Sirichana W, Dolezal BA, Neufeld EV, Wang X, Cooper CB. Wrist-worn triaxial accelerometry predicts the energy expenditure of non-vigorous daily physical activities. J Sci Med Sport. 2017;20:761–5.
Swartz AM, Strath SJ, Bassett WI, O’Brien DR, King GA, Anisworth BE. Estimation of energy expenditure using CSA accelerometers at hip ad wrist sites. Med Sci Sports Exerc. 2000;32:S450–6.
Telama R, Yang X, Leskinen E, Kankaanpää A, Hirvensalo M, Tammelin T, et al. Tracking of physical activity from early childhood through youth into adulthood. Med Sci Sports Exerc. 2014;46(5):955–62.
Tremblay MS, Barnes JD, Copeland JL, Esliger DW. Conquering childhood inactivity: is the answer in the past? Med Sci Sports Exerc. 2005;37:1187–94.
Treuth MS, Schmitz K, Catellier DJ, McMurray RG, Murray DM, Almeida MJ, et al. Defining accelerometer thresholds for activity intensities in adolescent girls. Med Sci Sports Exerc. 2004;36(7):1259–66.
Troiano RP, Berrigan D, Dodd KW, Mâsse LC, Tilert T, McDowell M. Physical activity in the United States measured by accelerometer. Med Sci Sports Exerc. 2008;40(1):181–8.
Trost S, Kerr L, Ward D, Pate R. Physical activity and determinants of physical activity in obese and non-obese children. Int J Obes Relat Metabol Disord. 2001;25:822–9.
Trost SG, Pate RR, Freedson PS, Sallis JF, Taylor WC. Using objective physical activity measures with youth: how many days of monitoring are needed? Med Sci Sports Exerc. 2000;30(2):426–31.
Trost SG, Loprinzi PD, Moore R, Pfeiffer KA. Comparison of accelerometer cut-points for predicting activity intensity in youth. Med Sci Sports Exerc. 2011;43:1360–8.
Trost SG. State of the art reviews: measurement of physical activity in children and adolescents. Am J Lifestyle Med. 2007;1:299–314.
Twisk J, Mellenbergh G, van Mechelen W. Tracking of biological and lifestyle cardiovascular risk factors over a 14-year period. Am J Epidemiol. 1997;145:888–95.
van Cauwenberghe EV, Labarque V, Trost SG, De Bourdeaudhuij I, Cardon G. Calibration and comparison of accelerometer cut points in preschool children. Int J Pediatr Obes. 2010;6(2–2):e582–9.
van Hees VT, Gorzelniak L, Dean León EC, Eder M, Pias M, Taherian S, et al. Separating movement and gravity components in an acceleration signal and implications for the assessment of human daily physical activity. PLoS ONE. 2013;8(4):e61691.
van Hees VT, Renström F, Wright A, Gradmark A, Catt M, Chen KY, et al. Estimation of daily energy expenditure in pregnant and non-pregnant women using a wrist-worn tri-axial accelerometer. PLoS ONE. 2011;6(7):e22922.
van Hees VT. GGIR: raw accelerometer data analysis. R package version 1.5–16. 2018. https://CRAN.R-project.org/package=GGIR. Accessed 2 May 2018.
Vanhelst J, Béghin L, Salleron J, Ruiz JR, Ortega FB, Ottevaere C, et al. Impact of the choice of threshold on physical activity patterns in free living conditions among adolescents measured using a uniaxial accelerometer: the HELENA study. J Sports Sci. 2014a;32(2):110–5.
Vanhelst J, Fardy PS, Duhamel A, Béghin L. How many days of accelerometer monitoring predict weekly physical activity behaviour in obese youth? Clin Physiol Funct Imaging. 2014b;34(5):384–8.
Ward DS, Evenson KR, Vaughn A, Rodgers AB, Troiano RP. Accelerometer use in physical activity: Best practices and research recommendations. Med Sci Sports Exerc. 2005;37(11):582–8.
Wareham N, Rennie K. The assessment of physical activity in individuals and populations: why try to be more precise about how physical activity is assessed? Int J Obes (Lond). 1998;22:S30–8.
Whelton PK, He J, Appel LJ, Cutler JA, Havas S, Kotchen TA, et al. National high blood pressure education program coordinating committee. Primary prevention of hypertension: clinical and public health advisory from the national high blood pressure education program. JAMA. 2002;288(15):1882–8.
Whitaker R, Wright J, Pepe M, Seidel K, Dietz W. Predicting obesity in young adulthood from childhood and parental obesity. N Engl J Med. 1997;337:869–73.
World Health Organization. Global recommendations on physical activity for health. 2010. http://www.who.int/dietphysicalactivity/publications/9789241599979/en/. Accessed 2 May 2018.
Yang CC, Hsu YL. A review of accelerometry-based wearable motion detectors for physical activity monitoring. Sensors. 2010;10(8):7772–88.
Zakeri IF, Adolph AL, Puyau MR, Vohra FA, Butte NF. Cross-sectional time series and multivariate adaptive regression splines models using accelerometry and heart rate predict energy expenditure of preschoolers. J Nutr. 2013;143(1):114–22.
Zhang JH, Macfarlane DJ, Sobko T. Feasibility of a chest-worn accelerometer for physical activity measurement. J Sci Med Sport. 2016;19(12):1015–9.
Zhou SM, Hill RA, Morgan K, Stratton G, Gravenor MB, Bijlsma G, et al. Classification of accelerometer wear and non-wear events in seconds for monitoring free-living physical activity. BMJ Open. 2015;5(5):e007447.
Zhou W, Owen N. Sedentary behavior and health concepts, assessments, and interventions. Champaign, IL: Human Kinetics; 2017.
Acknowledgements
The development of instruments, the baseline data collection, and the first follow-up work as part of the IDEFICS study (www.idefics.eu) were financially supported by the European Commission within the Sixth RTD Framework Programme Contract No. 016181 (FOOD). The most recent follow-up including the development of new instruments and the adaptation of previously used instruments was conducted in the framework of the I.Family study (www.ifamilystudy.eu) which was funded by the European Commission within the Seventh RTD Framework Programme Contract No. 266044 (KBBE 2010–14).
We thank all families for participating in the extensive examinations of the IDEFICS and I.Family studies. We are also grateful for the support from school boards, headmasters, and communities.
Author information
Authors and Affiliations
Corresponding author
Editor information
Editors and Affiliations
Rights and permissions
Copyright information
© 2019 Springer Nature Switzerland AG
About this chapter
Cite this chapter
Konstabel, K., Chopra, S., Ojiambo, R., Muñiz-Pardos, B., Pitsiladis, Y. (2019). Accelerometry-Based Physical Activity Assessment for Children and Adolescents. In: Bammann, K., Lissner, L., Pigeot, I., Ahrens, W. (eds) Instruments for Health Surveys in Children and Adolescents. Springer Series on Epidemiology and Public Health. Springer, Cham. https://doi.org/10.1007/978-3-319-98857-3_7
Download citation
DOI: https://doi.org/10.1007/978-3-319-98857-3_7
Published:
Publisher Name: Springer, Cham
Print ISBN: 978-3-319-98856-6
Online ISBN: 978-3-319-98857-3
eBook Packages: MedicineMedicine (R0)