Abstract
In this paper, a methodology to arrive at the optimal end milling parameters of a Al7075-SiC co-continuous ceramic composite is presented. The composite was manufactured by gravity infiltration and end milling was performed. The effect of machining parameters namely, cutting speed, feed and depth of cut on multi-responses of surface roughness and tool wear were evaluated. A Taguchi L9 orthogonal array was formulated and the significant factors were ascertained using ANOVA. The optimal milling parameters were then identified using the grey relational grade as a performance measure. Tests have shown that, by applying this technique, an improvement in the response characteristics of surface roughness and tool wear has been achieved.
Access provided by CONRICYT-eBooks. Download chapter PDF
Similar content being viewed by others
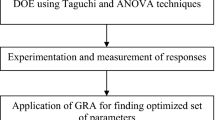
Keywords
- Al7075
- SiC
- Co-continuous composite
- End milling
- ANOVA
- Interpenetrating phase composite
- IPC
- C4
- Grey relational analysis
- GRA
1 Introduction
A metal-ceramic composite in which both the phases are continuous and interpenetrating is termed as a co-continuous composite [1]. These composites exhibit superior mechanical properties and thermal shock resistance owing to their uniform three dimensionally interconnected microstructure [2, 3]. Further, the addition of a metal (ductile) phase to the ceramic, leads to a composite with enhanced toughness [4]. By varying the volume fraction of each phase, the desired hardness, strength and fracture toughness can be achieved [5]. Due to these advantages, such composites have been widely studied and applied in defence sector [6], aerospace [7] and automotive industries [8, 9]. Co-continuous composites were first developed using the reactive metal penetration (RMP) technique by Breslin [10]. Vecchia et al. [11] performed RMP of silica glass with 99.9% pure aluminium and Al–Si (10%) alloy as infiltrating alloys. It was found that the composites thus fabricated, had very low thermal expansion, high stiffness, improved toughness and impact strength, appreciable bending and compression strength compared with traditional ceramics. Co-continuous ceramic composites (C4) were manufactured by infiltration of silica glass preform with 1050 A aluminium alloy by Pavese et al. [1]. The fatigue limit was estimated to be 91 MPa at 107 cycles. Such composites were suitable for applications involving high cycle fatigue. Banerjee and Roy [12] studied the displacement reaction between Al and SiO2 which yielded an interpenetrating composite. The study reported that the composite possessed enhanced density and compressive strength. It was also amenable for near net-shape fabrication. Evarts [13] studied advanced processing techniques for co-continuous ceramic composites. The study reported that addition of copper to liquid aluminium bath reduced the characteristic microstructure length of the composite. This led to an increase in strength, toughness and hardness of the composite. Daehn and Breslin [9] attempted to create co-continuous composites for friction and braking applications. The study showed that the presence of SiC improved thermal conductivity of the composite. Since they were light weight with excellent frictional properties, these composites were proposed to be next-generation rotor materials. Clarke [14] elaborated on the various processing techniques to fabricate interpenetrating composite structures. Though numerous studies on processing, fabrication techniques and properties of C4 are present in literature, limited studies are available on machinability of co-continuous composites. Roy et al. [15] studied the factors influencing the behaviour of Al2O3/Al co-continuous composites during wirecut electrical discharge machining (WEDM). It was found that the EDM method created a heat-affected surface layer. This led to the formation of pores and cracks in the composite. Goswami et al. [16] evaluated MRR, cutting forces and surface finish during electrochemical grinding (ECG) of an Al2O3/Al interpenetrating phase composite through Taguchi-design-based experimental studies. It was reported that applied voltage, concentration and depth of cut were the three important process parameters to obtain the best surface finish. Despite their low cost and appealing properties, challenges in fabrication and machining of interpenetrating composites have limited their usage in industrial applications [16]. In particular, very few studies are available on end milling of Al7075-SiC co-continuous metal-ceramic composite. In the present research, such a composite was at first, manufactured by gravity infiltration method. Secondly, the effect of three end milling parameters namely, speed, feed and depth of cut on tool wear and surface roughness of the composite was studied. The final objective was to solve this multi-response optimization problem using Grey Relational Analysis (GRA).
2 Experiments
2.1 Selection of Metal and Ceramic Phase
A three-dimensionally porous SiC foam with 75% purity as shown in Fig. 1, was selected as the ceramic phase. The foam is a commercially graded ceramic filter used in foundries for entrapping harmful slags, sand and dross inclusions. The pore size of the foam was 10 ppi, with a porosity of 80%.
The base metal alloy used in the study was Al7075. Among aluminium wrought alloys, the 7-series alloys exhibit the highest strength. Hence, they are typically used in defence applications [17]. Spectroscopic analysis was performed to verify the chemical composition of the commercial grade Al7075 alloy. As can be seen in Table 1, the major constituent of this alloy is zinc, followed by magnesium. The analysis substantiated that the commercially acquired sample confirmed to ASM alloy specifications.
2.2 Manufacturing of the Composite
The composite was fabricated by gravity infiltration technique. Here, the molten metal alloy was infiltrated into the ceramic foam in a resistance furnace having a maximum temperature of 1100 °C and maximum power of 4500 W. The schematic of the experimental setup is depicted in Fig. 2.
The SiC foam was cut into a sample of size 50 × 25 × 25 mm. It was preheated in a graphite crucible to a temperature of 800 °C. Simultaneously, the Al alloy was melted in another crucible. The temperature was set to 150 °C in excess of its melting point in order to ensure better fluidity of the alloy. After 1.5 h, the molten Al 7075 is poured into the crucible containing preheated SiC. To arrest the possibility of the foam floating on to the surface due to buoyancy, a carbon rod was used to hold it in place. The setup was maintained at 800 °C for a period of one hour to allow the molten metal to infiltrate the pores of the SiC foam. The crucible was then taken out and cooled for one hour at room temperature. The co-continuous composite thus fabricated, was machined out with utmost care by a sequence of operations namely, shaping, milling, and grinding.
3 End Milling of Al7075-SiC Composite
End milling was performed using a 5 mm solid carbide tool in uncoated condition as shown in Fig. 3. Generally, PCD tools are used in finish machining operations. However, the high cost of PCD tools increases the costs of machining composites. Hence, it is necessary to carry out machinability studies using carbide tools [18].
Slots were machined in the composite using a Makino VMC-S33 machine shown in Fig. 4. For aluminium alloys, the maximum rated speed and feed rate of the tool was 8960 rpm and 995 mm/min respectively. Speed, feed and depth of cut were the process parameters considered to investigate tool wear and surface roughness of the composite. The levels of the factors were fixed by conducting trial experiments and also, based on the rated speeds and feeds suggested by the tool manufacturer for aluminium alloys. Table 2 depicts the various levels of the parameters.
4 Taguchi Design
A Taguchi L9 orthogonal array [16] was chosen to analyse the results of machining. Two replications were carried out for each of the nine process designs and the averages were tabulated. The Taguchi array and response values for the experimental runs are shown in Table 3. The average surface roughness (Ra) in microns was measured using a KosakaLab Surfcorder and tool wear (wear-land wear) in mm was evaluated using DinoCapture software. The volume of material machined, material removal rate (MRR in mm3/min) was also observed and tabulated in Table 3.
4.1 Signal to Noise (S/N) Ratio
Table 4 lists the signal to noise (S/N) ratios obtained by the analysis of Taguchi design shown in Table 3. In order to diminish the effect of noise factors, higher values of S/N ratios are preferred in experiments. In machining, lower surface roughness and tool wear are desired. Hence, smaller-is-better characteristic is chosen for calculating the S/N ratio. The parameters with the largest value of delta are considered to be most significant.
From Table 4, it can be inferred that, for lesser tool wear and surface roughness during end milling of the composite, cutting speed is the most significant parameter; followed by feed rate and depth of cut. The main effects plots depicted in Fig. 5 and Fig. 6 supplement the outcome that cutting speed is the significant parameter.
4.2 General Linear Model (GLM) ANOVA
Using the adjusted sum of squares technique, GLM ANOVA was computed to determine the percentage contribution of each of the end milling parameters. ANOVA for surface roughness and tool wear is shown in Tables 5 and 6.
In case of surface roughness, the R-square value obtained was 97.58%. Cutting speed was of high significance with highest F-value of 25.79 as can be seen in Table 5. Similarly, for tool wear, the R-square value obtained was 98.62% with cutting speed being of highest significance as can be seen in Table 6. Moreover, P-values being less than 0.05 affirmed the S/N ratio results for surface roughness and tool wear indicating cutting speed to be the dominant cutting parameter.
4.3 Percentage Contribution of Factors in Milling of Composite
The percentage contribution of factors towards the variation in responses namely, surface roughness and tool wear were estimated using statistical analysis of means. The expression for finding percentage contribution is given by Eq. (1).
The total sum of squares and individual sum of squares for surface roughness and tool wear were obtained from Table 5 and Table 6 respectively.
It is evident from Table 7, that cutting speed has a major impact on both surface roughness and tool wear. This confirmed the results from S/N ratio analysis in Table 4.
5 Grey Relational Analysis (GRA)
A part of grey system theory, GRA is performed in cases where information on the model is incomplete. GRA provides an efficient solution to a multiple input, multiple-output problem [19]. The relationship between cutting parameters and machining performance can be found using this technique. The sequence of steps in GRA is as follows.
-
Step 1: Data Pre-processing: Normalization of the Responses
The responses obtained from the experimental runs need to be normalized to ensure that the scatter range of the sequence is small. The expressions for two of the most common scenarios are shown in Eqs. (2) and (3).
For larger is better;
$${\text{X}}_{\text{ij}} = \frac{{{\text{Y}}_{\text{ij}} - { \hbox{min} }({\text{Y}}_{\text{ij}} )}}{{\hbox{max} ( {\text{Y}}_{\text{ij}} ) - { \hbox{min} }({\text{Y}}_{\text{ij}} )}}$$(2)For smaller is better;
$${\text{X}}_{\text{ij}} = \frac{{\hbox{max} ( {\text{Y}}_{\text{ij}} ) - {\text{Y}}_{\text{ij}} }}{{\hbox{max} ( {\text{Y}}_{\text{ij}} ) - { \hbox{min} }({\text{Y}}_{\text{ij}} )}}$$(3)where Xij is the normalized response value and Yij is the response value obtained from experimental runs. During any machining process, larger values are desired for MRR and therefore Eq. (2) is utilized. However, for surface roughness and tool wear, smaller values are preferred. Hence, normalization is performed using Eq. (3). Table 8 shows the normalized results for the composite.
Table 8 GRA for composite -
Step 2: Grey Relational Coefficient (\(\upxi\))
This value indicates the degree of relation between every sequence in the experimental runs. It is calculated as shown in Eq. (4)
$$\upxi_{\text{ij}} = \frac{{\Delta _{ \hbox{min} } + {\zeta \Delta }_{ \hbox{max} } }}{{\Delta _{\text{i}} + {\zeta \Delta }_{ \hbox{max} } }}$$(4)where ‘\(\upxi\)’ is the grey relational coefficient, ‘\(\upzeta\)’ is the distinguishing coefficient. ‘∆’ is treated as a quality loss from the target value; calculated as difference between ‘1’ and normalized value. Table 8 displays the ‘\(\upxi\)’ for the composite computed using Eq. (4).
-
Step 3: Overall Grey Relational Grade
On averaging the grey relational coefficients, the overall grey relational grade ‘\(\upgamma_{\text{i}}\)’ is obtained according to Eq. (5).
$$\upgamma_{\text{i}} = \frac{1}{\text{n}}\mathop \sum \limits_{{{\text{k}} = 1}}^{\text{n}}\upxi_{\text{ij}}$$(5)The grey relational grade (GRG) and corresponding rankings for composite is depicted in Table 8. A higher value of GRG represents better quality of the product.
The factor effects and optimal level of each factor can be determined on the basis of GRG. For each level of the machining parameters of the composite, the mean value is computed and displayed in Table 9. The highest value of GRG corresponds to the optimal levels of the machining parameters. Table 9 shows that the optimum levels are A3, B3 and C2 with feed being the most significant factor as evident from the rank.
In order to quantify the percentage contribution of the end milling parameters, an ANOVA was performed on the GRG values of the composite as shown in Table 10. The main effects plot for the same is displayed in Fig. 7. The percentage contribution shows that feed rate, followed by cutting speed are the major parameters, which significantly affect the responses. The R-squared value was close to 97%.
5.1 Confirmation Tests
The final step is to predict the grey relational grade and verify whether the performance characteristic has improved using the optimal level of cutting parameters. The estimated grey relational grade can be computed by the formula in Eq. (6).
The predicted responses for surface roughness and tool wear were estimated by the formula in Eq. (7).
Table 11 shows the correlation between predicted values and experimental observations for the optimum settings during end milling of the composite. It can be seen that, the experimental values correspond to the values predicted by GRA. Also, the surface roughness and tool wear after GRA analysis is found to be less when compared with the initial settings. This is characterized by an improvement in the grey relational grade.
6 Conclusions
In this paper, the effect of varying three machining parameters namely speed, feed and depth of cut during end milling of a C4 consisting of Al7075-SiC was presented. Using GRA, from Table 9, it was found that the optimal setting for better surface finish and lesser tool wear was A3, B3 and C2. Furthermore, feed rate with 53.04% and cutting speed with 38.09% were found to be the significant factors contributing to the machining performance. Results from GRA analysis, presented in Table 11, have also shown that in case of the composite, the values of surface roughness have greatly improved by 63% and tool wear by 33% when compared with the initial machining settings. This paper has thus, applied Taguchi design and GRA to improve the multi-response characteristics during end milling of a co-continuous composite.
References
Pavese, M., Fino, P., Ugues, D., Badini, C.: High cycle fatigue study of metal-ceramic co-continuous composites. Scr. Mater. 55, 1135–1138 (2006)
Lu, Y., Yang, J., Lu, W., Liu, R., Qiao, G., Bao, C.: The mechanical properties of co-continuous Si3N4/Al composites manufactured by squeeze casting. Mater. Sci. Eng. A 527, 6289–6299 (2010)
Wang, L., Fan, Q., Li, G., Zhang, H., Wang, F.: Experimental observation and numerical simulation of SiC3D/Al interpenetrating phase composite material subjected to a three-point bending load. Comput. Mater. Sci. 95, 408–413 (2014)
Marchi, C.S., Kouzeli, M., Rao, R., Lewis, J.A., Dunand, D.C.: Alumina—aluminum interpenetrating-phase composites with three-dimensional periodic architecture. Scr. Mater. 49, 861–866 (2003)
Li, G., Zhang, X., Fan, Q., Wang, L., Zhang, H., Wang, F., Wang, Y.: Simulation of damage and failure processes of interpenetrating SiC/Al composites subjected to dynamic compressive loading. Acta Mater. 78, 190–202 (2014)
Chang, H., Binner, J., Higginson, R., Myers, P., Webb, P., King, G.: High strain rate characteristics of 3-3 metal-ceramic interpenetrating composites. Mater. Sci. Eng., A 528, 2239–2245 (2011)
Han, J., Hong, C., Zhang, X., Wang, B.: Thermal shock resistance of TiB2-Cu interpenetrating phase composites. Compos. Sci. Technol. 65, 1711–1718 (2005)
Etter, T., Kuebler, J., Frey, T., Schulz, P., Löffler, J.F., Uggowitzer, P.J.: Strength and fracture toughness of interpenetrating graphite/aluminium composites produced by the indirect squeeze casting process. Mater. Sci. Eng. A 386, 61–67 (2004)
Daehn, G.S., Breslin, M.C.: Co-continuous composite materials for friction and braking applications. JOM 58, 87–91 (2006)
Breslin, M.C.: United States Patent 5,214,011. 788, 783–788 (1993)
La Vecchia, G.M., Badini, C., Puppo, D., D’Errico, F.: Co-continuous Al/Al2O3 composite produced by liquid displacement reaction: relationship between microstructure and mechanical behavior. J. Mater. Sci. 38, 3567–3577 (2003)
Banerjee, S., Roy, S.K.: Net-shape forming of bi-continuous Al2O3/Al composite by displacement reaction. Mater. Chem. Phys. 67, 243–248 (2001)
Evarts, J.S.: Advanced processing techniques for co-continuous ceramic composites 102 (2008)
Clarke, D.R.: Interpenetrating phase composites. J. Am. Ceram. Soc. 75, 739–758 (1992)
Roy, S.K., Pal, S., Ghosh, S.K., Dey, P.P.: Machining of interpenetrating phase alumina/aluminium composite by electrical discharge technique machining of interpenetrating phase alumina/aluminium. Trans. Indian Ceram. Soc. 67, 75–80 (2008)
Goswami, R.N., Mitra, S., Sarkar, S.: Experimental investigation on electrochemical grinding (ECG) of alumina-aluminum interpenetrating phase composite. Int. J. Adv. Manuf. Technol. 40, 729–741 (2009)
Cobden, R., Alcan, B.: Aluminium: Physical Properties, Characteristics and Alloys (1994)
Kök, M.: A study on the machinability of Al2O3 particle reinforced aluminium alloy composite. In: 11th International In-organic Bonded Fiber Composites Conference, pp. 272–281 (2008)
Kuo, Y., Yang, T., Huang, G.-W.: The use of grey relational analysis in solving multiple attribute decision-making problems. Comput. Ind. Eng. 55, 80–93 (2008)
Author information
Authors and Affiliations
Corresponding author
Editor information
Editors and Affiliations
Rights and permissions
Copyright information
© 2017 Springer International Publishing AG
About this chapter
Cite this chapter
Prasanth, A.S., Ramesh, R. (2017). Investigation of Surface Roughness and Tool Wear in End Milling of Al7075-SiC Co-continuous Composite. In: Silva, L. (eds) Materials Design and Applications. Advanced Structured Materials, vol 65. Springer, Cham. https://doi.org/10.1007/978-3-319-50784-2_24
Download citation
DOI: https://doi.org/10.1007/978-3-319-50784-2_24
Published:
Publisher Name: Springer, Cham
Print ISBN: 978-3-319-50783-5
Online ISBN: 978-3-319-50784-2
eBook Packages: Chemistry and Materials ScienceChemistry and Material Science (R0)