Abstract
The dynamic of Land Use and Land Cover (LULC) at the Chittar catchment is to obtain the spatial deviation for the historical and forecast period from 2001 to 2026. Spatial deviation occurred within the five LULC features such as the agricultural land, urban land, wasteland, forest, and water bodies which are classified and mapped at the spatial distribution from the years 2001, 2006, 2011, and 2016 using supervised classification. Then, LULC forecasts 2021 and 2026 using the Artificial Neural Network-based Cellular Automata (ANN_CA) method. The catchment scale LULC changes; 2001 and 2006 data are used as base maps to determine the transition potential model. Then ANN_CA is supported to simulate the LULC for the year 2011. The level of spatial matching between the simulated and field LULC for the year 2011 is measured using kappa statistics. The overall spatial matching between the two LULC is 92%, and the kappa coefficient value is 0.88. Twenty-six years of average percentage in areal contribution for agricultural, forest, urban, water bodies, and wasteland is 58%, 16%, 7.5%, 3.5%, and 15%, respectively. This LULC change data is most significant in the applications of hydrological modeling, irrigation management, urban planning, land and water resource management, etc.
Access provided by Autonomous University of Puebla. Download chapter PDF
Similar content being viewed by others
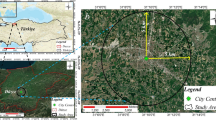
Keywords
1 Introduction
The phrase Land Use and Land Cover (LULC) generally refers to the spatial mapping of natural and anthropogenic sources of materials on the landscape within a definite time. Land cover is the physical process that generally covers the earth’s surface. Land use is to describe how the land is utilized. LULC changes are one of the important parameters that affect the global environment. The LULC change also helps to model environmental problems such as flooding, landslides, biodiversity loss, climate change, etc. (Mousavi et al., 2019). Thus, LULC change also causes the socio-economic problem due to improper land management (Reis, 2008). Mapping and forecasting of LULC are important for environmental monitoring studies which include:
-
Sustainable natural resource development and management.
-
Land and water resource development and management.
-
Wildlife habitat protection.
-
Baseline mapping for many hydrological modeling studies.
-
Urban planning.
-
Coastal management.
-
Urban growth modeling.
-
Transport planning and management.
-
Damage delineation (such as earthquake, drought, flood, forest fire, etc.)
-
Guiding the real estate business investment and development.
-
Target detection-identification of school, hotel, hospital, water bodies.
-
Deforestation and forest conservation.
The LULC changes occur within its own features of LULC wherein the environmental hazards occur due to urbanization (alteration of cropland to an urban area), for instance, the rotation of crops in accordance with the seasons. There is a direct impact on the social-economic development with the conversion of the seasonal cropland to the uncultivated, which is the effect of the water scarcity that the fluctuation has led in seasons during the semiarid conditions. The key research for most of the developing countries revolves around water resources, as most of the researchers are centered on the changes of LULC and their impacts on the water and land resources. The findings of the availability of water present either as seasonally or annually can be achieved by the morphological analysis of the surface water body, which is led mainly by the changes in LULC; also, this LULC changes to aid in developing the hydrological model, which analyzes the hydrological response impact.
This study explores the historical and future LULC changes over the Chittar catchment of Thamirabarani Basin, Tamil Nadu (Fig. 3.1). It aligns in a semiarid climate. The catchment has been divided into five LULC classes’ descriptions as shown in Table 3.1, viz., agricultural land, urban land, wasteland, forest, and water bodies (Roy & Inamdar, 2019). The historical period maps are developed from Landsat 5 Thermal Mapper, Landsat 7 Enhanced Thematic Mapper, and Landsat 8 Operational Land Imager in Geographical Information System (GIS) platform for the years 2001, 2006, 2011, and 2016. The commercially available LULC forecast model packages include CA_MARKO, Dyna-CLUE, and ANN_CA (Aarthi & Gnanappazham, 2018). This study uses an Artificial Neural Network-based Cellular Automata (ANN_CA) model to forecast the LULC over the Chittar catchment. The ANN_CA model is to learn the transition of LULC over Chittar catchment from historical data for the years 2001 and 2006 which are used to forecast LULC in 2011. The forecasted LULC in 2011 is validated with 2011 field data using kappa statistics (Al-Fares, 2013). The LULC is part of the hydrological response unit, which induces the finding the certain water balance and runoff component estimations such as manning roughness coefficient and SCS-CN. By these parameters it evolves the WBC, such as runoff and infiltration. The LULC also evolves the water balance components such as interception, depression storage, and evapotranspiration. LULC is dynamic in nature, caused by anthropogenic activity or natural causes. This concept is applied to the different time periods of LULC that link with the suitable land features with their standard parameter available in the default geodatabase.
2 Study Area
The Chittar River originated from the Courtallam hills in the southern part of Western Ghats and is one of the major tributaries of the Thamirabarani basin. Its area spans about 1300 km2 with latitude and longitude varying from 8°45′N to 9°15′N and 77°10′E to 77°50′E, respectively (Fig. 3.1). The length of the river travels about 82 km to reach the main river. The dividing line of the catchment separates the Kerala and Tamil Nadu border. It lies in a semiarid climate zone with temperatures ranging from 25 to 40 °C. Northeast monsoon of the Chittar catchment starts from October to December and receives a 50% of annual rainfall; the Southwest monsoon starts from the month of June to August and receives a 30% of rainfall. The total rainfall of the Chittar catchment is about 880 mm. The catchment relief varies from 25 to 1710 m above MSL. The upper catchment is very steep, with a slope of more than 15%. The downstream side of the catchment is generally plain, with an average elevation of about 60–80 m and a slope of less than 1%.
3 Methodology
The LULC map is delineated from Landsat imagery (URL: glovis.usgs.gov). Landsat is a multispectral image with 30 m of spatial resolution jointly released by NASA and USGS (details shown in Fig .3.1). The visible near-infrared (VNIR) region has three bands with a spectral range of blue (0.45–0.52 μm), green (0.52–0.60 μm), and red (0.63–0.69 μm) which were captured using multispectral satellite sensors. Using three bands of Landsat imagery, LULC features were delineated in the GIS environment (Trujillo-Jiménez et al., 2022). The Resources-2 is available only within India and has a succession of 5.8 m VNIR by the Indian Space Research Organization, Government of India. The Landsat 8 OLI is launched with 30 m VNIR by the Earth Resources Observation and Science Center, NASA; it’s available worldwide and has its finest provision on the coastal zone regulations and surface water resources. The Sentinel 2 was launched by European Space Agency to monitoring the morphology of land with 13 spectral bands. The Landsat satellite program series provides enormous information about LULC data. This is one of the world’s largest collections of global land resources data, which is the available free and open Internet.
The Landsat imagery has a temporal resolution of 8 days starting from 1970 to the present. Lone and Mayer (2019) used the IRS LISS III and P6 to classify the LULC features using supervised classification. The series of Landsat imagery, as mentioned in Table 3.2, are used in this study. The Landsat imagery data are projected by Universal Transverse Mercator (UTM) zone 44 N in the World Geodetic System (WGS) datum named WGS_1984_UTM_Zone_44N to certify the consistency between the datasets during analysis. The Level 1 of the National Remote Sensing Centre classification system is used in this study (NRSC, 2014). The LULC Level 1 consists of five features (Roy & Inamdar, 2019): land use considers the urban land and agricultural land, and land cover considers wasteland, forest, and water bodies (Table 3.2). The given features are classified with the supervised classification (Vivekananda et al., 2021). The maximum likelihood classifier is one of the popular supervised classification systems that gather each trained pixel information from Landsat imagery (Lone & Mayer, 2019). Trained pixels are processed in the maximum likelihood classifier per the equation below to classify the LULC features. The maximum likelihood classifier is one of the popular algorithms for the classification of satellite images, in which a pixel with the maximum likelihood (MLA) is classified into the corresponding class:
where A = features; x = number of bands in dimensional; p(A) = probability of all features; |ΣA| = determinant of the covariance matrix in features; ΣA−1 = inverse matrix; and X = mean vector.
The maximum likelihood classifier is used in this study, which is one of the popular satellite data processing methods. The LULC data for the years 2001, 2006, 2011, and 2016 over the Chittar catchment are prepared and used for ANN model training.
The concepts of Cellular Automata (CA) were jointly induced by two great mathematicians Alan Turing and John von Neumann, during 1930s. The CA is a very popular method applied for modeling the LULC and its changes, estimating the pixel value according to its initial state. The pixel value determines according to their surrounding neighborhood effects and transition rules. A CA only effectively models the nonlinear spatially stochastic LULC change processes. The LULC is delineated in the GIS environment and prepared for the year 2001, 2006, 2011, and 2016, respectively. Here, the ANN_CA model is capable of predicting the future LULC from the transition from the previous state of LULC. Artificial Neural Network (ANN) is used for training to find the transition potential within LULC features (Mas et al., 2014). Again, the CA technique is used to simulate future data by adopting the transition potential. Finally, ANN_CA is supported to forecast the future LULC (Li & Yeh, 2002; Qiang & Lam, 2015). Using Kappa statistics, ANN_CA-predicted 2011 LULC is validated with field 2011 LULC through the multi-resolution budget. The final model is used to forecast the LULC for the years 2021 and 2026. A flow chart of methodology to forecast LULC for 2021 and 2026 by using historical 5-year intervals of LULC is shown in Fig. 3.2.
Commercially available LULC forecasting model package includes CA_MARKOV, Dyna-CLUE, and ANN_CA. Here, the Chittar catchment is used to forecast the LULC using the ANN_CA model package (Basse et al., 2014; Qiang & Lam, 2015). Forecast LULC data for the years 2021 and 2026 are adopted for the methodology shown in Fig. 3.2. Modules for Land Use Change Simulations (MOLUSCE) is accessed as an open-source QGIS plugin to process the ANN_CA LULC change model (Measho et al., 2020). Input variables of ANN training datasets are of five stages: neighborhood pixels, learning rate, momentum, maximum iterations number, and hidden layers using multilayer perceptron. The number of hidden layers and neurons in each hidden layer has arrived arbitrarily. Input neurons are carried out as
where Cf = count of attained LULC features; Nb = neighborhood pixels size specified by the initial data; and Rf = summary band count of factor raster. Output neurons (M) usually are a count of unique features in the change map (Cf2). The classic back propagation algorithm with momentum is used to train the ANN model. Trained data rectification is performed as
where X = vector of neuron trained data; Dx = vector of trained LULC changes; n = iteration number; Lr = learning rate; and M = momentum.
The initial and final LULC datasets are used to delineate the transition potential. The transition potential is mapping the areal changes between the LULC features. The ANN is used in training to find out the transition potential of LULC features (Mas et al., 2014). Transition potential is trained between the years of 2001 and 2006 of LULC. Again, CA is the technique used for the simulation of future data by incorporating the modeled transition potential. Finally, the ANN_CA is supported to predict the 2011 LULC. Multi-resolution budget validates the two datasets on the five categories of plots (Fig. 3.3). These plots are based upon the quantity and location where perfect location information and perfect quantity information plots for ten iterations have reached a high value with others. It has achieved 88% of correctness with the historical 2011 LULC. Similarly, LULC of 2011 and 2016 is used for the ANN_CA to forecast LULC data for 2021. Further, the LULC of 2026 is forecasted from the LULC of 2016 and 2021.
4 Results and Discussions
The LULC change analysis is interpreted for 2001, 2006, 2011, and 2016 (Fig. 3.4). Thus, the process of LULC change analyzes the area contribution in km2 as shown in Fig. 3.5. Over the Chittar catchment, about 74% of the area is jointly covered by agricultural and forest land (Fig. 3.5). The remaining 26% area is covered by wasteland (20%), water bodies (4%), and urban land (2%). During the 20 years from 2001 to 2021, about 22 km2 of agricultural land gradually increased, and in the same period, 27 km2 of wasteland decreased. The increase in farming activities reclaims the wasteland over the Chittar catchment (Awotwi et al., 2015). Approximately 1% of the forest was converted to wasteland between 2001 and 2016, resulting in increased runoff in the foothills (Danáčová et al., 2020). About 20 km2 (i.e., 78% area) of urban land is increased from 2001 to 2016, and it’s driven by population growth. It is observed that agricultural and wastelands are converted into urban land to meet the sheltering needs of the increasing population. The 16% of water bodies in the Chittar catchment decreased between the periods of 2001 to 2016. The change of agricultural and urban land leads to causes critical environmental issues, likely flooding, landslides, etc., along the catchment.
The ANN_CA is used to delineate the LULC transition between 2001 and 2006. The trained ANN_CA model is used to forecast 2021 with the LULC data for 2011 and 2016. In the subsequent year 2016 and 2021, data are used to forecast 2026 LULC (Fig. 3.5). This model is further used to forecast 2011 from 2001 and 2006. A kappa value seems to be location matching with two spatial data. Here, this is matched up to approximately 88% of the area between the simulated 2011 and field 2011 LULC data. The validation results for ten iterations are shown in Fig. 3.3. Then the 2021 and 2026 LULC is forecasted using ANN_CA. From 2001 to 2026, about 6% of wasteland decreases, and alternately 3% of agricultural land is increased in the same period. Over the middle and lower portions of the Chittar watershed, agricultural land is being maintained to its greatest extent. Forest covers the upper part of the Chittar catchment. From 2001 to 2016, the forest decreased by 3.5% area was converted to a wasteland. The wasteland covers all parts of the catchment and scatters very well. Thus, a wasteland in 2016 was converted into agricultural land (about 1.5%) due to farming practices. Then agricultural land converts into an urban area (about 0.5%) in 2026 due to the rising of the population (Fig. 3.4). The maximum urban is the low region of forest area. They are Tenkasi, Shencottai, Courtallam, Kadaiyanallur, Alangulam, etc. Finally, 2026 water bodies continuously decrease up to 26% from 2001 over the Chittar catchment. Because of human activity, water bodies are being converted to urban and agricultural land.
Forecast LULC of 2026 shows fewer areal changes compared to the historical LULC; the areal changes of 2011 to 2016 show the frequent variation from 2001 to 2011 in agricultural and wasteland. The ANN_CA model is achieved by the neural systems that delineate areal changes between the LULC features. After 2016 LULC of area contribution describes the rise in the wasteland, and drop down from the agricultural land directly denotes the conversion of wasteland to agricultural. The wasteland is mostly a salt-affected region with low productivity. Again in 2026 LULC, it achieves the gain of agricultural land of approximately 5 km2 and a loss in the wasteland of about 13 km2. So, it seems to be a rise in moisture content due to climate change (Fig. 3.6). If the reduction of agricultural land happens within the catchments, it leads to an impact on the social and economic effects. Agriculture is the main source of increase in the national economy. In a historically agricultural community, a rise in uncultivated land creates social instability and conflict. Furthermore, the fall in agricultural productivity raises the likelihood of food production security threats (Rasool et al., 2021).
5 Change Detection
Change detection is the proper technique for estimating the spatial deviation between the two datasets. It can be calculated with the area units in km2. Change detection is the technique to analyze the mapped spatiotemporal LULC changes between the multi-temporal images. The change defection analysis is carried out for the period 2001 to 2026 between five subclasses over the Chittar catchment. Table 3.3 summarizes the area of increase (+) or decrease (−) in km2 during the consecutive 10-year periods. The 10 years of LULC maximum change is detection that occurred between agricultural and wasteland. Forest, urban land, and water bodies do not change much in 10 years. Due to the dominance of agricultural land, indirectly, the Chittar catchment indicates the water resources gained by their subsurface, i.e., groundwater or its surface, led by the irrigation practices. Forest is the only feature not disturbed by other features. Even though water bodies and urban land contribute at a minor level at this LULC change detection, efficiency is larger than any other features.
It shows the maximum gain of 20 km2 from 2011 to 2021 and 12 km2 from 2006 to 2016 for the agricultural land. Gain and loss of agricultural land denote the amount of food productivity. Loss of wasteland continuously changes by more than 5 km2 per decade. Suddenly, the increase of wasteland between 2016 and 2026 is 14 km2 due to the reduction of agricultural land. These changes occurred mainly due to soil degradation, land rehabilitation, and land productivity. Then, 2016 to 2026 has low gain/loss in LULC changes. Minimum LULC change happens in the forest and water bodies that range less than 10 km2. Agricultural and urban land was steadily incremented up to 2001–2026 and proportionally reduced in water bodies. It leads to the declination of water bodies as the massive increase in agricultural productivity in the riverbed. Similarly, the trend of 10 years is quite the same as 30 years also. The loss happened in the wasteland, forest, and water bodies and followed by gain happen in urban and agricultural land. The LULC change has been detected for 30 years and has a major impact on the hydrological model.
6 Conclusion
In the tropical climate of south India, it behaves as a seasonal variation in the temperature as well as the rainfall reflected in the landscape. In the Chittar catchment, hot weather (temperature) happens for 6 months in an annum, so it causes more evaporation and evapotranspiration in the agricultural land (70%) due to this solar energy; also, it converts the surface water bodies to dry land. However, surface irrigation completely stopped after 2011, as shown in the LULC dynamics map. Further, the probability of water resources purely depends upon rainfall and groundwater. The rest of the period leads to the direct supply of water (rainfall) into soil derived from the runoff, infiltration, and percolation characteristics over the catchments. As per the Chittar catchment, 16% of water bodies’ decline is visualized in 2001–2026 LULC dynamic map. The 40% of 2001 urban area rises to 2026 LULC map. Here, 58% of agricultural land is mapped along with the uncultivated land also, so the contribution of agricultural land has a meager change in the Chittar catchment. Uncultivated land has directed the wasteland, i.e., scrubland. Due to the source of groundwater potential site, agricultural activities still have achieved their best level in this Chittar catchment. Wasteland of 25 years has contributed to all the other features. The spatial distribution of wasteland is easier to interpret from the LULC dynamics map. Change detection helps to compare the LULC features in km2, where it interprets the major change between the agricultural land and wasteland. Other features are less contributed. Thus, the landscape phenomenon is attained by the combination of soil, LULC, and slope (i.e., HRU) of the Chittar catchment, which leads to a response of hydrologic characteristics in it. The HRU is the basis for many hydrological models at the catchment scale.
References
Aarthi, A. D., & Gnanappazham, L. (2018). Urban growth prediction using neural network coupled agents-based cellular automata model for Sriperumbudur Taluk, Tamil Nadu, India. Egyptian Journal of Remote Sensing and Space Science, 21(3), 353–362. https://doi.org/10.1016/j.ejrs.2017.12.004
Al-Fares, W. (2013). Historical land use/land cover classification using remote sensing: A case study of the Euphrates River basin in Syria. University of Jena, Germany, Springer Briefs in Geography, Springer. https://doi.org/10.1007/978-3-319-00624-6
Awotwi, A., Yeboah, F., & Kumi, M. (2015). Assessing the impact of land cover changes on water balance components of White Volta Basin in West Africa. Water Environment Journal, 29(2), 259–267. https://doi.org/10.1111/wej.12100
Basse, R. M., Omrani, H., Charif, O., Gerber, P., & Bódis, K. (2014). Land use changes modelling using advanced methods: Cellular automata and artificial neural networks. The spatial and explicit representation of land cover dynamics at the cross-border region scale. Applied Geography, 53, 160–171. https://doi.org/10.1016/j.apgeog.2014.06.016
Danáčová, M., Földes, G., Labat, M. M., Kohnová, S., & Hlavčová, K. (2020). Estimating the effect of deforestation on runoff in small mountainous basins in Slovakia. Water, 12, 3113. https://doi.org/10.3390/w12113113
Li, X., & Yeh, A. G. O. (2002). Neural-network-based cellular automata for simulating multiple land use changes using GIS. International Journal of Geographical Information Systems, 16(4), 323–343. https://doi.org/10.1080/13658810210137004
Lone, S. A., & Mayer, I. A. (2019). Geo-spatial analysis of land use/land cover change and its impact on the food security in district Anantnag of Kashmir Valley. GeoJournal, 84(3), 785–794. https://doi.org/10.1007/s10708-018-9891-2
Mas, J. F., Kolb, M., Paegelow, M., Olmedo, M. C., & Houet, T. (2014). Modelling land use/cover changes: A comparison of conceptual approaches and softwares. Environmental Modelling & Software, 51, 94–111. https://doi.org/10.1016/j.envsoft.2013.09.010
Measho, S., Chen, B., Pellikka, P., Trisurat, Y., Guo, L., Sun, S., & Zhang, H. (2020). Land use/land cover changes and associated impacts on water yield availability and variations in the Mereb-Gash River Basin in the Horn of Africa. Journal of Geophysical Research – Biogeosciences, 125(7), e2020JG005632. https://doi.org/10.1029/2020JG005632
Mousavi, S. M., Roostaei, S., & Rostamzadeh, H. (2019). Estimation of flood land use/land cover mapping by regional modelling of flood hazard at sub-basin level case study: Marand basin. Geomatics, Natural Hazards and Risk, 10(1), 1155–1175. https://doi.org/10.1080/19475705.2018.1549112
NRSC. (2014). Land use/Land cover database on 1:50,000 scale. Natural Resources Census Project, LUCMD, LRUMG, RSAA, National Remote Sensing Centre, ISRO, Hyderabad.
Qiang, Y., & Lam, N. S. (2015). Modeling land use and land cover changes in a vulnerable coastal region using artificial neural networks and cellular automata. Environmental Monitoring and Assessment, 187(3), 57. https://doi.org/10.1007/s10661-015-4298-8
Rasool, R., Fayaz, A., & ul Shafiq, M., Singh, H., Ahmed, P. (2021). Land use land cover change in Kashmir Himalaya: Linking remote sensing with an indicator based DPSIR approach. Ecological Indicators, 125, 107447. https://doi.org/10.1016/j.ecolind.2021.107447
Reis, S. (2008). Analyzing land use/land cover changes using remote sensing and GIS in Rize, North-East Turkey. Sensors, 8(10), 6188–6202. https://doi.org/10.3390/s8106188
Roy, A., & Inamdar, A. B. (2019). Multi-temporal Land Use Land Cover (LULC) change analysis of a dry semi-arid river basin in western India following a robust multi-sensor satellite image calibration strategy. Heliyon, 5(4), 01478. https://doi.org/10.1016/j.heliyon.2019.e01478
Trujillo-Jiménez, M. A., Liberoff, A. L., Pessacg, N., Pacheco, C., Díaz, L., & Flaherty, S. (2022). SatRed: New classification land use/land cover model based on multi-spectral satellite images and neural networks applied to a semiarid valley of Patagonia. Remote Sensing Applications: Society and Environment, 26, 100703. https://doi.org/10.1016/j.rsase.2022.100703
Vivekananda, G. N., Swathi, R., & Sujith, A. V. L. N. (2021). Multi-temporal image analysis for LULC classification and change detection. European journal of remote sensing, 54(sup2), 189–199. https://doi.org/10.1080/22797254.2020.1771215
Acknowledgments
Authors are very much thankful to our School of Civil Engineering, VIT, Chennai, Tamil Nadu, India, for providing research-related facilities to complete this research. Further, our institute is also sponsoring this publication. The authors are grateful to open-source Landsat series data provided by the US Geological Survey Earth explorer.
Declarations
Authors declare no conflict of interest.
Author information
Authors and Affiliations
Corresponding author
Editor information
Editors and Affiliations
Rights and permissions
Copyright information
© 2023 The Author(s), under exclusive license to Springer Nature Switzerland AG
About this chapter
Cite this chapter
Dinagarapandi, P., Saravanan, K., Mohan, K. (2023). Catchment Scale Modeling of Land Use and Land Cover Dynamics. In: Thambidurai, P., Dikshit, A.K. (eds) Impacts of Urbanization on Hydrological Systems in India. Springer, Cham. https://doi.org/10.1007/978-3-031-21618-3_3
Download citation
DOI: https://doi.org/10.1007/978-3-031-21618-3_3
Published:
Publisher Name: Springer, Cham
Print ISBN: 978-3-031-21617-6
Online ISBN: 978-3-031-21618-3
eBook Packages: Earth and Environmental ScienceEarth and Environmental Science (R0)