Abstract
The research envisaged focuses on risk management approach for development and optimization of enteric coated tablet of isoniazid giving extended release in pH 6.8 phosphate buffer. Risk assessment using failure mode and effects analysis was done to depict the effects of unambiguous failure modes related to particular formulation/process variable. A 23 full factorial design was employed for optimization of core tablet to investigate effect of amount of Polyox WSR 303 (A), hardness (B) and amount of ethyl cellulose (C) on percent drug release in pH 6.8 phosphate buffer. Main effects and interaction plots were generated to study effects of variables. The selection of optimized formulation was done on overlay contour plots and desirability function. The optimized formulation exhibited percent drug release at first hour of 26.97 %, second hour of 44.20 %, fourth hour of 66.15 % and eighth hour of 97.9 % in phosphate buffer pH 6.8. Akaike information criteria and Model selection criteria revealed that the model was best described by Korsmeyer–Peppas power law. The Kopcha and Peppas–Sahlin model revealed diffusion as predominant mechanism of release which may be due to high solubility of drug and drug loading. Enteric coating optimization revealed weight gain of 10 % w/w as optimum; giving nil release of isoniazid in 0.1 N hydrochloric acid. The composite desirability for optimized formulation computed using equations and software were 0.91 and 0.90 respectively. Capability analysis on reproducibility batches revealed all indices above 1.33 signifying process was within control of producing batches as per desired specifications.
Similar content being viewed by others
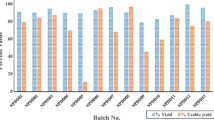
Avoid common mistakes on your manuscript.
Introduction
The drug delivery systems (DDS), which contemplates the carrier, the route and the target, has evolved into processes designed to enhance the efficacy of therapeutic agents through site-specific, modified or controlled release. This may involve augmented bioavailability, improved therapeutic index, improved biopharmaceutical properties, enhanced patient compliance, reduced side effects, etc. (du Toit et al. 2006; Batyrbekov et al. 1997). Amongst realm of DDS, oral route unambiguously, has been most sought especially in under developed or developing countries to epitomize the objectives like cost-effectiveness, feasibility and save resources (Singh et al. 2005). Of various oral DDS, site specific prolonged release formulations endow to be of greater interest to formulation scientists for ensuring optimal bioavailability or improve biopharmaceutical properties (du Toit et al. 2006).
Tuberculosis (TB) has remained, a significant health care problem since long times, particularly in developing countries. With respect to case notifications, 5.8 million newly diagnosed cases were notified to national TB control programmes conducted by World Health Organization in 2011 (Vora et al. 2013a). Isoniazid (INH) is the vital component in the current therapeutic treatment for TB. INH and rifampicin (RIF), the two most potent anti-TB drugs, kill more than 99 % of tubercular bacilli within 2 months of initiation of therapy (du Toit et al. 2006; Mitchison 1985; Iseman and Madsen 1989). But, one of the major drawbacks in the use of INH for the treatment of TB is the severe toxic/adverse effects associated with it; primarily hepatotoxicity because of metabolism of INH, especially acetylation, by N-acetyltransferase (Schaberg et al. 1996; Burman et al. 2001; Katzung 2001). The effect is genetically prominent in rapid acetylators leading to plasma concentrations approximately one third to half of that in slow acetylators and average half-lives are less than 1–3 h, respectively. Moreover these toxic effects lead to discontinuation of the therapy because of the lack of patient compliance which results in subtherapeutic concentrations of the drug in the blood, leading to treatment failure and also encourages the INH resistant strains of Mycobacterium tuberculosis (Ellard et al. 1972; Hiremath and Saha 2008). Secondly, as addressed in earlier studies that in the acidic pH of stomach, RIF reacts with INH to form an insoluble compound 3-formylrifamycin resulting in reduction of bioavailability of RIF to the extent of 30 % (Shishoo et al. 2001; Singh et al. 2001). Moreover, permeability studies have demonstrated INH is less permeated through the stomach due to its protonated form at acidic pH (pKa = 2) and is well absorbed through all the three segments of intestine (Mariappan and Singh 2003). Hence, this all urgently demanded and necessitated development of site specific extended release formulations of INH in order to improve biopharmaceutical properties, minimize interaction with RIF and providing sustained release especially in rapid acetylators.
Several studies have been reported in the literature addressing the importance of INH site specific formulations. Pund et al. reported delayed release INH formulation consisted of INH pellets (Pund et al. 2010). Gohel et al. developed floating tablets of RIF using hydrophilic hydroxypropylmethyl cellulose (HPMC) polymer and calcium carbonate which were consequently filled together with delayed release INH capsule in one large capsule (Gohel and Sarvaiya 2007). Patent WO2011/012987A1 disclosed composition of RIF, INH and piperine wherein the bioavailability of RIF by piperine is maintained in presence of INH. The invention claims delayed release form of INH preferably tablet for minimizing physical interaction of both the drugs in gastrointestinal tract by segregating drug delivery (Bhatt et al. 2011).
INH is an anti-microbial agent and its satisfactory amount should be released initially as loading dose to achieve its Minimum Inhibitory Concentration (MIC) to elicit required therapeutic effect in the body. On basis of its MIC, volume of distribution and fraction bioavailable, a minimum of approximately 16 % should be released as initial loading dose theoretically (data not shown). On succinct amount of literature search of site specific drug delivery of INH, no report has been found in the area of site specific extended release formulation using stepwise systemic quality by design (QbD) approach.
The major aims of this study were: (i) step wise systemic formulation development and optimization using QbD approach (ii) applying principles of risk assessment and Failure Mode and Effects Analysis (FMEA) for formulation development (iii) to implement 23 full factorial design as optimization technique for establishment of mathematical equations and graphical results, thus depicting a complete picture of variation of the product/process response(s) as a function of the input variables and (iv) to perform capability analysis to investigate spread and control of process on reproducibility.
Materials and methods
Materials
Isoniazid was purchased from S. D. Fine Chem Pvt. Ltd., India. Microcrystalline cellulose (MCC) (Avicel® PH 101 and Avicel® PH 102) and Talc (Signet Chemical Corporation, India), Polyethyleneoxide (Polyox WSR 303), Hydroxypropylmethyl cellulose (HPMC E5) and Ethyl Cellulose (Ethocel N 10) (Colorcon Asia Pvt. Ltd., India), colloidal silicon dioxide (Aerosil® 200) and Eudragit L-100 55 (Evonik Ind., Mumbai, India) were generously gifted from the indicated sources. Magnesium stearate, Butylated Hydroxy Toluene (BHT), Polyethylene Glycol (PEG 400) and Triethyl citrate (S. D. Fine Chem Pvt. Ltd., India) were purchased from the indicated source. All other ingredients, chemicals and reagents were of analytical grade and were used as received.
Quality Target Product Profile (QTPP) of INH site specific extended drug delivery
The quality traits that a formulation must possess so as to accomplish the objectives set in target product profile as quantitative attributes are enlisted as target product quality profile (Lionberger et al. 2008). The International conference of harmonization (ICH) Q8 (R2) recapitulates them as QTPP (ICH Q8 (R2) 2009). The QTPP should endow a quantitative surrogate to ascertain the features of clinical safety and efficacy. Thus the pillar for identifying critical quality attributes (CQAs), critical process parameters and control strategy is ought to be QTPP. QTPP for INH site specific extended drug delivery is depicted in Table 1.
Risk assessment by FMEA
The concept and spurts of quality risk management was introduced in ICH Q9, 2005 guidance (ICH Q9, 2005). The CQAs depend on dosage form designed; type of formulation, manufacturing method, etc. employed and is selected amongst many possible options. Thus based upon feasibility studies, we defined the formulation and manufacturing method as described in following below sections. An overall risk assessment of the formulation/process variables was executed using FMEA method. By this method, the failure modes were identified that could have highest impact on product performance and greatest chance of eliciting product failure. FMEA method facilitates in prioritizing failure modes for risk management purposes according to the seriousness of their consequences (effects), how frequently they occur and how easily they can be detected. The relative risk that each formulation/process variable presents was ranked according to risk priority number (RPN) (Vora et al. 2013b).
Those attributes that could have a major impact on the drug product attributes needed to be studied in detail whereas those attributes that had minor impact on the drug product attributes required no further investigation. Table 2 portrays the FMEA for INH site specific extended release tablet with their respective RPN for each failure mode. The RPN was calculated with Eq. 1 mentioned as below:
where O is the occurrence probability or the likelihood of an event to occur; we ranked these as 5, frequent; 4, probable; 3, occasional; 2, remote and 1, improbable to occur. The next parameter S is the severity, which is a measure of how severe of an effect a given failure mode would cause; we ranked these as 5, catastrophic; 4, critical; 3, serious; 2, minor and 1, negligible or no effect. The final parameter D is the detectability which means the ease that a failure mode can be detected. Thus the more detectable a failure mode is, the less risk it presents to product quality. For D, we ranked 1, absolute certain or easily detectable; 2, high detectable; 3, moderately detectable; 4, low or remote detectable and 5 as hard to detect or absolute uncertain.
Preparation of INH sustained release core tablets
Some preliminary studies were carried out before selecting method of granulation, appropriate binder concentrations and polymer proportions. Only the formulations and studies pertinent for the present investigation are presented here. The tablets were prepared by wet granulation method. Briefly, accurately weighed quantity of INH (75 mg/tab), MCC PH 101, talc (as an anti-static agent), colloidal silicon dioxide and binder, ethyl cellulose (EC) were sifted through sieve ASTM # 30 (Wire metal GMP products, Mumbai, India) and physically mixed for 10 min. The premix was wet granulated using isopropyl alcohol (IPA) and dried in oven at 55 °C (Shree Kailash Industries, India). To the dried granules; MCC PH 102, Polyox WSR 303 (previously sifted through sieve ASTM # 30) and BHT (previously sifted through ASTM sieve 60 #) were added and blended for 10 min. Then colloidal silicon dioxide (as glidant, previously sifted through ASTM sieve 60 #) was added and blended for 10 min. Finally, magnesium stearate (as lubricant, previously sifted through ASTM sieve 60 #) was added and blended for 3 min. The homogeneous blend was compressed on an eight station automatic rotary tablet machine (Modern Engineering Works, New Delhi, India) equipped with shallow concave punches of 7.0 mm diameter to a target weight of 150 mg/tab. The dried granules of the optimized batch was subjected to downsizing in two different sizes range viz. through ASTM 18/24#, and 24/30# respectively and investigated for their effects on drug release. The granules to fine ratio were kept constant (70:30) in all the batches.
Seal coating on optimized tablet
Seal coat on core tablets of optimized batch was carried out with 8 % (w/w) aqueous dispersion of HPMC E5 and PEG 400 (82:18) using perforated coating pan apparatus (Solace Engineers (Mktg) Pvt. Ltd., Vadodara, Gujarat) to achieve 3 % weight gain. The process conditions were pre-warming of the tablets at 40 °C for 10 min; spray nozzle diameter, 1 mm; atomizing air pressure, 1–1.2 bar; inlet air temperature, 50–55 °C; product temperature 38–42 °C; spray rate, 5–8 g/min; pan rpm 6–8, post-drying at 40 °C for 30 min. The coating was performed to achieve weight gain of 3 % w/w.
Enteric coating on seal coated tablet
Enteric coating of tablets was done using perforated coating pan apparatus [Solace Engineers (Mktg) Pvt. Ltd., Vadodara, Gujarat] machine using aqueous dispersion of Eudragit L-100-55. To the aqueous dispersion, triethyl citrate as plasticizer (15 % w/w of dry polymer) and talc as anti-tacking agent (25 % w/w of dry polymer) previously homogenized were added and mixed for about 30 min. Final suspension was diluted with purified water under stirring to obtain 15 % w/v aqueous dispersion. Finally, the suspension was passed through ASTM 60# sieve and further used. The process conditions were pre warming of the tablets at 40° C temperature for 10 min, inlet air temperature (40–50 °C), bed temperature (29–31 °C) atomizing air pressure (1–1.2 bar), rotating speed of pan (7–10 rpm) and spray rate (4–6 g/min). After finishing of the coating, tablets were kept in the pan at 40° C for curing up to 30 min. The coating was performed to achieve three different weight gains viz. 8, 10 and 12 % w/w of average tablet weight.
23 Full factorial design for optimization of core tablet
A 23 factorial design with three factors, two levels, and eight runs was selected for the optimization study independent and dependent variables with their constraints are listed in Table 3. Percent drug release in phosphate buffer pH 6.8 at first hour (Q3), second hour (Q4), fourth hour (Q6) and eighth hour (Q10) were selected as dependant variables. For predicting the optimal region, the linear polynomial equation generated for the variables was explained as follows (Eq. 2):
where Y is the predicted response, β 0 is model constant/coefficient, βi is the linear regression coefficient, βij is the interaction effect regression coefficient and Xi is the dimensionless coded value of the independent variables (Xi). All statistical treatments of design of experiment were performed using Design Expert software (ver. 8.0.7.1., Stat- Ease Inc., USA) Main effect plots, interaction plots and overlaid contour plots were generated using Minitab software (ver. 16.2.1., Minitab Inc., USA). All experimental runs were randomized to exclude any bias. Further the model was evaluated for best fit using parameters, coefficient of determination (r 2), adjusted r 2 (Adj-r 2), predicted r2 (Pred-r 2), adequate precision (Shah et al. 2008) and Q 2 (Singh et al. 2005).
Physical characterization of the tablets
The compressed core tablets were subjected to various physical investigations like appearance, weight variation, hardness, friability and drug content. The weight variation was carried out on 20 tablets using electronic balance (Shimadzu AX 120, Japan). Tablet hardness was determined using minimum six tablets for each batch with dial type tablet hardness tester (Scientific Engineering Corporation, Delhi, India) respectively. Friability was determined by Roche Friabilator for 4 min at 25 rpm. Coated tablet was subjected to investigations like appearance, percentage weight gain, loss on drying and drug content. A validated RP-HPLC (Shimadzu, Kyoto, Japan) method was used for drug content measurement in triplicate. The method was modified as described by Shah et al. (1992) and validated for drug content determination. Analysis was performed on Phenomenex C18 column (250 mm × 4.6 i.d., 5 micron) at 1.0 ml/min flow rate with 75:25 % v/v mixture of methanol: 0.02 M disodium hydrogen phosphate pH 4.5 buffer as mobile phase at 254 nm. Linearity was demonstrated in range of 5–50 μg/ml. The recovery data was in the range of 98–102 %. The RSD for the precision was below 2 %. Percentage weight gain was computed using following equation.
where Wta is the weight of tablet after coating and Wtb is the weight of tablet before coating (Patel et al. 2010).
In vitro drug release
The study was performed using United States Pharmacopeia (USP) 30 type II apparatus (VDA 6-DR, Veego Instruments Corporation, Mumbai, India) using Method B for delayed release products as specified in USP for enteric coated tablets (USP 30/NF 25 2007a). Six dosage units were analyzed for dissolution profiling and data were recorded at 1, 2, 3, 4, 6, 8, and 10 h. For optimization of core tablets dissolution was performed in pH 6.8 phosphate buffer. Samples withdrawn were filtered through a 0.45 µm membrane filter and then analyzed immediately for drug release. The drug released in acidic medium was analyzed as per method specified in USP (USP30/NF25 2007b) and released in pH 6.8 phosphate buffer was measured by HPLC method as described in above section.
Desirability function
The desirability function is an excellent tool to merge multicriteria responses in one single criterion measurement. The information obtained from it can be useful for predicting optimum levels of individual variables. If the value of the response is on target or is at optimum, its desirability value was allocated as 1 and for totally unacceptable value its desirability was given as 0. The individual desirability for each response was calculated (Shah et al. 2008) using the approaches discussed below.
Q3 was desired to be the maximum so as to achieve initial burst release of INH. But here target was specified for Q3 as it was anticipated that more of the initial release might not prolong drug release up to 8 h. Desirability d1 for response Q3 was calculated by Eq. 3:
Yi is the experimental result, and Ymin and Ymax represent the minimum and maximum possible values. Ymax, Ymin and Ytarget for this response were 33.16, 24.48 and 26.0 % drug release respectively.
For Q4 (d2) and Q6 (d3) there were no specific requirements for either obtaining maximum or minimum value. Q4 and Q6 response justifies that the drug releases in sustain manner from the dosage form. For Q4 and Q6; the formulations having percentage release within the constraint range selected (Table 3) was considered as optimum having desirability of 1, while formulations having values out of this range have a desirability of 0. This can be explained by below Eq. 4:
However for Q10 more than 90 % drug should be release to ascertain complete release from the dosage forms. Ymax and Ymin for this response were 98.9 and 95.8 % drug release respectively. Thus d4 for Q10 was calculated by formula shown in Eq. 5.
The overall desirability was calculated from the individual values by using the following Eq. 6.
Curve fitting and release mechanism
In order to study the drug release mechanism from the formulations, various models were considered to fit the experimental data using Microsoft Excel based DD solver software (Microsoft, USA) to execute and evaluate dissolution data modeling. The in vitro release pattern was evaluated to check the goodness of fit to the zero order (Costa and Sousa Lobo 2001), first order (Wagner 1969), Higuchi (Higuchi 1963), Baker (Baker and Lonsdale 1974), Hopfenberg (Costa and Sousa Lobo 2001; Katzhendler et al. 1997), Hixson–Crowell’s (Hixson and Crowell 1931), Weibull (Sathe et al. 1996) and Korsmeyer–Peppas power law equation (Korsmeyer et al. 1983; Ritger and Peppas 1987). For Korsmeyer–Peppas model, data were analyzed for first 60 % of the drug release. Importantly, the goodness of fit was evaluated using adjusted r2 (correlation coefficient) values. This is for the reason that r2 will always increase as more parameters are included, whereas r2 adjusted may decrease when over fitting has occurred (Zhang et al. 2010). The Akaike information criterion (AIC) and Model selection criteria (MSC) criteria were also applied as described elsewhere (Akaike 1974; Zhang et al. 2010; Vora et al. 2013b).
In order to understand the drug release mechanism, the release data of the optimized batch (ES 5) was fitted to empirical equations proposed by Kopcha (Kopcha et al. 1991).
In the above equations, M (≤70 %) is the percentage of drug released at time t, while A and B are, respectively, diffusion and erosion terms. According to this equation, if diffusion and erosion ratio, A/B = 1, then the release mechanism includes both diffusion and erosion equally. If A/B > 1, then diffusion prevails, while for A/B < 1, erosion predominates (Ratsimbazafy et al. 1996). Additionally, the data were also fitted into Peppas–Sahlin model to understand drug release mechanism (Peppas and Sahlin 1989; Grassi and Grassi 2005).
Capability analysis
Capability analysis is used to assess whether a process is capable of producing output that meets your desired quality traits. A capable process is able to produce products that meet desired specifications. The process here was assumed to be in statistical control. The normal probability plot was used to examine normal distribution of data (Bissell 1994). Additionally, Anderson–Darling, Ryan–Joiner and Kolmogorov–Smirnov test statistic at 5 % significance level were applied to assess whether data follows a normal distribution or not. Capability analysis was performed on five reproducibility batches (n = 30) using Minitab software (ver. 16.2.1., Minitab Inc., USA).Cp, CPU, CPL and Cpk were computed for potential within capability and Pp, PPU, PPL and Ppk for overall capability respectively (Rudisill and Litteral 2008; Shinde and Katikar 2012). The 3–σ standard deviation variation was everywhere considered for relating process spread to specification spread.
Packaging and stability study
The optimized batch was subjected to short term stability testing according to the ICH guidelines for zones III and IV (ICH Q1A(R2) 2003). Tablets were packed in count of 30 into high density polyethylene bottle with child resistant cap and were further induction sealed. Before induction sealed one silica bag was kept in bottle as desiccant. The sealed bottles were exposed to accelerated (40 ± 2 °C/75 ± 5 % relative humidity) and long term (25 ± 2 °C/60 ± 5 % relative humidity) stability for three months. The samples were withdrawn periodically (0, 15, 30, 60 and 90 days) and evaluated for different physicochemical parameters like visual inspection, drug content, gastric resistance and in vitro drug release.
Results and discussion
QTPP of INH site specific delivery
Laying down QTPP depends upon formulation type and process chosen (Yu 2008; Lionberger et al. 2008). Based on preliminary trials undertaken, the parameters that will be focused in our study were selected and enlisted as QTPP for INH site specific delivery (Table 1). Thus, except recitation of our QTPP, the further steps to describe the QTPP are not discussed. The said QTPP will lay down the foundation for determining CQA.
Risk assessment by FMEA
The factors that were embarked and assessed by FMEA in development of INH site specific delivery are highlighted in Table 3. In the current approach for development, the factors that exhibited RPN ≥ 40 was considered as high risk, ≥20 to <40 was considered as medium risk and <20 was considered as low risk (Vora et al. 2013b). From Table 2, it is clearly stipulated that amount of Polyox WSR 303, hardness, amount of EC and enteric coating have RPN ≥ 40 and require through investigation and optimization. Thus, the optimization of three main factors that affect the core tablet formulation i.e. amount of Polyox WSR 303, hardness and amount of EC was done statistically using 23 full factorial design for establishing design space. The enteric coating RPN also falls under high risk category and its optimization is discussed in its respective section. Granule sizes and packaging RPN fall under moderate risk category and are also discussed in their respective sections.
Physical evaluation of tablets
Physical appearance, friability, weight variation and assay of all the formulations of core tablets were found to be satisfactory. Hardness was studied in two ranges 3–5 Kp and 6–9 Kp. Friability of the core tablets was found to be less than 0.5 % (w/w). The coated tablet appearance was found to be satisfactory. Weight gain of seal coated tablets was checked by weighing different sets of 20 tablets three times and results were found to be comparable with low standard deviation (SD < 5 % of weight gain) while loss on drying was found to be 0.9 % considering initial pre-warmed uncoated tablet weight and loss on drying. Similar calculations were carried out for enteric coated tablet and results were found with low standard deviation (SD < 5 % of weight gain) and loss on drying of 1.1 %.
Effect of factors on the responses
Q3
Results of the measured response for 23 full factorial design are displayed in Table 4. Regression coefficients and ANOVA results of response variables are shown in Table 5. From the results, it can be concluded that hardness was the most influencing factor affecting negatively (Table 5) in initial burst release to achieve desired MIC. The same can be inferred from the Pareto chart, half normal plot and main effect plots for Q1 (Figs. 1a, 2a) respectively. The reason may be the higher hardness increased the bonding strength of the tablet which would have decreased the porosity and increased the tortuosity factor of the matrix which is responsible for its negative impact on drug release.
Moreover, the initial higher release was observed in all batches (Table 4). Looking deeply inside, it can be anticipated that in the beginning, drug close to surface of matrix might be released before the surrounding polymer reached the polymer disentanglement concentration. In addition, for water soluble drugs diffusional driving force would be highest and mean dissolution rates close to the mean water infiltration rates (Tahara et al. 1996). Also for high viscosity polymer like Polyox WSR 303, it would take a longer time to form a gel layer which provides enough time for initial burst release. Similar mechanisms have been reported in literature for initial high release of water soluble drug from matrix monolithic system (Barakat et al. 2008). Now interestingly, interaction effect was also observed between amount of Polyox WSR 303 and amount of EC which affected positively (Table 5; Fig. 1a). The same can be inferred from interaction plot (Fig. 3a). The quadratic equation for reduced model in coded units is as below:
Q4
From Table 5; Figs. 1b and 2b, it can be concluded that amount of Polyox WSR 303 and hardness were the most influencing factor affecting Q4 negatively (negative co-efficient; Table 5) to maintain the sustain release. The reason for factor A to retard drug release might be due to greater chain entanglement produced by high viscosity polymers, ultimately resulting in thicker gel layer after hydration of it. Furthermore, on formation of gel by high viscosity polymers, it is difficult for longer chains to dissolve since high energy is required for pulling them off the matrix. Similar mechanism have been proposed for high viscosity polymers for sustained drug release by some researchers (Hiremath and Saha 2008; Colombo et al. 2000). Thus, the longer diffusional path length created by gel layer resulted in the decreased effective diffusion of the drug and therefore a reduction in the drug release rate. The mechanism of hardness for decreasing drug release has been already discussed. The quadratic equation for reduced model in coded units is as below:
Q6
As shown in Fig. 1c, 2c and Table 5, it can be concluded that hardness was the most influencing factor affecting Q6 negatively. The batches (e.g. ES 5, 4 and 1) in which hardness was at higher level could sustain drug release in desired constraints. On the contrary, batches (e.g. ES 8, 3 and 6) in which hardness was at low level released more than 75 % drug. Interestingly, interaction effect was observed between AC and BC (Table 5) on percent drug release at Q6 time point. The same can be inferred from Pareto chart, half normal probability ploy (Fig. 1c) and p value of ANOVA table (Table 5). The results obtained surprisingly depict that there is no pronounced decrease in drug release on increasing binder EC concentration (Table 5) similar to results of Q4. The reason might be EC was added as dry binder in the premix. Then IPA was added to granulate the mass. In case of EC it is hypothesized that it dissolved on addition on IPA and it exhibits coating like effect. Here, addition of IPA was stopped on achieving end point in granulation. Thus it is hypothesized that quantity of IPA was not sufficient to dissolve dry binder in the premix to exhibit more hydrophobic coating like effect and as a result much binder remained in the dry state. As a result, there might not be significant difference in providing hydrophobic interaction as compared to lower binder concentrations. Secondary reason assumed may be high solubility of the drug and drug loading. Hence, due to early achieving end point in granulation with less consumption of IPA, together with high solubility of drug there is not much significant decrease in drug release on increasing binder concentration. The exact mechanism yet needs to be investigated.
The quadratic equation for reduced model in coded units is as below:
Q10
For Q10, more than 90 % drug release is desired to ensure complete drug release from developed formulation. As highlighted in Fig. 1d and p value from ANOVA table (Table 5), it is clearly stipulated that none factor neither interaction had influenced on percent drug release at Q10. All the batches showed more than 90 % drug release at Q10. The reason may be due to high solubility of drug and drug loading. Another factor is threshold level of retardation of drug release rate by polymer as drug release does not result solely from polymer erosion, but also on drug diffusion through the hydrated polymer layers.
The quadratic equation for model in coded units is as below:
Model fitting and statistics of the measured responses
Here, higher values of r2 for all dependent variables were found which statistically signify a good fit. Additionally, Adj-r2 and Pred-r2 values were also in reasonable agreement signifying good model fit (Table 6). Further model showed the adequate precision value greater than 4, indicating adequate model discrimination (Shah et al. 2008). A model fit value of Q2 > 0.5 is considered as fairly good and value of Q2 > 0.9 is generally taken as excellent (Singh et al. 2005). Q2 values for all the measured responses were good signifying good model fit.
Evaluation of model using cross-validation
The reliability of the model was assessed by conducting five experiments by varying the formulation variables at values other than that of the model. The experimental and predicted values for each response are shown in Table 7. Bias or percent relative error between predicted and experimental values for each response was calculated by the following Eq. (12).
Results from Table 7 reveal reasonable agreement between the predicted and the experimental value in all the five batches, due to low value of the bias was found. Thus it can be concluded that the equations express satisfactorily the influence of the chosen formulation variables on the responses under study.
Optimization using desirability function
Desirability function was calculated for percent drug release at Q3, Q4, Q6 and Q10 time. Based on the composite desirability data and overlay contour plots (Fig. 4), ES 5 was identified as the optimum batch having desirability of 0.91. Composite desirability found out for optimized batch with the help of Minitab software was 0.90. The weight and importance was allotted 1 for each response respectively.
Curve fitting and release mechanism
Values of adjusted r2, AIC and MSC value are presented in Table 8. The drug release data of the optimized batch ES 5 shows a good fit to the Korsmeyer–Peppas power law equation which can be confirmed by comparing the values of adjusted r2 with that of the other models. The values of release exponent (n) determined for the optimized formulation batch ES 5 was found to be 0.592 suggesting the probable release by anomalous transport (Ritger and Peppas 1987). The lowest AIC value; 13.3878, of optimized batch ES 5 indicates that Korsemeyer–Peppas power law was the best fit model in describing the dissolution behavior. Similarly, the highest MSC, 5.4208, of the optimized batch ES 5 indicates the same.
To investigate the underlying release mechanism, Kopcha model was applied. The A/B ratio for drug release was found to be 4.46 which is greater than 1, showing predominance of diffusion relative to erosion. The value of constants k1 (26.575) and k2 (2.966) of Peppas–Sahlin model are displayed in Table 8. k1 denotes relative contribution of drug diffusion to drug release and k2 denotes relative contribution of polymer relaxation to drug release. From Table 8, it is clearly stipulated that diffusion is the predominant mechanism for drug release. Thus data are in agreement with Kopcha model revealing diffusion as predominant mechanism which may be due to high solubility of the drug.
Effects of granule size
The granules of optimized batch ES 5 were subjected to downsizing in two particle sizes range viz. ASTM 18/24#, and 24/30# to investigate its effects on percent drug release. Results revealed that there is no statistically significant difference in drug release for two different sizes of granules (p > 0.05). The reason may be high water solubility of the drug together with its high percent drug loading. Probably, the high diffusion co-efficient of highly water soluble drug may have quash the effect of granule size. Hence, risk and criticality of this failure mode is low.
Effect of enteric coating
Here target was set to be zero percentage of drug release in acidic medium as it was anticipated that even small amount of INH release in acidic medium will augment the degradation of RIF.
Enteric coating of 10 % w/w was found to be optimum to achieve zero percentage of drug release in 0.1 N hydrochloric acid and similar dissolution profile as of core tablet in pH 6.8 phosphate buffer (Fig. 5). Weight gain of 8 % w/w provided similar dissolution profile as that of core tablet in phosphate buffer pH 6.8 but simultaneously drug release of 2.3 % (mean value) was obtained in acidic medium. On the contrary, 12 % w/w provided zero percentage drug release in acidic medium but slow down dissolution profile of the core tablet during initial hour in pH 6.8 phosphate buffer (Fig. 5).
Capability analysis
The probability plots result for detecting normality of distribution are depicted in Table 9 for Q3, Q4, Q6 and Q10 respectively together with p values of Anderson–Darling test, Ryan–Joiner (similar to Shapiro–Wilk test) and Kolmogorov–Smirnov test. The p values of all the three tests were greater than 0.5 indicating normal distribution of the data at 5 % significance level. Hence, capability analysis with normal distribution was undertaken.
Results of the various indices of capability analysis for Q3, Q4, Q6 and Q10 are displayed in Table 9. For a process to be capable to produce batches within specifications, all the indices value should be above 1.33 (Rudisill and Litteral 2008; Kane 1986). From the results of Table 9, it can be inferred that all the indices value were above 1.33 which indicates that the process passes the capability analysis at 3–σ standard deviation process spread and the process is capable of producing batches that conform to specifications. Therefore, the measurements are located within specification limits for Q3, Q4, Q6 and Q10.
For Q4, Cp is 2.10, which indicates that the specification spread is 2.10 times greater than the 3–σ spread in the process. Moreover Cp (2.10) and Cpk (2.05) are very close to one another, revealing that the process is centered. Regarding overall capability, Pp value is 2.13 indicating 2.13 times greater than the 3–σ spread in the process. Also Pp (2.13), Ppk (2.07) and Cpm (2.12) are very close to another, indicating that the process is centered on the target. Furthermore, the within and overall capability indices are very close to each other indicating process is within the control. For Q3 and Q6, Cp and Cpk are not as close to each other as compare to Q3 signifying the process is slight deviating from the center (Table 9). Nevertheless, all the indices of within and overall were above 1.33 (Table 9) and within desired constraints (Table 3) signifying process passes capability analysis at 3–σ standard deviation process spread. Regarding Q10, there is disparity between Cp (3.25) and Cpk (1.41), which is due to more than 95 % drug release in all the batches which was desired. The same can be inferred from CPL (5.10) value. Similar conclusions can be drawn for overall indices. Furthermore, all the indices were above 1.33 (Table 9) revealing process passes capability indices.
Packaging and stability studies
The optimized formulation ES 5 showed negligible change under the conditions of storage for parameters like appearance, drug content, gastric resistance and in vitro drug release. The similarity factor (f2) (Costa and Sousa Lobo 2001) was employed for comparison of dissolution profiles on each time point. It ranged from 83 to 92. Thus the data suggested that the formulation was stable for under the packaging material selected revealing that it risks it under control and low.
Risk mitigation and control strategy
23 full factorial design was employed to examine the multidimensional interaction of input variables of the core tablet which were ranked as high risk in the initial risk assessment for establishment of a design space. The acceptable region within which a quality of the product can be constructed is called as design space (Lionberger et al. 2008; Yu 2008). The risk mitigation and control strategy is fused outline of how quality is established based on current process and existing product knowledge.
For factor C, it can be inferred from main effect plots (Fig. 2a–d), overlay contour plots (Fig. 4) and p value from ANOVA (Table 5) that it did not significantly affected any of the dependant variables (p value >0.05) as main effect but showed interaction effects with other factors on some of the responses (Fig. 3a–d). Using software, we modulated the range of C by changing its setting level and observed the change in overlay contour plot of A versus B.
The range of C where region of A versus B in overlay contour plot was found to be maximum was selected as range of C. We found range of C of −0.3 to 0.6 in coded units as optimum range and thus we decided to use EC in that range. The risk with operating in this range is low. The risk mitigation strategy is to monitor the dissolution within desired constraints range.
From the ANOVA table and p value (Table 5), overlay contour plots (Fig. 1) and main effect plots (Fig. 2a–d) it is clearly observed that factor A have major impact on percent drug release at Q3 and Q4 and B on Q3, Q4 and Q6. Thus there is an optimum range for A and B where you can get the desired drug release in the set constraint range which is specified in overlay contour plot (Fig. 4) of B and C versus all four responses. Working in this zone, risk is low as all the responses will ascertain to be in the constraint range. The risk mitigation strategy for the same is to monitor percent drug release at Q3, Q4, Q6 and Q10 are in the constraint range.
The enteric coating was also in the high risk category which was optimized as discussed. Regarding moderate RPN failure modes, granule size and packaging were discussed in their respective sections. Figure 6 expresses the FMEA analysis before and after the execution of the control strategy. It was found that RPN of all the possible failure modes were below 20; making them to fall under the low risk category. The scalability can be further evaluated from subsequent transfer from lab to pilot and then scale up batch manufacturing. Thus it may be further cultured based on supplementary experience gained during the commercial lifecycle of the production.
Conclusion
There is no ambiguity that several initiatives are undertaken worldwide to circumvent development hiccups of anti-TB formulations. The formulation technology used here is simple, easily scalable and adopted in industries. Hence, it endows to be of greater interest especially in under developed or developing countries to epitomize the objectives like cost-effectiveness, feasibility and save resources. The manuscript describes the overall QbD approach along with risk assessment, risk analysis and control strategy to mitigate the risk for development of INH site specific sustained drug delivery. In an endeavor to accomplish the objectives of QbD, 23 full factorial design was employed for evaluating the failure modes with high RPN number of core tablet and defining the relationships between input variables and quality traits desired. The optimized formulation exhibited percent release at Q3 of 26.97 %, Q4 of 44.20 %, Q6 of 66.15 %, Q10 of 97.9 % and gastric resistance less than 10 %. Finally, the design space was established and control strategy was developed to mitigate the risk in future. The RPN of updated risk assessment represents that all the failure modes of FMEA analysis were in low risk category (Fig. 6). Finally, capability indices were performed on five reproducibility batches and results revealed that all indices were above 1.33 indicating process was significantly under control.
Thus the shift in exemplar from traditional approach to QbD approach can provide incisive insight for building quality within the product. Hence, the developed formulation may provide prudently a better substitute for conventional tablet in circumventing its hiccups; improve biopharmaceutical properties, providing biphasic release and may anticipate a better bioavailability. The developed formulation has shown promising results in vitro and is potential for assessing in vivo bioavailability. The further in vivo investigations in suitable animal models and human clinical trials are required to prove the clinical usability of the experimental tailored release formulation.
References
Akaike H (1974) A new look at the statistical model identification. IEEE Trans Autom Control 9:716–723
Baker RW, Lonsdale HS (1974) Controlled release: mechanisms and rates. In: Taquary AC, Lacey RE (eds) Controlled release of biologically active agents. Plenum Press, New York, pp 15–71
Barakat NS, Elbagory IM, Almurshedi AS (2008) Controlled release carbamazepine granules and tablets comprising lipophilic and hydrophilic matrix components. AAPS PharmSciTech 9:1054–1062
Batyrbekov EO, Rukhina LB, Zhubanov BA, Bekmukhamedova NF, Smailova GA (1997) Drug delivery systems for tuberculosis treatment. Polym Int 43:317–320
Bhatt DD, Trivedi MR, Modi IA, Khamar BF (2011) Pharmaceutical composition of INH. WO2011012987
Bissell D (1994) Statistical methods for SPC and TQM. Chapman and Hall, London
Burman WJ, Gallicano K, Peloquin C (2001) Comparative pharmacokinetics and pharmacodynamics of the rifamycin antibacterials. Clin Pharmacokinet 40:327–341
Colombo P, Bettini R, Santi P, Peppas NA (2000) Swellable matrices for controlled drug delivery: gel-layer behaviour, mechanisms and optimal performance. Pharm Sci Technol Today 3:198–204
Costa P, Sousa Lobo JM (2001) Modeling and comparison of dissolution profiles. Eur J Pharm Sci 13:123–133
du Toit LC, Pillay V, Danckwerts MP (2006) Tuberculosis chemotherapy: current drug delivery approaches. Resp Res 7:118
Ellard GA, Gammon PT, Laksminarayan S, Fox W, Aber VR, Mitchison DA, Citron KM, Tall R (1972) Pharmacology of some slow release preparations of isoniazid of potential use in intermittent treatment of tuberculosis. Lancet 1:340–343
Gohel MC, Sarvaiya KG (2007) A novel solid dosage form of rifampicin and isoniazid with improved functionality. AAPS PharmSciTech 8:E1–E7
Grassi M, Grassi G (2005) Mathematical modelling and controlled drug delivery: matrix systems. Curr Drug Deliv 2:97–116
Higuchi T (1963) Mechanism of sustained action medication. Theoretical analysis of rate of release of solid drugs dispersed in solid matrices. J Pharm Sci 52:1145–1149
Hiremath PS, Saha RN (2008) Controlled release hydrophilic matrix tablet formulations of isoniazid: design and in vitro studies. AAPS PharmSciTech 9:1171–1178
Hixson AW, Crowell JH (1931) Dependence of reaction velocity upon surface and agitation. Ind Eng Chem 23:923–931
ICH Harmonized Tripartite Guideline (2003) Stability testing of new drug substances and products Q1A(R2), February 2003
ICH Harmonized Tripartite Guideline (2009) Pharmaceutical development Q8 (R2), August 2009
ICH Harmonized Tripartite Guideline. Quality risk management Q9, November 2005
Iseman MD, Madsen LA (1989) Drug-resistant tuberculosis. Clin Chest Med 10:341–353
Kane VE (1986) Process capability indices. J Qual Technol 18:41–52
Katzhendler I, Hoffman A, Goldberger A, Friedman M (1997) Modeling of drug release from erodible tablets. J Pharm Sci 86:110–115
Katzung BG (2001) Basic and clinical pharmacology, 8th edn. McGraw-Hill, New York
Kopcha M, Lordi N, Tojo KJ (1991) Evaluation of release from selected thermosoftening vehicles. J Pharm Pharmacol 43:382–387
Korsmeyer RW, Gurny R, Doelker E, Buri P, Peppas NA (1983) Mechanisms of solute release from porous hydrophilic polymers. Int J Pharm 15:25–35
Lionberger RA, Lee SL, Lee L, Raw A, Yu LX (2008) Quality by design: concepts for ANDAs. AAPS J 10:268–276
Mariappan TT, Singh S (2003) Regional gastrointestinal permeability of rifampicin and isoniazid (alone and their combination) in the rat. Int J Tuberc Lung Dis 7:797–803
Mitchison DA (1985) Mechanism of drug action in short-course chemotherapy. Bull Int Union Tuberc 65:30–37
Patel SR, Patel PR, Vora CN, Patel ND, Patel JK (2010) Formulation, process parameter optimization and evaluation of delayed release tablets of rabeprazole sodium. Int J Pharm Pharm Sci 2:144–156
Peppas NA, Sahlin JJ (1989) A simple equation for the description of solute release III. Coupling of diffusion and relaxation. Int J Pharm 57:169–172
Pund S, Joshi A, Vasu K, Nivsarkar M, Shishoo C (2010) Multivariate optimization of formulation and process variables influencing physico-mechanical characteristics of site-specific release isoniazid pellets. Int J Pharm 388:64–72
Ratsimbazafy V, Bourret E, Brossard C (1996) Drug release from matrix tablets and minitablets containing glycerides. Pharm Ind 58:442–446
Ritger PL, Peppas NA (1987) A simple equation for description of solute release II. Fickian and anomalous release from swellable devices. J Control Release 5:37–42
Rudisill F, Litteral LA (2008) Capability ratios: Comparison and interpretation of short-term and overall indices. Int J Qual Stand 2(1), Paper 3, 67–86. http://www.bsieducation.org/Education/downloads/ijqs/paper19.pdf. Accessed 18 Nov 2013
Sathe PM, Tsong Y, Shah VP (1996) In vitro dissolution profile comparison: statistics and analysis, model dependent approach. Pharm Res 13:1799–1803
Schaberg T, Rebhan K, Lode H (1996) Risk factors for side effects of isoniazid, rifampin and pyrazinamide in patients hospitalized for pulmonary tuberculosis. Eur Respir J 9:2026–2030
Shah Y, Khanna S, Jindal KC, Dighe VS (1992) Determination of rifampicin and isoniazid in pharmaceutical formulations by HPLC. Drug Dev Ind Pharm 18:1589–1596
Shah PP, Mashru RC, Rane YM, Thakkar A (2008) Design and optimization of mefloquine hydrochloride microparticles for bitter taste masking. AAPS PharmSciTech 9:377–389
Shinde JH, Katikar RS (2012) Importance of process capability and process performance indices in machine tool. Int J Res Eng Appl Sci 2:1211–1217
Shishoo CJ, Shah SA, Rathod IS, Savale SS, Vora MJ (2001) Impaired bioavailability of rifampicin in presence of isoniazid from fixed dose combination (FDC) formulation. Int J Pharm 228:53–67
Singh S, Mariappan TT, Sankar R, Sarda N, Singh B (2001) A critical review of the probable reasons for the poor/variable bioavailability of rifampicin from anti-tubercular fixed-dose combination (FDC) products, and the likely solutions to the problem. Int J Pharm 228:5–17
Singh B, Kumar R, Ahuja N (2005) Optimizing drug delivery systems using systematic “design of experiments”. Part I: fundamental aspects. Crit Rev Ther Drug Carrier Syst 22:27–105
Tahara K, Yamamoto K, Nishihata T (1996) Application of model-independent and model analysis for the investigation of effect of drug solubility on its release rate from hydroxypropyl methylcellulose sustained release tablets. Int J Pharm 133:17–27
US Pharmacopoeia 30/NF25 (2007a) Chapter 711: dissolution. US Pharmacopoeial Convention, Rockville
US Pharmacopoeia 30/NF25 (2007b) Isoniazid tablets monograph. US Pharmacopoeial Convention, Rockville
Vora C, Patadia R, Miattal K, Mashru R (2013a) Recent patents and advances on anti- tuberculosis drug delivery and formulations. Recent Pat Drug Deliv Formul 7:138–149
Vora C, Patadia R, Mittal K, Mashru R (2013b) Risk based approach for design and optimization of stomach specific delivery of rifampicin. Int J Pharm 455:169–181
Wagner JG (1969) Interpretation of percent dissolved time plots derived from in vitro testing of conventional tablets and capsules. J Pharm Sci 58:1253–1257
Yu LX (2008) Pharmaceutical quality by design: product and process development, understanding, and control. Pharm Res 25:781–791
Zhang Y, Huo M, Zhou J, Zou A, Li W, Yao C, Xie S (2010) DDSolver: an add in program for modeling and comparison of drug dissolution profiles. AAPS J 12:263–271
Acknowledgments
This article does not contain any studies with human and animal subjects performed by any of the authors. All authors (C. Vora, R. Patatia, K. Mittal, R. Mashru) declare that they have no conflict of interest. Mr. Chintan Vora is highly grateful to the University Grants Commission, New Delhi, Government of India, for availing Junior Research Fellowship. The authors are grateful to the industries that provided respective gift samples.
Author information
Authors and Affiliations
Corresponding author
Rights and permissions
About this article
Cite this article
Vora, C., Patadia, R., Mittal, K. et al. Risk based approach for design and optimization of site specific delivery of isoniazid. Journal of Pharmaceutical Investigation 45, 249–264 (2015). https://doi.org/10.1007/s40005-014-0170-z
Received:
Accepted:
Published:
Issue Date:
DOI: https://doi.org/10.1007/s40005-014-0170-z