Abstract
Drought is a complex phenomenon that strong indices should be used to quantifying it. Reconnaissance drought index (RDI) is very strong index that extensively used in drought investigating researches. Major problem to calculate this index especially in arid and semi-arid regions at undeveloped countries is lack of data for calculating evapotranspiration. This study investigated this problem’s solution via simulating monthly RDI using other indices (PN, DI, SPI, CZI, MCZI and Z-Score) that in order to calculate these indices, only precipitation data is used. To simulate RDI, Generalized Estimating Equations (GEE) was used. For validation of estimating equations, different indices of goodness of fit were used (NSE, RMSE, MAE, R2, QIC and QICC). Results of this study indicated that the SPI, CZI, MCZI and DI indices had the most appropriateness for simulating RDI. When the SPI index (the best index for simulating RDI) was used to simulate the RDI, according to the results of T-Test, the observed and simulated data series hadn’t significantly difference (P value >0.05) in all stations. The average values of NSE, R2, RMSE, MAE, QIC and QICC obtained 0.976, 0.139, 0.088, 0.976, 21.24 and 17.82 sequential.
Similar content being viewed by others
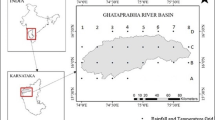
Avoid common mistakes on your manuscript.
1 Introduction
Powerful tools to monitor natural phenomena play a key role in coping with these phenomena. Drought, as one of the natural damaging phenomena, has affected people’s lives in many regions of the world (Zareiee 2014; Zarei et al. 2016a; Zarei and Mahmoudi 2017; Bennani et al. 2017; Ismail et al. 2017). Scientifically copping with this phenomenon, observed data monitoring should firstly have done using strong drought indices. Drought indices are important elements of drought monitoring and assessment since they simplify complex interrelationships between many climate and climate-related parameters. Reconnaissance Drought Index using data of two determinants, precipitation and potential evapotranspiration that proposed by Tsakiris (2004), is universal and comprehensive for assessment of drought severity. Morid et al. (2006) assessed and compared the some of the drought indices (seven indices) to consideration of drought in the Tehran province of Iran. The indices used include deciles index (DI), percent of normal (PN), standardized precipitation index (SPI), effective drought index (EDI), China-Z index (CZI), modified CZI (MCZI) and Z-Score. The comparison of indices is based on drought cases and classes that were detected in the province. The results show the ability of SPI and EDI to detect the onset of drought, its spatial and temporal variation consistently that the EDI was found to be more responsive to the emerging drought and performed better. Khalili et al. (2011) assessed the similarities and differences of the SPI and the reconnaissance drought index (RDI) drought indices (in 3-month, 6-month and 12- month time scales) SPI and RDI time in Iran using Markov chain. Results showed mentioned indices had an overall similar behavior to assess drought conditions. Banimahd and Khalili (2013) compared the behavioral aspects of EDI, SPI, RDI and standardized precipitation evapotranspiration index (SPEI) to assess drought conditions. Results of this study indicated that except for the EDI, all indices/cases (all climatic zones) showed significant correlation and in all climatic zones, the EDI values for wet (dry) periods were higher (lower), compared to other indices. Mashari Eshghabad et al. (2014), investigates the performance of six meteorological drought indices in 1-month, 3-month, 6-month, 9-month, 12- month, 24- month and 48-month time scales in the Tajan basin in Iran. The results showed that in 12-month time scale DPI, in 1-month, 6-month and 24-month time scale PNPI, in 9-month and 48-month time scale MCZI and in 3-month ZCI were the best indices. Jain et al. (2015), compared SPI, EDI, statistical Z-Score, China Z-Index (CZI), Rainfall Departure (RD), Rainfall Decile based on Drought Index (RDDI) for their suitability in drought prone districts of the Ken River Basin, in India. The results indicated that the drought indices are highly correlated at same time steps and can alternatively be used. Other different researches were carried out in the category of drought monitoring using different drought indices and comparison of these drought indices performances (Tsakiris et al. 2007; Mpelasoka et al. 2008; Raziei et al. 2009; Tabrizi et al. 2010; Zarei et al. 2016b; Khan et al. 2017; Kwarteng et al. 2017; Montaseri et al. 2017; Gabriele et al. 2017; Zarei 2018).
Due to the lack of data for calculating evapotranspiration, RDI index cannot be used in all areas. This study investigates the correlation between RDI and other indices (DI, PN, SPI, CZI, MCZI and Z-Score) in order to simulating RDI using these indices. Data are from stations in South of Iran.
2 Materials and Methods
2.1 Study Area and Relevant Data
Figure 1 shows study area boundaries enclosing a 594,996.54 km2 area between 25°17’N and 31°11’N latitudes and 50°49′E and 62°20′E longitudes. In this research for drought modeling and forecasting studies, spatially and temporally data series of 16 synoptic stations with suitable spatial distribution and adequate time duration of meteorological data (31 years from 1980 to 2010) was used. The central and northern areas are highlands and mountains, while the southern and western areas are mainly flat. The climate of this area is hyper-arid, arid, and semi-arid, with warm summers and cold winters. The general characteristics of the 16 surveyed stations, such as elevation, latitude, longitude, the average precipitation, and the climate zone status with respect to the aridity index is shown in Table 1.
2.2 Drought Indices
Seven drought indices as the most widely used indices selected for use in this research. They include the PN, DI, SPI, CZI, MCZI, the Z-Score and RDI indices. Using the only precipitation data, all of the selected indices calculated with the exception of RDI that used the evapotranspiration data in addition to precipitation data. All considered indices with a time step of 1 month applied to time series in this study. Following text presented a brief description of these indices.
2.2.1 PN Drought Index
PN drought index is one of the simple drought indices that is only based on precipitation parameter (Smakhtin and Hughes 2004). This index is based on the ratio of the amount of precipitation to the average of the amount of precipitation in the long-term for each region and each time scales. Based on PN index, drought severities classified in 5 classes that are presented in Table 2.
2.2.2 DI Drought Index
At first stage of this approach that suggested by Gibbs and Maher (1967), cumulative frequency distribution was constructed by ranking of monthly precipitation totals from a long-term record from highest to lowest. The second stage is splitting of distribution (0.0 to 100) into 10 deciles and note the minimum and maximum amount of rainfall in each of the deciles. The first and second deciles are the precipitation values equal or less 10% and 10 to 20% of all recorded precipitation values (respectively) etc. Final stage of this approach is recognition of drought classification (severity of drought) by comparing the amount of precipitation with maximum and minimum values of each of the deciles. The deciles of distribution grouped into five classes (two deciles per class). If precipitation falls into class 1 (deciles 1 and 2 or the lowest 20%), it is classified as much below normal. Class 2 (deciles 3 and 4 or “20 to 40 %”) indicate below normal precipitation, class 3 (deciles 5 and 6 or “40 to 60 %”) indicate near normal precipitation, class 4 (deciles 7 and 8 or “60 to 80 %”) indicate above normal precipitation and class 5 (deciles 9 and 10 or “80 to 100 %”) indicate much above normal precipitation.
2.2.3 SPI Drought Index
SPI computation (McKee et al. 1993) is involving two stage that at first stage a probability distribution (e.g. gamma distribution) fitted to a long-term precipitation record at the desired station. Transformation of gamma distribution into normal distribution is second stage that resulted in zero mean SPI. Both dry and wet conditions can be monitored using SPI drought index and this index may be computed with different time steps (e.g. 1 month, 3 months and 24 months). The use of different time scales allows the effects of a precipitation deficit on different water resource components (groundwater, reservoir storage, soil moisture and stream flow) to be assessed (Morid et al. 2006). How to calculate SPI drought index can be found in several papers including McKee et al. (1993), Guttman (1999) and Wu et al. (2001).
2.2.4 Z-Score Drought Index (ZSI)
For calculation of this index Eq. (1) is used:
Where j is the current month, S is the standard deviation, Pj is precipitation of j month and \( \overline{P} \) is mean monthly precipitation. The higher value of mentioned index, the higher severe the drought.
2.2.5 CZI Drought Index
The CZI is based on the Wilson–Hilferty cube-root transformation (Kendall and Stuart 1977). Assuming that precipitation data follow the Pearson Type III distribution, the index is calculated as:
Where j is the current month, Cs is coefficient of skewness, n is the total number of months in the record, σ is the standard deviation, φj is standard variate and xj is precipitation of j month.
2.2.6 MCZI Drought Index
To calculate the MCZI drought index that presented by Wu et al. (2001), the median of precipitation (Med) is used instead of the mean of precipitation in the calculation of the CZI (i.e. Med is substituted for x in Eqs. 2 to 4).
2.2.7 RDI Index
RDI index that presented by Tsakiris (2004) presented in the 3 forms includes: Initial value of RDI (αk), Normalized RDI (RDIn) and Standardized RDI (RDIst).
Where Pij is precipitation and PETij is potential or reference evapotranspiration within the months “j” of hydrological year and “i” that usually starts from October in Iran, \( {\overline{a}}_k \) is the arithmetic mean of αk value, yk is\( \ln \left({\upalpha}_{\mathrm{k}}^{\left(\mathrm{i}\right)}\right) \), \( {\overline{y}}_k \) is the arithmetic mean of yk and \( {\widehat{\sigma}}_{yk} \) is the standard deviation.
To calculate the RDIst fitted the gamma Probability Density Function (GPDF) at the given frequency distribution of αk(Tsakiris et al. 2007; Tsakiris and Vangelis 2005). The frequency or probability density function of gamma distribution is defined as follw:
Where α is shape factor; β is scale parameter and x is precipitation quantity and Γ(α) is gamma function.
Because the GPDF has not been defined for x = 0, the cumulative probability becomes:
Where as H(x) is cumulative probability, q is the probability of zero precipitation and G(x) is the cumulative probability of the incomplete GPDF. Table 2 indicate the classification of drought based on RDI index.
2.3 Statistical Analysis
For assessment of the relationship between RDI index and other drought indices was used through generalized estimating equations (GEE) using IBM SPSS Statistics software. Because in this research the data series are dependent and stationary, we faced with a repeated-measures panel data problem. To solve this problem GEE was used (Zarei et al. 2019; Ballinger 2004; Ghisletta and Spini 2004; Gardiner et al. 2009; Hu et al. 1998). In these equations, the dependent variable (response) was RDI index and the other drought indices were subject variables (predictors). The general estimating equation that used in this study was as follow:
Where Y is the RDI index, x is each of other indices and b0, b1, b2, b3 and b4 are the coefficients. According to the analysis of variance of model, all of coefficients that obtained in this model are significant (P value <0.05).
2.4 Model Validation
Using the different measures of goodness of fit, the simulation performance of all developed models evaluated. The measures used in this research are included Root Mean Squared Error (RMSE), Mean Absolute Error (MAE), Nash-Sutcliffe Efficiency coefficient (NSE), test of reliability between observed and simulated data and correlation coefficient (R-squared value). Numerous studies indicated the appropriateness of these measures to assess efficency of hydrological models (Legates and McCabe Jr 1999; Zarei and Moghimi 2017; Cancelliere et al. 2007; Djerbouai and Souag-Gamane 2016). In general, small values for RMSE and MAE and high values of NSE and correlation coefficients indicate a good model. Test of reliability shows the model ability to data simulation. Only when the perfect level of reliability is guaranteed that the reliability line is simply the diametrical. The more calculated reliability is closer to this line, the more reliable simulation has been made. NSE, RMSE, MAE and R2 calculated as Eqs. 11, 12, 13 and 14:
Where, Ti is observed RDI, \( \overline{T} \) is the arithmetic mean of Ti, \( {\widehat{T}}_{\mathrm{i}} \) is the simulated T and \( \overline{\widehat{T}} \) is the arithmetic mean of \( {\widehat{T}}_{\mathrm{i}} \).
In order to test of reliability between observed and simulated RDI, paired-samples T-Test was used at significance level of 0.95 (P Value>0.05).
3 Results and Discussion
All of the considered indices in this study (with the exception of DI and PN) are comparable because of an almost similar range of numerical values. To make the DI and PN indices comparable with the RDI classes, their values must be classified into similar classes (Table 2). Classification of drought severity based on applied indices in this paper are presented in Table 2. Scatter plot between RDI and other indices were shown in Figs. 2, 3, 4, 5, 6, 7 and 8 that represent the existing relationship between RDI and other indices that for calculating these indices only precipitation data was required.
3.1 Simulate the RDI Using PN
First, the coefficients of the generalized estimating equations between RDI and PN was obtained. According to the results, all of the coefficients of Eq. (10) is almost zero with the exception of “b0” and “b1”. However, in Chabahar station, only b1 coefficient is non-significant. Between coefficients, “b0”, had the highest effect that this effect is decreasing. The goodness of fit measures values (Figs. 8, 9, 10, 11, 12, 13, 14, 15, 16, 17, 18, 19 and 20) indicted that PN index is not appropriate index for simulating RDI because of lower value of NSE and R2 and higher values of RMSE, MAE, QIC and QICC compared to other indices with the exception of Z-Score. Meanwhile, results of T-Test indicated that, only in Bam, Saravan, Sharebabak and Zabol stations, the difference between observed and simulated data were not significant (P value >0.05).
3.2 Simulate the RDI Using DI
According to the results of the generalized estimating equations that obtained between RDI and DI, all of the coefficients of Eq. (10) were significant (P value <0.05) with the exception of “b0” in Saravan station and “b4” in Zabol station. These coefficients indicate the highest effect of second order of this index in simulating RDI that this effect is decreasing. Values of all goodness of fit measures (Figs. 9, 10, 11, 12, 13, 14, 15, 16, 17, 18, 19 and 20) indicated that DI index is appropriate index for simulating RDI because of high values of NSE and R2 and low values of RMSE, MAE, QIC and QICC. Meanwhile, results of T-Test indicated that, only in Bandar Abbas, Bushehr, Chabahar, Fasa, Shiraz and Sirjan stations, the difference between observed and simulated data were significant at significance level of 95% (P value >0.05). Generally, DI index is the best after SPI, CZI and MCZI indices. Although, DI index in Saravan station is the best index for simulating RDI.
3.3 Simulate the RDI Using CZI
Results of the generalized estimating equations that obtained between RDI and CZI indicated that, all of the coefficients of Eq. (10) were significant at significance level of 95% (P value <0.05) with the exception of “b0” in Bam and Zahedan stations, “b3” in Zabol station and “b4” in Iranshahr, Jask, Shahrebabk and Zabol stations. The first order of CZI has the highest effect in simulating RDI that this effect is incremental. Values of all goodness of fit measures (Figs. 9, 10, 11, 12, 13, 14, 15, 16, 17, 18, 19 and 20) indicated that, after SPI, CZI index is most appropriate index for simulating RDI because of high values of NSE and R2 and low values of RMSE, MAE, QIC and QICC. Meanwhile, results of T-Test indicated that, only in Bandar Abbas, Bushehr, Chabahar and Fasa stations, the difference between observed and simulated data were significant (P value >0.05).
3.4 Simulate the RDI Using MCZI
Results of the generalized estimating equations that obtained between RDI and MCZI indicated that, all of the coefficients of Eq. (10) were significant (P value <0.05) with the exception of “b4” in Zabol station. According to the coefficients, the first order of MCZI has the highest effect in simulating RDI that this effect is incremental. After SPI and CZI indices, MCZI is most appropriate index for simulating RDI, because of high values of NSE and R2 and low values of RMSE, MAE, QIC and QICC (Figs. 9, 10, 11, 12, 13, 14, 15, 16, 17, 18, 19 and 20). Meanwhile, results of T-Test showed that, only in Bandar Abbas, Bushehr, Chabahar, Fasa, Jask, Shiraz and Sirjan stations, the difference between observed and simulated data was significant (P value >0.05) and in other stations, this difference was non-significant.
3.5 Simulate the RDI Using SPI
Results indicated that, all of the coefficients of Eq. (10) were significant at significance level of 95% (P value <0.05) with the exception of “b4” in Abadeh, Bandar Abbas, Bandar Lengej, Bushehr, Chabahar, Jask, Iranshahr and Zabol stations. According to the coefficients, the first order of SPI has the highest effect in simulating RDI that this effect is incremental. Values of all goodness of fit measures (Figs. 9, 10, 11, 12, 13, 14, 15, 16, 17, 18, 19 and 20) indicated that SPI index is the best index for simulating RDI because of higher values of NSE and R2 and lower values of RMSE, MAE, QIC and QICC. The values of NSE, R,2 RMSE, MAE, QIC and QICC varies from 0.884 to 0.997, 0.960 to 0.996, 0.057 to 0.199, 0.028 to 0.235, 12.97 to 66.82 and 9.21 to 53.27, respectively. Meanwhile, results of T-Test indicated that, the difference between observed and simulated data were not significant (P value >0.05) in all stations. Results of comparison between RDI and SPI indices confirmed the results of Jamshidi et al. (2011).
3.6 Simulate the RDI Using Z-Score
Generalized estimating equations that obtained between RDI and Z-Score indicated that, all of the coefficients of Eq. (10) were significant at significance level of 95% (P value <0.05). These coefficients represent the maximum positive effect of first order of this index in simulating RDI. According to values of all goodness of fit measures (Figs. 9, 10, 11, 12, 13, 14, 15, 16, 17, 18, 19 and 20), Z-Score had lowest appropriateness for simulating RDI because of lowest values of NSE and R2 and highest values of RMSE, MAE, QIC and QICC compared to other indices that used in this study. Results of T-Test showed that, only in Bam, Saravan, Shahrebabak and Zabol stations, the difference between observed and simulated data was non-significant (P value >0.05) and in other stations, this difference was significant.
The results of this study indicated that for used time step (1-month) the most of indices had high correlation with RDI index, using generalized estimating equations. Whereas, the results of Jain et al. (2015) study in districts of Ken River Basin, located in central India, indicated that the best correlation between drought indices of SPI, CZI, Rainfall Departure (RD) and Rainfall Decile based drought index (RDDI) with Effective Drought Index (EDI) index occurred at 9-month time step, using Pearson Product-Moment correlation coefficient.
In Morid et al. (2006) research that considered the correlation of different drought indices (DI, PN, CZI, MCZI, Z-Score and EDI) with SPI, using Pearson correlation coefficient in Tehran province (Iran), results indicated the poor correlation between drought indices of DI, PN and EDI with SPI and the maximum correlation of CZI, MCZI, Z-Score with SPI was 0.89. Whereas the results of generalized estimating equations in our study indicate the high values of correlation coefficients between drought indices of DI, PN, SPI, CZI, MCZI and Z-Score with RDI, especially between SPI and RDI in all considered statins.
Generally, the results of this study indicated that in Bandar Abbas, Bushehr, Chabahar and Fasa stations, only the SPI index was appropriate for simulating RDI. In Shiraz and Sirjan stations, the CZI was appropriate for simulating RDI, in addition to the SPI. The PN and Z-Score indices were appropriate for simulating RDI only at Bam, Saravan, Shahrebabak and Zabol stations. In Jask station, only the CZI and DI were appropriate for simulating RDI, in addition to the SPI.
In developing countries, the number and distribution of synoptic stations are not within the WMO standards and at the existing stations the length of the statistical period is not enough. Also, in these countries, usually climatic parameters picked up by satellites are not readily available. However, in these regions, rain-gauge stations are well distributed by enough length of the statistical period. Therefore, it is suggested to simulate RDI index (as a strong drought index, that need different meteorological data (more than 10 parameters)) using SPI index that requires only precipitation data.
4 Conclusion
Reconnaissance drought identification and assessment index (RDI) is very strong index that extensively used for monitoring and forecasting drought characteristics because of the possible role of ET0 in the detection of drought events. Major problem to calculate this index is lack of data. This study investigated this problem solution via simulating RDI using other indices that for calculating these, only precipitation data is required. Results of this study indicated the appropriateness of DI, SPI, CZI and MCZI and indices for simulating RDI and non-appropriateness of Z-Score and PN for this purpose. Between these indices, SPI had most appropriateness for simulating RDI, so that results of T-Test indicated that, the difference between observed and simulated data were not significant (P value >0.05) in all stations. Meanwhile, values of all goodness of fit measures indicated that SPI index is the best index for simulating RDI because of higher values of NSE and R2 and lower values of RMSE, MAE, QIC and QICC. The values of NSE, R,2 RMSE, MAE, QIC and QICC varies from 0.884 to 0.997, 0.960 to 0.996, 0.057 to 0.199, 0.028 to 0.235, 12.97 to 66.82 and 9.21 to 53.27, respectively. According to values of all goodness of fit measures (Figs. 9, 10, 11, 12, 13, 14, 15, 16, 17, 18, 19 and 20), Z-Score had lowest appropriateness for simulating RDI. Morid et al. (2006) compared seven meteorological drought indexes (include DI, PN, SPI (reference method), CZI, MCZI, Z-Score and EDI indexes)) to drought evaluation in Iran. Results of this paper showed that SPI index, CZI index and Z-Score index perform similarly with regard to drought identification. Results of our paper in case of similarity of SPI and CZI indexes were similar to results of Morid et al. So, in stations with a shortage of climatological data series, it is suggested to simulate RDI index (as a strong drought index) using SPI index that requires only precipitation data.
References
Ballinger, G.A.: Using generalized estimating equations for longitudinal data analysis. Organ. Res. Methods. 7(2), 127–150 (2004)
Banimahd, S.A., Khalili, D.: Factors influencing Markov chains predictability characteristics, utilizing SPI, RDI, EDI and SPEI drought indices in different climatic zones. Water Resour. Manag. 27(11), 3911–3928 (2013)
Bennani, S., Nsarellah, N., Jlibene, M., Tadesse, W., Birouk, A., Ouabbou, H.: Efficiency of drought tolerance indices under different stress severities for bread wheat selection. Aust. J. Crop. Sci. 11(4), 395–405 (2017)
Cancelliere, A., Di Mauro, G., Bonaccorso, B., Rossi, G.: Drought forecasting using the standardized precipitation index. Water Resour. Manag. 21, 801–819 (2007)
Djerbouai, S., Souag-Gamane, D.: Drought forecasting using neural networks, wavelet neural networks, and stochastic models: case of the Algerois basin in North Algeria. Water Resour. Manag. 30, 2445–2464 (2016)
Gabriele, B., Tommaso, C., Nicola, R., Ilaria, G.: Assessment of drought and its uncertainty in a southern Italy area (Calabria region). Measurement. 113, 205–210 (2017)
Gardiner, J.C., Luo, Z., Roman, L.A.: Fixed effects, random effects and GEE: what are the differences? Stat. Med. 28(2), 221–239 (2009)
Ghisletta, P., Spini, D.: An introduction to generalized estimating equations and an application to assess selectivity effects in a longitudinal study on very old individuals. J. Educ. Behav. Stat. 29(4), 421–437 (2004)
Gibbs WJ, Maher JV Rainfall deciles as drought indicators. Bur Meteorol Bulletin No.48, Melbourne, Australia (1967)
Guttman, N.B.: Accepting the standardized precipitation index: a calculation algorithm. J Am Water Resour Assoc. 35(2), 311–322 (1999)
Hu, F.B., Goldberg, G., Hedeker, D., Flay, B.R., Pentz, M.A.: Comparison of population-averaged and subject-specific approaches for analyzing repeated binary outcomes. Am. J. Epidemiol. 147(7), 694–703 (1998)
Ismail, D., Ashok, K.M., Zekai, S.: Long-term spatio-temporal drought variability in Turkey. J. Hydrol. 552, 779–792 (2017)
Jain, V.K., Pandey, R.P., Jain, M., Byun, H.R.: Comparison of drought indices for appraisal of drought characteristics in the Ken River basin. Weather Clim Extrem. 8, 1–11 (2015)
Jamshidi H, Khalili D, Rezaeian Zadeh M, Zia Hosseinipour E Assessment and comparison of SPI and RDI meteorological drought indices in selected synoptic stations of Iran. World Environmental and Water Resources Congress, Bearing Knowledge for Sustainability, ASCE (2011)
Kendall, M.G., Stuart, A.: The Advanced Theory of Statistics. Vol.1, Distribution Theory, pp. 400–401. Charles Griffin Company, London (1977)
Khalili, D., Farnoud, T., Jamshidi, H., Kamgar-Haghighi, A.A., Zand-Parsa, S.: Comparability analyses of the SPI and RDI meteorological drought indices in different climatic zones. Water Resour. Manag. 25, 1737–1757 (2011)
Khan, M.I., Liu, D., Fu, Q., Saddique, Q., Faiz, M.A., Li, T., Qamar, M.U., Cui, S., Cheng, C.: projected changes of future extreme drought events under numerous drought indices in the eilongjiang province of China. Water Resour. Manag. 31, 3921–3937 (2017)
Kwarteng, F., Shwetha, G., Rahul, P.: Reconnaissance drought index as potential drought monitoring tool in a Deccan plateau, hot semi-arid climatic zone. International Journal of Agriculture Sciences. 9(1), 2183–2186 (2017)
Legates, D.R., McCabe Jr., G.J.: Evaluating the use of “goodness-of-fit” measures in hydrologic and hydroclimatic model validation. Water Resour. Manag. 35(1), 233–241 (1999)
Mashari Eshghabad, S., Omidvar, E., Solaimani, K.: Efficiency of some meteorological drought indices in different time scales (case study: Tajan Basin, Iran). Ecopersia. 2(1), 441–453 (2014)
McKee TB, Doesken NJ, Kleist JThe relationship of drought frequency and duration to time scales. In: Proc. 8th Conf. on Applied Climatology, 17–22 January, American Meteorological Society, Mass, 179–184 (1993)
Montaseri, M., Amirataee, B., Nawaz, R.: A Monte Carlo simulation-based approach to evaluate the performance of three meteorological drought indices in northwest of Iran. Water Resour. Manag. 31, 1323–1342 (2017)
Morid, S., Smakhtin, V., Moghadasi, M.: Comparison of seven meteorological indices for drought monitoring in Iran. Int. J. Climatol. 26(7), 971–985 (2006)
Mpelasoka, F., Hennesy, K., Jones, R., Bates, B.: Comparison of suitable drought indices for climate change impacts assessment over Australia towards resource management. Int. J. Climatol. 28, 1283–1292 (2008)
Raziei, T.B., Saghafian Paulo, A.A., Pereira, L.S., Bordi, I.: Spatial patterns and temporal variability of drought in western Iran. Water Resour. Manag. 23, 439–455 (2009)
Smakhtin VU., Hughes DA Review, automated estimation and analyses of drought indices in South Asia, International water management institute, Working Paper 83, Drought Series, Paper 1 (2004)
Tabrizi, A.A., Khalili, D., Kamgar-Haghighi, A.A., Zand-Parsa, S.: Utilization of time-based meteorological droughts to investigate occurrence of stream flow droughts. Water Resour. Manag. 24, 4287–4306 (2010)
Tsakiris G Meteorological Drought Assessment. Paper prepared for the needs of the European Research Program MEDROPLAN (Mediterranean Drought Preparedness and Mitigation Planning), Zaragoza, Spain (2004)
Tsakiris, G., Vangelis, H.: Establishing a drought index incorporating evapotranspiration. Eur Water. (9/10), 3–11 (2005)
Tsakiris, G., Pangalou, D., Vangelis, H.: Regional drought assessment based on reconnaissance drought index (RDI). Water Resour. Manag. 21(5), 821–833 (2007)
Wu, H., Hayes, M.J., Welss, A., Hu, Q.: An evaluation the standardized precipitation index, the China-Z index and the statistical z-score. Int. J. Climatol. 21, 745–758 (2001)
Zarei, A.R.: Evaluation of drought condition in arid and semi- arid regions, using RDI index. Water Resour. Manag. 32, 1689–1711 (2018). https://doi.org/10.1007/s11269-017-1898-9
Zarei, A.R., Mahmoudi, M.R.: Evaluation of changes in RDIst index affected by different potential evapotranspiration calculation methods. Water Resour. Manag. 31, 4981–4999 (2017). https://doi.org/10.1007/s11269-017-1790-7
Zarei, A.R., Moghimi, M.M.: Environmental assessment of semi-humid and humid regions based on modelling and forecasting of changes in monthly temperature. Int. J. Environ. Sci. Technol. 16, 1457–1470 (2017). https://doi.org/10.1007/s13762-017-1600-z
Zarei, A.R., Moghimi, M.M., Bahrami, M., Mahmoudi, M.R.: Evaluation of changes trend in seasonal drought based on actual data (1980-2014) and predicted data (1980-2019) in south-west of Iran. Desert Management journal. 7(1), 71–85 (2016a)
Zarei, A.R., Moghimi, M.M., Mahmoudi, M.R.: Analysis of changes in spatial pattern of drought using RDI index in south of Iran. Water Resour. Manag. 30(11), 3723–3743 (2016b)
Zarei, A.R., Shabani, A., Mahmoudi, M.R.: Comparison of the climate indices based on the relationship between yield loss of rain-fed winter wheat and changes of climate indices using GEE model. Sci. Total Environ. 661, 711–722 (2019)
Zareiee, A.R.: Evaluation of changes in different climates of Iran, using De-Martonne index and Mann-Kendall trend test. Natural Hazards and Earth System Science. Discuss. 2, 2245–2261 (2014)
Author information
Authors and Affiliations
Corresponding author
Additional information
Responsible Editor: Ashok Karumuri.
Publisher’s Note
Springer Nature remains neutral with regard to jurisdictional claims in published maps and institutional affiliations.
Rights and permissions
About this article
Cite this article
Moghimi, M.M., Zarei, A.R. Evaluating Performance and Applicability of Several Drought Indices in Arid Regions. Asia-Pacific J Atmos Sci 57, 645–661 (2021). https://doi.org/10.1007/s13143-019-00122-z
Received:
Revised:
Accepted:
Published:
Issue Date:
DOI: https://doi.org/10.1007/s13143-019-00122-z