Abstract
In this study, we analyse possible future climatic changes in three catchments, namely, Pyshma, Vagai and Loktinka located in the Western Siberian lowland region, and the resulting impact on hydrological regimes. It involved downscaling the GCM outputs based on the established statistical relationship between large-scale atmospheric variables and station data and simulating the effects of climate change on hydrological regimes via hydrological modelling. This was done for RCP 2.6, 4.5 and 8.5 based on second-generation Canadian Earth System Model used in the IPCC fifth assessment report. This paper provides the first climate change projections on a local scale in these catchments. The statistical downscaling showed that there will be an increase in both maximum and minimum temperature at all stations under all scenarios. The mean annual daily precipitation increased in Loktinka and Pyshma basins under all scenarios, but there was no clear trend in Vagai basin. The possible increase in annual precipitation is mostly due to the projected increase in autumn and winter precipitation. Annual streamflow tends to increase in all catchments under all scenarios.
Similar content being viewed by others
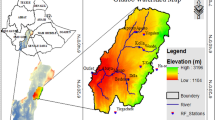
Avoid common mistakes on your manuscript.
Introduction
Climate change has potential serious impacts on human, society and environment. The Intergovernmental Panel on Climate Change (IPCC) fifth assessment report has shown an increase of 0.85 °C in the global mean temperature since 1880 until 2012 (IPCC 2013). These changes in global temperature have been accompanied by changes in climate in different ways (Feng et al. 2014). Many regions have experienced changes in precipitation leading to frequent occurrence of floods (Min et al. 2008, 2011) and droughts (Dai 2011, 2012). These changes in climate system have a strong impact on local and regional hydrological regimes in many regions of the world (Dibike and Coulibaly 2005; Hu et al. 2013: Kiesel et al. 2019). The impacts of climate change to society and natural resources depend on the response of the hydrological cycle to global warming (Marvel and Bonfils 2013). So, while discussing climate change impacts on hydrological regimes, it is extremely important to understand the hydrological cycle. The hydrological cycle comprises processes such as precipitation, infiltration, percolation, runoff, storage and evapotranspiration, through which water fluxes are continuously exchanged between the atmosphere, the land surface and sub-surfaces and the oceans. While precipitation is considered as a driver of the hydrological cycle (Htut 2014), increase in temperature leads to an intensification of this cycle (Marvel and Bonfils 2013). Thus, changes in climate affect the processes of the hydrological cycle, making climate change one of the significant causes of hydrological change (Bhuvandas et al. 2014).
The oceans and glaciers have experienced considerable changes (Feng et al. 2014). The Arctic Sea ice has been continuously decreasing and recorded its lowest extent in 2012 (Viñas 2014), while the snow caps and glaciers in the Himalayas have been continuously melting (Yao et al. 2012). The continuous shrinkage of glaciers and thawing of permafrost affect runoff and water resources downstream (IPCC 2013). If these changes continue and become even more pronounced in the future, they will likely have serious implications on the ecosystem, environment and the whole society. In these regards, it is extremely important to conduct research on the potential impacts of climate change on hydrological regimes so that people and society can foresee and respond to the tentative future challenges, either by mitigating the worst condition that are likely to happen in future or at least be well prepared and resilient to face the possible challenges.
Though there are several studies on climate change and hydrological impacts, these cover large spatial scale (Yang et al. 2002, 2004; Peterson et al. 2002; Kabanov and Lykosov 2006; Bulygina et al. 2011; Rawlins et al. 2010; Khon and Mokhov 2012; Shiklomanov et al. 2013; Groisman et al. 2013) and there are merely few studies that focus on the southern part of Western Siberia (Degefie et al. 2014). The limited studies again do not cover forecasting the possible shift in the hydrological components. Additionally, most of the management plans are tailored to specific ecoregions (Omernik and Bailey 1997), which makes watershed-scale studies essential (Kiesel et al. 2018). On top of that, Western Siberian lowlands (WSL) are characterized by highly variable hydrological conditions and the region has limited strategies to adapt to the impacts of climate change and mitigate the problem (SASCHA 2014), which certainly will have implications on the socio-economy of the area, making this region highly vulnerable to the change in climate. This study on the potential impacts of climate change on the hydrology of the Western Siberian lowland catchment is a small endeavour towards increasing the climatic and hydrological information of the area and filling the prevailing research gap. In this study, we try to explore: (1) how the future temperature and precipitation of the study sites will be and (2) how the changes in the climatic condition will affect the hydrological regime of the area.
Study area and data
Location and general characteristics
This study is focussed on three river catchments, namely, Pyshma (56°57′34.72′′N; 65°49′33.01′′E; gauge Bogandinskoye), Vagai (56°29′44.70′′N; 68°4′32.09′′E; gauge Ust-Lamenka) and Loktinka (56°3′52.99′′N; 69°15′34.13′′E; gauge Bykova), which are located in the southern part of the Western Siberian lowland region where permafrost is absent. The three rivers are tributaries of Irtysh River, which joins the Ob River, one of the longest rivers in the Northern Hemisphere, before draining into the Arctic Ocean. The selected three catchments collectively occupy the three ecoregions, West Siberian Taiga, Pre-Taiga and forest-steppe zones. Among these catchments, the Pyshma is the largest, covering an area of 16,762 km2 followed by the Vagai (3348 km2) and the Loktinka (373 km2). The topography of the WSL region is generally plain. The elevation of the Pyshma basin ranges from about − 90 m below mean sea level (due to mining activities) to 326 m above mean sea level (amsl), whereas the altitude of the Vagai and the Loktinka catchment ranges from 85 to 158 m and 80 to 143 m amsl, respectively (Jarvis et al. 2008). The relative size, location and respective ecoregions of these three catchments are shown in Fig. 1.
Location of the three catchments, Pyshma, Vagai and Loktinka, within three different ecoregions (WWF 2014). Black arrow on inset shows the location within Russia, and numbers on map show latitude and longitude
The climate in the WSL is primarily determined by cold air masses originating from the Arctic Ocean and Asian continent, and dry winds blowing from Kazakhstan and Middle Asia resulting in sudden changes in weather and hence also leading to change in climate. In general, the climate is continental in this area. The current annual mean temperature for Pyshma, Vagai and Loktinka basin is 2.4 °C, 1.5 °C and 1.4 °C, respectively (NOAA 2013). Precipitation constantly decreases from west to east: For the Pyshma basin, the annual mean precipitation is 470 mm, 514 mm and 509 mm in Tyumen, Kamyshlov and Ekaterinburg stations, respectively, whereas the annual mean precipitation in Vagai is 478 mm in Yalutorovsk station. Ishim station (Loktinka catchment) receives the lowest (396 mm) mean annual precipitation compared to the other two catchments (NOAA 2013).
The hydrology of all three basins is characterized by high seasonal variability as snowmelt in April and May causes very high river water levels. The daily mean streamflow in Pyshma River is 55 m3/s (102 mm/year). While the Vagai River has a daily mean streamflow of 4.3 m3/s (39.9 mm/year), Loktinka River has 0.5 m3/s (41.7 mm/year) (MDHS 1961–1988).
Data collection
The hydro-meteorological station data were provided by the hydrology sub-project of SASCHA (Sustainable land management and adaptation strategies to climate change for the Western Siberian grain belt) by the Department of Hydrology and Water Resources Management, University of Kiel, Germany, where data from multiple sources were collected (Kiesel et al. 2018). The temperature and precipitation data from 1961 to 2005 was used from climate station Ishim for Loktinka basin, Yalutorovsk station for Vagai basin, and Tyumen, Ekaterinburg and Kamyshlov stations for Pyshma basin. The proxy of observed and modelled large-scale atmospheric variables (predictor variables) is available from the National Centre for Environmental Prediction (NCEP) reanalysis project (Kalnay et al. 1996) and the second-generation Canadian Earth-System Model (CanESM2). The primary reason behind using NCEP is because they have created the global data sets for a long time period for different atmospheric parameters using the model similar to the one used for weather forecast (Kalnay et al. 1996). The data set is considered as a proxy to observed data and is available at temporal coverage of four times daily, daily and monthly values (Kalnay et al. 1996). CanESM2 is used, as it is the only model that supplied daily predictor variables available to be used in SDSM. Both the proxy of the observed and modelled large-scale atmospheric variables were extracted for the grid-point that contains the climate stations (Fig. 1) from the Canadian Climate Data and Scenarios website (http://ccds-dscc.ec.gc.ca/). CanESM2 employed T63 triangular truncation with spatial resolution of 128 × 64 and 35 vertical layers, whereas the Ocean component has 40 vertical layers with approximately 10 m resolution in the upper ocean and 1.41° (longitude) × 0.94° (latitude) horizontal resolution (Arora and Boer 2014). A total of four grid points were used, one for each station except for Ekaterinburg and Kamyshlov stations, which share the same grid point. The grid cell size is uniform along the longitude with horizontal resolution of 2.8° and nearly uniform along the latitude of roughly 2.8° (Radojevic 2014). The 26 predictor variables used in this study are given in Table 1.
Methodology
Statistical downscaling model (SDSM)
The statistical downscaling requires development of quantitative relationships between large-scale atmospheric variables/GCM outputs (predictors) and local-scale observed variables (predictands) (Wilby et al. 2004). Daily maximum and minimum temperature and daily precipitation data were used as predictand. Mathematically, the relationship between predictors and predictands can be written as (von Storch et al. 2000): Y = f (X), where Y = predictand, X = predictor and f = stochastic and/or deterministic function which has to be determined empirically from historical observations. Generally, three important assumptions are made while using this type of downscaling technique (von Storch et al. 2000): (1) the predictors are variables of relevance and are realistically modelled by the GCM, (2) the used predictors fully represent the climate change signal, and (3) the relationship is valid also under altered climate condition.
The SDSM (Ver. 4.2.9) was used in this study to downscale and project the future climate data. It is a downscaling tool developed by Wilby et al. (2002) for deriving local climate change impacts using statistical downscaling technique and is a hybrid of the stochastic weather generator and regression-based methods (Liu et al. 2011). The model has four main parts; identification of predictors, model calibration, weather generator and generation of future series of climate variables.
Selection of predictor variables
The selection of the predictor variables is the most significant step in the statistical downscaling procedure. The better output of SDSM depends on the selection of appropriate predictor variables while developing the predictor–predictand relationship, because the choice of predictors determines the character of the downscaled climate scenario. The process of selecting appropriate predictor variables in this study is based on Dibike and Coulibaly (2005), Hu et al. (2013) and Hassan et al. (2014): Therefore, the observed daily data of large-scale predictor variables representing the current climatic condition (1961–2005), derived from NCEP reanalysis data set, was used to investigate the explained variance by each predictor–predictand pairs. The predictor variables with high explained variance were selected for partial correlation to understand the level of association among themselves. It was done because there might be such kind of predictor variables which have high explained variance, but significantly correlated with other predictor variables. As such variables do not add much value in the decision-making process, these variables were neglected and only those not having significant correlation with other predictor variables were selected for the scatter plot. These were selected based on the significance level (P) and correlation coefficient (r). The association of each selected predictor variable with the predictand was visualized via the scatter plot and the decision was made accordingly to select the most appropriate set of predictor variables. Let us take an example of maximum temperature for station Kamyshlov. Among the available 26 predictor variables from the NCEP reanalysis project, the 5 potential predictor variables, namely, p500gl, p850gl, s850gl, shumgl and tempgl, which have high explained variances were selected. These were then put forward for partial correlation analysis to identify the correlation among each other and it was found that p850gl was significantly associated with other predictor variables. Hence, this variable was discarded from selection. The remaining four predictor variables were then checked individually in the scatter plot and it was revealed that all of them had good association with the predictand and thus all four of them were selected as the final set of predictor variables. The same procedure was followed for all the predictands and stations to select the most appropriate predictor variable.
Calibration and validation of the model
The 45 years observed historical data from 1961 to 2005 were split into 30 years (1961–1990) calibration and 15 years (1991–2005) validation period. During calibration, the model type was set to monthly which means that 12 regression equations were developed for 12 months for each station. The selected predictor variables were used to derive a parameter file which was then used for generating new synthetic data for 15 years (1991–2005). An iterative process was used to adjust variance inflation and bias correction until the synthesized data approached or resembled the observed data in terms of monthly mean and variance for maximum temperature (Tmax) and minimum temperature (Tmin), and monthly mean, variance, mean dry-spell length and mean wet-spell length for precipitation for all stations.
Among the variety of model evaluation techniques available (Moriasi et al. 2007), the performance of the SDSM was evaluated based on the correlation coefficient (R), coefficient of determination (R2) and root mean square error (RMSE). R is the measure of the linear relationship between the observed and simulated data, whereas R2 compares the explained variance of the modeled data with the total variance of the observed data (Liu et al. 2011). Similarly, the RMSE is an error index used to measure the difference between the observed and simulated values (Moriasi et al. 2007).
After validation of the model, the derived parameter file containing the regression weights was used for downscaling the future data based on the Canadian climate model output CanESM2, which supplies large-scale atmospheric predictor variables. For each of the representative concentration pathway (RCPs) 8.5, 4.5 and 2.6, 20 ensembles of synthetic daily time series data were generated for the period of 2006–2099 for tmax, tmin and precipitation for all stations. The mean of these 20 ensembles was then used as final daily weather data for the specified period. The analysis of future climatic variables was done by classifying the future data into three time windows (2011–2040, 2041–2070 and 2071–2100), which are denoted as the 2020s, 2050s and 2080s, respectively. The baseline period was defined from 1981 to 2010 to assess the anomaly of the future climate variables.
Soil and water assessment tool (SWAT)
SWAT is an ecohydrological, process-based model which can be run on the basin scale and for continuous time periods. It was developed in the early 1990s by the Agriculture Research Service of the United States Department of Agriculture (ARS-USDA) (Arnold and Fohrer 2005; Arnold et al. 1998). SWAT has been used in evaluating hydrological processes (Pfannerstill et al. 2015) and in studying the impacts of climate change on hydrological regimes (Guse et al. 2015; Zuo et al. 2015), as it has proven to be effective for assessing water resources at various scales and it is also computationally efficient and capable of continuous simulation over long periods (Gassman et al. 2007). SWAT divides a river basin into sub-basins and then into multiple units of unique slope, soil and land use characteristics called hydrological response units (HRUs). The water movements and losses are considered individually for each HRU and aggregated in sub-basin scale and then routed to basin outlets through the channel network (Zuo et al. 2015). SWAT simulates snowmelt and groundwater processes (percolation and groundwater contribution to streamflow). The baseline model used in this study was set up, calibrated and validated on a daily time step by Kiesel et al. (2018) and is optimized for lowland conditions considering depressional storages (Kiesel et al. 2010) and a complex groundwater concept (Pfannerstill et al. 2014) and is called SWAT-3s. Optimization of the models was carried out using multi-objective performance criteria that include the RSR (ratio of the root mean square error to the standard deviation of measured data, Moriasi et al. 2007) of four sections of the flow duration curve and the overall Kling–Gupta efficiency (KGE, Gupta et al. 2009). Model performances reached KGE values of 0.70–0.81 for calibration and 0.1–0.56 for validation (1 = ideal value). Considering that validation needed to be carried out against severely outdated discharge rating curves and a few years of data, the model results are considered acceptable (Kiesel et al. 2018). The baseline run was based on the measured data, whereas the downscaled future climate scenarios (Tmax, Tmin and precipitation for the three RCPs) from SDSM were run in SWAT for the period 2005–2099.
Results
Selection of predictor variables
The dominant predictor variables selected for both Tmax and Tmin for all stations were 1000 hPa specific humidity and temperature at 2 m. Similarly, for precipitation, total precipitation, relative vorticity of wind at 850 hPa and geopotential height at 850 hPa were the most common predictor variables selected at all the stations. Predictor variables for Tmax and Tmin have correlation coefficients ranging from 0.760 to 0.874, while these are below 0.35 for precipitation. The list of selected predictor variables, their correlation coefficient and significance level for all the climatic variables and stations are given in Table 2.
Evaluation of the performance of SDSM
The performance of the SDSM model was evaluated based on calculating RMSE, NSE, R and R2 value (Table 3). The results obtained from these evaluation criteria revealed that the SDSM performed well for downscaling Tmax and Tmin. The lower RMSE value (2.7–3.6), and higher R (> 0.97) and R2 (0.73–0.97) value for both calibration (1961–1990) and validation (1991–2005) period clearly demonstrate the better suitability of SDSM in simulating daily temperatures. Unlike temperature, the performance for simulating precipitation was less accurate, as the R (0.43–0.59) and R2 (0.23–0.38) values were constantly smaller for both the calibration and validation period. The results, however, can be considered as satisfactory, given the fact that precipitation downscaling is generally more problematic than temperature (Dibike et al. 2008; Chen et al. 2012; Fiseha et al. 2012; Hassan et al. 2014).
Projected change in climate variables
The downscaled temperature projections clearly show an increasing trend in mean annual Tmax in all future time horizons and all scenarios compared to baseline (1981–2010) period. In Vagai basin (Yalutorovsk station), mean annual Tmax under the RCP 2.6 scenario will increase by 2.6 °C in the 2020s and 3.3 °C in the 2050s, which will then decrease to 3.1 °C by the 2080s. It is projected to increase further under RCP 4.5, and RCP 8.5 shows the highest increase for all time windows reaching an increase of 8.6 °C by the end of the century. The projection revealed a similar trend for Loktinka basin (Ishim station) as well. The lowest increase (2.4 °C by the 2080s) will be under RCP 2.6 and the highest (7.4 °C by the 2080s) under RCP 8.5. The values for Tyumen, Kamyshlov and Ekaterinburg station, located in the Pyshma basin, were averaged for the interpretation. The projection for Pyshma basin was similar to that of other basins, showing the possibility of maximum increase by 9.3 °C by the end of the century. In general, the projection illustrated that the increase in annual average maximum temperature will range from 2.4 to 9.3 °C by the end of the century in the selected three catchments.
The monthly deviations in Tmax of the future climate conditions from the baseline period (1981–2010) are shown in Fig. 2. It can be seen that the increase in Tmax will be more prominent in cold seasons, especially from November to February and the smallest increase will occur during spring with slight increases in March and May and even slightly decreasing temperatures in April.
Similar to Tmax, the projection shows an increasing trend of Tmin for all stations. In Vagai, the annual average increase will be 2.9 °C, 3.3 °C and 5 °C under the RCP 2.6, 4.5 and 8.5 by the 2050s, which will increase to 2.9 °C, 4.5 °C and 8 °C by the end of the century. Loktinka will be subject to smaller increases in Tmin compared to Vagai under all scenarios in all future time slices: mean annual Tmin will rise by 2.6 °C, 4.1 °C and 7.7 °C under RCP 2.6, 4.5 and 8.5, respectively, by the end of the century. The highest increase will occur in the Pyshma: by the end of the century, the increment may reach 9 °C under RCP 8.5. Comparable to Tmax, the relative change in Tmin will be different in different months (Fig. 3). While the highest increase in Tmin will be experienced during November to February, there will be consistent decrease in the month of April and May especially in the 2020s, across all stations and under all scenarios. Changes of Tmin are most pronounced in winter in the 2080s and under RCP 8.5.
Unlike temperature variables, the projection of precipitation did not manifest a consistent increase or decrease in all future time slices. In Vagai basin, the model projected a possible decrease in mean daily precipitation in the 2020s under the RCP 4.5 and 8.5, and in the 2050s under RCP 2.6, whereas there will be an increase in precipitation during 2080s under all the scenarios ranging from 0.04 to 0.1 mm/day. In contrast, there will be an increase in precipitation ranging from 0.2 to 0.3 mm/day in the 2020s and the 2050s and 0.3 to 0.4 mm/day by the end of the century in Loktinka basin. Similarly, the mean daily precipitation is projected to increase by 0.2 mm/day in the 2020s, 0.2–0.3 mm/day in the 2050s and 0.3–0.4 mm/day in the 2080s in Pyshma basin as well.
Though the annual mean daily precipitation for these three time slices are projected to increase compared to the baseline period, some months will have increased precipitation and others will experience a decline (Fig. 4). In Vagai basin, all scenarios showed declining precipitation from March till September in all time horizons except for a few months in the 2020s and the 2080s, and an increase is expected from October to February. In contrast, there will be consistent increase in precipitation all year round except in May and September at Loktinka basin. Similar to Vagai, in Pyshma basin, in all scenarios, a consistent decrease is projected from May to August and a significant increase is expected during the remaining cold months leading to an overall increase in precipitation in the basin.
Simulated changes in the hydrological regime
The described changes in temperature and precipitation will lead to changes in streamflow. Figure 5 shows the simulated monthly streamflow at the outlet of the three catchments. In all basins, scenarios and time periods, higher monthly mean streamflow is simulated. In Loktinka, the mean annual streamflow is likely to attain 2.8 m3/s, 2.4 m3/s and 2.8 m3/s under the RCP 2.6, 4.5 and 8.5, respectively, by the 2080s which is 812%, 688% and 804% higher as compared to 0.3 m3/s in the baseline period. The highest increase in mean monthly streamflow during the 2080s is likely to occur in April with 6.2 m3/s and 4.3 m3/s increase under RCP 2.6 and RCP 4.5, respectively, while the lowest increase will occur in January with 0.9 m3/s and 1.1 m3/s under RCP 2.6 and RCP 4.5, respectively. Under RCP 8.5, the increment will range from 0.6 m3/s in May to 4.9 m3/s in November by the 2080s.
Mean annual streamflow is expected to be 27.7 m3/s, 26.3 m3/s and 27.3 m3/s by the 2080s under RCP 2.6, 4.5 and 8.5, respectively, in Vagai which is about 83–111% higher compared to 13.4 m3/s during the baseline period. Under RCP 2.6, the highest (31 m3/s) increment is simulated to occur in October during 2020s, whereas the lowest (4 m3/s) increase will occur during July in the 2050s and 2080s. Under the RCP 4.5 scenario, March is subject to the highest increase (29 m3/s each in the 2020s and 2050s, and 33 m3/s in the 2080s) in mean streamflow, whereas there will be a small decrease (2%) in April by the end of the century. Similarly, RCP 8.5 also shows that there will be a decrease of streamflow in April by 21% and 38% in the 2050s and 2080s, respectively, and in May by 7% in the 2080s. The highest increase will be in March with 24 m3/s, 44 m3/s and 42 m3/s in the 2020s, 2050s and 2080s, respectively under RCP 8.5.
In Pyshma, the mean annual streamflow will increase by 54%, 54% and 57% (107 m3/s, 106 m3/s and 113 m3/s) in the 2020s, 2050s and 2080s, respectively, under RCP 2.6 compared to the baseline flow of 92 m3/s. The highest (195 m3/s) and lowest (34 m3/s) increase will be seen in March and August of the 2050s, respectively. Similarly, the simulation based on RCP 4.5 also shows the highest (236 m3/s) and the lowest (24 m3/s) increase in March and August, respectively, during the 2080s. In general, the mean annual streamflow under this scenario will increase by 106 m3/s, 94 m3/s and 105 m3/s (54%, 48% and 53%) in the 2020s, 2050s and 2080s, respectively, compared to baseline flow. RCP 8.5 also follows this increasing pattern, being subject to the highest (274 m3/s) and the lowest (8.3 m3/s) increase in March and August, respectively, during the 2080s. However, this scenario also shows decreasing streamflow by 47 m3/s (15%) in April of the 2080s, and 14 m3/s (5%) and 107 m3/s (39%) in May of the 2050s and 2080s, respectively.
In general, streamflow is expected to be remarkably higher compared to the baseline period (1981–2010) for all time periods and under all scenarios in Loktinka. Though streamflow is expected to be higher in Vagai and Pyshma basin as well, the increase will not be as pronounced as in the Loktinka. Nevertheless, the changes in annual average discharge in future time slices will always be positive compared to baseline in all basins and during all time windows. From the temporal distribution of flow changes (Fig. 5), it can be seen that the changes in streamflow are mostly due to (1) an earlier snowmelt, which was less intense, but over a longer time period and (2) with a higher groundwater/baseflow contribution over the remaining year.
Discussion
Due to climate change, Siberia has already experienced a significant warming process which is higher than over the average Northern Hemisphere (Groisman et al. 2013) and this change is spatially non-uniform (Shulgina et al. 2011). This increase in temperature is even higher in Western Siberia (Kabanov and Lykosov 2006). Unlike temperature, no significant pattern of change in precipitation has been confirmed. Some researchers found increasing (Serreze et al. 2000; Aizen et al. 2001; Yang et al. 2002; Frey and Smith 2003; Rawlins et al. 2010) and others found decreasing trends (Fallot et al. 1997; Shulgina et al. 2011; Degefie et al. 2014). In these circumstances, what might be the possible future hydrological regimes in Western Siberia? To project the plausible streamflow in the three selected catchments, namely, Loktinka, Vagai and Pyshma, SDSM and SWAT models were used. SDSM was used for projecting the local temperature and precipitation conditions and SWAT was used to project the hydrological components based on the result of SDSM.
Selection of predictor variables
In general, the correlation between the selected predictor variables and daily precipitation was quite low in comparison to daily Tmax and Tmin. This was also found by Dibike and Coulibaly (2005), Gagnon et al. (2005), Dibike et al. (2008) and Hessami et al. (2008). The predictor variables selected in this study for temperature and precipitation are similar to other studies except for relative vorticity of wind selected for precipitation. This may be because of the unique climatic conditions of Western Siberia, where climate is strongly influenced by high-pressure weather systems that bring cold air masses from the Arctic. This predictor is statistically strong as it has always higher explained variance for most months and also Wilby et al. (2002) have selected this predictor (vorticity) based on a high correlation coefficient in their study in Toronto, Canada.
The plausibility of SDSM providing satisfactory downscaled temperature and precipitation data depends on the model calibration which can be described based on the percentage of the explained variance. In this study, the explained variance ranged from 75% to 82% for Tmax, 67% to 79% for Tmin and 19% to 26% for precipitation, which is quite comparable with other similar studies. Previous studies showed that the explained variance ranged from 52 to 59% in Northern Canada to 68–90% in Nagano, Japan, for temperature variables and 16% in Jilin Province, China, to 29–48% in Quebec, Canada, for precipitation (Zhang et al. 2012; Dibike et al. 2008; Hessami et al. 2008; Gagnon et al. 2005; Wilby et al. 1998, 2002). Wilby et al. (2002) stated that obtaining explained that variance above 70% for temperature and below 40% for precipitation is common, as temperature is more spatially homogenous than precipitation and further stated that it is not possible to specify an acceptance level as it varies geographically even for common sets of predictors.
Evaluating the SDSM performance
The results obtained from the statistical analysis are comparable to previous studies dealing with statistical downscaling. Fiseha et al. (2012) obtained R2 values ranging from 0.13 to 0.3 and 0.4 to 0.7 for calibration of precipitation and temperature, respectively, for three stations in Italy. Similarly, Hassan et al. (2014) got R values less than 0.3 and 0.6 for daily precipitation and daily temperature variables during the validation period. Likewise, for daily temperature variables and daily precipitation, Liu et al. (2011) obtained R values of 0.9 and 0.3 and R2 value 0.9 and 0.07 respectively, during validation period. Compared to these findings, the statistical results obtained in this study can be considered as statistically sound. Similarly, based on the visual representations (not shown here), simulated Tmax and Tmin show good agreement with the observed data, and the variances are also well preserved in simulations. Similarly, simulated mean monthly precipitation also shows good agreement with observed data but it could not capture well the rainfall characteristics such as mean wet and dry spell length. However, the result can be assessed as satisfactory considering that several other studies have already pointed out these discrepancies and still have moved forward using the model for downscaling future climate change data (Dibike et al. 2008; Chen et al. 2012; Fiseha et al. 2012; Hassan et al. 2014).
Projection of climatic variables
The climate projections of this study were based on the GCM model CanESM2 from which the three scenarios RCP 2.6, 4.5 and 8.5 were used. The projected increase in mean temperature found in this study (3 °C, 4.6 °C and 8.3 °C under RCP 2.6, 4.5 and 8.5, respectively, by the end of the century) is comparable to previous studies in Western Siberia. The studies by Kabanov and Lykosov (2006) and Frey and Smith (2003) found an increase by 0.5 °C per decade in Western Siberia since the second half of the twentieth century, while Shulgina et al. (2011) found increasing temperatures as high as 0.8–1.6 °C per decade from 1991 to 2007. Due to this increasing trend, Frey and Smith (2003) denote Western Siberia as a region of amplified warming. The projected increasing temperature can be contributed primarily to the winter temperature changes from November to February which matches with findings by Shulgina et al. (2011). Groisman et al. (2013) found that the annual mean surface air temperature of Siberia may increase by 3–5 °C by the end of the century and it is likely to increase even further to 7–9 °C in the polar regions, similar to our study, also due to strong increases of winter temperatures. Miao et al. (2014) also used the RCP 2.6, RCP 4.5 and RCP 8.5 scenarios and found an increase of surface air temperature of 1.0 °C, 3.1 °C and 7.1 °C, respectively, by the end of the century, which is in agreement with our study. They also found an accelerated warming trend with increasing latitude which is also true based on our findings; however, we cannot confirm if it is due to latitudinal differences or it is just a coincidence. It is of note here that Pyshma is located in a higher latitude, followed by Vagai and Loktinka and the projections showed the possibility of the highest increase of temperature in Pyshma followed by Vagai and Loktinka.
The projected precipitation change over Siberia is spatially non-uniform and has no significant spatial pattern. Some researchers have found an increasing trend (Serreze et al. 2000; Aizen et al. 2001; Yang et al. 2002; Groisman et al. 2013) and others a decreasing trend (Shulgina et al. 2011), where changes are temporally non-uniform and different in different seasons and months depending on the methods adopted, data used and spatial scale of the study. This study revealed that in the Vagai basin, autumn and winter precipitation is expected to increase, whereas spring and summer precipitation will decrease. Loktinka basin will receive overall higher precipitation in all future time periods and under all scenarios. In contrast, Pyshma basin will receive significantly less precipitation during summer and more during the other seasons. Similar to the findings in the Vagai basin, Serreze et al. (2000) also found an increase in autumn and winter precipitation in the region where the selected sites of this study are located. Similarly, Frey and Smith (2003) and Rawlins et al. (2010) also found an increase in cold season precipitation over most of Siberia. Other studies which also found an increasing trend of precipitation in the study region are those by Aizen et al. (2001); Yang et al. (2002) and Groisman et al. (2013). However, Shulgina et al. (2011) did not find any pronounced changes of winter precipitation in Siberia, but their finding of decreasing summer precipitation in Western Siberia is in agreement with the result of our study. Degefie et al. (2014) concluded that there is no significant trend of precipitation that is consistent across the Western Siberian lowlands, in line with our study which revealed different patterns of precipitation change in different catchments. The most important finding of Groisman et al. (2013) regarding the projection of precipitation is that the precipitation increase during winter will be intensified by the end of the century. Though not as strong as in winter, this increase will also be seen in the other seasons, which is also one of the findings of our study.
The inconsistencies in variation of precipitation over time and space may be due to the inherent characteristics of the CanESM2 model used in this study. A study by Liu et al. (2014) on seasonal and regional biases in CMIP5 precipitation simulations revealed that most of the CMIP5 models underestimate summer precipitation and overestimate winter precipitation, especially in Siberia and central Europe. Similarly, Mehran et al. (2014) also found that though most of the CMIP5 simulations are in good agreement with data from the Global Precipitation Climatological Project in many regions, they have problems in Northern Eurasia including some other regions.
Projection of hydrological regime
Snowmelt and precipitation play a major role in river discharge in this region. The characteristic features of river flow in this region are spring high flow period, summer–autumn low flow period interrupted by rainfall-induced flood events and very low winter flow period when rivers are mostly frozen or fed by groundwater flow (Zemtsov et al. 2014). Most of the studies (Peterson et al. 2002; Yang et al. 2004; Smith et al. 2007; Shiklomanov et al. 2013) on hydrological change in the Siberian region show increasing trends of river discharge. This study also showed a projected increase in mean annual streamflow. However, the change in monthly mean will be different for different months and also different for different catchments. Also Zemtsov et al. (2014) found spatial and temporal heterogeneity of projected changes in the hydrology of the region. Peterson et al. (2002), Yang et al. (2004) and Dai et al. (2009) have pointed out that this increase in streamflow is associated with climate change. The study by Dai et al. (2009) revealed that the increase in temperature causes melting of snow, contributing to increase in runoff and thus a decrease in surface snow cover especially over Siberia, which is true based on the result of our study as well. If snowmelt occurs faster due to higher temperatures, less water infiltrates into the soil and less water can be stored there. This will reduce evaporation from the soil, causing higher runoff volumes. Generally, the contribution of surface runoff is significant only during late winter and spring during snowmelt. Though the spring snowmelt will decrease compared to the baseline period at all the basins due to the decrease in snowfall itself, there will be consistent increase in winter snowmelt when there is sufficient snow cover to melt; however, a decrease in snowfall (Serreze et al. 2000; Bulygina et al. 2011) has also been reported by several researchers in Western Siberia. Khon and Mokhov (2012) and Shiklomanov et al. (2013) also found a possible increase in mean annual runoff of Siberian rivers by the end of the twenty-first century due to an increase in winter precipitation and spring snowmelt.
Conclusion and recommendation
Climate change is an indispensible issue to be dealt with. The global mean temperatures have been increasing rapidly and the change in precipitation has become more irregular and unpredictable. These changes and the resulting impacts are more pronounced in the northern high latitudes. Thus, these changes have critical implications on Siberia, as mentioned in Sect. “Introduction” above. The selected three river catchments which belong to the southern part of the Western Siberian lowland (WSL) region are not excluded from these challenges and hence this study aims to understand the possible future hydrological regime shift in the region. The study area is an important region for grain production in Siberia and the entire Russian Federation, so any changes in temperature, precipitation and water availability may result in significant stress on agricultural production, impacting adversely on the food security and economic development.
The statistical downscaling model projected a significant increasing trend of temperature variables. The precipitation is projected to be different in different seasons, different time slices and under different scenarios in the Pyshma and Vagai basin. The Loktinka basin will experience increased mean annual precipitation and mean seasonal precipitation under all scenarios and during all future time horizons. Hydrological simulations showed a likely increase in streamflow compared to baseline in all basins and during all time windows, with high relative streamflow increase in Loktinka basin, followed by Vagai and Pyshma basin. The reason is that the reduction in precipitation that is predicted for some stations in the summer will not impact streamflow, because the high evaporation pressure, high water storage capacity of the soils and low hydraulic gradients hamper streamflow. Therefore, the increase in streamflow can be attributed to the higher precipitation in autumn and winter, when evaporation is low and soil and groundwater storages are replenished. This leads to more snow and groundwater storage and contribution of groundwater flow to the streams during spring and summer.
In general, the results obtained in this study show good agreement with the findings of other research studies. The presented simulations of future hydrological regime do not incorporate the effects of land use and land cover change and, hence, if data may become available, it is suggested to incorporate both land use land cover change and climate change in future studies to obtain more robust predictions. In addition, it is highly recommended to use multiple downscaling techniques and multiple GCMs outputs to enhance the plausibility of the downscaling and climate change projection results. Due to different algorithms and boundary conditions used, applying multiple GCM offers the change to assess the uncertainty involved in climate change projections. The results of this study, hence, represent one possible climate change pathway, which projected the possible changes in temperature and precipitation, and hydrological regime shift, based on the three latest climate scenarios adopted by the IPCC fifth assessment report. The results can be used as broad figures for further planning and investigation of local level climate change impacts.
References
Aizen EM, Aizen VB, Melack JM, Nakamura T, Ohta T (2001) Precipitation and atmospheric circulation patterns at mid-latitudes of Asia. Int J Climatol 21(5):535–556. https://doi.org/10.1002/joc.626
Arnold JG, Fohrer N (2005) SWAT2000: current capabilities and research opportunities in applied watershed modelling. Hydrol Process 19(3):563–572. https://doi.org/10.1002/hyp.5611
Arnold JG, Srinivasan R, Muttiah RS, Williams JR (1998) Large area hydrologic modeling and assessment part i: model development. J Am Water Resour Assoc 34(1):73–89. https://doi.org/10.1111/j.1752-1688.1998.tb05961.x
Arora VK, Boer GJ (2014) Terrestrial ecosystems response to future changes in climate and atmospheric CO2 concentration. Biogeosciences 11(15):4157–4171
Bhuvandas N, Timbadiya PV, Patel PL, Porey PD (2014) Review of downscaling methods in climate change and their role in hydrological studies. Int J Environ Ecol Geol Mar Eng 8(10):648–653
Bulygina ON, Groisman PY, Razuvaev VN, Korshunova NN (2011) Changes in snow cover characteristics over Northern Eurasia since 1966. Environ Res Lett 6(4):45204. https://doi.org/10.1088/1748-9326/6/4/045204
Chen H, Xu C-Y, Guo S (2012) Comparison and evaluation of multiple GCMs, statistical downscaling and hydrological models in the study of climate change impacts on runoff. J Hydrol 434–435:36–45. https://doi.org/10.1016/j.jhydrol.2012.02.040
Dai A (2011) Drought under global warming: a review. Wiley Interdiscip Rev Clim Change 2(1):45–65. https://doi.org/10.1002/wcc.81
Dai A (2012) Increasing drought under global warming in observations and models. Nature Clim Change 3(1):52–58. https://doi.org/10.1038/nclimate1633
Dai A, Qian T, Trenberth KE, Milliman JD (2009) Changes in continental freshwater discharge from 1948 to 2004. J Clim 22(10):2773–2792. https://doi.org/10.1175/2008JCLI2592.1
Degefie DT, Fleischer E, Klemm O, Soromotin AV, Soromotina OV, Tolstikov AV, Abramov NV (2014) Climate extremes in South Western Siberia: past and future. Stoch Env Res Risk Assess 28(8):2161–2173. https://doi.org/10.1007/s00477-014-0872-9
Dibike YB, Coulibaly P (2005) Hydrologic impact of climate change in the Saguenay watershed: comparison of downscaling methods and hydrologic models. J Hydrol 307(1–4):145–163. https://doi.org/10.1016/j.jhydrol.2004.10.012
Dibike YB, Gachon P, St-Hilaire A, Ouarda TBMJ, Nguyen VTV (2008) Uncertainty analysis of statistically downscaled temperature and precipitation regimes in Northern Canada. Theoret Appl Climatol 91(1–4):149–170. https://doi.org/10.1007/s00704-007-0299-z
Fallot J-M, Barry RG, Hoogstrate D (1997) Variations of mean cold season temperature, precipitation and snow depths during the last 100 years in the former Soviet Union (FSU). Hydrol Sci J 42(3):301–327. https://doi.org/10.1080/02626669709492031
Feng S, Hu Q, Huang W, Ho CH, Li R, Tang Z (2014) Projected climate regime shift under future global warming from multi-model, multi-scenario CMIP5 simulations. Global Planet Change 112:41–52. https://doi.org/10.1016/j.gloplacha.2013.11.002
Fiseha BM, Melesse AM, Romano E, Volpi E, Fiori A (2012) Statistical downscaling of precipitation and temperature for the upper tiber basin in central Italy. Int J Water Sci 1(3):1–14. https://doi.org/10.5772/52890
Frey KE, Smith LC (2003) Recent temperature and precipitation increases in West Siberia and their association with the Arctic Oscillation. Polar Res 22(2):287–300. https://doi.org/10.1111/j.1751-8369.2003.tb00113.x
Gagnon S, Singh B, Rousselle J, Roy L (2005) An application of the statistical downscaling model (SDSM) to simulate climatic data for streamflow modelling in Québec. Can Water Resour J 30(4):297–314. https://doi.org/10.4296/cwrj3004297
Gassman PW, Reyes MR, Green CH, Arnold JG (2007) The soil and water assessment tool: historical development, applications, and future research directions. Trans ASABE 50(4):1211–1250. https://doi.org/10.13031/2013.23637
Groisman PY, Blyakharchuk TA, Chernokulsky AV, Arzhanov MM, Marchesini LB, Bogdanova EG, Vygodskaya NN (2013) Climate changes in Siberia. In: Groisman PY, Gutman G (eds) Regional environmental changes in siberia and their global consequences (pp 57–109). Springer Environmental Science and Engineering, Berlin. https://doi.org/10.1007/978-94-007-4569-8_3
Gupta HV, Kling H, Yilmaz KK, Martinez GF (2009) Decomposition of the mean squared error and NSE performance criteria: implications for improving hydrological modelling. J Hydrol 377(1–2):80–91
Guse B, Kail J, Radinger J, Schröder M, Kiesel J, Hering D, Wolter C, Fohrer N (2015) Eco-hydrologic model cascades: Simulating land use and climate change impacts on hydrology, hydraulics and habitats for fish and macroinvertebrates. Sci Total Environ 533:542–556
Hassan Z, Shamsudin S, Harun S (2014) Application of SDSM and LARS-WG for simulating and downscaling of rainfall and temperature. Theoret Appl Climatol 116(1–2):243–257. https://doi.org/10.1007/s00704-013-0951-8
Hessami M, Gachon P, Ouarda TBMJ, St-Hilaire A (2008) Automated regression-based statistical downscaling tool. Environ Model Softw 23(6):813–834. https://doi.org/10.1016/j.envsoft.2007.10.004
Htut AY (2014) Forecasting climate change scenarios in the Bago River Basin, Myanmar. J Earth Sci Clim Change 5:9. https://doi.org/10.4172/2157-7617.1000228
Hu Y, Maskey S, Uhlenbrook S (2013) Downscaling daily precipitation over the Yellow River source region in China: a comparison of three statistical downscaling methods. Theoret Appl Climatol 112(3–4):447–460. https://doi.org/10.1007/s00704-012-0745-4
IPCC (2013) Summary for policymakers. In: Stocker TF, Qin D, Plattner G-K, Tignor M, Allen SK, Boschung J, Nauels A, Xia Y, Bex V, Midgley PM (eds) Climate Change 2013: the physical science basis. Contribution of working group I to the fifth assessment report of the intergovernmental panel on climate change. Cambridge University Press, Cambridge
Jarvis A, Reuter HI, Nelson A, Guevara E (2008) Hole-Filled Seamless SRTM Data V4. International Centre for Tropical Agriculture (CIAT) [online] http://srtm.csi.cgiar.org/. Accessed May 2013
Kabanov MV, Lykosov VN (2006) Monitoring and modeling of climatic changes in Siberia. Atmos Ocean Opt 19(9):675–685
Kalnay E, Kanamisu M, Kistler R, Collins W (1996) The NCEP/NCAR 40 year reanalysis project. BAMS 77:437–472
Khon VC, Mokhov II (2012) The hydrological regime of large river basins in Northern Eurasia in the XX–XXI centuries. Water Resour 39(1):1–10. https://doi.org/10.1134/S0097807812010058
Kiesel J, Fohrer N, Schmalz B, White MJ (2010) Incorporating landscape depressions and tile drainages of a northern German lowland catchment into a semi-distributed model. Hydrol Process 24(11):14721486. https://doi.org/10.1002/hyp.7607
Kiesel J, Pfannerstill M, Schmalz B, Khoroshavin V, Sheldukov A, Veshkurseva T, Fohrer N (2018) Modelling of hydrological processes in snowmelt-governed permafrost-free catchments of the Western Siberian Lowlands. Int J Hydrol Sci Technol 8(3):289–316. https://doi.org/10.1504/IJHST.2018.10007182
Kiesel J, Gericke A, Rathjens H, Wetzig A, Kakouei K, Jähnig SC, Fohrer N (2019) Climate change impacts on ecologically relevant hydrological indicators in three catchments in three European ecoregions. Ecol Eng 127:404–416
Liu L, Liu Z, Ren X, Fischer T, Xu Y (2011) Hydrological impacts of climate change in the Yellow River Basin for the 21st century using hydrological model and statistical downscaling model. Quatern Int 244(2):211–220. https://doi.org/10.1016/j.quaint.2010.12.001
Liu Z, Mehran A, Phillips TJ, Aghakouchak A, Res C, Liu Z, Aghakouchak A (2014) Seasonal and regional biases in CMIP5 precipitation simulations. Clim Res 60(1):35–50. https://doi.org/10.3354/cr01221
Marvel K, Bonfils C (2013) Identifying external influences on global precipitation. Proc Natl Acad Sci USA 110(48):19301–19306. https://doi.org/10.1073/pnas.1314382110
MDHS (1961–1988) Hydrological yearbooks. Main Department of the Hydrometeorological Service, Council of Ministers, Russia
Mehran A, Aghakouchak A, Phillips TJ (2014) Evaluation of CMIP5 continental precipitation simulations relative to satellite-based gauge-adjusted observations. J Geophys Res Atmos 119(4):1695–1707. https://doi.org/10.1002/2013JD021152
Miao C, Duan Q, Sun Q, Huang Y, Kong D, Yang T, Gong W (2014) Assessment of CMIP5 climate models and projected temperature changes over Northern Eurasia. Environ Res Lett 9(5):55007. https://doi.org/10.1088/1748-9326/9/5/055007
Min S-K, Zhang X, Zwiers F (2008) Human-induced Arctic moistening. Science 320(5875):518–520. https://doi.org/10.1126/science.1153468
Min S-K, Zhang X, Zwiers FW, Hegerl GC (2011) Human contribution to more-intense precipitation extremes. Nature 470(7334):378–381. https://doi.org/10.1038/nature09763
Moriasi DN, Arnold JG, Liew MW, Van Bingner RL, Harmel RD, Veith TL (2007) Model evaluation guidelines for systematic quantification of accuracy in watershed simulations. Am Soc Agric Biol Eng 50(3):885–900. https://doi.org/10.13031/2013.23153
NOAA (2013) National Climatic Data Center, Precipitation and Temperature Data, National Oceanic and Atmospheric Administration [online] http://www.ncdc.noaa.gov/. Accessed Sept 2014
Omernik JM, Bailey RG (1997) Distinguishing Between Watersheds and Ecoregions. J Am Water Resour Assoc 33(5):935–949
Peterson BJ, Holmes RM, McClelland JW, Vörösmarty CJ, Lammers RB, Shiklomanov AI, Rahmstorf S (2002) Increasing river discharge to the Arctic Ocean. Science 298(5601):2171–2173. https://doi.org/10.1126/science.1077445
Pfannerstill M, Guse B, Fohrer N (2014) A multi-storage groundwater concept for the SWAT model to emphasize nonlinear groundwater dynamics in lowland catchments. Hydrol Processes 28(22):5599–5612
Pfannerstill M, Guse B, Reusser D, Fohrer N (2015) Process verification of a hydrological model using a temporal parameter sensitivity analysis. Hydrol Earth Syst Sci 19(10):4365–4376
Radojevic M (2014) The ensemble of daily predictor variables developed from the CanESM2 CMIP5 experiments. Canadian Centre for Climate Modelling and Analysis (CCCma), Canada
Rawlins M, Steele M, Holland MM, Adam JC, Cherry JE, Francis J, Zhang T (2010) Analysis of the Arctic system for freshwater cycle intensification: observations and expectations. J Clim 23(21):5715–5737. https://doi.org/10.1175/2010JCLI3421.1
SASCHA (2014) Annual report: SASCHA-sustainable land management and adaptation strategies to climate change for the Western Siberian Corn belt. Muenster, Germany
Serreze MC, Walsh JE, Chapin FS III, Osterkamp T, Dyurgerov M, Romanovsky V, Barry RG (2000) Observational evidence of recent change in the Northern high-latitude environment. Clim Change 46:159–207. https://doi.org/10.1023/A:1005504031923
Shiklomanov AI, Lammers RB, Lettenmaier DP, Polischuk YM, Savichev OG, Smith LC, Chernokulsky AV (2013) Regional environmental changes in Siberia and their global consequences (Google eBook). In: Groisman PY, Gutman G (eds) Regional environmental changes in Siberia and their global consequences (pp 111–154). Springer Environmental Science and Engineering, Berlin. https://doi.org/10.1007/978-94-007-4569-8
Shulgina TM, Genina EY, Gordov EP (2011) Dynamics of climatic characteristics influencing vegetation in Siberia. Environ Res Lett 6(4):45210. https://doi.org/10.1088/1748-9326/6/4/045210
Smith LC, Pavelsky TM, MacDonald GM, Shiklomanov AI, Lammers RB (2007) Rising minimum daily flows in northern Eurasian rivers: a growing influence of groundwater in the high-latitude hydrologic cycle. J Geophys Res 112(G4):G04S47. https://doi.org/10.1029/2006JG000327
Viñas M-J (2014) NASA: four decades of Arctic sea ice from space. http://www.reportingclimatescience.com/news-stories/article/nasa-four-decades-of-arctic-sea-ice-from-space.html. Accessed 8 July 2015
von Storch H, Hewitson B, Mearns L (2000) Review of empirical downscaling techniques. In: Regional climate development under global warming. General Technical Report No. 4, Conference Proceedings, Torbjornrud, Norway
Wilby RL, Hassan H, Hanaki K (1998) Statistical downscaling of hydrometeorological variables using general circulation model output. J Hydrol 205(1–2):1–19. https://doi.org/10.1016/S0022-1694(97)00130-3
Wilby RL, Dawson CW, Barrow EM (2002) SDSM—A decision support tool for the assessment of regional climate change impacts. Environ Model Softw 17(2):147–159. https://doi.org/10.1016/S1364-8152(01)00060-3
Wilby RLL, Charles SPP, Zorita E, Timbal B, Whetton P, Mearns LOO (2004) Guidelines for use of climate scenarios developed from statistical downscaling methods. Prepared for consideration by the IPCC at the request of its Task Group on Data and Scenario Support for Impacts and Climate Analysis (TGICA)
WWF (2014) The Terrestrial Ecoregions of the World Base Global Dataset. World Wildlife Fund Conservation Science Data and Tools [online] http://www.worldwildlife.org/pages/conservation-science-data-and-tools. Accessed Sept 2014
Yang D, Kane DL, Hinzman LD, Zhang X, Zhang T, Ye H (2002) Siberian Lena River hydrologic regime and recent change. J Geophys Res Atmos 107(23):1–10. https://doi.org/10.1029/2002JD002542
Yang D, Ye B, Shiklomanov A (2004) Discharge characteristics and changes over the Ob river watershed in Siberia. J Hydrometeorol 5(4):595–610. https://doi.org/10.1175/1525-7541(2004)005%3C0595:DCACOT%3E2.0.CO;2
Yao T, Thompson L, Yang W, Yu W, Gao Y, Guo X, Joswiak D (2012) Different glacier status with atmospheric circulations in Tibetan Plateau and surroundings. Nature Clim Change 2(9):663–667. https://doi.org/10.1038/nclimate1580
Zemtsov Va, Paromov VV, Kopysov SG, Kouraev aV, Negrul SV (2014) Hydrological risks in Western Siberia under the changing climate and anthropogenic influences conditions. Int J Environ Stud 71(5):611–617. https://doi.org/10.1080/00207233.2014.942530
Zhang L, Lu W, Yang Q, An Y, Li D, Gong L (2012) Hydrological impacts of climate change on streamflow of Dongliao River watershed in Jilin Province, China. Chin Geogr Sci 22(5):522–530
Zuo D, Xu Z, Zhao J, Abbaspour KC, Yang H (2015) Response of runoff to climate change in the Wei River basin, China. Hydrol Sci J. https://doi.org/10.1080/02626667.2014.943668
Acknowledgements
This work was conducted as part of project SASCHA (Sustainable land management and adaptation strategies to climate change for the Western Siberian grain belt). We are grateful for funding by the German Government, Federal Ministry of Education and Research within their Sustainable Land Management funding framework (funding reference 01LL0906C). JK acknowledges funding through the “GLANCE” project (Global change effects on river ecosystems; 01LN1320A) supported by the German Federal Ministry of Education and Research (BMBF). Further thanks go to our Russian partners of Tyumen State University (TSU) and State Agrarian University of the Northern Transurals (GAUSZ) for a great and successful cooperation. We would also like to thank Dr. D.T. Degefie, Dr. Laurent Terray and Ms. Milka Radojevic for their guidance in statistical downscaling and Dr. Matthias Pfannerstill for valuable discussions and support regarding SWAT-3S.
Author information
Authors and Affiliations
Corresponding author
Additional information
Publisher’s Note
Springer Nature remains neutral with regard to jurisdictional claims in published maps and institutional affiliations.
This article is a part of a Topical Collection in Environmental Earth Sciences on Climate Effects on Water Resources, edited by Drs. Zongzhi Wang and Yanqing Lian.
Rights and permissions
About this article
Cite this article
Sada, R., Schmalz, B., Kiesel, J. et al. Projected changes in climate and hydrological regimes of the Western Siberian lowlands. Environ Earth Sci 78, 56 (2019). https://doi.org/10.1007/s12665-019-8047-0
Received:
Accepted:
Published:
DOI: https://doi.org/10.1007/s12665-019-8047-0