Abstract
Land use patterns are changing and the environment has become more vulnerable in an agro-pastoral ecotone in Inner Mongolia. Modeling studies can be done through studying the relationships between the soil organic carbon density (SOCD) and the influencing factors in differing land uses such as cropland, forest and grassland. Such studies can provide the basis for predicting soil organic carbon (SOC) stocks and using land resources efficiently. Therefore, the relationships between the SOCD of surface soils and the influencing factors, such as soil type, slope and elevation, were selected for a study in differing land uses of Duolun County, Inner Mongolia. The results showed that: (1) The variation in SOCD among the soil types followed the same order in the three land uses: chernozems > meadow soils > bog soils > castanozems > gray-cinnamon soils > eolian soils. The sensitivity of SOCD to land use change was different for the six soil types. Both the degradation of grassland and the application of the government policy of returning forest from cropland induced the greatest variation in SOCD. (2) SOCD correlated significantly with soil type and elevation in each land use. There was a significant correlation between SOCD and slope in the forest. (3) Statistical models showing SOCD and the influencing factors were developed and provided the basis for predicting SOC stocks in Duolun County, Inner Mongolia.
Similar content being viewed by others
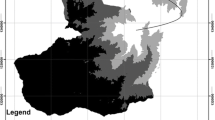
Explore related subjects
Discover the latest articles, news and stories from top researchers in related subjects.Avoid common mistakes on your manuscript.
Introduction
The terrestrial carbon cycle plays a vital role in the global carbon cycle. Soil organic carbon (SOC) is the largest terrestrial carbon pool and there is about three times as much carbon stored in the aboveground biomass, and twice as much as that in the atmosphere (Batjes and Sombroek 1997). Minor fluctuations in SOC can produce an important impact on the concentration of greenhouse gases (Bruce et al. 1999). SOC stocks mainly depend on the balance between the inputs (plant residues) and outputs (decomposition of organic material, mineralization process, crop mowing, soil erosion) (Wang et al. 2008; Schaefer et al. 2009), which are affected by both soil-forming factors and anthropogenic factors. Soil-forming factors include climate, time, parent material, vegetation and landforms. Here, anthropogenic factors mainly refer to changes in land use and the influence of agricultural activities (Dupouey et al. 2002).
There has been a considerable amount of research on the relationships between SOC content and the influencing factors. Post et al. (1982) simulated SOC stocks by considering vegetation and climate based on the Holdridge life zone model. Grigal and Ohman (1992) found that SOC varies with annual precipitation, forest type and clay content. Mueller and Pierce (2003) found significant relationships between SOC and elevation. Liu et al. (2006) explored the spatial distribution of SOC and the relationships between SOC and the influencing factors, such as land use, topography and soil type. In these studies, SOC stocks were predicted by statistical models, which were developed. The soil-forming factors were always taken into consideration as the influencing factors, while anthropogenic factors were often ignored. In fact, these factors will affect the content and distribution of SOC directly or indirectly through changing the rate of decomposition of soil organic matter. These changes, such as land use, will significantly affect the carbon cycles on different scales (Kirschbaum 2000; Lal 2002). However, little research has involved the study of the variation of SOC stocks with the influencing factors in differing land uses.
An agro-pastoral ecotone refers to a compound ecosystem, which has special characteristics in the natural environment, ecological characteristics and social economy. It has an obvious edge effect because it is formed through the interaction of two adjacent agricultural and pastoral ecosystems. It is more sensitive to human disturbance and can be regarded as the representative region, which can reflect the environment status of larger scale (Qiao, 2007). Desertification results from serious land deterioration due to excessive cultivation and use of grassland, which can lead to grassland degradation and yield reduction. These problems have restricted the development of the local social economy and caused a decline in the environmental quality of the surrounding area (Han et al. 2004). The study area, Duolun County of Inner Mongolia, southeast of Xilingol League, is a typical agro-pastoral region and represents 3,773 km2 in total land area (Fig. 1). Cropland and grassland coexist because of the effects of the natural environmental conditions, its unique history and human impacts. Grassland is the main original land use type, but the area of the cropland has expanded quickly since the 1950s. More recently, the local government and residents have owned the land and there has been unlimited control of its use in the county since the economic reforms and open policy in 1978. More and more grassland has been converted to cropland or has been over-grazed. The area of indigenous grassland has decreased rapidly and has become degraded in quality, and soil erosion and desertification have occurred to a large extent. The area of degraded grassland in Duolun County has reached 11.2 × 104 hm2, about 86% of the total. The area of sandy and desertification lands has also increased from 10.67 × 104 to 33.65 × 104 hm2 since the 1970s to about 89.2% of the total area of grassland (Baoyin and Bai 2004). The local government has been made aware of the severity of environment changes. Since then, ecological restoration, such as excluding grazing and restoring forest or grassland from cropland, have been practiced to deal with the environmental problems caused by intensive land use. Are SOC stocks of Duolun County increasing or decreasing with the frequent land use changes and the policy of returning cropland to forest and grassland? It is significant to estimate the SOC stocks, predict the trend and rate of SOC change and simulate the carbon cycle through analyzing the relationships between the SOCD and the influencing factors of agro-pastoral ecotone in differing land uses.
Currently, most research has been performed with regard to the mechanisms involved in the carbon cycle in agro-pastoral ecotone in the north of China. Fewer studies have focused on the relationships between SOC content and the influencing factors and on the estimation of the SOC content in Duolun County. The objectives of this study were to: compare SOCD at depths of 0–30 cm in differing land uses and explore the results of ecological restoration and structure regulation on land uses; clarify relationships between SOCD and the influencing factors and evaluate the contribution of each factor to SOCD variation at depths of 0–30 cm in the differing land uses; and to develop the statistical models of SOCD and the influencing factors, which can eventually be used to estimate the SOC stocks for Duolun County.
Materials and methods
Study area
The study was conducted in Duolun County of Inner Mongolia of latitude 41°46′E–42°36′N, longitude 115°51′E–116°54′E. This region belongs to a typical semiarid monsoon climate. The long-term mean annual temperature is 1.6°C and the mean annual precipitation is 385.5 mm. Most (67%) of the total annual precipitation falls in summer. Based on the Chinese Soil Taxonomy, the soil types of the county can be classified as: gray-cinnamon soils, chernozems, castanozems, meadow soils, bog soils, eolian soils and meadow solonchaks. The main soil type is castanozems, which comprises 70% of the whole area. The meadow solonchaks were not considered in later analysis due to their small distribution. The vegetation consists predominantly of grassland plants such as Leymus chinensis (Trin.) Tzve1., Stipa krylovii Roshev., Artemisia frigida Willd., Setaria viridis Beauv. and Melilotoides ruthenica (L.) Sojak.
The study area is located on the south edge of the Inner Mongolia Plateau. The landform is dominated by a low mountain knap, with an elevation of 1,150 ~ 1,800 m. There are river and ditch valleys, which form a mosaic pattern. Volcanic lava and pyroclastic rocks and tuff are widely found. Faults and crush zones have developed widely in this county; these are easily weathered and provide some of the source material for land desertification.
Data sources and processing
To analyze the relationships between SOCD and the influencing factors efficiently, a stratified sampling approach was employed. Soil type, land use type, slope and elevation were used as variables for the procedure.
According to the average SOC content of each soil type, a digital number from 1 to 6 was each assigned to eolian soils, gray-cinnamon soils, castanozems, meadow soils, bog soils and chernozems, respectively.
Grassland, cropland and other land use types contributed to one-third of the land area, respectively. The area of cropland and sandy soils was greater than that of the forest (Baoyin et al. 2000). Cropland and grassland always convert from one to the other frequently in the study area, the same as the inter-conversions that occur between forest and cropland. Therefore, the effects of the conversions on the three land use types on SOC stocks of Duolun County were considered. Cropland, forest and grassland were scored from 1 to 3.
Fields with a slope of more than ten were converted to grassland and forest since the government policy of returning cropland to forest or grassland was enacted. Based on the local topographic features, the slope gradient was grouped into three classifications: 0°–10°, 10°–20° and over 20°, and were scored from 1 to 3. Slope gradients were measured using a compass.
The sampling sites were orientated by a portable Global Positioning System. An altitude of <1,200 m was assigned 1, and other grades of elevation were increased by 1 for every 100 m increment in altitude.
Six soil types, three land use types, three slope gradient classes and six elevation classes were graded for the study area. These classes were combined for being stratified.
Soil sampling and laboratory analyses
Soil samples were taken in August 2009. Three 30 × 30 m plots were established on each stratified environment. Soil samples were collected from five random locations at a depth of 30 cm and bulked for the same plot using a 50-mm diameter soil sampler. Finally, 291 composite soil samples were collected for measurements of soil physical and chemistry properties.
Soils were air dried, gently crushed and passed through a 2-mm sieve. SOC content was determined using the dichromate oxidation method (external heat applied) (Nelson and Sommers 1982). The soil bulk density was measured with a cylinder carefully pushed horizontally into the soil profiles, the volume of which was 100 cm3.
Calculation of SOCD
SOC density refers to SOC mass per unit area for a given depth. It has been a significant index to evaluate SOC stocks because it is calculated based on the volume of a pedon, while the effect of area was excluded. The SOCD of the ith layer was calculated as follows (Batjes 1996; Schwartz and Namri 2002):
Where,
- SOCD i :
-
SOC density of the horizon (kg m−2);
- C i :
-
SOC content of the horizon (%);
- D i :
-
bulk density (g cm−3);
- E i :
-
thickness of the ith horizon (cm);
- G i :
-
volumetric percentage of the fraction >2 mm (rock fragments) in the layer i (%)
Statistical analysis
Statistical analysis was performed using SPSS 13.0 (Zhang and Yan 2004). Post hoc test with Fisher’s least significant difference (LSD) tests were used to analyze the variation in SOCD in the differing land use and soil types. Analysis of variance (ANOVA) was used to analyze the variation in SOCD from the influencing factors, which include soil type, slope and elevation. Means were considered to be significantly different when p < 0.05.
Canonical correlation analysis (CCA) was used to explain the contribution of each influencing factor to SOCD variation (Tan et al. 2003). Using the CCA method involved trying to find one or a few combined variables which could represent the original ones, and using the relationships of a few pairs of combined variables to replace that of the original variables (Zhang and Yan 2004). In this study, SOCD is the first set of variables, and the influencing factors were the second set variables, such as soil type, slope and elevation. The canonical variables of set 1 and 2 represented the linear combination of the original values.
Multiple linear regression analysis was used to develop the statistical models for SOCD and the influencing factors. Goodness of fit and multi-co-linearity tests were also performed.
Results and analysis
Variation of SOCD in differing land use types and soil types
The SOCD in the upper 30 cm of the soils in Duolun County varied widely with soil types (Table 1). For all the soil samples, the order of SOCD from highest to lowest was: chernozems > meadow soils > bog soils > castanozems > gray-cinnamon soils > eolian soils. The SOCD of eolian soils was only 27.6% of that of the chernozems. The SOCD decreased in a similar manner amongst the soil types in differing land use groups for all the soil samples (Table 1). However, the SOCD differed in the land use groups for each soil type (p < 0.05, Fig. 2). The variation of meadow soil SOCD in differing land use types was significantly different. The SOCD in the grassland was the highest in meadow soils, while that in the forest was the lowest. It was very high for SOC content in chernozems, and the variation of chernozems SOCD in differing land use types was not significant. The variation of SOCD between grassland and cropland was not significant in castanozems. Eolian soils always had the lowest SOCD in each land use type. The SOCD in the cropland was higher than that in the forest and grassland in eolian soils. The SOCD in the forest was a little higher than that in the grassland in chernozems, castanozems and eolian soils, which are the three mainly distributed soil types in Duolun County, but the variation (in SOCD) between forest and grassland was not significant.
ANOVA
The contributions of the individual controlling variables were different within differing land use types (Table 2). The influence of soil type was much larger than the other two factors in each land use, with the contribution of slope being the lowest. Elevation was important next to soil type in the forest and cropland.
Most of the interactions produced significant impacts on SOCD (p < 0.05). However, the interaction between soil type and slope was not significant in the croplands. The effect of each controlling variable on SOCD is shown in Table 3. The contribution of all the controlling variables to explain the variation in SOCD was 60.4, 47.2 and 56.0% in the three land use types, respectively.
CCA
Correlation between the two sets of original variables
Soil type and elevation were important regulators of SOCD at all the sites (p < 0.01) apart from the slope in Table 4, and were consistent with the results shown in Table 2. Only in the forest did the SOCD correlated with slope significantly.
Canonical correlations
The expressions for the canonical variable for set 1 in differing land use types are shown below (standardized canonical coefficients were used as the numerical values):
A negative correlation was found between each influencing factor and the canonical variables for set 1 in the expressions 1, 2 and 3, except between slope and the canonical variable of set 1 for the cropland. However, it should be pointed out that a positive or negative sign was dependent on the value of the influencing factors, which were assigned in ascending or descending order. The canonical variable for set 1 was closely correlated with soil types, but not with slope and elevation in all the land uses. There was a closer correlation between the canonical variable for set 1 and elevation in the grassland and forest, but not between the canonical variable for set 1 and slope.
Canonical redundancy analysis can explain the contribution of each canonical variable to the variation in the original variables (Table 5). The canonical variable of set 2 can explain 51.7, 49.1 and 56.0% of the variation of the canonical variable of set 1 (SOCD) in grassland, cropland and forest, which also explained 39.9, 37.9 and 46.5% of the variation of the original variables, as in Table 1. Moreover, the canonical variable of set 1 (SOCD) explained 20.6, 18.6 and 26.0% of the variation of canonical variable of set 2, which indicated that SOCD can also have an influence on the influencing factors.
Multiple regression analysis
The SOCD in each land use type was predicted by the statistical model based on the influencing factors (Table 6) and approximately 51, 55 and 47% of the variability in SOCD could be explained. There was not serious multi-co-linearity in the variables of each model, because the variance inflation factor (VIF) was close to the value of 1.
The order of the significance of the influencing factors in grassland and forest was (Table 6): soil type > elevation > slope and slope > soil type > elevation. Elevation was eliminated from the model because there was not a significant correlation between SOCD and elevation in the cropland. The order of the other two influencing factors in the cropland was: soil type > slope.
Discussion
The variation of SOCD in the differing soil types indicated that SOCD was affected by soil-forming processes (Table 1). For example, the accumulation rate of soil organic matter is much higher than its decomposition rate and hence a fertile soil layer develops on the surface of chernozems (Chen and Huang 1988). The variation in SOCD of the differing land use types in each soil type was not similar (Fig. 2). The variation in SOCD between grassland and cropland was not significant in castanozems, because the large extent of grassland degradation-induced SOC content decreased (Li et al. 2002). There was a significant variation in SOCD between cropland and forest, which demonstrated that the restoration of practice of returning cropland to forest had produced the desired effect. Liu et al. (Liu and Tong 2005) pointed out that the change in the land use structure of Duolun County had led to soil fertility characteristics changing considerably. Returning cropland to forest is helpful for the accumulation of soil organic matter and the conservation of soil and water. The SOCD of eolian soils was low because the vegetation coverage was sparse and hence the availability of plant residues was reduced. Therefore, reclaiming the croplands in the fertilized area containing eolian soils will also alter the terrestrial ecosystem balance, which will then lead to greater chances of soil erosion and desertification (Su 2006). The SOCD in the forest was close to that in the grassland found on the chernozems, castanozems and eolian soils, which again indicated that returning forest from cropland was productive. Other benefits of returning the croplands to forest will be improving soil quality, preventing and controlling desertification.
Different processes regulating humus accumulation occur in different soil types and can lead to the differences in SOC content. Therefore, SOCD depends on the soil type to a large extent (Zinn et al. 2005). Elevation and slope produces impacts on soil water balance, soil erosion and the process of geological deposition, which will also have important roles in contributing to the variation of SOC stocks (Ritchie et al. 2007). The correlation between SOCD and slope was only significant in the forest (Table 6). This was largely due to the effect of returning cropland to forest, which has been done since 2000, especially over slopes of >20°. The different times the cropland has reverted to forest and the different degrees of site disturbance have led to differences in SOCD as the slope increased. The variation in the canonical variables for set 2 can be explained by the canonical variable of set 1, which implies that SOCD can have an influence on the influencing factors.
Multiple linear regression models (MLR) were developed to relate SOCD to the influencing factors in the differing land uses. It is likely that the regression models can be used to estimate and predict the variation in SOC, which can be sequestered in differing land use types of Duolun County (based on the digital maps of land use, soil type, slope and elevation, Table 5). Other studies have used statistical models to predict SOC. For example, Moore et al. (1993) used stream power, wetness index and aspect as predictors of SOC and from these they explained 48% of its variation. Florinsky et al. (2002) likewise analyzed the relationship between SOC and the influencing factors and were able to explain 37% of the variation with factors such as elevation, slope, plan and profile curvatures. MLR seem to be the preferred method for assessing the SOC stock on different scales (Nyssen et al. 2008; Singh et al. 2007).
In general, the results implied that not only soil type, but also slope can play an important role in regulating the variation of SOCD in each land use type. Other studies have also clarified the close relationship between SOC and terrain features. Gessler et al. (2000) have shown that SOC is highly correlated with the combination of slope and log flow accumulation. Terra et al. (2004) have also pointed out that at their site elevation, slope, compound topographic index, electrical conductivity, water table depth, as well as sand and silt content can be used to explain the variation in SOC efficiently. The results of MLR were similar to that of ANOVA and CCA (Fig. 3). The total contribution of the influencing factors to the variation in SOCD in differing land use types was around 50%, indicating that more factors such as those related to biological and pedogenic processes should have been taken into account to explain the SOCD variation.
Conclusion
-
1.
The SOCD of the top 30 cm of soil varied significantly among the soil types within each land use. This was caused by the different processes involved in the accumulation of soil organic matter in each soil type. The sensitivity of SOCD to land use change was different in six soil types. In general, the degradation of the soil led SOCD to decrease remarkably, which was a result of the extensive degradation of the grassland. Consequently, the variation in SOCD between the cropland and forest was significant in most cases. The SOCD of the forest has been increasing after a large proportion of cropland was required to be returned to forest due to governmental policy change, but no differences (in SOCD) between forest and grassland have been observed yet. Thus, the practice of allowing cropland to revert to forest and grassland should be ongoing.
-
2.
There are close relationships between SOCD and the influencing factors, which varies with land use types. SOCD depended on soil type to a greater extent, especially in the grassland. Except slope, other influencing factors were significantly correlated with SOCD in each land use. There were significant correlations between SOCD and slope in the forest. This indicated that allowing sloping fields to return to forest will have a positive effect on the SOCD in the forest.
-
3.
The statistical model, which was developed based on multiple linear regression, can explain approximately 50% of the variation in SOCD for each land use type. Using this model, the SOC stocks for the region can then be predicted based on the maps of land use, soil type, slope and elevation.
References
Baoyin T, Bai YF (2004) Problems in development of agriculture–animal husbandry ecotone and its countermeasures. Chin J Appl Ecol 15(2):245–248 (in Chinese with English abstract)
Baoyin T, Zhao LH, Bai YF, Li ZH (2000) The analyses of status quo of using land on DUO LUN County Nei Mongol. Acta Scientiarum Naturalium Universitatis Neimongol 31(5):517–520 (in Chinese with English abstract)
Batjes NH (1996) Total carbon and nitrogen in the soils of the world. Eur J Soil Sci 47(2):151–163
Batjes NH, Sombroek WG (1997) Possibilities for carbon sequestration in tropical and subtropical soils. Glob Change Biol 3:161–173
Bruce JP, Frome M, Haite E, Janzen H, Lal R, Paustian K (1999) Carbon sequestration in soils. J Soil Water Conserv 54:382–389
Chen ZZ, Huang DH (1988) The characteristics of meadow grassland and its effects on chernozem formation process in the Xilin River valley, Inner Mongolia. Scientia Geographica Sinica 1:38–46 (in Chinese with English abstract)
Dupouey JL, Dambrine E, Laffite JD, Moares C (2002) Irreversible impact of past land use on forest soils and biodiversity. Ecology 83:2978–2984
Florinsky IV, Eilers RG, Manning GR, Fuller LG (2002) Prediction of soil properties by digital terrain modelling. Environ Model Softw 17:295–311
Gessler PE, Chadwick OA, Chamran F, Althouse L, Holmes K (2000) Modeling soil-landscape and ecosystem properties using terrain attributes. Soil Sci Soc Am J 64(6):2046–2056
Grigal DG, Ohmann LF (1992) Carbon storage in upland forestland of the Lake States. Soil Sci Soc Am J 56:935–943
Han JG, Han YW, Sun TJ, Wang XS (2004) Effects of returning cultivated land to herbage on soil organic matter and nitrogen in the agro-pastoral transitional zone of north China. Acta Prataculturae Sinica 13(4):21–28 (in Chinese with English abstract)
Kirschbaum MUF (2000) What contribution can tree plantations make towards meeting Australia’s commitments under the Kyoto Protocol? Environ Sci policy 3:83–90
Lal R (2002) Soil carbon dynamics in cropland and rangeland. Environ Pollut 116(3):353–362
Li SL, Chen YJ, Guan SY, Kang SA (2002) Relationships between soil degradation and rangeland degradation. J Arid Land Resour Environ 16(1):92–96 (in Chinese with English abstract)
Liu QY, Tong YP (2005) The relationship between land use and soil nutrients of agro-pastoral ecotone: a case study of Duolun County, Inner Mongolia. Quat Sci 25(1):117–118 (in Chinese with English abstract)
Liu DW, Wang ZM, Zhang B, Song KS, Li XY, Li JP, Li F, Duan HT (2006) Spatial distribution of soil organic carbon and analysis of related factors in croplands of the black soil region, Northeast China. Agr Ecosyst Environ 113:73–81
Moore ID, Gessler PE, Nielsen GA, Peterson GA (1993) Soil attribute prediction using terrain analysis. Soil Sci Soc Am J 57:443–452
Mueller TG, Pierce FJ (2003) Soil carbon maps: enhancing spatial estimates with simple terrain attributes at multiple scales. Soil Sci Soc Am J 67:258–267
Nelson DW, Sommers LE (1982) Total carbon, organic carbon and organic matter. In: Page AL, Miller RH, Keeney DR (eds) Methods of soil analysis, Part 2, 2nd edn. Agronomy Monograph, vol 9. ASA and SSSA, Madison, pp 534–580
Nyssen J, Temesgen H, Lemenih M, Zenebe A, Haregeweyn N, Haile M (2008) Spatial and temporal variation of soil organic carbon stocks in a lake retreat area of the Ethiopian Rift Valley. Geoderma 146:261–268
Post WM, Emanuel WR, Zinke PJ, Stangenberger AG (1982) Soil carbon pools and world life zones. Nature 298:156–159
Qiao Q (2007) Research on landscape pattern and ecological frangibility assessment of Chuan-Dian farming-pastoral zone. Dissertation, Beijing Forestry University, Beijing
Ritchie JC, McCarty GW, Venteris ER, Kaspar TC (2007) Soil and soil organic carbon redistribution on the landscape. Geomorphology 89:163–171
Schaefer DA, Feng WT, Zou XM (2009) Plant carbon inputs and environmental factors strongly affect soil respiration in a subtropical forest of southwestern China. Soil Biol Biochem 44:1000–1007
Schwartz D, Namri M (2002) Mapping the total organic carbon in the soils of the Congo. Glob Planet Change 33:77–93
Singh SK, Singh AK, Sharma BK, Tarafdar JC (2007) Carbon stock and organic carbon dynamics in soils of Rajasthan, India. J Arid Environ 68:408–421
Su YZ (2006) Soil carbon and nitrogen sequestration following cropland to forage grassland conversion in the marginal land in the middle of Heihe River basin, Northwest China. Environ Sci 27(7):1312–1318 (in Chinese with English abstract)
Tan ZX, Lal R, Smeck NE, Calhoun FG, Gehring RM, Parkinson B (2003) Identifying associations among soil and site variables using canonical correlation analysis. Soil Sci 168:376–382
Terra JA, Shaw JN, Reeves DW, Raper RL, Santen EV, Mask PL (2004) Soil carbon relationships with terrain attributes, electrical conductivity, and a soil survey in a coastal plain landscape. Soil Sci 169:819–831
Wang FG, Wang QJ, Wang WY, Jing ZC (2008) Research progress on soil organic matter. Prata Sci 2(25):48–55 (in Chinese with English abstract)
Zhang WT, Yan J (2004) Advanced course of statistical analysis for SPSS. Higher Education Press, Beijing, pp 278–279 (in Chinese)
Zinn YL, Lal R, Resck DVS (2005) Texture and organic carbon relations described by a profile pedotransfer function for Brazilian Cerrado soils. Geoderma 127:168–173
Acknowledgments
This work was supported by the National Natural Science Foundation of China (Grant No. 31040012) and State Key Laboratory of Earth Surface Processes and Resource Ecology (Grant No. 2008-ZZ-08). We also thank several anonymous reviewers for their valuable comments and suggestions to improve this manuscript.
Author information
Authors and Affiliations
Corresponding author
Rights and permissions
About this article
Cite this article
Li, B., Tang, H., Wu, L. et al. Relationships between the soil organic carbon density of surface soils and the influencing factors in differing land uses in Inner Mongolia. Environ Earth Sci 65, 195–202 (2012). https://doi.org/10.1007/s12665-011-1082-0
Received:
Accepted:
Published:
Issue Date:
DOI: https://doi.org/10.1007/s12665-011-1082-0