Abstract
Background
As evidence mounts regarding associations between genetics and body weight, it is essential to understand how to communicate this information, and factors like emotion that could moderate the effectiveness of messages.
Purpose
We assessed influences of emotion on reactions to weight-related genomic information in a virtual clinical setting.
Methods
An online representative US sample of overweight women was randomized to receive an emotion induction (anger, fear, or neutral) paired with information about genomic or behavioral influences on weight in an interaction with a virtual doctor.
Results
Receiving genomic information led to reduced attributions of lifestyle causes for weight and behavioral intentions, but only among individuals in a fear state.
Conclusions
The current study is among the first to reinforce the concern that discussing genomic underpinnings of overweight could undercut health behavior, and highlights the importance of identifying factors like emotion that influence interpretation of genomic information.
Clinicaltrials.gov NCT01888913.
Similar content being viewed by others
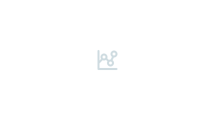
Avoid common mistakes on your manuscript.
Introduction
Weight counseling in primary care for overweight individuals is recommended [1]. However, existing weight management programs are often ineffective over the long term [2–4], suggesting the need for innovative strategies. A new federal initiative for precision medicine adds to emerging scientific efforts aimed at integrating genetics and genomics into personalized prevention and treatment programs [5]. Routine genomic integration will take some time to penetrate clinical practice; nevertheless, discussions of gene-environment and gene-behavior interactions already occur due to, for example, discussions of family health history, information learned through media, or consumer genetics products [6–8]. By assessing potential effects of communicating about genomics and weight, we may be able to optimize the way in which this information is disseminated in health-care settings.
Of course, we should not expect uniform and consistent effects of genomic information. This is due, in part, to wide variability in characteristics of the genomic information that will be disclosed, and variability in the context of disclosure. Indeed, the literature linking receipt of genetic or genomic risk information to health behavior and its precursors is mixed, and often shows no effect [9, 10]. There is a need to identify individual and contextual factors that will moderate this relationship. In so doing, we could determine the conditions under which genomic information can be optimally communicated so as to maximally encourage health-promoting behaviors. Psychological theory suggests that the emotional state of patients when they receive genomic information may be an important such moderator.
Genomic Information and Health Attitudes and Cognitions
In considering the integration of weight-related genomic information into the clinical encounter, concerns have been raised that such information has the potential to engender fatalism among patients [11]. These concerns are based on theoretical models such as the Integrative Model of Behavioral Prediction [12]; see Fig. 1 for logic model. Because one’s DNA is inherently fixed and genomic risk is often presented as uncontrollable in lay communication, presentation of genomic information could alter outcome expectancies, suggesting that patients cannot overcome a genetic predisposition for obesity. Genomic information could furthermore undermine patient self-efficacy for weight management [12]. Similarly, information involving specific genomic influences on one’s behavior (e.g., that genetic factors influence one’s dietary choices) could suggest that one’s behavior is also under less volitional control. Finally, provision of genomic information related to weight could also lead to beliefs that diet and physical activity are less influential, reducing motivation and intention to perform those behaviors [13, 14]. Through the Integrative Model of Behavioral Prediction, each of these influences is posited to lead to decreased healthy behavior (Fig. 1). Despite this theoretical reasoning, there has, to-date, been very little evidence to support these assertions in the realm of weight and obesity [15].
Most work examining the potential for genomic risk information to motivate or de-motivate health behavior has shown no effect [9, 10]. However, a handful of studies have provided evidence that weight-related genomic information may be helpful in promoting the beliefs and attitudes that underlie health behavior [16–19]. These pathways are depicted in Fig. 1, and include, for example, when genomic risk information increases individuals’ perceptions of personal obesity risk. Genomic information might also evoke the idea that one can or must “fight against” or “overcome” genetic predisposition [20]. Through these types of mechanisms, introduction of genomic information into weight-focused clinical encounters theoretically has the capacity both to undercut and promote attitudes and beliefs underlying health behavior change.
The Role of Emotion
One reason for the inconsistency in effects of genomic information provision on decision-making and behavior may be a failure to acknowledge the potentially influential individual and contextual factors present in the clinical interaction. Here, we consider the role of the patient’s emotional state. Patient emotion in a given medical visit may or may not be related to the clinical interaction per se. Everyday life is replete with affectively laden experiences, and patients are likely to bring emotional “tags” with them into a clinical encounter. Although the source of an emotion may not be normatively related to the clinical counter, research shows that these types of unrelated (i.e., incidental) emotions can carry over to influence ostensibly unrelated decisions [21–23]. These unrelated emotions may be compounded, or augmented, by emotions that are elicited in the context of the clinical encounter (e.g., by the prospect of an anxiety-provoking medical procedure). In the context of clinical encounters, understanding the role of fear and anger may be particularly instructive. Emotions occur regularly within the health-care context [24]. Anger and fear both have robust—and often very different—influences on judgment and decision-making tendencies, particularly in the context of risk and uncertainty [23], despite the fact that both emotions are negatively valenced.
The Appraisal Tendency Framework has described ways that incidental emotions influence processing of messages [23, 25]. This is the theoretical lens through which we consider the potential influences of emotional state (see Fig. 1 for logic model). From a theoretical perspective, fear and anger are posited to have opposite effects on information processing and risk perception. Specifically, the personal control and certainty cognitive appraisal dimensions associated with anger versus fear are quite different [26]. This may predispose certain action tendencies related to information processing and judgments about risk. Anger, which is associated with heightened appraisals of control and certainty, is associated with lower perceptions of susceptibility and greater tolerance for risk than is fear, which is low on these appraisal dimensions [22]. Moreover, because of high certainty appraisals, anger is associated with less motivation to process information systematically, and thus often triggers heuristic or stereotypical thinking patterns, whereas the opposite patterns are observed for fear [27]. In this way, anger could trigger more reliance on expertise (e.g., a doctor) when evaluating a message. On the other hand, anger is also associated with reduced trust and cooperation [28]. Thus, as Fig. 1 depicts, one would expect different outcomes when patients are exposed to genomic health information depending upon whether patients are in a state of fear or anger.
When a provider conveys information about genomic underpinnings of weight to a patient, it could be interpreted to suggest that patients have less control over their weight. If the patient is angry (associated with heightened generalized perceptions of control and reduced trust and cooperation), she might be less likely to believe that she has a genomic predisposition, or that such a predisposition undercuts her control of her weight. She may also be more likely to believe that she has the ability to reduce her weight and her risk for weight-related health conditions. The opposite would be expected for a patient in a fear state. In short, a patient’s emotional state is expected to act as a lens through which weight-related genomic information is interpreted (see Fig. 1).
The Current Study
The current study is an experimental study that assessed the influence of specific (induced) emotional states (neutral versus anger versus fear) on processes related to receipt of information about general genomic, versus behavioral, underpinnings of body weight provided by a simulated virtual physician. Outcomes assessed included causal attributions, comprehension, and behavioral intentions. This study was administered via virtual, online clinical interactions using a probability-based research panel representative of US adults. We had several hypotheses:
-
Hypothesis 1: Genomic causal attributions will be increased and lifestyle causal attributions will be decreased in the genomic information provision conditions. These patterns will be most pronounced among participants in the fear group and least pronounced in the anger group given tendencies associated with high control for anger, and low control for fear.
-
Hypothesis 2: Message comprehension will be worse for genomic information given its novelty. Comprehension will be particularly low among participants in the anger condition given the association between anger and heuristic message processing.
-
Hypothesis 3: The provision of genomic information will be associated with increased behavioral intentions for those in the anger condition and decreased behavioral intentions for those in the fear condition (and thus no overall main effect of information type on change intentions).
Method
Participants were 1126 women in a probability-based online research panel though the market research firm GfK. The study was conducted via the Time-Sharing Experiments in the Social Sciences (TESS) program, a National Science Foundation-funded initiative that facilitates experiments through GfK after being deemed meritorious by external peer-review. To be eligible for this study, the women were required to have a self-reported body mass index of 25 or greater at most recent assessment (typically less than 1 year). The study employed a 3 × 2 design, in which participants were randomized to six conditions wherein they received an emotion induction (fear, anger, or neutral), and received information about genomic (including genetic, gene-environment, and gene-behavior interactions) versus behavioral causal factors in body weight.
A probability-stratified sample of panelists was invited to participate in the experiment via email. Those who chose to participate consented to the study. The project was described broadly as a study of “people’s psychological experiences and how these relate to the responses people have when they encounter different types of communication stimuli.” Participants were then given a writing prompt that served as the emotion elicitation [29]. Participants in the anger condition were asked to write for 5 min about a situation that had made them very angry such that someone reading the story would also feel that emotion. Participants in the fear condition were given the same prompt, substituting fear for anger. Those in the neutral condition were asked to spend the same amount of time describing a room in their house. This autobiographical method for emotional elicitation has been shown to effectively induce emotion in internet-based studies in a recent meta-analysis [30].
Following the writing task, participants were asked to watch a series of videos depicting a virtual physician delivering information about clinical weight management. Participants were asked to answer the questions that the virtual doctor posed either mentally or aloud. The virtual doctor presented information related to the importance of weight management, the link between overweight and increased breast cancer risk, and that one’s lifestyle behaviors are important for health. The virtual doctor also presented information about either the genomic or behavioral underpinnings of weight, depending upon condition. In the genomic condition, information included topics such as high heritability of weight, that those with a genomic predisposition for overweight may need to work harder to manage weight, and the notion that genes interact with the environment and one’s behavior to influence weight. In the behavior condition, material focused on the importance of both diet and exercise, the difficulty that overweight individuals may have in managing weight, and the role that environmental influences have on weight. Finally, participants completed a questionnaire and were debriefed. Participants were not compensated beyond standard panel compensation. This study was approved by the IRB of the National Human Genome Research Institute.
Measures
Lifestyle causal beliefs were assessed using items that asked participants the extent to which they agree or disagree that “eating too much or too many unhealthy foods” and “not doing enough exercise” contribute to their body weight. Responses were collected on a 1–7 scale (strongly disagree to strongly agree), and the two responses were averaged (r = 0.63, p < 0.0001). Genetic causal beliefs were assessed with an item that asked about the extent to which participants agree or disagree that “genetics” contributes to their weight. Causal belief items were adapted from Ogden and Flanagan [31].
Dietary intentions were assessed, following disciplinary standards, with an average of two items (r = 0.85, p < 0.0001): “I intend to make changes to my diet in the next 6 months” and “how likely is it you will try to change your diet in the next 6 months” (1–7 scale; strongly agree to strongly disagree and very unlikely to very likely, respectively). Exercise intentions were assessed using the same items, substituting “get more exercise” for “change your diet” (r = 0.086, p < 0.0001).
Comprehension of the message was measured by asking participants to list three facts or findings from the simulated interaction that they would remember. These facts were coded based on (a) how well participants captured the main themes of the interaction, and (b) how detailed and correct responses were. Coding was performed on a 1–5 scale for both metrics, and collapsed to a 1–3 scale for better reliability. Coding was performed by three trained coders who began by arriving at acceptable reliability on 10 % of the data set (intra-class correlations 0.965 and 0.933 for themes and detail, respectively). Remaining data were single-coded. The themes and detail scores were summed for all three facts, resulting in a single score for each participant.
Data Analysis
Upon examination of the data set from the first run of the study (n = 570), we discovered an issue wherein participants’ responses to the emotion elicitation writing task were capped at 1024 characters. Participants who exceeded this cap were given an error message and required to edit their response to meet this limit. This issue was corrected, and the study was then completed again with a new sample (n = 555). The final data set included all participants from the second iteration of the study, and participants from the first run whose response to the emotion elicitation writing task was 925 characters or fewer. We arrived at this criterion by calculating the average number of characters per sentence in participants’ responses, and including only those participants whose responses were at least two average sentences shorter than the cut-off. This minimizes chances that any participants in the final data set were limited by the character cap and had to revise their responses to fit. This resulted in a data set of 1046 individuals. We further excluded from analysis participants who reported having breast cancer (n = 11; this was due to information provided about links between obesity and breast cancer within the manipulation), and those who did not attempt the writing task (n = 153). The final sample for analysis was n = 882. Analyses run with and without participants excluded due to the character cap revealed comparable patterns (with the exception that the interaction for lifestyle causal beliefs measure went from significant to marginal; p < 0.10).
Significance was assessed at p < 0.05. Differences between conditions on demographic variables were assessed using ANOVA for continuous outcomes and chi square tests for categorical ones. Analyses assessing the influence of the manipulations on outcome variables were performed on data weighted to match the US population. These analyses employed ANOVAs with planned comparisons.
Results
Participant demographics did not differ significantly by condition (see Table 1).
Causal Beliefs: Hypothesis 1
For genetic causal beliefs, as predicted, there was a significant information type main effect such that participants who received genomic information believed that genes were a more substantial contributor to their weight, F(1850) = 29.07, p < 0.0001, partial η 2 = 0.033. There was also a significant interaction, F(1850) = 7.19, p = 0.001, partial η 2 = 0.017. Contrary to predictions, causal beliefs were increased among those who received genomic information (versus behavioral information) in the control and anger conditions, but not the fear condition. In addition, among those who received behavioral information, genetic causal beliefs were lowest among those in the anger condition. All effects are displayed in Fig. 2.
There were no significant main effects for lifestyle causal beliefs, contrary to hypotheses. There was a significant interaction between information type and emotion type on lifestyle causal beliefs, F(1868) = 3.25, p = 0.039, partial η 2 = 0.007. Consistent with hypotheses, lifestyle causal beliefs were decreased among participants who received genomic information in the fear condition only.
Comprehension: Hypothesis 2
There was a hypothesis-consistent main effect of information type such that comprehension of the behavioral message (M = 12.78, SD = 2.98) was higher than the genomic message, M = 12.25, SD = 3.40; F(1750) = 5.47, p = 0.020, partial η 2 = 0.008. There was a hypothesis-inconsistent main effect of emotion such that comprehension was lower when participants were in a fear state (M = 12.33, SD = 3.17) or anger state (M = 12.25, SD = 3.42) than when they were in a neutral emotional state, M = 12.88, SD = 3.03; F(1750) = 3.13, p = 0.044, partial η 2 = 0.007. There was not a significant interaction.
Behavioral Intentions: Hypothesis 3
As predicted, there were no significant main effects for dietary intentions, but there was a significant interaction, F(1867) = 3.59, p = 0.028, partial η 2 = 0.008. We hypothesized an increase in intentions among those who received genomic information and were in the anger condition, and a decrease among those who received genomic and were in the fear condition. We found that intentions were lower for those who received genomic information in the fear condition only. Dietary intentions among those who received the behavioral information were higher than the control condition in both the anger and fear conditions. For exercise intentions, there were no main effects, but there was a significant interaction, F(1866) = 3.53, p = 0.030, partial η 2 = 0.008. Once again, intentions were lower for those who received genomic information in the fear condition only, consistent with findings for other measures.
Discussion
In this study, a pattern emerged such that receipt of genomic information related to the causes of overweight led to more negative outcomes with respect to beliefs and attitudes that underlie health behavior, but only among individuals in a fear state. Among fearful participants, those who received genomic etiology information (as compared to those who received behavioral etiology information) rated diet and exercise as less influential for body weight and had lower intentions to change their dietary and exercise behavior. On the other hand, receiving information about behavioral underpinnings of weight while in a fearful state led to no such declines, nor were these effects seen for participants receiving information about genomic underpinnings of weight while in an angry or neutral state. This pattern of results was inconsistent with the more complex set of associations between genomic information and emotion that we hypothesized based on the Appraisal Tendency Framework. Instead, it appears that there is something special about the combination of fear and receipt of genomic causal information with respect to body weight that results in a profile of psychological outcomes that could be detrimental to performing health behaviors and/or achieving a healthy weight. With few exceptions [15], previous literature has reported that provision of genetic or genomic information related to body weight does not undercut health behavior [16–18, 32, 33]. In contrast, the current work demonstrates that genomic information can indeed have such negative effects when it is received in the context of a particular emotional state. In addition, this work shows that the effects are specific to fearful emotional states, as opposed to a general influence of negative affect (given that a different pattern emerged among angry participants).
Notably, of all groups receiving genomic causal information, the fear group also reported the lowest levels of endorsements related to the role of genomics in causing body weight. Unlike angry and neutral participants, participants in the fear condition who received genomic information were no more likely to endorse genomic causes of overweight than were those participants who received behavioral information. This is important because it means that the negative influence of genomic information on health behavior-related outcomes does not seem to function by increasing genomic causal attributions to the detriment of other causal attributions. In other words, it is not the case that fearful individuals believe that genes are more influential on their weight, and therefore other factors such as diet and exercise are less influential. Instead, beliefs about the influence of both types of causes were depressed. This pattern is consistent with predictions made by the Extended Parallel Process Model [34]. Although genomic information related to common health conditions like overweight is not typically considered to be threatening [10, 35], participants who are already in a fear state may be more likely to interpret it as such. These heightened threat perceptions may be matched with lower levels of self-efficacy and response-efficacy sometimes associated genetic explanations for weight, and thus lead to defensive processing. In this case, defensive processing was evidenced by depressed causal attributions for both behavioral and genomic mechanisms and can also explain decreased intentions to change dietary and physical activity behavior displayed by these participants. The current findings, therefore, add to a small literature so far confined to other health domains (e.g., lung cancer, skin cancer), that reports effects of genetic information exposure that are consistent with defensive processing [35, 36].
Patterns for comprehension of the message the virtual doctor conveyed did not follow the patterns seen for other outcomes. As anticipated, comprehension was lower for genomic information, perhaps due to its novelty. However, both types of negative emotion led to reduced comprehension versus control. This is consistent with previous work showing that negative mood or emotion can impair comprehension by expending cognitive resources [37]. Furthermore, although the Appraisal Tendency Framework differentiates the influence of anger and fear on comprehension-related outcomes gist and heuristic processing, our comprehension measure contained elements of both. This may have also led to the lack of differentiation in comprehension between the two negative emotions.
In the current study, we focused on the effect of incidental rather than integral emotions (i.e., emotions directly related to an interaction, judgment, or decision). Incidental and integral emotions can function similarly to influence decision-making [22]; that is, angry individuals should seek out risk regardless of whether their anger is related or unrelated to the risky situation. Nonetheless, examining incidental emotions allows us to experimentally capture the direct and unconfounded effects of discrete emotions on behavior. Doing so provides generalizable knowledge about how individuals may process genomic and behavioral communications about obesity when they are in angry or fearful states that are related or unrelated to the clinical encounter [22, 38]. This is crucial because although genomic information related to common conditions like obesity is not reliably expected to elicit fear or other negative emotions on its own [18, 39], there are many other aspects of the medical context or encounter that can. This could include, for example, the use of fear appeals related to weight management and overweight, or fear-inducing medical procedures (e.g., blood draws, injections).
The current findings also have implications for theory and research on the influence of emotions on information processing. Specifically, the influence of emotion on shaping appraisal dimensions and action tendencies may depend upon the nature of the information being conveyed. In some cases, there may be better or worse fit between the naturally occurring reactions to communication content and the incidental emotional states of targets. As such, targets may display outcomes theoretically consistent with emotions to a greater or lesser extent.
This study was administered online, using a video recording of a virtual simulated physician to deliver information about underpinnings of weight. Although this setting is somewhat lacking in ecological validity, validity is likely increased over traditional simulation techniques such as the use of text-based hypothetical vignettes. In addition, health information is increasingly being conveyed using online and multimedia channels, so this presentation mode is not all that unusual. Use of this method also allowed us to reach a large, probability-based sample of respondents. In this initial study, we limited participation to women whose BMI was classified as overweight. We chose to limit the sample to women because some of the risk messages conveyed pertain to breast cancer. Future work should examine the potential for similar effects among men, and among individuals who are not overweight. Finally, the current study examines only the beliefs and attitudes that underlie health behavior but does not measure health behavior itself. The methods employed here (online survey of a standing panel) precluded the measurement of actual behaviors; however, this next step will be crucial in understanding the potential health influence of these processes.
The current work is among the first to show that there may be some truth to the concern that discussing the genomic underpinnings of overweight could undercut health behavior among individuals who are overweight. Indeed, we saw reductions in some of the key beliefs and attitudes that have been shown to underlie health behavior change in previous work (lifestyle causal attributions, health message comprehension, and behavioral intentions). However, these outcomes appear to occur only among individuals who receive this information while in a fear state. Patient emotional state does not receive much attention as a modifiable aspect of clinical interactions. However, should patient emotions in the clinical encounter prove to predictably influence the processing of genomic health information, such interventions might be considered. Through attention to these processes, we can ensure that the genomic information provided in medical contexts serves only to improve patient health.
References
Moyer V. Screening for and management of obesity in adults: U.S. Preventive Services Task Force recommendation statement. Ann Intern Med. 2012; 157(5): 373-378.
Douketis J, Macie C, Thabane L, et al. Systematic review of long-term weight loss studies in obese adults: clinical significance and applicability to clinical practice. Int J Obes. 2005; 29(10): 1153-1167.
Anderson J, Konz E, Frederich R, et al. Long-term weight-loss maintenance: A meta-analysis of US studies. Am J Clin Nutr. 2001; 74(5): 579-584.
Kraschnewski J, Boan J, Esposito J, et al. Long-term weight loss maintenance in the United States. Int J Obes. 2010; 34(11): 1644-1654.
Collins FS, Varums H. A new initiative on precision medicine. N Engl J Med. 2015; 372: 793-795.
Bloss C, Wineinger N, Darst B, et al. Impact of direct-to-consumer genomic testing at long term follow-up. J Med Genet. 2013; 50(6): 393-400.
Persky S, Sanderson S, Koehly L. Online communication about genetics and body weight: implications for health behavior and internet-based education. J Health Comm. 2013; 18(2): 241-249.
Zlot A, Bland M, Silvery K, et al. Influence of family history of diabetes on health care provider practice and patient behavior among nondiabetic Oregonians. Prev Chron Dis. 2009; 6(1): A27.
Marteau T, French D, Griffin S. et al. Does communicating DNA-based risk estimates motivate people to change their behaviour? Cochrane Database Syst Rev 2010; (10): Art. No: CD007275.
McBride C, Sanderson S, Kaphingst K, et al. The behavioral response to personalized genetic information: Will genetic risk profiles motivate individuals and families to choose more healthful behaviors? Ann Rev Pub Health. 2010; 31: 89.
Senior V, Marteau T, Peters T. Will genetic testing for predisposition for disease result in fatalism? A qualitative study of parents responses to neonatal screening for familial hypercholesterolaemia. Soc Sci Med. 1999; 48(12): 1857-1860.
Fishbein M, Yzer MC. Using theory to design effective health behavior interventions. Comm Theory. 2003; 13(2): 164-183.
Leventhal H. Findings and theory in the study of fear communications. Adv Exp Soc Psychol. 1970; 5: 119-186.
Marteau T, Weinman J. Self-regulation and the behavioural response to DNA risk information: A theoretical analysis and framework for future research. Soc Sci Med. 2006; 62(2): 1360-1368.
Dar-Nimrod I, Cheung B, Ruby M, et al. Can merely learning about obesity genetics affect eating behavior? Appetite. 2014; 81: 269-276.
Harvey-Berino J, Gold EC, West DS, et al. Does genetic testing for obesity influence confidence in the ability to lose weight? A pilot investigation. J Am Diet Assoc. 2001; 101(11): 1351-1353.
Frosch D, Mello P, Lerman C. Behavioral consequences of testing for obesity risk. Cancer Epidemiol Biomarkers Prev. 2005; 14(6): 1485-1489.
Meisel S, Walker C, Wardle J. Psychological responses to genetic testing for weight gain: A vignette study. Obesity. 2012; 20(3): 540-546.
Sanderson S, Persky S, Michie S. Psychological and behavioral responses to genetic test results indicating increased risk of obesity: does the causal pathway from gene to obesity matter? Public Health Genom. 2010; 13(1): 34-47.
Meisel S, Wardle J. 'Battling my biology': Psychological effects of genetic testing for risk of weight gain. J Genet Couns. 2014; 23(2): 179-186.
Lerner JS, Li Y, Valdesolo P, et al. Emotion and decision making. Ann Rev Psychol. 2015; 66: 799-823.
Han S, Lerner JS, Keltner D. Feelings and consumer decision making: The appraisal-tendency framework. J Consum Psychol. 2007; 17(3): 158-168.
Lerner JS, Keltner D. Beyond valence: Toward a model of emotion-specific influences on judgement and choice. Cogn Emot. 2000; 14(4): 473-493. doi:10.1080/026999300402763.
Finset A. "I am worried, doctor!" Emotions in the doctor-patient relationship. Patient Educ Couns. 2012; 88(3): 359-363.
Lerner JS, Tiedens LZ. Portrait of the angry decision maker: How appraisal tendencies shape anger’s influene on cognition. J Behav Decis Mak. 2006; 19(2): 115-137.
Smith C, Ellsworth P. Patterns of cognitive appraisals in emotion. J Pers Soc Psychol. 1985; 48(4): 813-838.
Tiedens L, Linton S. Judgment under emotional certainty and uncertainty: the effects of specific emotions on information processing. J Pers Soc Psychol. 2001; 81(6): 973-988.
Dunn J, Schweitzer M. Feeling and believing: The influence of emotion on trust. J Pers Soc Psychol. 2005; 88(5): 736-748.
Strack F, Schwarz N, Gschneidinger E. Happiness and reminiscing: The role of time perspective, affect, and mode of thinking. J Pers Soc Psychol. 1985; 49(6): 1460-1469.
Ferrer R, Grenen E, Taber J. Effectiveness of internet-based affect induction procedures: A systematic review and meta-analysis. Emotion. 2015; 15(6): 752-762.
Ogden J, Flanagan Z. Beliefs about the causes and solutions to obesity: A comparison of GPs and lay people. Patient Educ Couns. 2008; 71(1): 72-78.
Lippa N, Sanderson S. Impact of informing overweight individuals about the role of genetics in obesity: An online experimental study. Hum Hered. 2013; 75: 186-203.
Persky S, Street RL. Evaluating approaches for communication about genomic influences on body weight. Ann Behav Med. 2015; 49(5): 675-684.
Witte K. Putting the fear back into fear appeals: The extended parallel process model. Comm Monogr. 1992; 59(4): 329-349.
Lipkus I, McBride C, Pollak K, et al. Interpretation of genetic risk feedback among African American smokers with low socioeconomic status. Health Psychol. 2004; 23(2): 178-188.
Aspinwall L, Taber J, Kohlmann W, et al. Perceived risk following melanoma genetic testing: A 2-year prospective study distinguishing subjective estimates from recall. J Genet Couns. 2014; 23(3): 421-437.
Ellis H, Ottaway S, Varner L, et al. Emotion, motivation and text comprehension: The detection of contradictions in passages. J Exper Psychol. 1997; 126(2): 131-146.
Lerner JS, Keltner D. Fear, anger, and risk. J Pers Soc Psychol. 2001; 81(1): 146-159.
Bloss C, Schork N, Topol E. Effect of direct-to-consumer genomewide profiling to assess disease risk. N Engl J Med. 2011; 364: 524-534.
Acknowledgments
This research was supported by the Intramural Research Program of the National Human Genome Research Institute, National Institutes of Health. Data collected by Time-sharing Experiments for the Social Sciences, NSF Grant 0818839, Jeremy Freese and James Druckman, Principal Investigators. The authors thank Paul Han, MD, for advice and feedback during study development. We acknowledge Janine Beekman, Allison Sypher, and Peter Hanna for assistance with code book development and coding.
Author information
Authors and Affiliations
Corresponding author
Ethics declarations
Conflict of Interest Statement
Authors’ Statement of Conflict of Interest and Adherence to Ethical Standards Authors Persky, Ferrer, and Klein declare that they have no conflict of interest. All procedures, including the informed consent process, were conducted in accordance with the ethical standards of the responsible committee on human experimentation (institutional and national) and with the Helsinki Declaration of 1975, as revised in 2000.
About this article
Cite this article
Persky, S., Ferrer, R.A. & Klein, W.M.P. Genomic Information may Inhibit Weight-Related Behavior Change Inclinations Among Individuals in a Fear State. ann. behav. med. 50, 452–459 (2016). https://doi.org/10.1007/s12160-016-9771-2
Published:
Issue Date:
DOI: https://doi.org/10.1007/s12160-016-9771-2