Abstract
Improvements in energy efficiency will be instrumental in meeting China’s ambitious emission targets while providing adequate energy to support the country’s rising living standards. We investigate whether the low-carbon city pilot (LCCP) program has improved energy efficiency in participating cities. To this end, we analyze prefecture-level data in 249 cities between 2004 and 2016 using the difference-in-differences approach. The findings reveal that the program significantly improved the energy efficiency of pilot cities by promoting technological innovation and restructuring the cities’ industry mix. The positive impacts of the LCCP program on energy efficiency were more pronounced in cities in China’s eastern region and areas lacking natural resources. Furthermore, the program was more effective in cities with high energy consumption and per capita income on the one hand and low foreign direct investment and sulfur dioxide emissions on the other.
Similar content being viewed by others
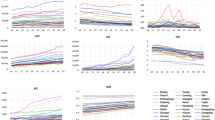
Avoid common mistakes on your manuscript.
Introduction
China’s energy use has risen steeply during the twenty-first century. It is now the world’s largest energy consumer in the world. The country accounted for almost all of the global electricity and heat sector emission increase between 2019 and 2021 (IEA, 2022). There is a consensus that China’s climate policy is integral to achieving global carbon neutrality in the fight against climate change—China has committed to reducing its environmental footprint. In the weeks leading up to the COP26 summit, China announced its goal of achieving carbon neutrality by 2060 (Zhou & Hu, 2021). Achieving this ambitious goal will require replacing carbon-emitting fossil fuels with clean energy sources; however, energy transitions are long and gradual (Smil, 2014, 2021). Moreover, with China’s energy demand expected to peak in 2035, there are still challenges for its current energy mix to achieve carbon neutrality by 2060. Energy conservation and improvements in energy efficiency will be instrumental in meeting emission targets on time while providing adequate energy to support the country’s rising living standards.
Chinese cities account for 85% of the country’s total direct carbon emissions (Shan et al., 2019). To curb these emissions, the Chinese government has enacted a series of environmental protection policies (Qin et al., 2021). China’s environmental regulatory policy tools have gradually shifted from relying exclusively on administrative orders from the government to a three-dimensional integrated environmental regulatory framework consisting of command and control (CAC), market-based incentives (MBI), and public participation (PP) tools (Du et al., 2022). Despite concerted efforts to improve its energy efficiency, China lags behind the developed countries in this regard (International Energy Agency, 2021). To bring urban emissions under control, the National Development and Reform Commission (NDRC) of China, responsible for implementing the central government’s policies and decisions on development and reform, launched the low-carbon city pilot (LCCP) program in eight cities in 2010. Since then, 81 cities have participated in this project. As part of a comprehensive environmental regulatory framework, low-carbon pilots are aimed at promoting low-carbon urban development by restructuring the industry, increasing renewable energy use, and improving energy efficiency.
However, whether and to what extent these pilots have improved the energy efficiency of participating cities remains an open question. This paper is devoted to answering it. We also analyzed the variability in the effects on the energy efficiency of the pilot across different cities. Treating the LCCP program as a quasi-experiment, we use the difference-in-differences model to study its impact on different prefectures in China.
The present study contributes to this literature by addressing four critical challenges inherent in impact analyses of policies and programs to promote energy efficiency: measuring energy consumption, identifying causal effects, gathering data on small geographical units such as cities and prefectures, and understanding the channels through which the impact is mediated. Let us consider them in more detail. First, any measure of energy efficiency is predicated on energy consumption. Thus, the precision of energy consumption data is crucial—we use the Defense Meteorological Satellite Program (DMSP) Operational Linescan System (OLS) nighttime lighting data to determine energy consumption. This is a marked improvement over previous studies relying on electricity consumption and total energy consumption (Jianhuan Huang et al., 2018). Second, we use the DID model, which lends itself well to handling endogeneity and sample selection bias (Baker et al., 2022; Roth et al., 2022), thus identifying the causal relationship between environmental regulations and energy efficiency. Third, we examine the impact of the LCCP program on the energy efficiency of prefectures, thus offering a more detailed perspective on the program's efficacy. This differs from previous studies investigating the factors influencing the energy efficiency of countries, provinces, and industries (Sun et al., 2019). Last, we study the role of technological innovation and changes in the industrial structure in mediating the effects of the LCCP program on energy efficiency.
The remainder of the paper is structured as follows. The “Literature review” section reviews previous literature that evaluates the effects of environmental policy on energy efficiency. The “Methodology” section details the methodology. Data, variables, and descriptive statistics are presented in the “Data, variables, and descriptive statistics” section. The “Results and discussion” section discusses the empirical results, and the “Conclusions and policy implications” section concludes the paper and presents policy implications.
Literature review
China’s energy efficiency is low mainly due to its energy-intensive industries and production processes (Shixiang Li, 2008). The LCCP program can help redress this by encouraging innovation. Innovation entails risk. Thus, investors are often wary of funding it, leading to suboptimal investment in technological innovation (Jaffe et al., 2005). The LCCP program can help overcome this hurdle in two ways. First, the LCCP sets strict emission targets that program participants must meet, helping local governments persuade businesses to adopt new technologies and upgrade their infrastructure to lower their environmental footprint. Second, the LCCP also makes it easier for local governments to give businesses grants, subsidies, and low-interest loans to increase investment in research and development (R&D) initiatives.
The program can also rebalance the weights of secondary and tertiary sectors in the overall economy such that the tertiary sectors predominate. It has helped cities’ transition from relying mainly on industrial and manufacturing sectors to having sizeable services sectors—the predominance of the service sector is also associated with low energy intensity (Mulder & de Groot, 2012; Xiong et al., 2019; Xiong et al., 2019).
The LCCP program digressed from the top-down environmental management programs by adopting a bottom-up approach (Wang et al., 2015). Participating regions formulated their own plans conducive to transitioning to a low-carbon economy while fostering economic development. With considerable autonomy, regional actors calibrated the programs to meet local needs and challenges with only general guidance from the central government. The NDRC organized a series of meetings to evaluate the progress and performance of participating cities, which helped regional program managers learn from the experience of others. Sharing knowledge and information has helped entrench practices that have contributed to achieving the desired environmental outcomes. Nevertheless, the central government's strong support for the LCCP program has been instrumental in its success. Considerable resources have been allocated to developing low-carbon products, technologies, and industries that have enhanced the program's effectiveness (Lee et al., 2022).
Internationally, in Japan and Germany, for instance, the governments mainly play a guiding role; like the government of China, they are not overly prescriptive in how the programs should be run. In Japan, for example, the central government sets regulations, provides information, advice, and guidance, and uses market-based mechanisms to guide partnerships between government, universities, enterprises, and other parties to accelerate the development of low-carbon cities.
Some recent studies have examined the relationship between environmental policy and energy efficiency (Chen et al., 2021; Huang et al., 2021). Focusing on the effects of the emissions trading scheme (ETS) on energy efficiency in China, Chen et al. (2021) found that ETS improved single-factor and total-factor energy efficiencies; technological advances underpinned the improvements. Huang et al. (2021) concluded that environmental regulations and government subsidies stimulated research and development expenditures. Other researchers have provided suggestive evidence that the National Energy Efficiency Action Plan (NEEAP) implemented by the European Union played a positive role in improving energy efficiency (Economidou et al., 2022). According to Johansson et al. (2022), the Swedish regional energy efficiency network program doubled energy efficiency in some cases.
Energy efficiency is often called the world’s first fuel. Improving it is the lowest-cost option available to reduce greenhouse gas emissions, energy demand, and the use of renewable energy sources while increasing output and providing a buffer against energy shocks (World Bank, 2017). Given the importance of improving energy efficiency, the paucity of studies examining the effects of the LCCP program on energy efficiency is somewhat surprising. Nevertheless, the program has been examined in various contexts (Cheng et al., 2019; Feng et al., 2021; Song et al., 2020). For example, Yu and Zhang (2021) showed that the LCCP program improved carbon emissions efficiency by 1.7%; to put this into perspective, a 1% improvement reduced carbon emissions by 8.37 million tons, whereas a 2% improvement reduced them by 8.84 million tons. The LCCP program, noted Song et al. (2020), significantly improved ecological efficiency by spurring technological innovation. Cheng et al. (2019) reported similar findings—building low-carbon cities promoted technological progress, industrial restructuring, and green growth. The studies above have surely contributed to our understanding of how environmental policy affects energy efficiency. However, there are still gaps in the literature that warrant attention.
First, the majority of the previous studies have not adequately overcome the challenges of measuring the effectiveness of environmental policies—they suffer from measurement bias. Several approaches have been used to address this issue. For example, Walter and Ugelow (1979) used qualitative indicators, relying mainly on expert or weighted ratings. The data collected in this manner is inherently prone to subjectiveness and the respondents’ judgments and, thus, may lead to incorrect conclusions. Although quantitative secondary data can help researchers obviate these concerns, these data can be hard to obtain, and researchers often have to rely on proxy variables to approximate the information that is actually needed to address research problems. For example, Xing and Kolstad (2002) and Botta and Koźluk, (2014) used pollutant emissions data and Gollop and Roberts (1983) analyzed data on the costs to treat pollutants to examine the impacts of environmental policy. The primary concern with using proxy data may not accurately represent the data they have replaced. Of course, researchers can directly measure the effect of the environmental policy itself, which avoids measurement inaccuracies caused by proxy variables (Levinson, 1996; McConnell & Schwab, 1990). And recent studies have tried to measure the effects of environmental policies in this fashion (Du et al., 2022; Xu & Xu, 2022). However, they have not addressed the endogeneity and selection bias ingrained in environmental initiatives: the very choice of cities in which these initiatives are implemented may be based on how cities may respond to the initiatives and how polluted the cities are, to begin with.
Second, the impact of environmental regulation policies on energy efficiency has been widely discussed in several countries worldwide, including the EU (Economidou et al., 2022; Román-Collado & Economidou, 2021), Sweden (Bertoldi & Mosconi, 2020), and others. The LCCP program has also been studied, and its positive effects on the environment have been documented (Song et al., 2020; Yu & Zhang, 2021). However, due to the lack of energy consumption data on prefectures and cities, previous studies investigating energy efficiency have relied on aggregated data on countries, provinces, or industries, lacking specificity and leading to aggregation bias (Sun et al., 2019).
Methodology
Malmquist-Luenberger index for measuring the total energy efficiency
Data envelopment analysis (DEA) has been applied widely for measuring the total factor efficiency in input–output analyses. However, DEA ignores random errors and does not distinguish statistical noise from inefficiency, leading to inaccurate measurements (Wang & Wang, 2020). Furthermore, it fails to account for pollutants such as wastewater, solid wastes, and carbon emissions that accompany production processes. Ignoring this so-called undesirable output neither captures the energy efficiency of production activities nor their impact on the environment (Seiford and Zhu, 2002).
The cost of pollution should be deducted from the output to reflect the actual GDP. Traditional income accounting methods do not effectively address this issue. DEA, which can account for the undesirable output and input factors, lends itself well to this task (P. Zhou et al., 2008). We have used DEA for two main reasons: first, it allows for the inclusion of undesirable outputs; second, it enables the measurement of dynamic energy efficiency that can be compared across different periods, making it apt for addressing the issue at hand. While other models like the Enhanced Russell-based Directional Distance Measure (ERBDDM) model employed by P. C. Chen et al. (2015) may be more suitable for specific research scenarios, both models can consider undesirable outputs. The key distinction lies in the ERBDDM model’s ability to handle zero inputs and outputs, a condition that does not exist in our dataset. As for handling undesirable outputs within the DEA framework, our approach incorporates them into the production function as additional outputs in a nonlinear model; this aligns with Halkos and Petrou (2019). The Super Slacks-Based Malmquist-Luenberger index, an extension of Tone’s (2001) original Super Slacks-Based model, employs a ratio approach to balance the reduction of undesirable outputs with the increase in desirable outputs. Chung et al. (1997) incorporated undesirable outputs into the DEA model and constructed the Malmquist-Luenberger productivity index. They used the Malmquist-Luenberger index, accounting for both economic output and environmental degradation. This approach lends itself well to our study. Thus, we used the Malmquist-Luenberger index to express undesirable output in terms of industrial sulfur dioxide emissions, industrial fumes emissions, and industrial discharge emissions. The DEA model requires the inputs and outputs to vary in the same proportion, and the choice of the width of the window is mostly based on empirical selection, which is somewhat arbitrary, leading to inaccurate evaluations (Wang & Wang, 2020). Compared with the traditional static DEA method, the super SBM-Malmquist-Luenberger index used in this paper has the following improvements: (1) the measured energy efficiency is a dynamic indicator and comparable across periods; (2) the method not only takes into account the influence of input and output slack on energy efficiency but also eliminates the need to choose the measurement angle. The reasons why we did not adopt the SFA model are listed in Appendix 2.
Suppose there are \(n\) DMUs, and each DMU contains \(m\) inputs; then \(s1\) and \(s2\) are the desirable and undesirable outputs, respectively. The input–output matrix contains \(X=\left[{x}_{1,\cdots ,}{x}_{n}\right]\in {R}^{m\times n},{Y}^{d}=\left[{y}_{1}^{d}\cdots ,{y}_{n}^{d}\right]\in {R}^{s1\times n}\), and \({Y}^{u}=\left[{y}_{1}^{u}\cdots ,{y}_{n}^{u}\right]\in {R}^{s2\times n}\). The Super Slacks-Based model (super SBM) with undesirable outputs is expressed in Eq. (1):
where \({\rho }^{*}\) is the optimal solution of the model and, when \({\rho }^{*}\ge 1\), the DMU is effective; \(\stackrel{-}{x,}\overline{{y }^{d}}\) and \(\overline{{y }^{u}}\) are the slack variables of input, desirable output, and undesirable output, respectively; and \({\lambda }_{j}\) is the weight vector. The Malmquist-Luenberger index from period t to period t + 1 is calculated as follows:
where ML is the calculated Malmquist-Luenberger index, representing the level of total energy efficiency, where \(x\), \(y\), and \(b\) represent input, desirable output, and undesirable output, respectively; \(\overrightarrow{{D}_{0}^{t}}\left({x}^{t},{y}^{t},{b}^{t};{y}^{t},-{b}^{t}\right)\) and \(\overrightarrow{{D}_{0}^{t+1}}\left({x}^{t},{y}^{t},{b}^{t};{y}^{t},-{b}^{t+1}\right)\) are the distance functions of period t and \(t+1\), respectively; \(\overrightarrow{{D}_{0}^{t}}\left({x}^{t+1},{y}^{t+1},{b}^{t+1};{y}^{t},-{b}^{t+1}\right)\) is the distance function of \(t+1\) period under the technical condition of period \(t\); and \(\overrightarrow{{D}_{0}^{t+1}}\left({x}^{t},{y}^{t},{b}^{t};{y}^{t},-{b}^{t}\right)\) is the distance function of \(t\) period under the technical condition of \(t+1\) period.
Difference-in-differences model
We employed the difference-in-differences (DID) method to estimate whether and to what extent the LCCP program increases energy efficiency. Since the model is well-known (Zhou et al., 2022), we only provided a brief summary in the interest of brevity. The DID model first identifies the pilot cities and clubs them into the treatment group. The non-pilot cities constitute the control group. Then, differences in energy efficiency between the treatment and control groups before and after the cities participate in the LCCP program are calculated. The DID approach is expressed in Eq. (3):
where \({EE}_{it}\) represents the total factor energy efficiency by city i in year t; \({lccpprogram}_{it}\) is a dummy variable, which equals 1 if a city participates in the LCCP program, and 0 otherwise; \({control}_{it}\) denotes a vector of variables affecting energy efficiency; \({A}_{i}\) is a vector of city dummies, capturing the city-fixed effects and acknowledging all characteristics in cities that are time-invariant; \({T}_{t}\) is a vector of year dummies, capturing the time-fixed effects; and \({\varepsilon }_{it}\) is the stochastic distribution term.
The DID framework calls for testing two important criteria. First, the treatment and control groups should conform to the parallel trend hypothesis. That is to say that without the LCCP program, the difference in the energy efficiency of the pilot cities and the non-pilot cities would remain unchanged: the trends will be approximately parallel. This paper used the event analysis method to test the parallel trend assumption. The model is specified as follows:
where \({EE}_{it}, {lccpprogram}_{it}\), \({control}_{it}\), \({A}_{i}\), and \({T}_{t}\) are defined previously; \({\mu }_{0}\) is a constant; \({\mu }_{k}\) and \({\chi }_{2}\) are parameters to be estimated; and \(k\) represents the \(k\)-th year after the implementation of the LCCP program. The coefficient of \({\mu }_{k}\) denotes the difference in energy efficiency between pilot cities and non-pilot cities in the \(k\)-th year that is the beginning if the LCCP program. If the trend of \({\mu }_{k}\) is relatively flat during the period of \(k<0\), it conforms to the parallel trend hypothesis. Conversely, if the trend of \({\mu }_{k}\) is significantly increased or decreased during the period of \(k<0\), it shows that the treatment and control groups are different before the start of the LCCP program, which is not in line with the parallel trend hypothesis.
Second, to confirm that changes in energy efficiency are due to the LCCP program rather than other unknown factors, we assigned pilot cities randomly to conduct a placebo test (Li et al., 2016). This allows us to examine how the unobserved and omitted factors influence the baseline regression results. The estimated coefficient of the placebo test can be expressed as follows:
where \({\widehat{\theta }}_{1}\) represents the coefficient of \({lccpprogram}_{it}\); when \(\omega\) equals 0, we can obtain an unbiased estimate of \({\widehat{\theta }}_{1}\), that is, the regression coefficients are not affected by omitted and unobserved variables. However, whether the coefficient is zero cannot be directly verified. Therefore, we adopted an indirect placebo test, which finds a variable to replace \({lccpprogram}_{it}\) that, in theory, does not affect energy efficiency. Specifically, this paper randomly generates a list of the LCCP program cities, resulting in an incorrect estimate: \({\widehat{\theta }}_{1}^{randomselection}\), and repeats this process 1,000 times to generate 1,000 \({\widehat{\theta }}_{1}^{randomselection}\) accordingly. Such randomization ensures that the implementation of the LCCP program does not affect the corresponding energy efficiency, that is, \({\theta }_{1}=0\); \({\widehat{\theta }}_{1}=0\) would imply that \(\omega =0\). If this erroneously estimated variable affects the estimation result, that is. \({\widehat{\theta }}_{1}\ne 0\), then the estimating equation in this paper is deemed incorrect, indicating that other characteristic factors affect the estimates.
PSM-DID approach
Social and economic policies lend themselves poorly to randomized controlled experiments. Thus, quasi-experimental techniques are often applied to study the efficacy of policies and programs. Matching techniques are one example that allows researchers to construct control groups against which they can measure the outcomes for the treatment groups. PSM has been extensively applied in the field of policy analysis (Ferris et al., 2014; Nie et al., 2022). Hence, to further confirm the results from our DID analysis, we employ propensity score matching (PSM) before using the DID method to control for potential selectivity bias.Footnote 1 In particular, we treated control variables as covariates and use one-to-one neighbor matching. Specifically, the outcome variable is the energy efficiency of all cities, the treatment variable is the dummy variable of the LCCP program, and the covariate variable includes all the control variables in the DID estimation. Using PSM, we first derived the probability of a city being chosen as a pilot city:
where \({Probability}_{i}\) refers to the probability that a city is being treated, that is, within the LCCP program, and \({Control}_{it}\) refers to a vector of variables that influence the probability of a city being selected in the treatment group. This study uses the Logit model to estimate \({Probability}_{i}\), and nearest-neighbor matching is conducted for PSM.Footnote 2 The results of PSM help us identify the cities in the control group with similar probabilities to those in the treatment group to be chosen to participate in the LCCP program. After excluding cities without corresponding matches, the differences between pilot and non-pilot cities across the matching variables are insignificant. Thus, PSM addresses the selection bias and ensures that participating cities are randomly selected, thereby improving the accuracy of the DID estimation.
Mediation analysis
We explored whether technological innovation and the rationalizing and upgrading the industry structure mediate the effects of the LCCP program on energy efficiency using the stepwise approach proposed by Baron and Kenny (1986). To be clear, industrial structure refers to the relative concentrations of the primary, secondary, and tertiary sectors. And upgrading the industrial structure involves moving the factors of production to the high-value-added, high-efficiency, and low-consumption industries from the low-value-added, low-efficiency, and high-consumption industries (Pipkin and Fuentes, 2017; Zhu et al., 2019). In the first step, urban technological innovation is regressed on the dummy variable representing the LCCP participation status of cities and control variables. In the second step, urban energy efficiency is used as the dependent variable and technological innovation as the explanatory variable to test the impact of technological innovation on urban energy efficiency. The empirical specification is presented in Eqs. (7)–(9):
where \({innovation}_{it}\) represents the level of technological innovation in city \(i\) in year \(t\), which is captured by the number of innovation patent applications in the cities. The data are collected from the website of the State Intellectual Property Office (2004 to 2016).
We used the same method to examine the mediation effects of transforming the industrial structure through upgrading and rationalizing the industrial structure (Zhang et al., 2019). The Theil index (see Appendix 1 for details) represents the industrial structure rationalization of industrial structure (Zheng et al., 2021); industrial structure upgrades are increases in the ratio of the value of the tertiary sector to the secondary sector.
Data, variables, and descriptive statistics
Data
The data used in this study were collected from the official statistical yearbook published by National Statistical Bureau. Specifically, we gathered data from the China City Statistical Yearbook (2004–2016), the China Energy Statistical Yearbook (2004–2016), and the National Oceanic and Atmospheric Administration (NOAA) of the United States (2004–2016) (https://www.noaa.gov/). To focus on the real effects of program participation, we deflated the nominal variables using 2004 prices. The data span 17 years, from 2004 to 2016, and include 249 cities.Footnote 3 There were gaps in the data on 44 cities, including Sansha, Danzhou, Hong Kong, Macau, and all cities in Tibet and Taiwan. Therefore, these cities are excluded from the analysis.
Measurement of key variables
We employed Eqs. (1) and (2) to calculate energy efficiency. The desirable output includes GDP, and the undesirable outputs include SO2 emissions (i.e., industrial sulfur dioxide (SO2) emissions), smoke emissions (i.e., industrial fumes emissions), and wastewater emissions (i.e., industrial wastewater discharge). We selected these variables drawing upon the literature in this field (Bi et al., 2014; Guo & Yuan, 2020). The city-level GDP is used as the desirable output variable. The undesirable output comprises pollutants resulting from energy consumption. We have three input variables for calculating energy efficiency: labor, capital stock, and energy consumption. Specifically, labor is defined as the number of people employed by the secondary and tertiary industries. The capital stock is measured based on total fixed-asset investment calculated using the perpetual inventory method, as detailed capital input data are unavailable. The variable representing energy consumption is estimated from the Defense Meteorological Satellite Program (DMSP)/Operational Linescan System (OLS) night-time lighting data.
Due to a lack of energy consumption statistics at the municipal level in China, we were unable to obtain the energy consumption of various cities directly. Power consumption is generally regarded as an alternative indicator of energy consumption; however, the data on this indicator is not accurate (Yang & Wei, 2019). Nighttime lights can approximate the energy and power consumption of an area. In fact, using nighttime data to study energy consumption dates back to the 1980s (Foster, 1983; Welch, 1980). Since then, advances have been made to use these data better. For example, Elvidge et al. (1997) and Elvidge et al. (2001) constructed logit models to examine correlations between regional light grayscale values and regional electric energy consumption using the DMSP/OLS nighttime light data. Letu et al. (2009) validated the feasibility of nighttime light data in estimating electricity consumption by analyzing the correlation between electricity consumption and nighttime light intensity in Asian countries. Since 2010, Chinese scholars have focused on using time-series nighttime lighting data to construct accurate inverse models of electricity consumption for spatial and temporal dynamics of energy consumption (He et al., 2014; Shi et al., 2018).
In this paper, we did not directly use nighttime lighting data to replace electricity or energy consumption data for prefecture-level municipalities. Instead, we obtained total energy consumption data for each province from the statistical yearbooks. The details are presented in Eq. (12) in Appendix 1.
Following previous studies (Yang et al., 2016; Yu & Zhang, 2021), we chose six commonly used control variables in the DID model. They are as follows: (1) per capita GDP, measured as the natural logarithm of per capita GDP, to represent the economic development of each prefecture-level city; (2) SO2 emissions, measured as the natural logarithm of the industrial sulfur dioxide emissions, to represent various sources of pollution in different cities; (3) energy consumption, measured using the Nighttime Light data; (4) population density, measured by the natural logarithm of average population per square kilometer of each city's administrative area; (5) industrial structure, proxied by the proportion of the industrial value-added to the GDP in each city; and (6) foreign direct investment (FDI), measured by the natural logarithm of the FDI denominated in RMB, using the relevant RMB-USD exchange rate.
Descriptive statistics
Table 1 presents the definitions and descriptive statistics of the variables. The average yearly energy efficiency per city over the sample period was 0.863, with a standard deviation of 0.127, a minimum of 0.469, and a maximum of 1.243. This points to significant differences in energy efficiency across cities, which provides a basis for examining the impact of the LCCP program on energy efficiency. We found that fewer than half of the cities are nearing the end of their participation in the LCCP program. Thus, there are opportunities to optimize the program and glean insights to inform future climate-friendly endeavors.
The average per capita GDP was 9.853, with a standard deviation of 0.705. We also found significant differences in FDI across cities. Specifically, on average, the FDI was 11.414; it ranged from a minimum of 6.3031 to a maximum of 15.321, indicating a considerable range across the cities. The average population density was also widely dispersed, ranging from 3.616 to 7.213 (Tan et al., 2008). In addition, the bottom panel of Table 1 reports the variables used in the heterogeneity and mediation analyses.
Results and discussions
Impacts of the LCCP program on energy efficiency
Table 2 presents the regression results for the impacts of the LCCP program on energy efficiency. Three different specifications were considered. In Model (1), we did not include the six control variables but controlled for the year- and city-fixed effects. In Model (2), we included the control variables and year fixed effects but did not consider the city fixed effects. In Model (3), we included the control variables and controlled for both year-fixed effects and city-fixed effects. The Akaike Information Criterion (AIC) is used to identify the appropriate model: accordingly, Model (3) is chosen for the analysis—it is associated with the lowest AIC.
The results show that the coefficient of the LCCP program was positive and statistically significant, suggesting that the LCCP program significantly improved energy efficiency. In other words, on average, the LCCP pilot cities use energy more efficiently than non-pilot cities. Two reasons can help explain the findings. First, comprising a comprehensive suite of environmental regulation policies at the city level, the LCCP program promoted the use of clean energy and materials, the adoption of advanced technology and equipment, and the optimization of production processes. The government launched a series of environmental regulation policies within the LCCP program to regulate the production behaviors of enterprises and increase the cost of polluting the environment. Higher costs and stringent regulatory requirements encouraged enterprises to adopt green technologies (Shao et al., 2020), such as updating equipment and adopting energy-saving technologies, thus improving energy efficiency. Second, enterprises generating considerable pollution due to high energy consumption seized to operate, as they could not conform to environmental protection regulations. This led to a reallocation of factors of production to enterprises that met environmental standards. Access to more capital and labor spurred innovation within these enterprises, leading to improvement in energy efficiency.
The coefficients of control variables also show some interesting findings. For example, the coefficient of per capita GDP is positive and statistically significant, suggesting that the higher the level of economic development, the larger the effect of the LCCP program on energy efficiency. At the same time, the coefficient of SO2 emissions is negative and statistically significant at the 1% level. This indicates that the LCCP program was less effective in improving energy efficiency in cities with high levels of SO2 emissions. Wu and Lin (2022) have found similar results. The coefficient of energy consumption is significant and positive—the higher the energy consumption of an area, the more successful the LCCP program is in increasing its energy efficiency. That areas consuming more energy stand to benefit more from the program is a welcome sign and bodes well for the program as a whole, as such areas are also likely to generate more pollution. The program’s success in high energy-consumption areas may be attributed to economies of scale in energy production: large energy infrastructure is more efficient than smaller ones. The coefficient of FDI is negative and statistically significant, suggesting that an increase in FDI is associated with lower energy efficiency. This may reflect the transfer of foreign pollution-intensive industries to China. This reasoning is consistent with the pollution haven hypothesis.
Diagnostic tests
Results of the parallel test
Figure 1 illustrates the results of the parallel trend test. It shows that before the implementation of the LCCP program (from d_6 to d_1 on the x-axis), the energy efficiency between the pilot cities and non-pilot cities was not significantly different.Footnote 4 Thus, the parallel trend condition is satisfied. Figure 1 also shows that in the first two years of the program (i.e., d1 and d2 along the x-axis), the pilot and non-pilot cities still had no significant difference in energy efficiency. From the third year onwards (i.e., d3), energy efficiency is notably different across pilot and non-pilot cities. Technological innovation, an important driver of energy efficiency, is complex and often gradual. Thus, the effects of innovation become do not become evident immediately. It can take some years before it bears fruit (Wang & Wang, 2020). Previous research has also shown that the implementation of the LCCP program had a significant impact on carbon emission efficiency and industrial supererogation after 2012 (Zheng et al., 2021). Our results are consistent with prior research on this subject.
Results of the placebo test
Figure 2 illustrates the results of the placebo test, showing the mean values of regression estimates after random assignment. It demonstrates that the average cost of all estimated coefficients of the LCCP program was almost zero. The findings indicate that the LCCP program had no significant effect on energy efficiency from the perspective of random sampling experiments. Therefore, the regression results in this study are unlikely to be driven by unknown factors.
In general, our findings in Figs. 1 and 2 confirm that there was no significant difference between the treatment group (i.e., pilot cities) and the control group (i.e., non-pilot cities) before the implementation of the LCCP program. Therefore, the DID approach employed in the “Impacts of the LCCP program on energy efficiency” section is appropriate to estimate the impact of the program on energy efficiency.
Robustness checks
The baseline regression results suggest that the LCCP program improved the energy efficiency of the pilot cities. We confirmed this result using the parallel trend test and the placebo test. To be sure, the parallel trend test indicated that there was no significant difference between pilot cities and non-pilot cities before the implementation of the LCCP program. However, even if the parallel-trend condition is satisfied, it does not mean that the LCCP program improved energy efficiency. The changes in energy efficiency may have been affected by other a multitude of factors: changes in the attitudes of people; policies implemented in some cities and not in others; and a greater awareness of the environmental problems arising from inefficient energy use. Therefore, we conducted a placebo test by randomly generating a treatment group to determine whether the improvements in energy efficiency ascribed to the LCCP program were, in fact, due to other factors.
Elimination of interference from other policies
Our focus is on examining the impact of the LCCP program on energy efficiency from 2004 to 2016. However, the LCCP was not the only program designed to address environmental quality in China implemented during this period. The possible effects of other environmental policies pursued during this time interval, particularly area-based environmental policies, are indeed relevant to our results. It bears emphasis that other policies and pilots, including New Energy Vehicles Pilot (NEVP), Air Quality Monitoring Standards (AQMS), and Special Emission Limits for Air Pollutants (SELAP), were also implemented during the same period.Footnote 5 In 2011, various departments jointly issued a notice on pilot Subsidies for Private Buyers of New Energy Vehicles. Pilots were proposed for 26 cities, a subset of which also participated in the LCCP program. In 2012, the Ministry of Environmental Protection issued the First-Stage Monitoring Implementation Plan for New Air Quality Standards, to increase compliance with measures designed to improve the air quality in the Beijing-Tianjin-Hebei region, the Yangtze River Delta, the pearl River Delta, and other key regions, as well as in municipalities directly under the Central Government and provincial capital cities. In 2013, the Ministry of Environmental Protection released the Notice on the Implementation of special Emission Limits of Air Pollutants, which stipulates the scope of key monitoring areas.
These policies may also have affected energy efficiency, and this possibility should be considered to study the effects of the LCCP program. Following Zhang et al. (2022), to control for the effect of the NEVP, an interaction term \({NEVP}_{i}\times {Post}_{t}\) was included in the regression model (3), where \({NEVP}_{i}\) is a dummy variable equal to 1 for cities participating in the NEVP and 0 otherwise. \({Post}_{t}\) is also a dummy variable capturing the year during which the NEVP was implemented; it is assigned a value of 1 for every year from 2012 onward. Similarly, we also included two interaction terms, \({AQMS}_{i}\times {Post}_{t}\) and \({SELAP}_{i}\times {Post}_{t}\), into the regression model Table 6.
Column 2 of Table 6 presents the empirical results. The results show that the coefficients of the three policy variables are statistically insignificant. Besides, the coefficient of the LCCP program is still significant and positive at the 1% level after controlling for the potential effects on the energy efficiency of other policies (NEVP, AQMS, and SELAP). These findings confirm that specific regional policies do not engender an upward bias in the impact of the LCCP program on energy efficiency.
Results of the PSM-DID model
The DID method requires that the pilot cities and non-pilot cities be selected randomly; otherwise, the method yields biased results. However, the practical realities do not lend themselves well to random selection. In fact, the LCCP pilot cities are selected for specific reasons: a city may have a strong representation holding sway or high energy consumption (Fu et al., 2021). To address the potential selectivity bias, we employed the PSM-DID method to study the impact of the LCCP program on energy efficiency. The results, which are presented in the last column of Table 6 in Appendix 2, show that the coefficient of the LCCP program is positive and statistically significant. This result confirms the positive effects of the LCCP program on energy efficiency.
Heterogeneous effects
Considering that different regional properties may affect our results, we also examine the impacts of the LCCP program on energy efficiency in different regions with different resource endowments. Columns 2–4 of Table 3 show the effects of the LCCP program on energy efficiency regionally. The estimates reveal that the LCCP program had a positive and significant impact on energy efficiency in the eastern parts of China. In comparison, the program’s impact on the energy efficiency of central and western parts of China was insignificant. These findings stand to reason. Eastern China is the most economically developed region in the country; it is technologically advanced and has the most educated people. Thus, the region is well-positioned to absorb new ideas and adapt to change, allowing government policies to gain traction relatively quickly. Furthermore, industrial agglomeration in eastern China is likely to have contributed to energy efficiency in the region (Li et al., 2020; Liu et al., 2017). This accords with Q. Zheng and Lin (2018), who found that industrial agglomeration positively affected industrial energy efficiency. Zhao and Lin (2019) found that promoting industrial agglomeration improved energy efficiency even when industrial agglomeration was low.
Our results are consistent with Lin et al.(2021), who concluded that the high-tech industry in eastern China is significantly more energy efficient than the rest of the country. In contrast, traditional industries dominate in central and western China, while high-tech industries occupy a higher proportion in eastern China. These factors could explain why the LCCP program was less effective in China’s central and western regions than in its eastern region in improving energy efficiency.
Cities in regions endowed with natural resources attract resource-intensive industries. Having downstream and administrative operations nearby upstream activities is advantageous on many accounts. Furthermore, proximity to natural resources may also increase the dependence on resource-intensive industries, contributing to high energy consumption and low energy efficiency. Therefore, we investigate the heterogeneous effects of the LCCP program on the energy efficiency of cities based on their resource endowments. To this end, we classified our sample cities into resource-based and non-resource-based cities following the National Sustainable Development Plan for Resource-Based Cities (2013–2020) issued by the State Council of China in 2013.
The findings are reported in the last two columns of Table 3. The results show that the LCCP program had a positive and significant impact on the energy efficiency of non-resource-based cities but had no significant impact on resource-based cities. Previous studies have found that the majority of high-tech sectors, such as communications equipment, computers, and other electronic devices, are not energy-intensive (Arslan et al., 2022). The cities hosting these sectors are well-positioned to improve energy efficiency. Some previous studies have argued that over-reliance on resource-based industries in the local economy have stifled economic growth and innovation (Zhang et al., 2009). Moreover, studies have confirmed these results for different regions in China (Cheng et al., 2020). This result chimes with the resource curse hypothesis—an abundance of resources may stifle growth and development, preventing resource-rich regions from reaching their full socio-economic potential. These cities usually lack the incentives to transition from resource-intensive industries. Such transitions are slow and expensive, making them all the more difficult. This is not conducive to improving energy efficiency. On the other hand, non-resource-based cities are not tethered to the resource sector. Thus, they can adapt to programs and initiatives and assimilate technologies designed to improve energy efficiency. The results highlight the importance of considering how dependent cities are on natural resources when formulating low-carbon policies.
Mechanism analysis
Mediation effects of technological innovation
Table 4 presents the results capturing the mediation effects of technological innovation on energy efficiency. Column 2 of Table 4 shows that the coefficient of innovation was positive and statistically significant at the 5% level, suggesting that technological innovation significantly improved energy efficiency. When firms increase energy use, they generate more emissions and consequently pay higher environmental taxes and fines and lose the opportunity to receive subsidies. Firms innovate and invest in research and development to improve energy efficiency to avoid these consequences.
Column 3 of Table 4 shows that the LCCP program significantly increased technological innovation, and the effect is statistically significant at the 1% level. When faced with environmental regulations, firms reduce emissions by implementing environmentally friendly practices and policies and adopting green technologies. However, this may increase production costs and dissuade firms from investing in research and development, which is critical to effecting lasting change. Also, because of the inherent risk in innovation, firms may struggle to raise capital to fund their projects. Thus, to encourage innovation and research, the government should introduce targeted fiscal and tax policies conducive to developing low-carbon industries and support innovative enterprises through subsidies and other means. The market-based incentives of the LCCP program are mainly used to internalize the cost of controlling pollution through market-based instruments such as subsidies and carbon trading (Bergquist et al., 2013), which, in turn, motivate enterprises to improve energy efficiency through technological innovation. Energy-efficient companies can sell their excess carbon emission allowances to finance their investment in innovative technologies. In addition, government subsidies in market-based policy instruments can reduce the risk that technological innovation entails and alleviate financial constraints for enterprises, thus creating an environment suitable for research and innovation (Shao & Chen, 2022).
Mediation effects of rationalizing and upgrading the industrial structure
Table 5 shows the mediation effects of rationalizing and upgrading the industrial structure on energy efficiency. The results show that industrial structure rationalization did not affect energy efficiency significantly but did (see columns 2 and 5 of Table 5). Therefore, we further analyzed the impact of the LCCP program on upgrades to the industrial structure. The results are presented in Column 4 of Table 5. They show that the program promoted upgrades to the industrial structure. The last column of Table 5 shows that, even after controlling for the effects of these changes, the coefficient of the LCCP program is still positive and significant. This result suggests that the LCCP program improved energy efficiency regardless of upgrades to the industrial structure. That is not to say that such changes cannot improve energy efficiency. Rather, on the contrary, they are likely to do so. Command-and-control instruments in the LCCP program are designed to eliminate outdated production capacity, set up new industries, and establish emission standards. Strict environmental regulation policies increase the cost of emissions and pollution control for enterprises and raise the costs to enter and operate in industries that are unfriendly to the environment, thus paving the way for a transition to cleaner industries (Yu & Wang, 2021).
Conclusions and policy implications
The rapid economic development globally has increased energy consumption, contributing to environmental degradation and climate change, raising questions about the sustainability of economic growth. Recognizing the gravity of the problem, China launched the Low Carbon City Pilot (LCCP) program in 2010 to reduce carbon emissions. This paper is devoted to analyzing how effective this program has been in improving energy efficiency, which is critical to its success. The study analyzed panel data from 249 Chinese cities over the period 2004–2016, using the difference-in-differences approach. The instrumental variable and propensity-score-matching-difference-in-differences (PSM-DID) methods are used to confirm the robustness of the results, and the Super Slacks-Based Malmquist-Luenberger index is used to measure energy efficiency.
The results are promising, showing that the LCCP program significantly improved energy efficiency. The results were robust across the three modeling frameworks. The LCCP program improved energy efficiency via technological innovation and upgrades to the industrial structure. Disaggregated analyses showed that the program was more effective in cities in the eastern part of China and those not located in resource-based areas. Emissions tended to increase with energy consumption, and participating cities with higher energy consumption experienced more considerable improvements in energy efficiency—this bodes well for the program, as it was more successful in cities that most needed it. The program was also more effective in cities with higher per capita income. On the other hand, higher foreign direct investment and sulfur dioxide emissions lessened its effectiveness. Technological innovation and upgrades to the industrial structure mediated the positive effects of the LCCP program.
These findings have practical implications. First, they underscore the value of subsidies and tax incentives to encourage research and development and spur green innovation. This will expedite a transition to clean technologies, boost local economies, and improve living standards. As technologies advance, traditional approaches to saving energy, such as planned power outages, will become passe. Second, they highlight the importance of customizing the program to regional needs—the LCCP program has been less effective in China’s western and central regions. Third, the findings bring foreign direct investment into sharper focus, pointing to its potential downsides. Foreign direct investment tempered the effectiveness of the LCCP program. This is plausibly a manifestation of the pollution haven hypothesis—advanced economies transfer production processes to developing countries to circumvent strict environmental regulations. Thus, screening criteria for FDI to ensure its consistency with ecological sustainability should be evaluated. Fourth, the findings show that cities in resource-rich regions have not benefitted as much from the program. These cities warrant special attention. Sustained efforts and long-term investments are in order as successful energy transitions happen gradually.
Consider the main result: the LCCP program improves energy efficiency. This is uplifting and encouraging—pro-environment programs are achieving their objectives. We propose that these programs be vetted, improved further, and scaled, so future iterations can prove even more beneficial to China’s efforts to tackle climate change. We showed that the LCCP program improved energy efficiency by promoting technological innovation and motivating upgrades to the industrial structure—these may be the proximate causes underpinning the improvements in energy efficiency and deserve attention in their own right. Accordingly, China’s government would be well-advised to nurture innovation and facilitate and reward efforts to upgrade the industrial structure in the country. Because innovation is inherently risky and private enterprises may be unwilling to expose themselves to the risk, the government may step in to cover the risk to spur innovation; this could be done in partnership and consultation with venture capital firms, which have experience in identifying promising ideas.
Last, we want to draw attention to some limitations of this study. First, we concentrated on two channels through which the LCCP program can influence energy efficiency: technological innovation and industrial structure. Other channels, such as governance, corruption, business friendliness, and fiscal autonomy of the provinces, were not examined. We leave examinations to future research. Second, the third batch of the LCCP program cities was not included in this paper, as the requisite data were unavailable. Thus, the results are not up-to-date. It would be insightful to re-estimate the models above to include data from the latest batch of cities. In fact, we propose that the performance of initiatives such as the LCCP program be monitored regularly. Third, the accuracy and granularity of the data on energy consumption are foundational to studying the effectiveness of initiatives such as the LCCP program. We have used data on total energy consumption for each province. The correspondence between these data and those on the energy consumption of specific industries may not be strong; more detailed data on energy consumption, for example, energy consumed in areas with the same postal code would be more insightful. As and when these data become available, studies that use them will improve our understanding of how well pro-environment policies and programs perform. We want to acknowledge that despite our results being robust to using alternate model specifications, they are not entirely devoid of the effects of confounding factors, especially of the effects of other contemporaneous environmental policies designed to improve energy efficiency.
Data availability
Data and computer code that support the findings of this study are available upon request from the corresponding author.
Notes
In our analysis below, we provide evidence supporting a parallel trend between the pilot and non-pilot cities, indicating that the DID approach is appropriate to estimate the impact of the LCCP program on energy efficiency. The reason for adopting the PSM-DID method is only to further validate the results of the DID model.
We performed robustness checks using alternative matching methods and found the main results unchanged.
The three batches of pilot cities are listed in Table 7 in the Appendix. We chose the first two batches of pilot cities for our research. There are two reasons for this: first, the data on industrial waste gas, sulfur dioxide, and dust data for some variables are only available up to 2016; second, there are differences in the administrative levels included in the third batch of pilot cities; for example, Gongqingcheng, Yining, Sunk, and Qiongzhong belong to county-level administrative units, while the other cities belong to prefecture-level cities, and the two types of cities may have large differences in various characteristics, which are not suitable for comparison with other cities. Therefore, the third batch of pilots was not included in the scope of the study.
The coefficient in Fig. 1 is not statistically significant when the confidence interval contains zero.
The list of cities for the NEVP, AQMS, and SELAP were obtained from the Chinese government’s websites: http://www.gov.cn/gzdt/2010-06/04/content_1620735.htm; https://www.mee.gov.cn/gkml/hbb/bgt/201205/t20120524_230080.htm; and https://www.mee.gov.cn/gkml/hbb/bgg/201303/t20130305_248787.htm.
We utilized the wind speed at 10 m height and boundary layer height (as a proxy for the mixing height for a global grid of 75°*75°cells) obtained from the ERA-Interim database to match the cities using latitudes and longitudes obtained from world-gazetteer.com.
References
Arslan, A., Ahokangas, P., Haapanen, L., Golgeci, I., Tarba, S. Y., & Bazel-Shoham, O. (2022). Generational differences in organizational leaders: An interpretive phenomenological analysis of work meaningfulness in the Nordic high-tech organizations. Technological Forecasting and Social Change, 180(April), 121717.
Baker, A. C., Larcker, D. F., & Wang, C. C. Y. (2022). How much should we trust staggered difference-in-differences estimates? Journal of Financial Economics, 144(2), 370–395.
Baron, R. M., & Kenny, D. A. (1986). The moderator-mediator variable distinction in social psychological research: conceptual, strategic, and statistical considerations. Journal of Personality and Social Psychology, 51(6), 1173–1182.
Bergquist, A. K., Söderholm, K., Kinneryd, H., Lindmark, M., & Söderholm, P. (2013). Command-and-control revisited: Environmental compliance and technological change in Swedish industry 1970–1990. Ecological Economics, 85, 6–19.
Bertoldi, P., & Mosconi, R. (2020). Do energy efficiency policies save energy? A new approach based on energy policy indicators (in the EU Member States). Energy Policy, 139(October 2018), 111320.
Bi, G. B., Song, W., Zhou, P., & Liang, L. (2014). Does environmental regulation affect energy efficiency in China’s thermal power generationα empirical evidence from a slacks-based DEA model. Energy Policy, 66, 537–546.
Botta, E., & Koźluk, T. (2014). Measuring environmental policy stringency in OECD countries. OECD Economics Department Working Papers No, 1177(1177), 47.
Broner, F., Bustos, P., & Carvalho, V. M. (2012). Sources of comparative advantage in polluting industries (NBER Working Paper No. 18337). National Bureau of Economic Research.
Chen, P. C., Yu, M. M., Chang, C. C., Hsu, S. H., & Managi, S. (2015). The enhanced Russell-based directional distance measure with undesirable outputs: Numerical example considering CO2 emissions. Omega (united Kingdom), 53, 30–40.
Chen, Z., Song, P., & Wang, B. (2021). Carbon emissions trading scheme, energy efficiency and rebound effect – evidence from China’s provincial data. Energy Policy, 157, 112507.
Cheng, Z., Li, L., & Liu, J. (2020). Natural resource abundance, resource industry dependence and economic green growth in China. Resources Policy, 68(May), 101734.
Cheng, J., Yi, J., Dai, S., & Xiong, Y. (2019). Can low-carbon city construction facilitate green growth? Evidence from China’s pilot low-carbon city initiative. Journal of Cleaner Production, 231, 1158–1170.
Chung, Y. H., Färe, R., & Grosskopf, S. (1997). Productivity and undesirable outputs: a directional distance function approach. Journal of Environmental Management Journal of Environmental Management, 51(3), 229–240.
Du, W., Li, M., & Wang, Z. (2022). The impact of environmental regulation on firms’ energy-environment efficiency: Concurrent discussion of policy tool heterogeneity. Ecological Indicators, 143(March), 109327.
Economidou, M., Ringel, M., Valentova, M., Castellazzi, L., Zancanella, P., Zangheri, P., et al. (2022). Strategic energy and climate policy planning: Lessons learned from European energy efficiency policies. Energy Policy, 171, 113225.
Elvidge, C. D., Baugh, K. E., Kihn, E. A., Kroehl, H. W., Davis, E. R., & Davis, C. W. (1997). Relation between satellite observed visible-near infrared emissions, population, economic activity and electric power consumption. International Journal of Remote Sensing, 18(6), 1373–1379.
Elvidge, C. D., Hobson, V. R., Baugh, K. E., Dietz, J. B., Shimabukuro, Y. E., Krug, T., et al. (2001). DMSP-OLS estimation of tropical forest area impacted by surface fires in Roraima, Brazil: 1995 versus 1998. International Journal of Remote Sensing, 22(14), 2661–2673.
Feng, T., Lin, Z., Du, H., Qiu, Y., & Zuo, J. (2021). Does low-carbon pilot city program reduce carbon intensity? Evidence from Chinese cities. Research in International Business and Finance, 58, 101450.
Ferris, A., Shadbegian, R., & Wolverton, A. (2014). The effect of environmental regulation on employment: An examination of the 1990 Clean Air Act amendments and its impact on the electric power sector (No. 2168-2018-8153).
Foster, J. L. (1983). Observations of the Earth using nighttime visible imagery. In Proc SPIE, 0414, 187–193.
Fu, Y., He, C., & Luo, L. (2021). Does the low-carbon city policy make a difference? Empirical evidence of the pilot scheme in China with DEA and PSM-DID. Ecological Indicators, 122, 107238.
Gollop, F. M., & Roberts, M. J. (1983). Environmental regulations and productivity growth: The case of fossil-fueled electric power generation. Journal of Political Economy, 91(4), 654–674.
Guo, R., & Yuan, Y. (2020). Different types of environmental regulations and heterogeneous influence on energy efficiency in the industrial sector: evidence from Chinese provincial data. Energy Policy, 145, 111747.
Halkos, G., & Petrou, K. N. (2019). Treating undesirable outputs in DEA: A critical review. Economic Analysis and Policy, 62, 97–104.
He, C., Ma, Q., Liu, Z., & Zhang, Q. (2014). Modeling the spatiotemporal dynamics of electric power consumption in mainland China using saturation-corrected DMSP/OLS nighttime stable light data. International Journal of Digital Earth, 7(12), 993–1014.
Hering, L., & Poncet, S. (2014). Environmental policy and exports: Evidence from Chinese cities. Journal of Environmental Economics and Management, 68(2), 296–318.
Hong, Q., Cui, L., & Hong, P. (2022). The impact of carbon emissions trading on energy efficiency: evidence from quasi-experiment in China’s carbon emissions trading pilot. Energy Economics, 110(March 2021), 106025.
Huang, J., Yu, Y., & Ma, C. (2018). Energy efficiency convergence in China: Catch-up, lock-in and regulatory uniformity. Environmental and Resource Economics, 70(1), 107–130.
Huang, Jingchang, Zhao, J., & Cao, J. (2021). Environmental regulation and corporate R&D investment—evidence from a quasi-natural experiment. International Review of Economics & Finance, 72, 154–174.
International Energy Agency. (2021). E4 country profile: energy efficiency in China. IEA. Retrieved January 7, 2022, from https://www.iea.org/articles/e4-country-profile-energy-efficiency-in-china
IEA. (2022). Global energy review: CO2 emissions in 2021. IEA. https://iea.blob.core.windows.net/assets/c3086240-732b-4f6a-89d7-db01be018f5e/GlobalEnergyReviewCO2Emissionsin2021.pdf
IEA. (2020). Energy Efficiency 2020. IEA. https://iea.blob.core.windows.net/assets/59268647-0b70-4e7b-9f78-269e5ee93f26/Energy_Efficiency_2020.pdf
Jaffe, A. B., Newell, R. G., & Stavins, R. N. (2005). A tale of two market failures: Technology and environmental policy. Ecological Economics, 54(2–3), 164–174.
Johansson, I., Johnsson, S., & Thollander, P. (2022). Impact evaluation of an energy efficiency network policy programme for industrial SMEs in Sweden. Resources, Environment and Sustainability, 9(December 2021), 100065.
Lee, C. C., Feng, Y., & Peng, D. (2022). A green path towards sustainable development: The impact of low-carbon city pilot on energy transition. Energy Economics, 115(July), 106343.
Letu, H., Hara, M., Yagi, H., Tana, G., & Nishio, F. (2009). Estimating the energy consumption with nighttime city light from the DMSP/OLS imagery. In 2009 Joint Urban Remote Sensing Event (pp. 1–7). IEEE.
Levinson, A. (1996). Environmental regulations and manufacturers’ location choices: Evidence from the census of manufactures. Journal of Public Economics, 62(1–2), 5–29.
Li, P., Lu, Y., & Wang, J. (2016). Does flattening government improve economic performance? Evidence from China. Journal of Development Economics, 123, 18–37.
Li, C., Wu, K., & Gao, X. (2020). Manufacturing industry agglomeration and spatial clustering: Evidence from Hebei Province, China. Environment, Development and Sustainability, 22(4), 2941–2965.
Li, Z., Yang, C., & Huang, Z. (2022). How does the fintech sector react to signals from central bank digital currencies? Finance Research Letters, 50(21), 103308.
Lin, S., Lin, R., Sun, J., Wang, F., & Wu, W. (2021). Dynamically evaluating technological innovation efficiency of high-tech industry in China: provincial, regional and industrial perspective. Socio-Economic Planning Sciences, 74(February 2020), 100939.
Liu, J., Cheng, Z., & Zhang, H. (2017). Does industrial agglomeration promote the increase of energy efficiency in China? Journal of Cleaner Production, 164, 30–37.
Machiba, T. (2010). Eco-innovation for enabling resource efficiency and green growth: Development of an analytical framework and preliminary analysis of industry and policy practices. International Economics and Economic Policy, 7(2), 357–370.
McConnell, V. D., & Schwab, R. M. (1990). The impact of environmental regulation on industry location decisions: The motor vehicle industry. Land Economics, 66(1), 67–81.
Mulder, P., & de Groot, H. L. F. (2012). Structural change and convergence of energy intensity across OECD countries, 1970–2005. Energy Economics, 34(6), 1910–1921.
Nie, F., Li, J., Bi, X., & Li, G. (2022). Agricultural trade liberalization and domestic fertilizer use: evidence from China-ASEAN free trade agreement. Ecological Economics, 195(July 2021), 107341.
Pipkin, S., & Fuentes, A. (2017). Spurred to Upgrade: A Review of Triggers and Consequences of Industrial Upgrading in the Global Value Chain Literature. World Development, 98, 536–554.
Qin, M., Sun, M., & Li, J. (2021). Impact of environmental regulation policy on ecological efficiency in four major urban agglomerations in eastern China. Ecological Indicators, 130, 108002.
Román-Collado, R., & Economidou, M. (2021). The role of energy efficiency in assessing the progress towards the EU energy efficiency targets of 2020: evidence from the European productive sectors. Energy Policy, 156, 112441.
Roth, J., Sant’Anna, P. H. C., Bilinski, A., & Poe, J. (2022). What’s trending in difference-in-differences? A synthesis of the recent econometrics literature, 1–58.
Seiford, L. M., & Zhu, J. (2002). Modeling undesirable factors in efficiency evaluation. European Journal of Operational Research, 142(1), 16–20.
Shan, Y., Liu, J., Liu, Z., Shao, S., & Guan, D. (2019). Data descriptor: An emissions-socioeconomic inventory of Chinese cities. Scientific Data, 6, 1–10.
Shao, Y., & Chen, Z. (2022). Can government subsidies promote the green technology innovation transformation? Evidence from Chinese listed companies. Economic Analysis and Policy, 74, 716–727.
Shao, S., Zhang, Y., Tian, Z., Li, D., & Yang, L. (2020). The regional Dutch disease effect within China: A spatial econometric investigation. Energy Economics, 88, 104766.
Shi, K., Yu, B., Huang, C., Wu, J., & Sun, X. (2018). Exploring spatiotemporal patterns of electric power consumption in countries along the Belt and Road. Energy, 150, 847–859.
Shixiang Li, J. C. (2008). Study on the energy efficiency of China and its determinants. Statistical Research, 25(10), 18–27.
Smil, V. (2014). A global transition to renewable energy will take many decades. Scientific American, 310(1), 52–57.
Smil, V. (2021). Numbers don’t lie. IEEE Spectrum.
Song, M., Zhao, X., & Shang, Y. (2020). The impact of low-carbon city construction on ecological efficiency: empirical evidence from quasi-natural experiments. Resources, Conservation and Recycling, 157, 104777.
Sun, H., Edziah, B. K., Sun, C., & Kporsu, A. K. (2019). Institutional quality, green innovation and energy efficiency. Energy Policy, 135(February), 111002.
Tan, M., Li, X., Lu, C., Luo, W., Kong, X., & Ma, S. (2008). Urban population densities and their policy implications in China. Habitat International, 32(4), 471–484.
Tone, K. (2001). A slacks-based measure of efficiency in data envelopment analysis. European Journal of Operational Research, 130(3), 498–509.
Walter, I., & Ugelow, J. L. (1979). Environmental policies in developing countries. Ambio, 8, 102–109.
Wang, Y., Song, Q., He, J., & Qi, Y. (2015). Developing low-carbon cities through pilots. Climate Policy, 15, 81–103.
Wang, H., & Wang, M. (2020). Effects of technological innovation on energy efficiency in China: evidence from dynamic panel of 284 cities. Science of the total environment, 709, 136172.
Welch, R. (1980). Monitoring urban population and energy utilization patterns from satellite data. Remote Sensing of Environment, 9(1), 1–9.
World Bank. (2017). Energy efficiency. World bank. Retrieved January 12, 2021, from https://www.worldbank.org/en/results/2017/12/01/energy-efficiency
Wu, R., & Lin, B. (2022). Environmental regulation and its influence on energy-environmental performance: evidence on the Porter Hypothesis from China’s iron and steel industry. Resources, Conservation and Recycling, 176, 105954.
Xing, Y., & Kolstad, C. D. (2002). Do lax environmental regulations attract foreign investment? Environmental and Resource Economics, 21(1), 1–22.
Xiong, S., Ma, X., & Ji, J. (2019). The impact of industrial structure efficiency on provincial industrial energy efficiency in China. Journal of Cleaner Production, 215, 952–962.
Xu, B., & Xu, R. (2022). Assessing the role of environmental regulations in improving energy efficiency and reducing CO2 emissions: Evidence from the logistics industry. Environmental Impact Assessment Review, 96(February), 106831.
Yang, Z., & Wei, X. (2019). The measurement and influences of China’s urban total factor energy efficiency under environmental pollution: Based on the game cross-efficiency DEA. Journal of Cleaner Production, 209, 439–450.
Yang, X., Wang, S., Zhang, W., Li, J., & Zou, Y. (2016). Impacts of energy consumption, energy structure, and treatment technology on SO2 emissions: A multi-scale LMDI decomposition analysis in China. Applied Energy, 184, 714–726.
Yu, Y., & Zhang, N. (2021). Low-carbon city pilot and carbon emission efficiency: Quasi-experimental evidence from China. Energy Economics, 96, 105125.
Yu, X., & Wang, P. (2021). Economic effects analysis of environmental regulation policy in the process of industrial structure upgrading: Evidence from Chinese provincial panel data. Science of the Total Environment, 753(5677), 142004.
Zhang, X., Xing, L., Fan, S., & Luo, X. (2009). Resource abundance and regional development in China. Regional Inequality in China: Trends, Explanations and Policy Responses, 16(1), 99–120.
Zhang, P., Shi, X. P., Sun, Y. P., Cui, J., & Shao, S. (2019). Have China’s provinces achieved their targets of energy intensity reduction? Reassessment based on nighttime lighting data. Energy Policy, 128, 276–283.
Zhang, S., Zhang, M., Qiao, Y., Li, X., & Li, S. (2022). Does improvement of environmental information transparency boost firms ’ green innovation ? Evidence from the air quality monitoring and disclosure program in China. Journal of Cleaner Production, 357(April), 131921.
Zhao, H., & Lin, B. (2019). Will agglomeration improve the energy efficiency in China’s textile industry: evidence and policy implications. Applied Energy, 237(Jaunary 2018), 326–337.
Zheng, J., Shao, X., Liu, W., Kong, J., & Zuo, G. (2021). The impact of the pilot program on industrial structure upgrading in low-carbon cities. Journal of Cleaner Production, 290, 125868.
Zheng, Q., & Lin, B. (2018). Impact of industrial agglomeration on energy efficiency in China’s paper industry. Journal of Cleaner Production, 184, 1072–1080.
Zhou, P., Ang, B. W., & Poh, K. L. (2008). A survey of data envelopment analysis in energy and environmental studies. European Journal of Operational Research, 189(1), 1–18.
Zhou, Q., Li, T., & Gong, L. (2022). The effect of tax incentives on energy intensity : Evidence from China ’ s VAT reform. Energy Economics, 108, 105887.
Zhou, M., & Hu, T. (2021). Analysis of carbon emission status under the carbon neutral target in China for Earth’s atmospheric balance. In IOP conference series: earth and environmental science (Vol. 804, No. 4, p. 042082). IOP Publishing.
Zhu, B., Zhang, M., Zhou, Y., Wang, P., Sheng, J., He, K., et al. (2019). Exploring the effect of industrial structure adjustment on interprovincial green development efficiency in China: A novel integrated approach. Energy Policy, 134, 110946.
Acknowledgements
Jian Li and Huiting Niu acknowledge support from the National Natural Science Foundation of China (Grant No. 72173052).
Author information
Authors and Affiliations
Corresponding author
Ethics declarations
Conflict of interest
The authors declare no competing interests.
Additional information
Publisher's note
Springer Nature remains neutral with regard to jurisdictional claims in published maps and institutional affiliations.
Appendices
Appendix 1
The Theil index can be expressed as
where \(\frac{{H}_{in}}{{H}_{i}}\) represents the proportion of the industrial \(n\) in region \(i\) of GDP and \(\frac{{L}_{in}}{{L}_{i}}\) is the proportion of employees in the \(n\) industry in the region \(i\). When the value of the Theil index equals 0, it means that the industrial structure is in equilibrium; otherwise, it means that it deviates from the equilibrium state. The formula used in calculating capital input is as follows:
where \({K}_{t}\) represents the capital stock of period t, \({I}_{t}\) indicates the total fixed-asset investment of period t, and \(\delta\) is the depreciation rate. The formula used in calculating energy consumption is as follows:
where \({E}_{it}\) is the energy consumption of province i in period t, \({k}_{t}\) is the coefficient of period t, and \({DN}_{it}\) is the sum of grey values of all grids in province i in period t.
ArcGIS 10.0 was used to calculate the sum of \(DN\) value of each prefecture-level city in mainland China, and the simulated energy consumption of each city could be inversely calculated according to the final model results established by Eq. (12).
Appendix 2
The list of low-carbon pilot cities is compiled by the author from the official website of the National Development and Reform Commission.
The DID approach can address the endogeneity arising from the fact that the pilot cities are not randomly chosen. It does so by matching the pilot and non-pilot cities with relatively similar characteristics. However, several factors may have influenced the selection of the pilot cities. The factors include the socioeconomic conditions of the cities, the influence of the cities’ officials on decisions related to the LCCP program, and whether cities have energy-intensive industries with high carbon footprints. Differences in energy efficiency over time are expected to arise due to such factors. To address this issue, following Broner et al. (2012) and Hering and Poncet (2014), we use the ventilation coefficient as the instrumental variable in our regressions. This instrumental variable is suitable for the following reasons: first, the ventilation coefficient reflects the meteorological conditions that influence the speed of dispersion of pollutants in the air.Footnote 6 In general, given a fixed concentration of pollutants, cities with smaller ventilation coefficients tend to adopt more stringent environmental regulations and have a higher probability of being selected as low-carbon pilot cities. Cities, where air pollutants dissipate slowly, are more likely to be chosen as participants in the LCCP program—for given local carbon emissions, the carbon concentration in the air remains higher for longer (Broner et al., 2012). Second, as the ventilation coefficient is determined by large-scale weather systems, it can be considered an exogenous variable.
Table 8 in the Appendix presents the results estimated using the extended regression models (ERMs). We consider two estimation settings: the estimates in Model 1 do not include the control variables while the estimates in Model 3 do. The results presented in columns 2 and 4 (i.e., Stage 1 in Model 1 and Stage 2 in Model 2) reflect the impact of the ventilation coefficient on the LCCP program, indicating that the ventilation coefficient is significantly and positively correlated with the likelihood of being selected for the LCCP program. The results presented in columns 3 and 5 show that the LCCP program significantly increases energy efficiency, confirming the robustness of our estimates in Table 2.
Energy efficiency, defined as the ratio of service output to energy use (or input), can be calculated in two ways: total factor energy efficiency and single factor efficiency (Hong et al., 2022). Single-factor energy efficiency is usually measured by energy intensity. Total-factor efficiency considers the impact of multiple inputs and outputs. In this section, we first measure the total-factor energy efficiency of cities by changing the combination of undesirable outputs to test the robustness of the baseline results. We consider capital, labor, and energy consumption as inputs and the real GDP as the output and replace undesirable outputs with industrial sulfur dioxide and industrial fumes emissions. The higher the index value, the higher the energy efficiency. The results in Column 2 of Table 9 show that after replacing the proxy variable of undesirable outputs, the regression coefficient of the core dependent variable is positive and statistically significant.
We also consider single-factor energy efficiency as an alternative way to calculate energy efficiency. Currently, the calculation methods of energy efficiency mainly include single-factor indicator and total-factor indicator; single-farcor energy efficiency is also called energy intensity, which is generally expressed by the level of energy consumption per unit of gross domestic product (Z. Li et al., 2022). This method is also used in energy efficiency calculations by the International Energy Agency (2020) and the National Bureau of Statistics of China. The estimation results are shown in Column 3 of Table 9. We observe that implementing the LCCP program improved the energy efficiency of the pilot cities, thus confirming the robustness of our results.
We explained the reasons for not using the SFA model in this section. Specifically, the SFA is a typical representative of parametric methods in frontier analysis, which requires the determination of the specific form of the production frontier. Compared with non-parametric methods, its biggest advantage is that it takes into account the influence of stochastic factors on output. DEA is a linear programming approach to measure efficiency; it is a non-parametric method that does not need to know the specific form of the production frontier, but only needs to know the input–output data, DEA can easily handle the case where the decision unit is multiple outputs.
The complexity of the basic assumptions and model extensions of the SFA and DEA models are different. The basic assumptions of the SFA model are more complex and require consideration of the production function, the specific form of the distribution of the technical inefficiency term, which directly leads to the difficulty of further model extensions. Because of the complex form of the density function of the synthetic error term, the corresponding likelihood function is more complex, which brings a lot of computational difficulties to the parameter estimation, so it is difficult to further analyze the heteroskedasticity and other situations or do further model extensions. The main advantage of DEA is that it does not need to consider the specific form of the production frontier, only input–output data are required, and the model is easy to do other forms of extensions. The Super SBM-ML index differs from the traditional DEA model in that it uses the furthest distance to the frontier function type, which takes into account the influence of the slack variables on the efficiency value and provides a more accurate measure of the efficiency value. The super-efficiency model improves the traditional DEA that can only distinguish invalid units (when the efficiency value is less than 1) and can distinguish the advantages and disadvantages between efficient and effective values, reflecting the data results more comprehensively.
Rights and permissions
Springer Nature or its licensor (e.g. a society or other partner) holds exclusive rights to this article under a publishing agreement with the author(s) or other rightsholder(s); author self-archiving of the accepted manuscript version of this article is solely governed by the terms of such publishing agreement and applicable law.
About this article
Cite this article
Niu, H., Vatsa, P., Ma, W. et al. Environmental regulation and energy efficiency: empirical evidence from the low-carbon city pilot program in China. Energy Efficiency 16, 61 (2023). https://doi.org/10.1007/s12053-023-10140-6
Received:
Accepted:
Published:
DOI: https://doi.org/10.1007/s12053-023-10140-6