Abstract
The global physiological function of specifically expressed genes of prostate cancer in Chinese patients is unclear. This study aims to determine the genome-wide expression of genes related to prostate cancer in the Chinese population. Genes that were differentially expressed in prostate cancer were identified using DNA microarray technology. Expressions were validated by using real-time PCR. The identified genes were analyzed using the ingenuity pathway analysis (IPA) to investigate the gene ontology, functional pathway and network. A total of 1,444 genes (Fold time ≥ 1.5; P ≤ 0.05) were differentially expressed in prostate primary tumor tissue compared with benign tissue. IPA revealed a unique landscape where inductions of certain pathways were involved in Cell Cycle Regulation and proliferation. Network analysis not only confirmed that protein interactions lead to the deregulation of DNA Replication, Recombination and Repair, Cellular Compromise and Cell Cycle, Genetic Disorders and Connective Tissue Disorders, but it was also observed that many of the genes regulated by Myc contributed to the modulation of lipid Metabolism and Nucleic Acid Metabolism. Both pathway and network analysis exhibited some remarkable characteristics of prostate cancer for Chinese patients, which showed profound differences from that of other non-Chinese populations. These differences may provide new insights into the molecular cascade of prostate cancer that occurs in Chinese patients.
Similar content being viewed by others
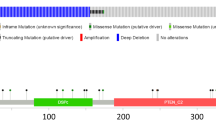
Avoid common mistakes on your manuscript.
Background
Prostate cancer is the most common cancer in men in western countries [1]. Diet, hormone levels, age, and chemical and carcinogen exposure may be potential risk factors for prostate cancer [2]. Historically, the rate of prostate cancer occurrence in the Chinese populations was lower than that of the western countries. Potential reasons are as follows: (1) genetic factors; (2) the differences in the levels of diagnosis; (3) the diversified clinical regulations. All these factors may be combining to cause the low rate of incidence of prostate cancer in the Chinese population.
Digital rectal examination and serum prostate–specific antigen (PSA) determination are the most commonly used screening methods worldwide. However, PSA has an excellent sensitivity with a low specificity; moreover, a more sensitive and more specific prostate cancer marker providing valuable information in the diagnosis and treatment of the disease is of particular importance. Previous studies show that quantitative real-time PCR and microarray analysis determinations have been used in the search to determine the molecular mechanism of the development of prostate cancer and to find new diagnostic and prognostic markers [3–6]. These reports have described specific expressed genes of prostate cancer in these patients from Japanese and Western which involve several signaling and metabolic pathways. The results obtained in pathway and network analyses in Japanese were different from that of Western [5, 6]. The difference is actually consistent with the previous study. Genetic polymorphisms that alter the activity of enzymes of biotransformation pathway are associated with cancer development and progression [7], so it should be kept in mind that there are genetic characteristics of prostate cancer patients from different populations. However, the global physiological function of the differentially expressed genes of prostate cancer in Chinese patients is unclear. In this study, a whole genome approach combined with bioinformatics assays was performed in order to elucidate the molecular function of these differently expressed genes of prostate cancer in Chinese patients.
Materials and methods
Patients and sample collection
Prostate tissues were obtained from the tissue bank at Guangzhou Medical College. All patients who participated in the study had given informed consent. The collection of the tissue specimens were in accordance with the protocol that was approved by the Institutional Ethical Board of Guangzhou Medical College. The tissues were procured immediately after surgery, cut into the size of ~1 cm2 × 0.5 cm pieces, fast-frozen in liquid nitrogen and stored at −80°C. 4 prostate primary tumor tissues and 4 paired benign tissues adjacent to tumor from October of 2009 to March of 2010 were studied. The selection of patients was based on digital rectal examinations and on serum PSA levels, and was confirmed by histological examination later. Gleason’s grades were assigned.
LCM and RNA preparation
Frozen sections of the tissue specimens were stained with hematoxylin and eosin for pathological analysis. If the tissue on the slice contains a tumor, then the rest of the uncut section was chosen to direct RNA extraction. If the tissue on slice is benign, then the rest uncut section was used for laser capture microdissection (LCM). Slides were cut 12 µm, then fixed in 75% ethanol, and stained with HE, and then air-dried. LCM was performed using a LCM System (Leica). The LCM cap was immediately placed in a microcentrifuge tube containing 400 μL Lysis/Binding Buffer (Ambion, USA), which was vortex mixed and stored upside down at −80°C until RNA isolation.
Total RNA was extracted and purified using RNA Isolation Kit (Ambion, USA). Possible genomic DNA contamination was removed with DNase treatment step, using the RNase-free DNase set (Qiagen, USA). The amount and quality of RNA preparations were evaluated on the Agilent 2100 Bioanalyzer with RNA6000 Nano Reagents and Supplies (Agilent, USA). Only samples with RIN (RNA Integrity Number) >6 were included for microarray analysis.
Microarray hybridization
Five hundred nanograms of RNA were reverse-transcribed to cDNA and carried out according to the manufacturer’s instructions, using the Agilent Fluorescent Direct Label kit to allow incorporation of nucleotides containing cyanine 3 (Cy3-dCTP) (Perkin-Elmer LifeSciences). Labeled cDNA was purified using QIAquick PCR purification columns (Qiagen, USA), and then concentrated by vacuum centrifugation. Labeled cDNA was resuspended in hybridization buffer and hybridized for 18 h at 68°C to a Whole Human Genome Microarray (4 × 44 K) (Agilent, USA), according to Agilent protocols.
Microarray data analysis
The microarray image information was converted into spot intensity values using Scanner Control Software Rev. 7.0 (Agilent, USA). The signal after background subtraction was exported directly into the GeneSpring GX11 software (Agilent, USA) for quantile normalization. The mean normalized signal from biological replicates was used for comparative expression analysis. Paired t test with Benjamini-Hochberg correction (P value ≤ 0.05) was used to identify differentially expressed mRNAs between tumor and pairs benign control. The fold changes of expression signals between tumor and control were calculated from the normalized values. Hierarchical clustering was performed with Pearson correlation using the differentially expressed mRNAs. Insignificant genes that changed by less than 1.5-fold and P value > 0.05 were removed from subsequent analysis. The microarray data used in this analysis have been submitted to NCBI GEO database under accession number GSE28204.
Hierarchical cluster analysis
After removing duplicate probe sets, a total of 1,042 genes (478 up genes and 564 down genes) showed estimated FC ≥ 2.0 in three patients. This list was used to perform a hierarchical cluster analysis and to construct a heat map using the Gene Cluster 3.0 and Tree View software (Stanford University, 2002).
Real-time quantitative reverse transcriptase PCR
Quantitative PCR technology was used to verify the differential expression of the 15 genes up and down respectively. cDNA was synthesized using random primers (hexamers) and Oligo 18dT and Superscript II Reverse Transcriptase (Invitrogen). Gene expression was determined using SYBR Green PCR mix (Toyoko) and 10 ng of template. Real-time PCR was performed on a MyiQ™2 Two-Color Real-Time PCR Detection System (Bio-Rad), using the following amplification conditions: 5 min, 95°C; followed by 40 cycles of 10 s 95°C, 20 s 60°C and 20 s 72°C. All assays were carried out in triplicate. CT values were determined using the IQ5 software (Bio-Rad). Gene expression in each sample was normalized with the housekeeping gene (β-actin) expression. The primers mostly were searched from PrimerBank (http://pga.mgh.harvard.edu/primerbank/index.html). Primers of target genes are listed in Table S2. Relative quantification of target gene expression was evaluated using the comparative cycle threshold (CT) method. P ≤ 0.05 was considered statistically significant. Correlation analysis was performed by comparing expression ratios from the microarray results with the ratios tested by the qRT-PCR analysis. The Pearson correction coefficient between the qRT-PCR and microarray was analyzed.
Functional interpretation of microarray data as well as pathway and network analysis
Ingenuity Pathways Analysis (IPA) (Ingenuity Systems http://www.ingenuity.com) is a web-based software application tool which is designed to organize biological information in a way that allows one to gain a high-level overview of the general biology that is associated with microarray data. Fischer’s exact test was used to calculate a P value determining the probability that each bio-function assigned to that dataset is due to chance alone. IPA Canonical Pathways Analysis tool was used to identify the signaling and metabolic pathways associated with the database. Genes from the dataset that met the fold change cut-off of 1.5 (P < 0.05) were considered for the analysis. The significance of the association between the dataset and the canonical pathway was measured in two ways, the ratio and the significance. The ingenuity network analysis was used to display an interactive graphical representation of the interrelationships between molecules.
Immunohistochemistry
Immunohistochemistry was performed with the Dako catalyzed signal amplification kit (DAKO, USA). Slides were steamed for 30 min in EDTA solution (Zymed, USA) for antigen retrieval. Slides were incubated with the rabbit monoclonal anti-MYC (Invitrogen, USA) and mouse monoclonal anti-TP63 (Invitrogen, USA) antibody overnight at 4°C. Biotinylated anti-rabbit IgG (Vector, USA) was used as secondary antibody. Staining was visualized using 3,30-Diaminobenzidine (DAB) (Sigma, USA), and slides were counterstained with hematoxylin.
Results
Differentially expressed genes of prostate cancer
In this study, we performed microarray hybridization with RNA from primary cancer tissue and adjacent benign tissues of the prostate gland. Biotin-labeled target cDNAs prepared from total RNA extracted were hybridized to the microarray chip containing 41,000 unique genes and transcripts. Clustering algorithm analysis for 717 genes (Fold change ≥ 2.0 and P ≤ 0.05) indicated that the data generated in different arrays from two group samples were tightly clustered (Figure S1). The gene expression profiles of prostate cancer were analyzed using the high-throughput gene chip. We sought to identify genes consistently expressed in prostate tumor tissues.
A total of 1,444 genes (Fold time ≥ 1.5; P ≤ 0.05) were differentially expressed. Of these 1,444 genes, 769 genes (53%) were upregulated and 675 (47%) were downregulated respectively in the primary tumor compared with the benign control. About 50% selected genes showed a 1.5–2-fold times change (Fig. 1, black and gray). There were 396 upregulated and 182 downregulated genes with a fold change range from 1.5 to 2 times. Figure 1 also showed that 308 upregulated genes and 334 downregulated genes with a fold change range from 2 times to 5 times, 46 upregulated genes and 78 downregulated genes with a fold change range from 5 times to 10 times, and 19 upregulated genes and 81 downregulated genes with a fold change >10 times. The data for all differentially expressed genes are shown in supplementary Table S1.
We further analyzed the top upregulated and downregulated genes. The list of top 5 upregulated or downregulated genes is shown in Table 1. These genes are considered the candidate genes to characterize prostate cancer.
Validation of differentially expressed genes by real-time PCR
To validate the microarray results, we analyzed top 15 upregulated and downregulated transcripts by quantitative real-time PCR. Results showed that most of genes exhibited a similar transcriptional profile to that of microarray data (Fig. 2; Table S2). The Pearson correction coefficient between the qRT-PCR and microarray data for the 15 top ranking differentially expressed was 0.83. Hence, the microarray provided a reliable comparison of gene expression between prostate primary tumors and benign tissues.
Real-time PCR analysis and microarray comparison. Transcript levels for genes of interest were determined in triplicates with primary tumor group and benign control group and normalized to β-actin transcript levels. Expression level of these interest genes derived from benign control group were set to one. Error bars indicate the standard deviation: mean ± SEM (n = 3). a Real-time PCR analysis and microarray comparison for upregulated gene. b Real-time PCR analysis and microarray comparison for downregulated gene
Relevant functions, pathway and biological networks in prostate cancer
In order to identify the gene function and associated biological process for all differentially expressed genes, the 769 upregulated genes and 675 downregulated genes were assigned to Ingenuity Pathways Analysis (IPA). In total, 1,068 upregulated and downregulated Gene IDS were eligible to biological function and pathway. In order to further define how individual upregulated and downregulated genes interact to have a coordinated role in specific pathways, we further identified potential networks.
As shown in Fig. 3, major functional gene categories that were specifically included were Gene Expression, Cellular Growth and Proliferation, Cellular Development, Cell Cycle, Cell Death, Cellular Movement, Cell-To-Cell Signaling and Interaction, Cell Signaling, Cellular Assembly and Organization, and Cellular Compromise. All of the function categories generated are shown in Table S3.
An over-representation of a specific biological process does not indicate whether the process in question is being stimulated or repressed overall. The signaling steps taking place during the development of prostate cancer remain largely unknown. Therefore, we used IPA software to further investigate over- or under-represented pathways regulated by these differentially expressed genes between primary tumors and benign tissues.
The most significant 10 canonical pathways within all of differentially expressed genes are shown in Fig. 4. All of the pathways generated are shown in Table S4. As shown in Fig. 4, most of the upregulated genes involved in these pathways: Role of CHK Proteins in Cell Cycle Checkpoint Control, Cell Cycle: G1/S Checkpoint Regulation, Cdc42 Signaling, ATM Signaling and Mismatch Repair in Eukaryotes. IPA analysis revealed that these canonical pathways were evidently activated, which indicates an increase in biological processes related to DNA Replication, Recombination, and Repair and Cell Cycle in prostate cancer. Three pathways: Cdc42 Signaling, 14-3-3-mediated Signaling and Role of Oct4 in Mammalian Embryonic Stem Cell Pluripotency were involved in Cellular Growth, Proliferation, and Development. P53 signaling involved in Cell Cycle, Cell Death, Cell-mediated Immune Response also showed significant change.
Canonical pathways identified by IPA associated with differentially expressed genes of prostate cancer. The 10 most significant canonical pathways across the entire dataset, and across multiple datasets, y-axis displays the significance. For the ratio, taller bars have more genes associated with the canonical pathway than shorter bars. Canonical pathway displays the number of upregulated (red), downregulated (green), and unchanged molecules (gray) in each canonical pathway
In order to further investigate the global expression occurring in prostate cancer and to define how individual upregulated and downregulated genes interact to have a coordinated role in specific pathways, we identified potential networks for these differentially expressed genes of prostate cancer.
We identified 25 highly significant networks of potentially interacting upregulated genes and downregulated gene (Table S5). The highest ranked, network1, is provided in Fig. 5 and Table S6. The network1 consists of 30 differentially expressed genes and the network is associated with DNA Replication, Recombination, and Repair, Cellular Compromise, and Cell Cycle. The second ranked, network 2, is provided in Fig. 6 and Table S7. The network2 consists of 30 DEGs genes and is associated with Dermatological Diseases and Conditions, Genetic Disorder, and Connective Tissue Disorders. The third ranked, network 3, is provided in Fig. 7 and Table S8. The network3 consists of 29 DEGs genes and is associated with Lipid Metabolism, Nucleic Acid Metabolism, and Small Molecule Biochemistry (Fig. 7 and Table S8).
Ingenuity pathway Analysis network 1 depicting relationships among differentially expressed genes of prostate cancer. Intensity of the red color indicates the degree of upregulation. Intensity of the green color indicates the degree of downregulation. Nodes are displayed using various shapes that represent the functional class of the gene product. Edges are displayed with various labels that describe the nature of relationship between the nodes: continuous line represents direct relationship; broken line represents indirect relationship; arrow represents acts on
Ingenuity pathway Analysis network 2 depicting relationships among differentially expressed genes between of prostate cancer. Intensity of the red color indicates the degree of upregulation. Intensity of the green color indicates the degree of downregulation. Edges are displayed with various labels that describe the nature of relationship between the nodes: continuous line represents direct relationship; broken line represents indirect relationship; arrow represents acts on. Nodes are displayed using various shapes that represent the functional class of the gene product same as shown in Fig. 5
Ingenuity pathway Analysis network 3 depicting relationships among differentially expressed genes of prostate cancer. Intensity of the red color indicates the degree of upregulation. Intensity of the green color indicates the degree of downregulation. Edges are displayed with various labels that describe the nature of relationship between the nodes: continuous line represents direct relationship; broken line represents indirect relationship; arrow represents acts on. Nodes are displayed using various shapes that represent the functional class of the gene product same as shown Fig. 5
In order to indentify the difference between the Chinese population and other populations, we further performed pathway comparison analysis between the American population published in 2010 (GSE21035) [8] and our microarray data.
Figure 8 lists the 10 representative pathways yielded by this comparison analysis. We found many pathways with different signaling responses between American population and Chinese populations. As shown in Fig. 8 and Table S9, Role of CHK proteins in Cell cycle checkpoint control, Cell cycle: G1/S check point Regulation, Aminocyl-tRNA biosynthesis, and DNA Double-strand Break Repair by Non-Homologous End Joining and Mismatch Repair in Eukaryotes are significant in our microarray data; however, these pathways linked to these genes in Tylor’s Microarray data had no profound change.
Discussion
In the current study, we focused on investigating the most affected genes, networks, and pathways for these differentially expressed genes between prostate primary tumors and benign tissues. In the results, the dramatic changes in thousands of gene expressions are characterized in prostate cancer of Chinese patients. IPA software revealed that 959 network eligible genes and 780 functional pathway eligible genes existed. A series of pathways associated with cell cycle, cell proliferation, cell apoptosis, and developmental disorder were appraised. Three top networks were identified and crossed with some identified signaling pathways.
Top upregulated and downregulated genes
The IPA data base showed that the 5 highest upregulated genes were associated with endocrine system disorders. Given that the endocrine system regulates growth processes, many endocrine system diseases often result in growth disorders. We suggested the top 5 upregulated genes may have important implications for prostate cancer progression. All of the top 5 downregulated genes were related to metabolism processes. Interestingly, we observed that these enzymes are also involved in other metabolic pathways including propionate metabolism, fatty acid metabolism, and β-alanine metabolism. Thus, downregulation of these important genes may play a key role in the disorder of metabolism activities of prostate cancer patients.
Of the 5 annotated upregulated genes, PPFIA2 were significantly upregulated with the largest fold change in prostate primary tumors relative to benign tissues. PPFIA2 is a member of the LAR protein-tyrosine phosphatase-interacting protein (liprin) family. Liprins interact with members of the LAR family of transmembrane protein tyrosine phosphatases, which are known to be important for axon guidance and mammary gland development [9]. The family protein is involved in cell–matrix adhesion. In agreement with our results, Fujinami et al. [10] also reported the gene was expressed in LNCaP cells as well as human prostate cancer tissues.
Two PTP family members, PTPRR and PTPRT, were significantly expressed. PTPs are known to be signaling molecules that regulate a variety of cellular processes including cell growth, differentiation, mitotic cycle, and oncogenic transformation [11]. Several of these members have been implicated in tumorigenesis [12, 13] with PTPRT as the most frequently mutated PTP gene in colorectal cancers [14]. Mutant human PTPRR gene (SNP substitution mutation, allelic variations: C/T (rs12581294)) is associated with Crohn’s disease (P value = 4.90262E−4) [15]; however, no relationship with prostate cancer has been reported, so further research is needed to establish the role both genes play in prostate cancer of Chinese patients.
Mutant human PRR16 gene (SNP substitution mutation, allelic variations: A/G (rs1037569)) is associated with prostate cancer (P value = 2.8E−5) [16]. In our study, the gene showed high amounts of upregulation, so further research is needed to establish whether the gene is a new molecular marker in Chinese patients or not.
CHRM2 (cholinergic receptor, muscarinic 2) is a member of the G-protein-coupled receptor family. The functional diversity of the protein family is defined by the binding of acetylcholine to these receptors and includes cellular responses such as adenylate cyclase inhibition, phosphoinositide degeneration, and potassium channel mediation. IPA database showed that the protein is found to be upregulated in multiple cancer cell lines and involved in Alzheimer’s disease, Parkinson’s disease, Non-small lung cancer, and so on. But the role of CHRM2 protein in prostate cancer development and progression is unknown. In our study, the gene showed high upregulation. Further studies combining proteomics are necessary to find out more details about gene.
Of the top 5 annotated downregulated genes, KRT23 (CK23) (histone deacetylase inducible) is a kind of Cytokeratin. The protein encoded by this gene is a member of the keratin family, the IPA database showed that the protein has been used as a biomarker in pancreatic cancer, breast cancer, colorectal cancer, gastric cancer, intestinal cancer, and non-small cell lung cancer. Recently, a detailed analysis of transcript profiling of normal colon mucosas and adenocarcinomas showed Keratin23 to be increased by 78% microsatellite-stable tumors [17]. They showed further Keratin23 impaired the proliferation of human colon cancer cells significantly. Also, KRT23 was found to be as blood marker of major depressive disorders which is a heritable disorder with high lifetime prevalence [18]. But little is known about its function in prostate cancer. Our microarray analysis revealed that this gene possibly is one of the key genes in Prostate cancer. Future studies should examine whether this biomarker will have the same function in prostate caner.
Human cytochrome P450 (CYP) family protein plays a key role in the metabolism of drugs and environmental chemicals [19]. In addition, the family members have been reported to be converting environmental procarcinogens to reactive intermediates with carcinogenic effects [20]. Recently, Fujimura T [21] demonstrated differential CYP3A4 expression in prostatic tissues, indicating that decreased CYP3A4 expression may contribute to the development of prostate cancer. Also, recently, Siemes C [22] reported that primarily the amount of CYP3A7 expression seemed to affect steroid hormone levels. Nevertheless, testosterone, a precursor of the prostate growth and differentiation stimulating dehydrotestosterone, was not influenced by CYP3A genotype. In line with this, they think that no significant associations were observed for CYP3A genotypes and Prostate cancer incidence. However, based on our microarray data, CYP3A4 and CYP3A7 showed high downregulation in prostate cancer, so we suggested further study polymorphisms in genes encoding for CYP3A4 and CYP3A7 enzymes in Chinese prostate cancer patients.
DPYS protein (Dihydropyrimidinase) catalyzes the conversion of 5,6-dihydrouracil to 3-ureidopropionate in pyrimidine metabolism. Dihydropyrimidinase is expressed at a high level in liver and kidney as a major 2.5-kb transcript and a minor 3.8-kb transcript. Defects in the DPYS gene are linked to dihydropyrimidinuria. In agreement with previous result, DPYS gene has been used as a biomarker for diagnosis of Prostate Cancer [23].
The DuoxA1 protein is a glycoprotein and a member of the NADPH oxidase family. DuoxA1 were involved in response to oxidative stress, cytokine-mediated signaling pathway, cuticle development; and hormone biosynthetic process. The NADPH oxidases DUOX1 and DUOX2, which are one of the main sources for reactive oxygen species production in the airway, are frequently silenced in human lung cancer [24]. Additionally, bisulfite sequencing and methylation-specific PCR revealed that CpG-rich promoter regions in both DUOX1 genes are hypermethylated [24]. Because our microarray showed also downregulated DUOX1, we suggested that an area on chromosome 15 that includes DUOX1 and their maturation factors may also become a frequent target for epigenetic silencing in Chinese patients with prostate cancer.
Pathway analysis
Interestingly, the top 10 pathway analysis (Fig. 4) revealed a unique landscape where induction of certain pathways involved in Cellular Stress and Injury Cell Cycle Regulation, Cellular Stress and Injury (e.g., Role of CHK Proteins in Cell Cycle Checkpoint Control, DNA Double-Strand Break Repair by Non-Homologous End Joining, Cell Cycle: G1/S Checkpoint Regulation, p53 Signaling, 14-3-3-mediated Signaling and ATM signaling and Cyclins and Cell Cycle Regulation). These data suggested that the signaling response for cell cycle is a major event in this study.
Several common genes involved in these above pathways (e.g. ATR(ATM), RAD50, CDC25A, MRE11 and GSK3B) showed upregulation. Role of CHK Proteins in Cell Cycle Checkpoint Control was the most significant signaling pathway modulated by the upregulated genes in prostate cancer (Figure S2). The pathways functionally related to cell cycle control and gene expression displayed activation in prostate primary tumors relative to benign tissues. Several studies have demonstrated a close association between deregulation of cell cycle progression and development of cancer and suggested that inhibition of unchecked cell cycle regulation in cancer cells could be a potential target for the management of cancer [25, 26]. Based on the report, further report showed that silibinin and silymarin modulate the ATM–Chk1/2–Cdc25–Cdc2–cyclin B1 pathway for G2–M arrest in PC3 cells. ATM knockdown in p53-defective PC3 prostate cancer cells accelerated their cell cycle transition, increased both E2F activity and proliferating cell nuclear antigen expression, and compromised cell cycle checkpoints, which are normally induced by DNA damage [27]. RAD50 forms a complex with MRE11 and NBS1. The protein complex binds to DNA and displays numerous enzymatic activities that are required for nonhomologous joining of DNA ends, which is important for DNA double-strand break repair, cell cycle checkpoint activation, telomere maintenance, and meiotic recombination. Knockout studies of the mouse homolog suggest this gene is essential for cell growth and viability. The heterotrimeric MRN complex, consisting of Mre11, Rad50 and Nbs1, which is required for the repair of double-strand breaks, plays a key role in telomere length maintenance. Kavitha et al. [28] reported that siRNA-mediated depletion of any of the subunits of MRN complex led to complete ablation of other subunits of the complex. The pathway analysis raises the possibility of developing anti-cancer drugs targeting MRN complex subunits.
In addition, one pathway related to cell growth and proliferation, Cdc42 signaling also showed significant change. The ability of CDC42 to influence diverse activities stems from its interactions with a large number of kinase and non-kinase effector proteins. Dysregulation of Cdc42 signaling was reported to contribute to prostate cancer previously [29]. Mahajan showed the activated Cdc42-associated kinase Ack1 promotes prostate cancer progression via androgen receptor tyrosine phosphorylation, but it is not easy to determine the pathway that really participated in prostate tumorigenesis in our study since many other pathway members were not detected.
Network analysis
Network1 indicated most of the upregulated genes are involved in DNA Replication, Recombination, and Repair, Cellular Compromise and Cell Cycle. Most of the genes showed mild upregulated, several genes showed protein–protein interactions, such as binding of human ATR protein and TOPBP1 protein occurs. Using the well-defined Escherichia coli lac repressor/operator system, Lindsey-Boltz LA [30] have found that directly tethering the ATR-activator TopBP1 to DNA is sufficient to induce ATR phosphorylation of Chk1 in an in vitro system as well as in vivo in mammalian cells. In K562 cells, SAHA increases the binding of human Akt protein(s) and human Hsp70 protein(s) that is increased by 17AAG [31]. Actually, the network analysis supports that cell cycle-related signaling was activated in prostate cancer. Overall, we recommend exploring these protein–protein interactions for monitoring diseases activity and response to therapy in patients with prostate cancer.
The network2 was identified around the JUN (JUNB) and TP63 respectively. Two genes, SDK1 and PKIB, were highly over-expressed around JUN. Upregulation of SDK-1 in podocytes is an important pathogenic factor in FSGS and that the mechanism involves disruption of the actin cytoskeleton possibly via alterations in MAGI-1 function [32]. Recently, PKIB were reported that over-expressed PKIB in prostate cancer promotes its aggressiveness by linking between PKA and Akt pathways [33]. In particular, PKIB were found to be highly upregulated around JUN in our study, and the result further confirms aggressive phenotype of prostate cancer. In controlling prostate carcinogenesis, JUNB plays an important role and may be a new target for cancer prevention and therapy [34]. PKIB should be a promising molecular target to JUN-mediated signaling for prostate cancer treatment.
TP63 are considered to have a significant role in tumor angiogenesis and cancer metastasis [35]. TP63 has been shown to play a critical role in prostate development [36]. TP63 protein expression is rare in prostate adenocarcinoma [37], which is consistent with the study; TP63 mRNA level showed significant downregulation in the prostate primary tumors related to benign tissues. Moreover, TP63 protein showed dramatic decrease in prostate primary tumors compared with benign tissues (Figure S3). Importantly, recent experimental evidence also suggests that TP63 is essential for normal stem cell function in the prostate as well as other epithelial organs [36], so future studies aimed at better defining the role of TP63 in the renewal of the adult prostate epithelium are likely to shed new light on the mechanisms involved in prostate carcinogenesis.
We observed three collagen family members: COL17A1, COL4A2 and COL22A1 showed downregulation in this network. Previous study suggested the gene expression profiles mediated by type I collagen may influence the proliferative capacity of prostate cancer cells in the bone microenvironment and facilitate development of prostate cancer bone metastases [38]. Further studies are required to establish the role this gene family plays in prostate cancer.
Myc protein is a multifunctional, nuclear protein that plays a role in cell cycle progression, apoptosis and cellular transformation. It functions as a transcription factor that regulates transcription of specific target genes. Mutations, overexpression, rearrangement and translocation of this gene have been associated with a variety of hematopoietic tumors. The third network was identified around the Myc (Fig. 7 and Table S8). Myc showed up as highly upregulated in this analysis. However, elevated MYC mRNA does not necessarily imply elevated MYC protein, in order to confirm the physiological relevance of MYC in vivo, we further investigated the distribution of the MYC protein in prostate tumor tissues and benign tissues. As shown in Figure S4, significant differences were found between prostate tumor tissues and benign tissues. MYC protein showed dramatic increase in prostate tumor tissues compared with benign tissues. Gurel et al. [39] provide evidence that upregulation of nuclear MYC protein expression is a highly prevalent and early change in prostate cancer and suggest that increased nuclear MYC may be a critical oncogenic event driving human prostate cancer initiation and progression. Actually, the network analysis supports the central role that Myc regulates transcription of specific target genes, for example, most of Myc-induced gene, such as FXC1, VARS, PREP, CLPB, EPCM and SIK were upregulated; thus, these genes could be candidate genes to understand the Myc function involved in metabolism activity in prostate cancer. Interestingly, in the network, we also observed CLDN7 involvment in Myc network. CLDN7 were highly upregulated in microarray data. CLDN7 encodes a member of the Claudin family. Claudins are integral membrane proteins and components of tight junction strands. Differential expression of this gene has been observed in different types of malignancies, including prostate cancer [40, 41]. Further research is needed to validate our analysis and how Myc regulates the expression of CLDN7 in prostate cancer.
Conclusions
In this current study, we are the first to show a bioinformatics strategy to investigate global pathway and network of these differentially expressed genes between prostate primary tumors and benign tissues in Chinese prostate cancer patients. We analyzed over one thousand altered genes regarding functions and communications using the IPA tool and detected new their relationships.
Moreover, we observed a general activated process of signaling pathways, specifically shown in the cell cycle-related biological process. In contrast, the significance values of the pathways were low in American populations [8]. Further compared with previous gene network and pathway analysis for Hungary prostate cancer patients [6] and Japanese prostate cancer patients [5], our analysis obtained in Chinese patients showed profound difference in both pathway and network; these difference may provide some clues to reveal specific causes for Chinese prostate cancer, which suggest that the deregulation of cell cycle progression maybe a “key” to differentiate the epidemiological differences between Chinese and non-Chinese populations. We speculate that the relevant target genes presumably increase the opportunities of tumor growth in prostate cancer and can further lead to prostate cancer diseases in the Chinese patients.
Network analysis not only help us confirm protein interaction leads to the deregulation of DNA Replication, Recombination, and Repair, Cellular Compromise and Cell Cycle, Genetic Disorder and Connective Tissue Disorders, but it was also observed that many of the genes regulated by Myc contributed to the modulation of lipid Metabolism and Nucleic Acid Metabolism.
Overall, our data not only provide new candidate genes for understanding the biologic properties of prostate cancer in Chinese population, but also provide useful pathway and network maps for further understanding of the pathogenesis and growth of prostate cancer. It will help us to develop a new protocol in prostate cancer for bio-maker, risk assessment, and new therapeutic targets.
References
Jemal A, Siegel R, Xu J, Ward E. Cancer statistics, 2010. CA Cancer J Clin. 2010;60:277–300.
Mettlin C. Recent developments in the epidemiology of prostate cancer. Eur J Cancer. 1997;33:340–7.
Hughes C, Murphy A, Martin C, Sheils O, O’Leary J. Molecular pathology of prostate cancer. J Clin Pathol. 2005;58:673–84.
Quinn DI, Henshall SM, Sutherland LR. Molecular markers of prostate cancer outcome. Eur J Cancer. 2005;41:858–87.
Endo T, Uzawa K, Suzuki H, Tanzawa H, Ichikawa T. Characteristic gene expression profiles of benign prostatic hypertrophy and prostate cancer. Int J Oncol. 2009;35:499–509.
Savli H, Szendröi A, Romics I, Nagy B. Gene network and canonical pathway analysis in prostate cancer: a microarray study. Exp Mol Med. 2008;40:176–85.
Alavanja MC, Samanic C, Dosemeci M, Lubin J, Tarone R, Lynch CF, Knott C, Thomas K, Hoppin JA, Barker J, Coble J, Sandler DP, Blair A. Use of agricultural pesticides and prostate cancer risk in the Agricultural Health Study cohort. Am J Epidemiol. 2003;157(9):800–14.
Taylor BS, Schultz N, Hieronymus H, Gopalan A, Xiao Y, Carver BS, Arora VK, Kaushik P, Cerami E, Reva B, Antipin Y, Mitsiades N, Landers T, Dolgalev I, Major JE, Wilson M, Socci ND, Lash AE, Heguy A, Eastham JA, Scher HI, Reuter VE, Scardino PT, Sander C, Sawyers CL, Gerald WL. Integrative genomic profiling of human prostate cancer. Cancer Cell. 2010;18:11–22.
Florio T. Somatostatin/somatostatin receptor signalling: phosphotyrosine phosphatases. Mol Cell Endocrinol. 2008;286:40–8.
Fujinami K, Uemura H, Ishiguro H, Kubota Y. Liprin-alpha2 gene, protein tyrosine phosphatase LAR interacting protein related gene, is downregulated by androgens in the human prostate cancer cell line LNCaP. Int J Mol Med. 2002;10:173–6.
Blume-Jensen P, Hunter T. Oncogenic kinase signalling. Nature. 2001;411:355–65.
Ostman A, Hellberg C, Bohmer FD. Protein-tyrosine phosphatases and cancer. Nat Rev Cancer. 2006;6:307–20.
Scott A, Wang Z. Tumour suppressor function of protein tyrosine phosphatase receptor-T. Biosci Rep. 2011;31:303–7.
Wang Z, Shen D, Parsons DW, Bardelli A, Sager J, Szabo S, Ptak J, Silliman N, Peters BA, van der Heijden MS, Parmigiani G, Yan H, Wang TL, Riggins G, Powell SM, Willson JK, Markowitz S, Kinzler KW, Vogelstein B, Velculescu VE. Mutational analysis of the tyrosine phosphatome in colorectal cancers. Science. 2004;304:1164–6.
Wellcome Trust Case Control Consortium. Genome-wide association study of 14, 000 cases of seven common diseases and 3, 000 shared controls. Nature. 2007;447:661–78.
Murabito JM, Rosenberg CL, Finger D, Kreger BE, Levy D, Splansky GL, Antman K, Hwang SJ. A genome-wide association study of breast and prostate cancer in the NHLBI’s Framingham Heart Study. BMC Med Genet. 2007;8(Suppl 1):S6.
Birkenkamp-Demtroder K, Mansilla F, Sørensen FB, Kruhøffer M, Cabezón T, Christensen LL, Aaltonen LA, Verspaget HW, Ørntoft TF. Phosphoprotein Keratin 23 accumulates in MSS but not MSI colon cancers in vivo and impacts viability and proliferation in vitro. Mol Oncol. 2007;1:181–95.
Spijker S, Van Zanten JS, De Jong S, Penninx BW, van Dyck R, Zitman FG, Smit JH, Ylstra B, Smit AB, Hoogendijk WJ. Stimulated gene expression profiles as a blood marker of major depressive disorder. Biol Psychiatry. 2010;68(2):179–86. Epub 2010 May 14.
Agundez JA. Cytochrome P450 gene polymorphism and cancer. Curr Drug Metab. 2004;5:211–24.
Bartsch H, Nair U, Risch A, Rojas M, Wikman H, Alexandrov K. Genetic polymorphism of CYP genes, alone or in combination, as a risk modifier of tobacco-related cancers. Cancer Epidemiol Biomarkers Prev. 2000;9(1):3–28.
Fujimura T, Takahashi S, Urano T, Kumagai J, Murata T, Takayama K, Ogushi T, Horie-Inoue K, Ouchi Y, Kitamura T, Muramatsu M, Homma Y, Inoue S. Expression of cytochrome P450 3A4 and its clinical significance in human prostate cancer. Urology. 2009;74:391–7.
Siemes C, Visser LE, de Jong FH, Coebergh JW, Uitterlinden AG, Hofman A, Stricker BH, van Schaik RH. Cytochrome P450 3A gene variation, steroid hormone serum levels and prostate cancer–The Rotterdam Study. Steroids. 2010;75:1024–32.
Chung W, Kwabi-Addo B, Ittmann M, Jelinek J, Shen L, Yu Y, Issa JP. Identification of novel tumor markers in prostate, colon and breast cancer by unbiased methylation profiling. PLoS One. 2008;3:e2079.
Luxen S, Belinsky SA, Knaus UG. Silencing of DUOX NADPH oxidases by promoter hypermethylation in lung cancer. Cancer Res. 2008;68:1037–45.
McDonald ER III, El-Deiry WS. Cell cycle control as a basis for cancer drug development (Review). Int J Oncol. 2000;16:871–86.
Owa T, Yoshino H, Yoshimatsu K, Nagasu T. Cell cycle regulation in the G1 phase: a promising target for the development of new chemotherapeutic anticancer agents. Curr Med Chem. 2001;8:1487–503.
Mukhopadhyay UK, Senderowicz AM, Ferbeyre G. RNA silencing of checkpoint regulators sensitizes p53-defective prostate cancer cells to chemotherapy while sparing normal cells. Cancer Res. 2005;65:2872–81.
Kavitha CV, Choudhary B, Raghavan SC, Muniyappa K. Differential regulation of MRN (Mre11-Rad50-Nbs1) complex subunits and telomerase activity in cancer cells. Biochem Biophys Res Commun. 2010;399:575–80.
Mahajan NP, Liu Y, Majumder S, Warren MR, Parker CE, Mohler JL, Earp HS, Whang YE. Activated Cdc42-associated kinase Ack1 promotes prostate cancer progression via androgen receptor tyrosine phosphorylation. Proc Natl Acad Sci USA. 2007;104:8438–43.
Lindsey-Boltz LA, Sancar A. Tethering DNA damage checkpoint mediator proteins TopBP1 and Claspin to DNA activates ATR phosphorylation of Chk1. J Biol Chem. 2011;286:19229–36.
Gao T, Newton AC. The turn motif is a phosphorylation switch that regulates the binding of Hsp70 to protein kinase C. J Biol Chem. 2002;277:31585–92.
Kaufman L, Potla U, Coleman S, Dikiy S, Hata Y, Kurihara H, He JC, D’Agati VD, Klotman PE. Up-regulation of the homophilic adhesion molecule sidekick-1 in podocytes contributes to glomerulosclerosis. J Biol Chem. 2010;285:25677–85.
Chung S, Furihata M, Tamura K, Uemura M, Daigo Y, Nasu Y, Miki T, Shuin T, Fujioka T, Nakamura Y, Nakagawa H. Overexpressing PKIB in prostate cancer promotes its aggressiveness by linking between PKA and Akt pathways. Oncogene. 2009;28:2849–59.
Konishi N, Shimada K, Nakamura M, Ishida E, Ota I, Tanaka N, Fujimoto K. Function of JunB in transient amplifying cell senescence and progression of human prostate cancer. Clin Cancer Res. 2008;14:4408–16.
Tomkova K, Tomka M, Zajac V. Contribution of p53, p63, and p73 to the developmental diseases and cancer. Neoplasma. 2008;55:177–81.
Grisanzio C, Signoretti S. p63 in prostate biology and pathology. J Cell Biochem. 2008;103:1354–68.
Parsons JK, Gage WR, Nelson WG, De Marzo AM. p63 protein expression is rare in prostate adenocarcinoma: implications for cancer diagnosis and carcinogenesis. Urology. 2001;58:619–24.
Kiefer J, Alexander A, Farach-Carson MC. Type I collagen-mediated changes in gene expression and function of prostate cancer cells. Cancer Treat Res. 2004;118:101–24.
Gurel B, Iwata T, Koh CM, Jenkins RB, Lan F, Van Dang C, Hicks JL, Morgan J, Cornish TC, Sutcliffe S, Isaacs WB, Luo J, De Marzo AM. Nuclear MYC protein overexpression is an early alteration in human prostate carcinogenesis. Mod Pathol. 2008;21:1156–67. Epub 2008 Jun 20.
Sheehan GM, Kallakury BV, Sheehan CE, Fisher HA, Kaufman RP Jr, Ross JS. Loss of claudins-1 and -7 and expression of claudins-3 and -4 correlate with prognostic variables in prostatic adenocarcinomas. Hum Pathol. 2007;38:564–9.
Wang Q, Zheng JY, Kreth J, Yan X, Kamata M, Campbell RA, Xie Y, Chiu R, Berenson JR, Shi W, Chen IS, Pang S. Regulation of prostate-specific antigen expression by the junctional adhesion molecule A. Urology. 2009;73:1119–25.
Acknowledgments
This work was supported by grants from the Natural Science Foundation of Guangdong Province (04003650), National Natural Science Foundation of China (30872960), Key projects of Bureau of Health in Guangzhou Municipality (201102A212015) and projects of Guangdong Key Laboratory of Urology (2010A060801016).
Conflict of interest
All authors disclose any association that poses a conflict of interest in connection with the manuscript.
Author information
Authors and Affiliations
Corresponding authors
Additional information
Jia-hong Chen and Hui-chan He contributed equally to this work.
Microarray data are available on GEO (GSE28204).
Electronic supplementary material
Below is the link to the electronic supplementary material.
Rights and permissions
About this article
Cite this article
Chen, Jh., He, Hc., Jiang, Fn. et al. Analysis of the specific pathways and networks of prostate cancer for gene expression profiles in the Chinese population. Med Oncol 29, 1972–1984 (2012). https://doi.org/10.1007/s12032-011-0088-5
Received:
Accepted:
Published:
Issue Date:
DOI: https://doi.org/10.1007/s12032-011-0088-5