Abstract
Disposal of dead pigs by pig farmers may have a direct impact on pork safety, public health, and the ecological environment in China. Drawing on the existing literature, this study analyzed and summarized the main factors that could affect the disposal of dead pigs by pig farmers by conducting a survey of 654 pig farmers in Funing County, Jiangsu Province, China. The purpose of this analysis was to investigate the disposal of dead pigs in China and provide useful regulatory strategies for the government. The interrelationships among dimensions and factors that affect the disposal of dead pigs by farmers were analyzed, and critical factors were identified by a hybrid multi-criteria decision-making method, which is a combination of decision-making trial and evaluation laboratory (DEMATEL) and analytic network process (ANP). Our results demonstrated that production characteristics were the most important dimensions and that costs and profits, scale of farming, pattern of farming, knowledge of relevant laws and regulations, and knowledge of pig disease and prevention were the five most critical factors affecting the disposal of dead pigs by farmers in China at this stage. The significance of this study lies in further discussing some management policies for the Chinese government regarding strengthen regulation of disposing dead pigs.
Similar content being viewed by others
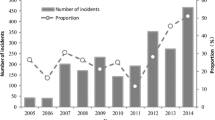
Explore related subjects
Discover the latest articles, news and stories from top researchers in related subjects.Avoid common mistakes on your manuscript.
Introduction
Food of animal origin has been recognized as a main carrier of foodborne disease, which is a significant threat to human health (Jacob et al. 2010; Loretz et al. 2011). Pork is the most popular food of animal origin in China. However, pigs are a major reservoir for Yersinia enterocolitica, a leading foodborne enteric pathogen (Tan et al. 2014). Therefore, ensuring pork safety is of significance to the protection of public health in China. Unfortunately, there have been increasingly frequent reports of negative behaviors in the disposal of dead pigs in recent years.Footnote 1 Of these, the most shocking incident is the dumping of dead pigs into Huangpu River in metropolitan Shanghai in early March 2013. As of March 20, 2013, a total of 10,395 floating dead pigs had been collected from Huangpu River. Since dead pigs contain vast amounts of pathogenic microorganisms, dumping of dead pigs into rivers and lakes can lead to deteriorated water quality and environment pollution (Shen et al. 2013). These issues not only caused concern over water quality among Shanghai citizens but also sparked international scathing derision regarding the food safety situation in China (Caixin 2013).
Dead pigs are an inevitable product of pig farming. The annual growth rate of natural pig deaths is 2.09 % in China under normal circumstances and without the interference of various diseases. The number of natural pig deaths can thus be calculated to be 21,580,000 in 2012.Footnote 2 Despite strengthened regulation by the government, dead pigs were found floating in Xinyang of Henan Province, Yichang of Hubei Province, and other places following the incident of dumping of dead pigs into the Huangpu River in March 2013. Additionally, illegal sale of 20,000 t of pork from ill and dead pigs was reported by the Chinese media in January 2014. Dead pigs not only carry harmful microorganisms but also contain a high concentration of veterinary drug residues or metabolites, as well as pathogens and sources of contagion (Ni 2012). Numerous studies confirm that harmful microorganisms and veterinary drug residues or metabolites in meat and other foods of animal origin may threaten the health of consumers, and in particular, may undermine the human gastrointestinal system, causing infections (Reig and Toldrá 2008; Marshall and Levy 2011). Moreover, in 2008, according to data reported by the Ministry of Health of China, gastroenteritis is the second most common chronic disease in Chinese residents after hypertension. Although undetected consumption of pork from dead pigs can generate problems of gastroenteritis, which is difficult to diagnose because of a lack of relevant information on pork from dead pig ingestion, negative behaviors in the disposal of dead pigs by pig farmers have highlighted some issues in China regarding public health, food safety, and ecological environmental protection (Liu 2013). Therefore, it is a major practical and unavoidable task of China’s food safety management to investigate and identify critical factors that affect the disposal of dead pigs by farmers.
The disposal of dead pigs by farmers is influenced by many dimensions and factors, and if these can be found and the extent of their impact and interrelationships among the dimensions and factors can be determined, a list of management strategies can be developed by government to curb negative behaviors in disposal of dead pigs by farmers. In fact, the factors are usually not independent, and the dimensions may also be mutually influential. This study applied a method which is a combination of both the decision-making trial and evaluation laboratory (DEMATEL) and the analytic network process (ANP) (called DEMATEL-based ANP, DANP) to explore the interrelationships among the dimensions and factors that affect the disposal of dead pigs by farmers. Huang et al. (2014) demonstrated that DANP is an effective method that can quantify complex qualitative issues and define their interdependencies and importance. In the past, few studies have used the proposed method to identify the critical factors that affect the disposal of dead pigs by farmers and then examine or provide the management policy decision-making. However, compared with the traditional tools that use binary logistic regression, logit, and probit models to investigate factors affecting production behaviors of pig farmers, DANP can effectively overcome the problem of interest demands of survey samples that inevitably exist in these traditional measurement tools. Additionally, it is not reasonable to use DANP to identify critical factors affecting the disposal of dead pigs by simply drawing on existing research findings, since factors affecting the disposal of dead pigs by farmers vary among different countries and different regions within a country. Therefore, in order to provide more objective and practical investigation, this study set main factors that may affect the disposal of dead pigs by farmers based on a field survey of 654 pig farmers in Funing County, Jiangsu Province, China. In addition to setting these factors, our analysis assessed existing research findings and analyzed the interrelationships among the dimensions and factors and identified the critical factors using DANP. The purpose and significance of this study is to provide useful policy recommendations for identifying a realistic way to prevent negative behaviors in disposal of dead pigs by pig farmers based on the interrelationships among the dimensions and factors and the identification of critical factors.
Materials and methods
Case survey
Demographics and data related to disposal of dead pigs were collected from pig farmers in Funing County, Jiangsu Province, China. Funing County was selected because it is a famous pig farming county, known as “the hometown of piglets” in China and has held the title of “No. 1 Pig Farming County” in Jiangsu Province for 15 consecutive years. The county produced 1,576,600 and 1,661,600 pigs ready to be slaughtered in 2011 and 2012, respectively. Pig farming is an important source of family income and a main career for many farmers in this location.
The survey was conducted in Funing County, Jiangsu Province from January to March 2014. A preliminary survey was first performed in pig farmers of different scales in Longwo Village, Shuanglian Village, Xinlian Village, and Wangji Village of Luoqiang Town and Sanzao Town in Funing County. A final questionnaire was developed according to the results of the preliminary survey. A village with a moderate level of farmer household income was selected from all 13 towns of Funing County. A group of villagers, including 40–60 households, was randomly selected from each committee by the village committee. In this way, 690 pig farmer households were surveyed, with a valid sample of 654 households (with a valid response rate of 94.78 %). Since face-to-face interview can effectively avoid respondents’ possible misunderstanding of survey questions and generate a high response rate (Boccaletti and Nardella 2000), the actual survey was performed by trained investigators during face-to-face interview.
Analysis of case survey
Demographics and disposal of dead pigs
As shown in Table 1, most respondents were older male farmers with a low level of education, which is consistent with the characteristics of pig farmers in China. Survey data showed that a slightly higher proportion of male farmers than female farmers might have negative behaviors in disposal of dead pigs and that older farmers with a low level of education were more likely to have negative behaviors in the disposal of dead pigs. These findings are in accordance with those of previous studies, which suggested that gender, age, and education level of farmers were important factors affecting their behavioral decisions (Deressa et al. 2009; Cheng et al. 2014). In terms of household characteristics, most households had a household size of 5 or more, accounting for 51.4 % of all households. In order to investigate the degree of specialization of farmers, the proportion of pig farming income in total household income was also assessed; pig farming income accounted for 30 % or less of total household income according to 66.1 % of respondents. The correlation between household characteristics and negative behaviors in disposal of dead pigs is shown in Table 1. Pig farmers with a household size of less than 3 and a pig farming income accounting for less than 50 % of the total household income had a higher propensity toward negative behaviors in the disposal of dead pigs. This is consistent with the conclusion of Abdulai et al. (2008) and Cui et al. (2011) that household size and income structure had an impact on producer’s behavioral decisions. Therefore, it was assumed that the demographics (individual and household characteristics) of pig farmers affected their behavior with respect to the disposal of dead pigs.
Production characteristics and disposal of dead pigs
As shown in Table 2, 67.0 % the 654 farmers surveyed had more than 10 years of pig farming experience and 73.9 % of the respondents were backyard farmers, which meant a farming scaleFootnote 3 of less than 50 pigs. These findings coincide with the pig production pattern of Funing County. Moreover, a higher proportion of farmers with more than 10 years of pig farming experience and a farming scale of less than 50 pigs had negative behaviors in disposal of dead pigs. This finding is consistent with those of previous studies, which suggested that years of farming experience, scale of farming, and pattern of farming were associated with safe producer behavior (Sun et al. 2012; Yu et al. 2012; Ithika et al. 2013). Therefore, it was assumed that years of farming experience, scale of farming, and pattern of farming affected the disposal of dead pigs by farmers.
Knowledge of farmers and disposal of dead pigs
As shown in Table 3, 58.3 and 20.6 % of respondents, respectively, had very little knowledge and little knowledge of relevant laws and regulations,Footnote 4 and 62.8 % had very little knowledge of pig disease and prevention. A description of the farmers’ relevant knowledge and the data related to the negative behaviors in disposal of dead pigs are shown in Table 3. A higher proportion of pig farmers with little knowledge of relevant laws and regulations and pig disease and prevention had negative behaviors in disposal of dead pigs. This is consistent with the conclusions of previous studies that knowledge was an important factor that affected producer behavior (Vignola et al. 2010; Launio et al. 2014). Therefore, it was assumed that knowledge of relevant laws and regulations and pig disease and prevention affected the disposal of dead pigs by farmers.
Behaviors and motivations in disposal of dead pigs
As shown in Table 4, 24.3 % of respondents did not carry out harmless disposal of dead pigs, with cost being the major reason. This is consistent with the argument of Edwards-Jones (2006) and Chen et al. (2011) that farmers are rational people, with a behavioral objective to maximize profit. Therefore, it was assumed that costs and profits affected the disposal of dead pigs by farmers.
Setting of factors that affect disposal of dead pigs by farmers
According to the results shown in Tables 1–4, possible factors that affected the disposal of dead pigs by farmers were gender, age, education level, family size, income structure, years of farming experience, scale of farming, pattern of farming, knowledge of relevant laws and regulations, knowledge of pig disease and prevention, and costs and profits. The setting of these factors was highly consistent with the research findings of the existing literature (Table 5).
The disposal of dead pigs by farmers is not only affected by the internal factors of farmers but is also influenced by the external environment. Since the new century, the Chinese government has introduced a series of policies and regulations regarding scientific disposal of dead pigs. For example, a document issued by the Chinese Ministry of Agriculture in 2012 clarified a subsidy policy on the harmless disposal of dead pigs. The Animal Epidemic Prevention Law of the People’s Republic of China (2013 Amendment) promulgated by the State Council of China in 2013 clearly defines that carcasses of animals that died of disease or unknown cause must not be thrown away and provides clear penalties. In a word, the relevant policy and environment of government have an important role in affecting the disposal of dead pigs by farmers. It has been demonstrated that variables related to policy environment significantly affected the behavior of farmers (Launio et al. 2014).
The government can encourage farmers to choose good behavior, by means of subsidies (Danso et al. 2006; Läpple 2010). Liu (2012) noted that a sound pig insurance policy could effectively control the entry of dead pigs into the market. Chinese laws impose a small punishment on negative behaviors with respect to the disposal of dead pigs, resulting in persistence of such behaviors (Lian 2013). Wu et al. (2013) showed that government supervision was one of the critical factors affecting negative behaviors of Chinese producers. However, Mol (2014) believes that it is difficult to solve food safety problems in China by excessive reliance on laws and regulations and government regulation; moreover, it is difficult to discover negative behaviors in the disposal of dead pigs, and participation of the general public should thus be encouraged. A particular example is that of Zhejiang Province, China, where a reward system was implemented for reporting negative behaviors in the disposal of dead pigs; remarkable results have been achieved through this system. Therefore, inclusion of incentives in government regulation may allow more effective management of food safety problems (Jayasinghe-Mudalige and Henson 2007). In addition, behavioral decisions of pig farmers are subject of the influence of surrounding groups (Mzoughi 2011). Peer behavior has an influence on disposal of dead pigs by farmers.
Thus, there are inevitable limitations in the conclusions derived from the analysis of factors affecting disposal of dead pigs by farmers from the perspective of farmers only. Both farmers and government must be fully considered. With this in mind, based on our survey of pig farmers in Funing County, as well as existing research results, 17 factors that may affect disposal of dead pigs by farmers, which fall into four dimensions, are summarized in Table 5.
Research methods
In order to quantify the relationship among the dimensions and factors affecting disposal of dead pigs by farmers and to obtain the influential weights of each factor and dimension, the DANP method was adopted in this study. An expert group comprising nine committee members from China Food Industry Association, College of Food Science of China Agricultural University, College of Economics and Management of Shandong Agricultural University, College of Food of Jiangnan University, Food Safety Research Base of Jiangsu Province, and Institute of Agriculture Science of Jiangsu Province was invited to propose the degree of direct influence that factor i exerts on each factor j. Those members were familiar with the standard practices and professional management of dead pigs. Before the assessment, experts could discuss with each other and express their opinions. Assessment given by experts is always considered to be a linguistic variable rather than integer number; thus, each expert was asked to use the assumed scales in Table 6. Finally, the initial relationships among the 17 factors were obtained. According to Huang et al. (2014), the average deviation of the scoring results of the expert group was calculated using \( \frac{1}{n^2}{\displaystyle \sum_{i=1}^n{\displaystyle \sum_{j=1}^n\frac{\left|{a}_{ij}^p-{a}_{ij}^{p-1}\right|}{a_{ij}^p}}}\times 100\% \), where p is the number of experts, a p ij is the average impact of factor i on factor i, and n is the number of influencing factors set in this study. For the nine experts, the average deviation was only 3.86 %, which meant that the assessment by the expert group was valid.
The path to determine the interrelationships among the dimensions and factors and calculate their influential weights using the DANP method is shown below (Hsu et al. 2012; Lu et al. 2013; Huang et al. 2014).
-
Step 1
Acquire the initial direct-relation matrix A = [a ij ] n × n , i, j = 1, 2,......, n, from the assessment by the expert group.
-
Step 2
Convert the initial direct-relation matrix to the standardized impact matrix D
$$ D=z\times A $$(1)$$ z= \min \left\{1/{ \max}_i{\displaystyle \sum_{j=1}^n{a}_{ij}},1/{ \max}_j{\displaystyle \sum_{i=1}^n{a}_{ij}}\right\}\kern1em where\kern1em i,j\in \left\{1,2,......,n\right\} $$(2) -
Step 3
Calculate the total influence matrix T = [t ij ] n × n , i, j = 1, 2, ⋯ ⋯, n. The element t ij shows the direct and indirect impacts of factor i on factor j.
$$ T=D+{D}^2+{D}^3+\dots +{D}^h=D\left(I-{D}^h\right){\left(I-D\right)}^{-1} $$where I is denoted as the identity matrix. Then,
$$ T=D{\left(I-D\right)}^{-1} when\kern0.5em h\to \infty, {D}^h={\left[0\right]}_{n\times n} $$(3)where D = [d ij ] n × n , 0 ≤ d ij < 1, 0 ≤ ∑ i d ij ≤ 1, 0 ≤ ∑ j d ij ≤ 1, and at least one (but not all) of the columns or rows of the summation is equal to 1 in \( {\displaystyle \sum_{j=1}^n{d}_{ij}} \) and \( {\displaystyle \sum_{i=1}^n{d}_{ij}} \) and thus can guarantee that \( \underset{h\to \infty }{ \lim }{D}^h={\left[0\right]}_{n\times n} \)
-
Step 4
Denote the row and column sums of the matrix T.
$$ {r}_i={\displaystyle \sum_{j=1}^n{t}_{ij}} $$(4)$$ {c}_j={\displaystyle \sum_{i=1}^n{t}_{ij}} $$(5)Here, r i shows the sum of the direct and indirect influences that factor i gives to all other factors. Similarly, c j shows the sum of direct and indirect influences that factor j receives from the other factor. In addition, where i = j, r i + c i provides an index of the strength of influences given and received, that is, r i + c i shows the degree of total influences factor i has in this system. Therefore, if (r i − c i ) is positive, then factor i has a net influence on the other factors, and if (r i − c i ) is negative, then factor i is, on the whole, being influenced by the other factors.
-
Step 5
Divide the total influence matrix T into T D based on the dimensions and T C based on the factors.
$$ {T}_C=\begin{array}{c}\hfill {{}_{D_1}}_{\begin{array}{l}\vdots \\ {}{c}_{1{m}_1}\end{array}}^{c_{11}}\hfill \\ {}\hfill {}_{\vdots}\hfill \\ {}\hfill {{}_{D_i}}_{\begin{array}{l}\vdots \\ {}{c}_{i{m}_i}\end{array}}^{c{}_{i1}}\hfill \\ {}\hfill {}_{\vdots}\hfill \\ {}\hfill {{}_{D_n}}_{\begin{array}{l}\vdots \\ {}{c}_{n{m}_n}\end{array}}^{c_{n1}}\hfill \end{array}\overset{\begin{array}{ccccc}\hfill \underset{C_{11\cdots {C}_{1{m}_1}}}{D_1}\hfill & \hfill \cdots \hfill & \hfill \underset{C_{j1\cdots {C}_j{m}_j}}{D_i}\hfill & \hfill \cdots \hfill & \hfill \underset{C_{n1\cdots {C}_{n{m}_n}}}{D_n}\hfill \end{array}}{\left[\begin{array}{ccccc}\hfill {T}_C^{11}\hfill & \hfill \cdots \hfill & \hfill {T}_C^{1j}\hfill & \hfill \cdots \hfill & \hfill {T}_C^{1n}\hfill \\ {}\hfill \vdots \hfill & \hfill \hfill & \hfill \vdots \hfill & \hfill \hfill & \hfill \vdots \hfill \\ {}\hfill {T}_C^{i1}\hfill & \hfill \cdots \hfill & \hfill {T}_C^{ij}\hfill & \hfill \cdots \hfill & \hfill {T}_C^{in}\hfill \\ {}\hfill \vdots \hfill & \hfill \hfill & \hfill \vdots \hfill & \hfill \hfill & \hfill \vdots \hfill \\ {}\hfill {T}_C^{n1}\hfill & \hfill \cdots \hfill & \hfill {T}_C^{nj}\hfill & \hfill \cdots \hfill & \hfill {T}_C^{nn}\hfill \end{array}\right]} $$(6)$$ {T}_D=\left[\begin{array}{ccccc}\hfill {t}_D^{11}\hfill & \hfill \cdots \hfill & \hfill {t}_D^{1j}\hfill & \hfill \cdots \hfill & \hfill {t}_D^{1n}\hfill \\ {}\hfill \vdots \hfill & \hfill \hfill & \hfill \vdots \hfill & \hfill \hfill & \hfill \vdots \hfill \\ {}\hfill {t}_D^{i1}\hfill & \hfill \cdots \hfill & \hfill {t}_D^{ij}\hfill & \hfill \cdots \hfill & \hfill {t}_D^{in}\hfill \\ {}\hfill \vdots \hfill & \hfill \hfill & \hfill \vdots \hfill & \hfill \hfill & \hfill \vdots \hfill \\ {}\hfill {t}_D^{n1}\hfill & \hfill \cdots \hfill & \hfill {t}_D^{nj}\hfill & \hfill \cdots \hfill & \hfill {t}_D^{nn}\hfill \end{array}\right] $$(7) -
Step 6
Normalize the total influence matrix T C by each dimension shown as T α C .
$$ {T_C}^{\alpha }=\begin{array}{c}\hfill {{}_{D_1}}_{\begin{array}{l}\vdots \\ {}{c}_{1{m}_1}\end{array}}^{c_{11}}\hfill \\ {}\hfill {}_{\vdots}\hfill \\ {}\hfill {{}_{D_i}}_{\begin{array}{l}\vdots \\ {}{c}_{i{m}_i}\end{array}}^{c{}_{i1}}\hfill \\ {}\hfill {}_{\vdots}\hfill \\ {}\hfill {{}_{D_n}}_{\begin{array}{l}\vdots \\ {}{c}_{n{m}_n}\end{array}}^{c_{n1}}\hfill \end{array}\overset{\begin{array}{ccccc}\hfill \underset{C_{11\cdots {C}_{1{m}_1}}}{D_1}\hfill & \hfill \cdots \hfill & \hfill \underset{C_{j1\cdots {C}_j{m}_j}}{D_i}\hfill & \hfill \cdots \hfill & \hfill \underset{C_{n1\cdots {C}_{n{m}_n}}}{D_n}\hfill \end{array}}{\left[\begin{array}{ccccc}\hfill {T}_C^{\alpha 11}\hfill & \hfill \cdots \hfill & \hfill {T}_C^{\alpha 1j}\hfill & \hfill \cdots \hfill & \hfill {T}_C^{\alpha 1n}\hfill \\ {}\hfill \vdots \hfill & \hfill \hfill & \hfill \vdots \hfill & \hfill \hfill & \hfill \vdots \hfill \\ {}\hfill {T}_C^{\alpha i1}\hfill & \hfill \cdots \hfill & \hfill {T}_C^{\alpha ij}\hfill & \hfill \cdots \hfill & \hfill {T}_C^{\alpha in}\hfill \\ {}\hfill \vdots \hfill & \hfill \hfill & \hfill \vdots \hfill & \hfill \hfill & \hfill \vdots \hfill \\ {}\hfill {T}_C^{\alpha n1}\hfill & \hfill \cdots \hfill & \hfill {T}_C^{\alpha nj}\hfill & \hfill \cdots \hfill & \hfill {T}_C^{\alpha nn}\hfill \end{array}\right]} $$(8) -
Step 7
Obtain the unweighted super-matrix w of the factors affecting disposal of dead pigs by farmers.
$$ W={\left({T}_C^{\alpha}\right)}^{\prime } $$(9) -
Step 8
Calculate the weighted normalized super-matrix W α.
$$ {T_D}^{\alpha }=\left[\begin{array}{ccccc}\hfill {t}_D^{11}/{d}_1\hfill & \hfill \cdots \hfill & \hfill {t}_D^{1j}/{d}_1\hfill & \hfill \cdots \hfill & \hfill {t}_D^{1n}/{d}_1\hfill \\ {}\hfill \vdots \hfill & \hfill \vdots \hfill & \hfill \vdots \hfill & \hfill \vdots \hfill & \hfill \vdots \hfill \\ {}\hfill {t}_D^{i1}/{d}_i\hfill & \hfill \cdots \hfill & \hfill {t}_D^{ij}/{d}_i\hfill & \hfill \cdots \hfill & \hfill {t}_D^{in}/{d}_i\hfill \\ {}\hfill \vdots \hfill & \hfill \vdots \hfill & \hfill \vdots \hfill & \hfill \vdots \hfill & \hfill \vdots \hfill \\ {}\hfill {t}_D^{n1}/{d}_n\hfill & \hfill \cdots \hfill & \hfill {t}_D^{nj}/{d}_n\hfill & \hfill \cdots \hfill & \hfill {t}_D^{nn}/{d}_n\hfill \end{array}\right]=\left[\begin{array}{ccccc}\hfill {t}_D^{\alpha 11}\hfill & \hfill \cdots \hfill & \hfill {t}_D^{\alpha 1j}\hfill & \hfill \cdots \hfill & \hfill {t}_D^{\alpha 1n}\hfill \\ {}\hfill \vdots \hfill & \hfill \vdots \hfill & \hfill \vdots \hfill & \hfill \vdots \hfill & \hfill \vdots \hfill \\ {}\hfill {t}_D^{\alpha i1}\hfill & \hfill \cdots \hfill & \hfill {t}_D^{\alpha ij}\hfill & \hfill \cdots \hfill & \hfill {t}_D^{\alpha in}\hfill \\ {}\hfill \vdots \hfill & \hfill \vdots \hfill & \hfill \vdots \hfill & \hfill \vdots \hfill & \hfill \vdots \hfill \\ {}\hfill {t}_D^{\alpha n1}\hfill & \hfill \cdots \hfill & \hfill {t}_D^{\alpha nj}\hfill & \hfill \cdots \hfill & \hfill {t}_D^{\alpha nn}\hfill \end{array}\right] $$(10)$$ where\kern0.5em {d}_{ci}^{11}={\displaystyle \sum_{j=1}^{m_1}{t}_{ij}^{11},i=1,2,\dots {m}_1} $$(11)$$ {W}^{\alpha }={T}_D^{\alpha }W $$(12) -
Step 9
Find the limit of the weighted super-matrix W α by raising it to a sufficiently large power g (i.e., \( \underset{g\to \infty }{ \lim }{\left({W}^{\alpha}\right)}^g \)), until the result converges and becomes a long-term stable super-matrix W*.
Results and discussion
Interrelationships among dimensions and factors affecting the disposal of dead pigs by farmers
The sum of the influence given (r i − c i ) and received (r i + c i ) for each factor and dimension is shown in Table 7. According to Chen and Chi (2015), interrelationships among dimensions and factors affecting the disposal dead pigs by farmers were analyzed by net influence. As shown in Table 7, demographics (D 1) and external environment (D 4) with a net influence of 0.174 and 0.089, respectively, were included in the cause dimensions. Production characteristics (D 2) and level of knowledge (D 3) with a net influence of −0.099 and −0.164, respectively, could be grouped as effect dimensions. Based on the theory of impact-direction map (IDM) developed by Yang and Tzeng (2011) and the dataset of (r i + c i , r i − c i ), an influential network-relationship map (INRM) of the four dimensions and 17 factors that affect disposal of dead pigs by farmers is illustrated in Fig. 1.
As seen in Table 7 and Fig. 1, the four dimensions influence mutually. “Demographics (D 1)” affects “external environment (D 4),” “production characteristics (D 2),” and “level of knowledge (D 3)” (\( {D}_1\to \left\{\begin{array}{ccc}\hfill {D}_4\hfill & \hfill {D}_2\hfill & \hfill {D}_3\hfill \end{array}\right\} \)); external environment (D 4) affects production characteristics (D 2) and level of knowledge (D 3) (\( {D}_4\to \left\{\begin{array}{cc}\hfill {D}_2\hfill & \hfill {D}_3\hfill \end{array}\right\} \)); production characteristics (D 2) affects level of knowledge (D 3) (D 2 → {D 3}). This finding suggests that among the dimensions that affect the disposal of dead pigs by farmers, demographics (D 1) exerted the largest influence on the other three dimensions, level of knowledge (D 3) received the largest influence from the other three dimensions, and external environment (D 4) and production characteristics (D 2) both influenced and were influenced by other dimensions. Additionally, negative behaviors in the disposal of dead pigs by farmers cannot be eliminated despite the fact that the government has been committed to encouraging farmers to expand the scale of farming, cracked down on the sale of pork from dead and diseased pigs, provided subsidies for rural facilities for disposing dead pigs and pig disease insurance, and strengthened regulatory means in recent years; according to this finding, the most plausible reason is that policy-makers did not adequately consider whether farmers in all regions had the ability to understand or participate in relevant policies, thus affecting the effect of the policies.
The value of (r i − c i ) in Table 7 reflects the degree of influence among the 17 factors. “Age (C 12)” was ranked first in terms of direct or indirect influence, indicating that it had varying degrees of influence on all other factors in the system; while “costs and profits (C 24)” was ranked last, indicating that other factors in the system had varying degrees of influence on it. This finding suggests that other factors in the system only had an indirect influence on the disposal of dead pigs by farmers, and the final selection of disposal options was based on the calculation of costs and profits. Therefore, it is speculated that pig farmers decide whether to take positive behaviors in the disposal of dead pigs based on an expectation of profits and costs arising from the action.
Furthermore, Fig. 1 shows the direct relationship among factors in each dimension. In the demographics (D 1) dimension, age (C 12) affects “level of education (C 13)”, “gender (C 11),” “number of household members (C 14),” and “income structure (C 15) (\( {C}_{12}\to \left\{\begin{array}{cccc}\hfill {C}_{13}\hfill & \hfill {C}_{11}\hfill & \hfill {C}_{14}\hfill & \hfill {C}_{15}\hfill \end{array}\right\} \)); level of education (C 13) affects gender (C 11), number of household members (C 14), and income structure (C 15) (\( {C}_{13}\to \left\{\begin{array}{ccc}\hfill {C}_{11}\hfill & \hfill {C}_{14}\hfill & \hfill {C}_{15}\hfill \end{array}\right\} \)); gender (C 11) affects number of household members (C 14) and income structure (C 15) (\( {C}_{11}\to \left\{\begin{array}{cc}\hfill {C}_{14}\hfill & \hfill {C}_{15}\hfill \end{array}\right\} \)); number of household members (C 14) affects income structure (C 15) (C 14 → {C 15}). Using the same logic, we can easily understand the direct relationship among factors in the dimensions production characteristics (D 2), level of knowledge (D 3), and external environment (D 4). In the level of knowledge (D 3) dimension, “knowledge of relevant laws and regulations (C 31)” and “knowledge of pig disease and prevention (C 32)” had very close degrees of net influence (less than 5 %); thus, they should be treated equally with strong interrelationship.
Identifying critical factors affecting disposal of dead pigs by farmers
Table 8 shows the influential weights of four dimensions and 17 factors that affected disposal of dead pigs by farmers. According to the judgment criterion of important factors developed by Huang et al. (2014), critical factors can be identified by influential weights and net influence.
First, in the four dimensions, production characteristics (D 2) had the greatest influential weight (0.360) and a negative net influence (−0.099). This indicates that D 2 was the most important dimension that affected disposal of dead pigs by farmers, plays the largest role in the whole system, and can be influenced by changing the other dimensions. Second, knowledge of relevant laws and regulations (C 31) and knowledge of pig disease and prevention (C 32) had an influential weight of 0.177 and 0.172, respectively, which were the greatest among all factors in the system and the degree of net influence both smaller than zero, so that C 31 and C 32 were two critical factors in the system. These findings are similar to the relevant research conclusions of Toma et al. (2013) and Launio et al. (2014). Third, costs and profits (C 24) had the third greatest influential weight and smallest net influence (−1.073), so that C 24 could also be considered one of the critical factors in the system. This conclusion is corroborated by our survey results. In fact, the primary consideration was the cost factor in decision-making regarding disposal of dead pigs by the 654 farmers surveyed. Fourth, “scale of farming (C 22)” and “pattern of farming (C 23)” had the same influential weight (0.097), which was ranked fourth, and its corresponding dimension was the most important; thus, C 22 and C 23 could also be considered two critical factors in the system. The result that C 22 and C 23 had the same influential weight may be because of the fact that they are both related to the annual production of pigs ready to be slaughtered and that C 22 is the subdivision of C 23. This result is consistent with the conclusions of Sun et al. (2012) that scale of farming and pattern of farming were the underlying causes affecting positive behaviors of farmers in China.
As shown in Table 8, gender (C 11), age (C 12), education level (C 13), and the number of household members (C 14) were four factors that had the smallest influential weight. Moreover, these factors are inherent attributes of farmers and thus can hardly be influenced by other factors in the system, so they are non-critical factors. “Income structure (C 15),” “years of farming experience (C 21),” “government subsidies for harmless disposal (C 41),” “insurance and compensation for dead pigs (C 42),” “legal restrictions and penalties (C 43),” “incentives (C 44),” “government regulation (C 45),” and “peer behavior (C 46)” had a moderate influential weight among all the factors; these factors can both influence and be influenced by other factors in the system and therefore are secondary critical factors.
In general, researchers identify critical factors using DEMATEL based on the value of (r i + c i ) (Afsharkazemi et al. 2013; Wu et al. 2013). In order to verify the applicability of DANP, the research findings were compared to the value of (r i + c i ) in Table 7. Production characteristics (D 2) had the highest value of (r i + c i ). According to the value of (r i + c i ), the top five influence factors were the scale of farming, pattern of farming, costs and profits, knowledge of relevant laws and regulations, and income structure. This was fairly consistent with the critical factors identified based on influential weights. Although knowledge of pig disease and prevention was ranked sixth, it had a difference of only 0.012 from income structure in the degree of total influence. Moreover, as previously demonstrated, the two knowledge factors significantly affected each other. Therefore, the five critical factors identified in this study were accurate. In other words, DANP is a powerful and effective method to identify the critical factors affecting disposal of dead pigs by farmers.
Conclusions and policy implications
The main conclusions are
-
1.
The results of this study reveals that the four dimensions and 17 factors that affect the disposal of dead pigs by farmers are interconnected in a complex network of mutual influence and interaction.
-
2.
Production characteristics are closely related to the disposal of dead pigs by farmers. Among the dimensions that affect the disposal of dead pigs by farmers, production characteristics are the most important dimension. This is reflected in the fact that the current improper production pattern may be one of the main causes of dumping of dead pigs into rivers and lakes and illegal selling of dead pigs to middlemen or direct processing and marketing of dead pigs in China.
-
3.
Among the 17 factors that affect the disposal of dead pigs by farmers, knowledge of relevant laws and regulations, knowledge of pig disease and prevention, costs and profits, scale of farming, and pattern of farming are five critical factors. This is reflected as follows: Pig farmers’ poor knowledge of relevant laws and regulations and pig disease and prevention may be the main cause of frequent negative behaviors in the disposal of dead pigs; negative disposal of dead pigs by farmers may be primarily driven by the pursuit of economic gain, i.e., to compensate loss from pig deaths and save harmless disposal costs; and common negative behaviors in the disposal of dead pigs by farmers may be significantly related to small, scattered pig farming in China.
Base on the above conclusions, the following recommendations are made for eliminating negative behaviors in the disposal of dead pigs by farmers by the relevant governments:
-
1.
To transform the pattern of pig farming. Relevant government departments should guide the transformation of small, scattered pig farmers to large-scale, professional ones using economic and policy instruments. Moreover, the transformation capacity of individual pig farmers has to be considered during policy guidance.
-
2.
To guarantee normal economic benefits of farmers in harmless disposal of dead pigs. The government should consider the actual cost of harmless disposal of dead pigs and develop appropriate financial assistance policies to ensure that financial compensation for harmless disposal is greater than the costs for farmers.
-
3.
To improve of farmers’ knowledge of pig disease and prevention. Scientific knowledge of pig farming and disease prevention should be promoted among farmers through a variety of effective ways, with emphasis on importance of harmless disposal of dead pigs, in order to reduce the number of dead pigs from the source.
-
4.
To educate farmers about laws and regulations regarding harmless disposal of dead pigs. Local governments should adopt flexible ways to educate pig farmers about Animal Epidemic Prevention Law, Food Safety Law, Livestock Pollution Regulations, and other laws and regulations, thus raising their awareness of the law, in order to control the negative behaviors in disposal of dead pigs by farmers by legal constraints.
The efforts of this study are summarized as follows: First, the interrelationships among dimensions and factors that affect the disposal of dead pigs by farmers were systematically analyzed; second, it was demonstrated that DANP was effective in identifying critical factors affecting the disposal of dead pigs by farmers; and third, the expert scoring method and field survey were combined to avoid interest demands of the sample and also allow a scientific setting of influencing factors. The contribution of this study is to reveal the complexity of factors affecting the disposal of dead pigs by farmers in China; it is a long process and requires joint efforts of the government and farmers to eliminate the dumping of dead pigs into rivers and lakes and illegal selling of dead pigs to middlemen or direct processing and marketing of dead pigs. There are, of course, limitations to the present study that should be noted. In general, the survey sample, 654 pig farmers in Funing County, Jiangsu Province, is not representative of the entire country. More regions should be surveyed, and a large sample should be collected in future studies in order to provide more universal findings.
Notes
In China, dead pigs must be harmlessly disposed according to governmental regulations. Therefore, harmless disposal of dead pigs by farmers is referred to as a positive behavior in this report. On the other hand, dumping of dead pigs into rivers and lakes and illegal selling of dead pigs to middlemen or direct processing and marketing of dead pigs are referred to as negative behaviors.
Source: Statistical Bureau of People’s Republic of China, http://219.235.129.58/reportYearQuery.do?id=1400&r=0.43901071841247474. The number and growth rate of pig deaths were calculated based on the lowest normal death rate of 3 % in adult pigs.
According to the Chinese statistical standard, the scale of farming refers to the annual production of pigs ready to be slaughtered in this report.
China has enacted a series of policies, laws, and regulations for the scientific disposal of dead pigs. Knowledge of relevant laws and regulations mainly refers to knowledge of such policies, laws, and regulations in this study.
References
Abdulai A, Monnin P, Gerber J (2008) Joint estimation of information acquisition and adoption of new technologies under uncertainty. J Int Dev 20(4):437–451
Afsharkazemi MA, Manouchehri J, Salarifar M, Nasiripour AA (2013) Key factors affecting the hospital performance: a qualitative study using fuzzy logic. Qual Quan 47(6):3559–3573
Boccaletti S, Nardella M (2000) Consumer willingness to pay for pesticide-free fresh fruit and vegetables in Italy. Int Food Agribus Manag Rev 3(3):297–310
Caixin Online (2013) NBC talk show on dumping of dead pigs into Huangpu River. http://video.caixin.com/2013-03-25/100505834.html. Accessed 12 January 2014
Chen FH, Chi DJ (2015) Application of a new DEMATEL to explore key factors of China’s corporate social responsibility: evidence from accounting experts. Qual Quant 49(1):135–154
Chen XG, Chen LT, Zhu HH, Zhang KC, He GL, Yang JH (2010) Investigation of a case of illegal selling of dead pigs and some reflections. Shanghai J Anim Husb Vet Med 6(1):52–53
Chen Y, Chen K, Li Y (2011) Simulation on influence mechanism of environmental factors to producers’ food security behavior in supply chain. In: IEEE Eighth International Conference on Fuzzy Systems and Knowledge Discovery (FSKD), pp. 2104-2109
Cheng CY, Hsieh CH, Yang YS (2014) Who would engage in unethical behavior? Should organizations bear the responsibility? Qual Quant 48(4):2341–2354
Cui XL, Cai YY, Zhang AL (2011) Willingness of farmers to reduce pesticide application rate of chemical fertilizer and the influencing factors. Rural Econ 11(25):97–100
Danso G, Drechsel P, Fialor S, Giordano M (2006) Estimating the demand for municipal waste compost via farmers’ willingness-to-pay in Ghana. Waste Manage 26(12):1400–1409
Deressa TT, Hassan RM, Ringler C, Alemu T, Yesuf M (2009) Determinants of farmers’ choice of adaptation methods to climate change in the Nile Basin of Ethiopia. Glob Environ Change 19(2):248–255
Edwards-Jones G (2006) Modelling farmer decision-making: concepts, progress and challenges. Anim Sci 82(6):783–790
Greiner R, Gregg D (2011) Farmers’ intrinsic motivations, barriers to the adoption of conservation practices and effectiveness of policy instruments: empirical evidence from northern Australia. Land Use Policy 28(1):257–265
Hsu CH, Wang FK, Tzeng GH (2012) The best vendor selection for conducting the recycled material based on a hybrid MCDM model combining DANP with VIKOR. Resour Conserv Recyc 66(12):95–111
Huang CN, Liou JJH, Chuang YC (2014) A method for exploring the interdependencies and importance of critical infrastructures. Knowl-Based Syst 55(8):66–74
Ithika CS, Singh SP, Gautam G (2013) Adoption of Scientific Poultry Farming Practices by the Broiler Farmers in Haryana, India. Iran J Appl Anim Sci 3(2):417–422
Jacob C, Mathiasen L, Powell D (2010) Designing effective messages for microbial food safety hazards. Food Control 21(1):1–6
Jayasinghe-Mudalige U, Henson S (2007) Identifying economic incentives for Canadian red meat and poultry processing enterprises to adopt enhanced food safety controls. Food Control 18(11):1363–1371
Läpple D (2010) Adoption and abandonment of organic farming: an empirical investigation of the Irish drystock sector. J Agric Econ 61(3):697–714
Launio CC, Asis CA, Manalili RG, Javier EF, Belizario AF (2014) What factors influence choice of waste management practice? Evidence from rice straw management in the Philippines. Waste Manag Res 32(2):140–148
Lian JY (2013) Legal reflections on burying of dead pigs in rivers. Wuhan Acad J 3(7):29–32
Liu DY (2012) Importance, problems, and solutions of pig insurance. Tech Advis Anim Husb 2(178):281–281
Liu CY (2013) Dead pigs scandal questions China’s public health policy. The Lancet 381(9877):1539
Loretz M, Stephan R, Zweifel C (2011) Antibacterial activity of decontamination treatments for pig carcasses. Food Control 22(8):1121–1125
Lu MT, Lin SW, Tzeng GH (2013) Improving RFID adoption in Taiwan's healthcare industry based on a DEMATEL technique with a hybrid MCDM model. Decis Support Syst 56(26):259–269
Marshall BM, Levy SB (2011) Food animals and antimicrobials: impacts on human health. Clin Microbial Rev 24(4):718–733
Mol APJ (2014) Governing China's food quality through transparency: a review. Food Control 43(9):49–56
Mzoughi N (2011) Farmers’ adoption of integrated crop protection and organic farming: do moral and social concerns matter? Ecol Econ 70(8):1536–1545
Ni YF (2012) Hazards, identification and control of dead pigs. Meat Ind 11(19):40–44
Reig M, Toldrá F (2008) Veterinary drug residues in meat: concerns and rapid methods for detection. Meat Sci 78(1):60–67
Shen YJ, Zhao LX, Meng HB (2013) Present situation and countermeasures of harmless disposal of dead livestock. J Agric Sci Technol 15(6):167–173
Sun SM, Zhang YY, Zhang JR (2012) Empirical analysis of factors influencing willingness of pig farms (farmers) to perform positive behaviors based on the Logit-ISM model. Chin Rural Econ 10(5):24–36
Tan LK, Ooi PT, Thong KL (2014) Prevalence of Yersinia enterocolitica from food and pigs in selected states of Malaysia. Food Control 35(1):94–100
Tey YS, Brindal M (2012) Factors influencing the adoption of precision agricultural technologies: a review for policy implications. Precis Agric 13(6):713–730
Toma L, Stott AW, Heffernan C, Ringrose S, Gunn GJ (2013) Determinants of biosecurity behavior of British cattle and sheep farmers—a behavioral economics analysis. Prev Vet Med 108(4):321–333
Vignola R, Koellner RT, Scholz RW, McDaniels TL (2010) Decision-making by farmers regarding ecosystem services: factors affecting soil conservation efforts in Costa Rica. Land Use Policy 27(4):1132–1142
Wu LH, Zhang QQ, Shan LJ, Chen ZX (2013) Identifying critical factors influencing the use of additives by food enterprises in China. Food Control 31(2):425–432
Yang JL, Tzeng GH (2011) An integrated MCDM technique combined with DEMATEL for a novel cluster-weighted with ANP method. Expert Syst Appl 38(3):1417–1424
Yu Y, Zhang H, Hu H (2012) Identifying factors influencing investment in environmental protection by farmers from the perspective of sewage disposal subsidies: based on a survey among pig farmers in Shanghai, Jiangsu, and Zhejiang. China Popul Resour Environ 22(2):159–163
Zhang GX, Zhang SX (2013) Factors influencing prevention and control by farmers under the risk of animal diseases. Rural Econ 2(25):105–108
Acknowledgments
This research work was financially supported by Study of Co-governance for Food Safety Risk in China, one of the Key Projects of National Social Science Foundation of China in 2014 (Project Approval No. 14ZDA069), and the National Natural Science Foundation of China (Project Approval No. 71273117), and Central University Basic Research Funds (Project Approval Nos. JUSRP51325A and JUSRP51416B), and Study of Food Safety Consumption Policy: the Case of Traceable Pork, a project of the Six Top Talents in Jiangsu Province (Project Approval No. 2012-JY-002), and Research on Chinese Food Safety Risk Control, a project of college Innovation Team of Jiangsu Province social science (Project Approval No. 2013-011).
Author information
Authors and Affiliations
Corresponding author
Additional information
Responsible editor: Philippe Garrigues
Rights and permissions
About this article
Cite this article
Wu, L., Xu, G. & Wang, X. Identifying critical factors influencing the disposal of dead pigs by farmers in China. Environ Sci Pollut Res 23, 661–672 (2016). https://doi.org/10.1007/s11356-015-5284-y
Received:
Accepted:
Published:
Issue Date:
DOI: https://doi.org/10.1007/s11356-015-5284-y