Abstract
Polycyclic aromatic hydrocarbons (PAHs) associated with the inhalable fraction of particulate matter were determined for 1 year (2009–2010) at a school site located in proximity of industrial and heavy traffic roads in Delhi, India. PM10 (aerodynamic diameter ≤10 μm) levels were ∼11.6 times the World Health Organization standard. Vehicular (59.5 %) and coal combustion (40.5 %) sources accounted for the high levels of PAHs (range 38.1–217.3 ng m−3) with four- and five-ring PAHs having ∼80 % contribution. Total PAHs were dominated by carcinogenic species (∼75 %) and B[a]P equivalent concentrations indicated highest exposure risks during winter. Extremely high daily inhalation exposure of PAHs was observed during winter (439.43 ng day−1) followed by monsoon (232.59 ng day−1) and summer (171.08 ng day−1). Daily inhalation exposure of PAHs to school children during a day exhibited the trend school hours > commuting to school > resting period in all the seasons. Vehicular source contributions to daily PAH levels were significantly correlated (r = 0.94, p < 0.001) with the daily inhalation exposure level of school children. A conservative estimate of ∼11 excess cancer cases in children during childhood due to inhalation exposure of PAHs has been made for Delhi.
Similar content being viewed by others
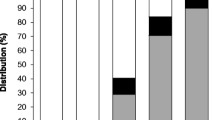
Explore related subjects
Discover the latest articles, news and stories from top researchers in related subjects.Avoid common mistakes on your manuscript.
Introduction
Children represent the largest subgroup of the population susceptible to adverse health effects of air pollution (Dockery et al. 2005).Chronic exposure to outdoor air pollution is associated with increased respiratory symptoms in children (Hoek et al. 2012) along with respiratory hospital admissions, school and kindergarten absences, reduced lung function, and increased medication use in children with asthma (American Thoracic Society 1996). Children have a higher rate of ventilation per minute, spend more time outdoors than adults, and therefore have increased exposure to outdoor air pollution (Tuntawiroon et al. 2007). Epidemiological and experimental data indicate increased cancer risks following childhood exposure to carcinogens as compared with exposure occurring at a mature age because of the significant amount of time after exposure at childhood for the possible manifestation of resultant health effects later in life (Anderson et al. 2000).
Of the various constituents of ambient particulate matter contributing to health concerns, polycyclic aromatic hydrocarbons (PAHs) are among the most ubiquitous combustion-derived organic contaminants. PAHs are known for their carcinogenic, mutagenic, and teratogenic effects on organisms (Perera 1997). Various adverse health effects such as decline of lung function, retardation of growth in infants, and delay in child's neurodevelopment from prenatal exposure have been reported among children residing near a known PAH source (Northridge et al. 1999; Dejmek et al. 2000; Perera et al. 2006).
Outdoor air quality ranks sixth among various risk factors in South Asia (including India, Pakistan, Bangladesh, and Sri Lanka) according to Lancet’s 2010 Global Burden of Disease (Lim et al. 2012). The World Health Organization (WHO) has declared 1,68,601 deaths attributable to outdoor air pollution in 2008 as against 1,40,937 in 2004 in India, which is second to China. However, in children under 5 years, India ranks first in number of deaths attributable to outdoor air pollution during 2004 and 2008. Delhi, the capital of India, is one of the worst affected cities in terms of air pollution. A recent study has estimated an excess mortality of ∼10,500 persons/year due to air pollution in Delhi (Gurjar et al. 2010) while the incidence of respiratory ailments in the city is known to be as high as 12 times the national average (Pandey et al. 2005). Alarmingly, according to the Harvard International Review, two out of every five residents of Delhi suffer from a respiratory disease (Sequeira 2008). Despite the awareness, there has been comparatively little work done in estimating the effect of school location and exposure during commuting to and from the school on child health, with most of the studies focusing instead on residential locations (Rickwood and Knight 2009).
In light of the above, the present study seeks to assess the potential health risks of exposure to carcinogenic air pollutants in children studying in a school located in close proximity of an industrial area and high traffic roads in Delhi, India.
Materials and methods
Study area
Delhi (28°24′E to 28°53′E, 76°20′N to 77°20′N; mean elevation ∼216 m a.m.s.l.) is spread over an area of 1,483 km2. It is the most populous metropolis in India with a population of around 17 million and also has the highest population density of 11,297 persons per square kilometer in India (Census 2011). The region is characterized by semi-arid climate, with intensely hot summers (monthly mean temperatures of 32–34 °C in May–June) and cold winters (monthly mean temperatures of 12–14 °C in December–January). The mean annual rainfall is 714 mm, of which around 80 % is received during the monsoon months (July to October). Low temperatures, ground-based temperature inversions, and calm atmospheric conditions are a regular feature of winters in the region that restricts the mixing height to low levels that in turn impedes pollutant dispersion. The predominant wind direction is from the north and northwest except during the monsoon season that is characterized by easterly or southeasterly winds.
Delhi houses around 155,000 industrial units in 32 industrial estates or flatted factories complex. The vehicular population of Delhi (6.9 million) is the highest in India and has grown at a rate of 105 % between 2000–2001 and 2010–2011 with an average annual increase of approximately 7.3 % from 2008–2009 to 2010–2011. In addition to industrial and vehicular sources, air quality in Delhi is faced with challenges from emissions from two coal-based power plants (Badarpur thermal power station, 720 MW; and Rajghat power station, 135 MW). It also houses four gas-based power plants [Pragati Bawana combined cycle gas turbine (CCGT) power station, 1,000 MW; Indraprastha (Delhi) CCGT power station, 282 MW; Pragati CCGT power station, 330 MW; and Rithala CCGT power station, 108 MW]. Liquefied petroleum gas (LPG)/piped natural gas (PNG) is the most commonly used cooking fuel (82.7 %) followed by kerosene (6.4 %) and biomass (7.2 %) in households of Delhi (Census 2011). Another anthropogenic source includes burning of wood, charcoal, kerosene, cow dung, paper, or other solid wastes by population dwelling in the city slums (∼3.6 million) to keep themselves warm during cold winter nights and for cooking (Ganguly et al. 2006). A recent study by Sahu et al. (2011) estimated 34 %, 29 %, 26 %, and 11 % relative contributions from residential, transport, industry, and power sectors, respectively, to PM10 emissions (excluding road dust) in 2010 in the National Capital Region of Delhi.
Sampling site
Location of the sampling site is shown in Fig. 1. Sampling was carried out on the rooftop (∼12 m from the ground) of Kulachi Hansraj Model School, Ashok Vihar Phase-1 in North Delhi. This particular campus houses the primary section (classes I–VI) and the “Manovikas Kendra”, a center for children with special needs, of the school. In the immediate proximity of the school is a residential colony. However, the school lies just ∼400 m south east of Wazirpur Industrial Area which is one of the major industrial estates in Delhi that houses activities such as pickling, annealing and re-rolling of stainless steel sheets, electroplating, steel fabrication, dyeing, rubber and plastic goods manufacturing, copper and brass sheets with casting and melting, re-treading of tires, automobile spare part manufacturing, metal casting, etc. Additionally, Mangolpuri Industrial Area, Lawrence Road Industrial Area, Badli Industrial Area, Jahangirpuri Industrial Area, and Dr Lohia Industrial Area are present within a radius of about ∼7 km. The heavy-traffic ring road (∼600 m) and the grand trunk road (∼1.2 km) pass nearby the sampling site.
Sampling protocol
PM10 samples were collected at the site for a period of 1 year (December 2009–November 2010). The sampling frequency was once a week and sampling duration for each sample was 24 h. A number of samples were lost due to logistical problems such as electrical failure and blower malfunction. A total of 38 samples were collected over the course of this study. PM10 was trapped on Whatman GF/A (8″ × 10″) glass fiber filters (GFFs) (precombusted at 450 °C for 12 h) using high-volume samplers (Respirable Dust Sampler, Model NPM-HVS/R; Netel India Ltd.) having a constant flow rate of 1.2 m3 min−1. The combination of high volume sampling with GFFs has been used widely for estimation of particulate PAHs (Delgado-Saborit et al. 2010; Tan et al. 2006). Filters were transported to and from the field in sealed polyethylene bags and were desiccated for 48 h before and after use. Utmost care was taken to avoid handling losses. PM10 loads were determined gravimetrically by weighing the filters twice in a microbalance (sensitivity of 0.0001 g, Model AE163; Mettler, Switzerland) after proper conditioning. Samples were stored in a refrigerator (4 °C) until chemical analysis.
Chemicals and reagents
Standard mixture containing 16 PAHs (16 compounds specified in EPA Method 610) and deuterated PAHs internal standard mixture (naphthalene-d8, acenaphthene-d10, phenanthrene-d10, chrysene-d12, and perylene-d12) were procured from Supelco (Bellefonte, PA, USA). All solvents (toluene, n-hexane, and acetonitrile) were purchased from Merck (India) Ltd. and were of high-performance liquid chromatography (HPLC) grade. Water used in the analyses was high-purity deionized water (18.2 MΩ cm−1) taken from the Milli-Q system (Millipore, USA).
PAHs determination
Analysis of PAHs was carried out based on the protocol described in Sarkar et al. (2010). Briefly, PAHs were extracted using ultrasonication with toluene as solvent (Sonicator 3000; Misonix Inc., USA) and were subsequently concentrated to 0.5–2 ml by rotary evaporation (Büchi rotavapor, Switzerland). This was followed by silica gel column cleanup and final analysis using a HPLC system (Model 510; Waters, USA) equipped with a tunable absorbance UV detector (254 nm) and a PAH C18 column (4.6 mm × 250 mm, particle size 5 μm; Waters). Quantification of PAHs was done by internal calibration method and their identification was carried out by comparing their retention times with those of analytical standards. Filters were spiked with internal standard solution prior to extraction in order to check procedural performance and matrix effects. Surrogate compounds were represented for the analyses as follows: phenanthrene-d10 for fluoranthene (Flan) and pyrene (Pyr), chrysene-d12 for benz[a]anthracene (B[a]A) and chrysene (Chry), perylene-d12 for benz[b]fluoranthene (B[b]F), benz[k]fluoranthene (B[k]F), benz[a]pyrene (B[a]P), dibenz[a,h]anthracene (DB[ah]A), benz[ghi]perylene (B[ghi]P), and indeno[1,2,3-cd]pyrene (IP).
Although all of the 16 US EPA priority PAHs were analyzed, the low molecular weight species (two- and three-ring) were not considered for calculating total PAHs, diagnostic ratios, source apportionment by principal component analysis–multiple linear regression (PCA–MLR), and risk assessment. The high volatility of these PAHs and the use of high volume sampling might impose uncertainties in the measured concentrations.
The focus of the present study rests on addressing the particulate mass fraction of PAHs and the associated health risks. The two- and three-ring PAHs are known to exist almost exclusively in the gaseous phase in the atmosphere while a considerable fraction of the four-ring PAHs are also known to partition to the gaseous phase (Finlayson-Pitts and Pitts 2000; Masih et al. 2012). The five- and six-ring PAHs, on the other hand, are known to exist predominantly in the particulate phase in the ambient air. Estimation of particulate PAH fractions is important because high molecular weight PAHs associated with particles are known to possess higher toxic equivalence factors and are widely regarded as carcinogenic and/or mutagenic (Xue and Warshawsky 2005). Studies have shown that inflammatory reactions due to particles in lung tissues are likely to increase the carcinogenicity of PAHs associated with inhaled particulates (Bonn and Driscoll 1996). It must, however, be mentioned at this point that PAH levels reported in this study correspond to their particulate mass fractions only and are not representative of their total atmospheric levels. It is noteworthy that the gaseous fractions of these PAHs contribute to an additional health risk which is not addressed here. Besides gas–particle partitioning, the study is also limited to some extent by the fact that same total PAH levels but under different conditions (temperature, nature of aerosol matrix, etc.) might lead to a different phase equilibrium and, therefore, a different risk.
Analytical quality control
The HPLC system was periodically calibrated using at least five standards covering the range of concentrations encountered in ambient air work. The calibration curves were linear in the concentration range with linear regression coefficients (R 2) >0.99 for linear least-squares fit of data. Samples were analyzed in triplicates (RSD < 10 %) to ensure precision. Field blanks (around 10 % of total number of samples) and reagent blanks (one for each batch of samples) were analyzed to determine analytical bias. PAHs in blank samples were <2 % of real sample concentrations. Detection limits (3σ) of analyzed PAHs varied from 0.01 to 0.11 ng m−3 while their recoveries ranged from 77 to 94 %.
Results and discussion
Levels and profiles of PM10-bound PAHs
Mean annual concentration of PM10 at the study site was 222.2 ± 111.3 μg m−3. The observed value is ∼3.8 times the Indian National Ambient Air Quality Standard (NAAQS) of 60 μg m−3 (MoEF 2009) and ∼11.6 times the WHO air quality standard (20 μg m−3) for PM10. The 24 h PM10 standard by NAAQS (100 μg m−3) and WHO (50 μg m−3) were exceeded on all the sampling days. Seasonal variation of PM10 at the study site was statistically significant (ANOVA, F = 3.6, p < 0.05) with highest mean concentration in the summer followed by winter and monsoon. High concentration of PM10 in summers can be explained by the frequent occurrence of dust storms in Delhi, carrying approximately 500–800 tons per square mile of coarse particulates from the Thar Desert into Delhi (Khillare and Sarkar 2012). Crustal resuspension during the hot dry season is also responsible for the elevated summertime PM10 levels in Delhi. Wintertime concentrations, though not significantly different (p = 0.11) from summertime PM10 concentrations, exhibit high levels because of the lower mixing height of the atmosphere along with relatively calm atmospheric conditions. The monsoon season, marked by high humidity and precipitation scavenging, is expected to record lower ambient PM10 concentrations.
Individual concentrations of the studied PAHs are provided in Table 1. The annual mean of Σ10PAHs (the sum of four-, five-, and six-ring PAHs among the 16 USEPA priority PAHs) at the study site was 103.3 ± 50.4 ng m−3 with a range of 38.1–217.3 ng m−3. The observed value is found to be an order of magnitude higher than the ones reported from European countries (Saarnio et al. 2008), South America (Quiteiro et al. 2007; Mugica et al. 2010), USA (Park et al. 2002), and UK (Lee and Jones 1999). Chinese studies (Wang et al. 2008; Shi et al. 2009) have reported higher concentration of PAHs, and comparable levels have been reported from Turkey (Akyüz and Çabuk 2009). Overall, it has also been reported that India ranks second to China in terms of PAH emissions (90 Gg/year in 2004) and high emission of PAHs from Indian cities has been attributed to rapid growths of population and economy, and less energy utilization efficiency (Zhang and Tao 2009).
Winter time PAHs at the study site was significantly higher (F = 63.37, p < 0.001) than monsoon and summer time concentrations (Fig. 2). Figure 3 shows the monthly variation of PAH concentrations at the site along with monthly mean temperatures observed during the study period. Average winter time PAHs concentration was ∼2.6 and ∼2 times higher than those observed during summer and monsoon months, respectively. Summer and monsoon levels of PAHs did not exhibit significant differences (p = 0.16). Higher concentration of organic pollutants in winter period is associated with lower ambient temperature, slower rate of photolysis, and lower dispersion (Li et al. 2006; Caricchia et al. 1999). PAH emission from vehicles is also reported to be higher during the winter because of low ambient temperature and increased cold start impacts (Ludykar et al. 1999). Traffic congestion, rampant in Delhi, especially during winters due to poor visibility resulting from fog and haze, is also reported to be responsible for increased level of PAHs in the winter time atmosphere (Rajput and Lakhani 2010). Wintertime space heating is not very conspicuous in Delhi; instead, biomass and wood burning in suburban and slum areas is usually observed. Summer concentrations are influenced by high temperature, high rate of photolysis, better dispersion, and gas–particle partitioning. Humidity and precipitation play a scavenging role during the monsoon period. However, sparse precipitation during the monsoon might have resulted in higher mean concentration during the season than summer.
The five-ring PAHs that include B[b]F, B[k]F, B[a]P, and DB[ah]A accounted for ∼42 % of the Σ10PAHs followed by four-ring PAHs (Flan, Pyr, B[a]A, and Chry) accounting for ∼38 % and six-ring (B[ghi]P and IP) PAHs accounting for ∼19 % (Fig. 4).
Sources of PAHs
PAH isomer diagnostic ratios
Characteristic PAH isomer ratios have been used to distinguish and trace source types of PAHs in the environment. The observed PAH isomer ratios along with the corresponding source signatures from published literature are provided in Table 2. B[a]A/B[a]A + Chry ratio indicated towards combustion sources of PAHs. Flan/Flan + Pyr ratio indicated coal or wood combustion. Ratios of IP/B[ghi]P and IP/IP + B[ghi]P indicated gasoline emissions from vehicular sources. B[a]A/Chry ratio suggested coal or coke combustion while B[b]F/B[k]F ratios indicated vehicular emissions. B[a]P/B[ghi]P ratio indicated a coal combustion source of PAHs. Overall, PAH isomer diagnostic ratios indicated vehicular and coal combustion as the source of PAHs at the study site.
Principal component analysis
Principal component analysis coupled with multiple linear regression (PCA–MLR) performed with SPSS 14 was used to identify and quantify sources of PAHs. Details of the PCA–MLR procedure can be found in Larsen and Baker (2003). The results are shown in Table 3.
Seventy-five percent of the variance in the data was explained by two components. PC1 was loaded with B[a]A, B[b]F, B[k]F, B[a]P, DB[ah]A, B[ghi]P, and IP. PC1 was assigned to vehicular sources since high loadings of B[a]A, DB[ah]A, B[ghi]P, and IP have been associated with gasoline emissions (Guo et al. 2003) while B[b]F and B[k]F are known to be markers of diesel emissions (Khalili et al. 1995). Vehicular traffic impacts at the site is evident by its immediate proximity (∼600 m northwest) to the high-traffic inner ring road. The two ring roads (outer and inner) carry a major portion of traffic movements in Delhi and surround the study site in north and northwestern directions. Several studies have concluded that there is a pollution gradient trend with respect to distances from major roads (Beckerman et al. 2008). Particularly for particles, levels in upwind sites are known to drop off to background levels within 100 m whereas the same does not equate with the background levels until 300–800 m downwind (Zhou and Levy 2009; Reponen et al. 2003). In this context, it is important to mention that around 55 % of Delhi's population lives within 50 m of major roads and is exposed most to high air pollution levels (Rajarathnam et al. 2011). PC2 was loaded with Flan, Pyr, and Chry that are tracers of coal combustion (Khalili et al. 1995) possibly originating from domestic coal combustion in surrounding low income group residential colonies and JJ clusters (shack settlements that have the attributes of sub-standard housing, unsanitary conditions, and poverty of the slums but are not notified under the Slum Areas Act are called JJ clusters; abbreviated colloquial Hindi term Jhuggi jhopdi) of Pitampura, Azadpur, Shakurpur, and Wazirpur. It is worth mentioning that Delhi houses ∼2.8, 3.6, and 2.4 million people in JJ clusters, slums, and re-settlement colonies, respectively, as of 2011 (Planning Department 2011), and coal/coke combustion is rampant in these areas for domestic cooking as is winter time heating by wood and biomass combustion. These PAH tracers could also be sourced from coal combustion in small-scale boilers used in industrial units of Wazirpur Industrial Area that is located in close proximity to the sampling site.
The PCA–MLR model apportioned the following annual percentage contributions of the identified sources to ∑10PAHs: vehicular emissions, 59.5 %; and coal combustion, 40.5 %. Observed and model-predicted ∑10PAHs agreed strongly (R 2 = 0.994), indicating that the variations in the PAHs level were very well explained by the apportioned sources (Fig. 5). Time evolution of source contributions (ng m−3) to Σ10PAHs is shown in Fig. 6.
Health risk assessment
Carcinogenic PAHs
The International Agency for Research on Cancer (IARC) has classified seven PAHs as human carcinogens (group 1) and probable and possible human carcinogens (group 2A and 2B) (IARC 2012). These include B[a]A, Chry, B[b]F, B[k]F, B[a]P, DB[ah]A, and IP. The sum of these seven PAHs (Σ7PAHs) at the site was 77.5 ± 38.6 ng m−3 (annual mean) while mean contribution of Σ7PAHs to Σ10PAHs was ∼75 %. Σ7PAHs were highly correlated (r = 0.96, p < 0.001) to Σ10PAHs suggesting that Σ10PAH distributions at the study site were heavily influenced by the distribution of carcinogenic PAHs. Importantly, the overall exposure to carcinogenic compounds in atmosphere is also increased by aromatic ketones and quinones, often more carcinogenic than the parent PAHs, produced by atmospheric photooxidation and/or photolysis of PAHs (Saarnio et al. 2008).
B[a]P equivalents
B[a]P equivalent concentrations of a mixture of PAHs is useful in assessing carcinogenic risk from exposure to PAHs. Toxic equivalent factors (TEF) for each of PAHs, with respect to B[a]P, the most potent human carcinogen, developed by Nisbet and LaGoy (1992) have been used to calculate the B[a]P equivalent concentrations as follows:B[a]P eq = Σ i C i * TEF i ; where C i is the concentration of ith species
The mean annual B[a]Peq concentration at the site was found to be 30.8 ± 16 ng m−3. This implies a potentially strong exposure to the children when compared with B[a]P levels of 0.99, 2.13, 1.29, 2.48, and 6.27 ng m−3 from provinces of Thailand, Bangkok, Nagasaki, Rome, and Copenhagen, respectively (Pengchai et al. 2009; Norramit et al. 2005; Wada et al. 2001; Menichini et al. 1999; Nielsen et al. 1996).
Additionally, the relative potency factor (RPF) approach, as given by USEPA (2010), was applied to calculate the B[a]Peq concentrations as follows:
where E is the benzo[a]pyrene equivalent exposure presented by the mixture, RPF i is the relative potency factor of the ith PAH detected in the mixture, C i is the dose or concentration of the ith PAH detected in the mixture, and X is the dose or concentration of benzo[a]pyrene in the mixture.
B[a]Peq concentrations by the RPF method was found to be 165.7 ± 118.1 ng m−3 which is more than five times the value deduced from the approach adopted by Nisbet and LaGoy (1992). Therefore, in order to have a conservative estimate of B[a]Peq concentrations, the values obtained by using TEF values of Nisbet and LaGoy (1992) were subsequently used for calculating daily exposure levels and health risk assessment.
Mutagenicity of individual PAHs relative to B[a]P has also been assessed using the mutagenic equivalency factor (MEF) (Durant et al. 1996, 1999; Jung et al. 2010). Mutagenic activity may have implications for other non-cancerous adverse health effects, such as pulmonary diseases, if not always cancer (Zeiger 2001; DeMarini et al. 2004). B[a]P-MEQ (mutagenic equivalents, ng m−3) for the sum of nonvolatile PAHs (∑8PAH; molecular weight ≥ 228) were calculated by multiplying the concentrations of each PAH with its MEF relative to B[a]P as follows:
The B[a]P-MEQ∑8PAH at the site was 26.4 ± 11.9 ng m−3. B[a]P equivalent concentrations through both TEF and MEF approach exhibit exposure risks in the seasonal order of winter > monsoon > summer at the study site. Similar trend of higher B[a]P equivalent PAH exposure in heating period rather than non-heating period has been reported in young children of New York city (Jung et al. 2010).
Occupancy probability and daily exposure level in children
This section describes a simplified PAH inhalation exposure risk model for children in the study area by using a quantitative risk assessment method for PAHs based on B[a]Peq concentrations. Occupancy probability (OP), as described by Chen and Liao (2006) for estimating daily PAHs exposure in a particular setting for infants, children, and adults of Taiwan, was used to quantify the likelihood of the time spent by children in a specific setting during a day. Briefly, the 24-h period was divided into five time intervals as j = 1 (spent in home), 2 (spent in commuting), 3 (spent in school), 4 (spent in commuting), and 5 (spent in home) representing the time periods from 00:00 to 07:00, 07:00–09:00, 09:00–17:00, 17:00–19:00, and 19:00–24:00, respectively. Daily exposure level through inhalation (E I) for children can be calculated as summation of daily exposure levels in various settings (j = 1, 2, 3, 4, and 5) in which children spent the entire day. The formula takes into account the B[a]Peq concentrations associated with an age-specific inhalation rate in a particular setting and occupancy time. It can thus be represented as follows:
where E I is the daily exposure level for inhalation, a = 1.5 is the average PAHs indoor/outdoor (I/O) ratio, OP j is the OP value in the jth time interval (j = 1, 2, 3, 4, and 5), C is the mean B[a]Peq concentration (ng m−3), OT j is the occupancy time for the jth time interval (h), and IR j is the inhalation rate in the jth time interval (m3 day−1) when j = 1 and 5 are the resting activity and j = 2, 3, and 4 are the active activity. Due to the absence of measured indoor PAH concentrations in the present study, we considered I/O ratios from published literature. It was found that PAH I/O ratios vary considerably between geographical locations with reported values of 0.9 for Boston, USA (Levy et al. 2003); 1.2 for Taipei, Taiwan (Li and Ro 2000); 1.3 for Hangzhou, China (Liu et al. 2001); 1.9 for Houston, USA (Naumova et al. 2002); and 3.4 for Los Angeles, USA (Naumova et al. 2002). In order to ensure that the chosen PAH I/O ratio is representative of the present study, we decided to adopt the value of 1.5 reported by Masih et al. (2010, 2012) from Agra, a city at a distance of ∼250 km from Delhi in northern India with a similar climate and PAH emission pattern as Delhi.
The daily inhalation exposure level in children during school hours, commuting time, and resting time in home was calculated to be 283.93 ng day−1 (Table 4). The inhalation exposure levels during school hours was the highest followed by the same during commuting from school to home. The least exposure was found during resting time in home. The trend is explained by greater susceptibility of children to combustion-related pollutants because they spend more time outside, where combustion sources are generally higher (Schwartz 2004). Interestingly, since India is a tropical country, most of the buildings are naturally ventilated and their air exchange rates are higher than heating, ventilation, and air conditioning (HVAC)-equipped buildings, resulting in a significant impact of outdoor air on indoor air quality (Kolokotroni et al. 2002). In the apparent absence of any indoor PAH sources, outdoor concentrations and in turn air exchange rates (that are specific for infiltration and natural ventilation pathways) play a key role in assessing PAH exposure. In this context, Zhou and Zhao (2012) reported a median contribution of 78 % by outdoor sources to indoor PAH exposure in the overall population of Beijing, China. Moreover, Goyal and Khare (2011) have concluded that a higher indoor level of PM10 in a school located near high traffic roads in Delhi is due to prevalent natural ventilation in Indian schools/buildings and higher ambient outdoor concentrations. They also attributed the high concentration of indoor PM10 to occupant activities in the school premises. A school environment is marked by intense indoor activities and movements of school children resulting into resuspension or delayed deposition of PM10. Similarly, mechanical and aero-electrical forces from human activities have been shown to incur significant resuspension in indoor environment (Hu et al. 2007). Furthermore, a study by Chithra and Shiva Nagendra (2012) indicated that school location and building ventilation rate in Indian schools as well as other factors such as high population density in schools, unlike offices and homes, and the associated indoor classroom activities are reasons for poor indoor air quality in a school in Chennai, India. However, it is important to quantify the air exchange rates and have extensive information on ventilation rates in Indian schools, having predominant natural ventilation.
Commuting to and from the school has been correlated with not only a rush hour traffic peak but also a generally lower mixing height that inhibits pollution dispersion in the morning hours leading to highest exposures to traffic-related air pollution during the morning walk/ride to school (Spira-Cohen et al. 2010). Studies have also shown appreciable association of traffic-generated particulate matter air pollution and both personal and indoor pollutant exposure (Koistinen et al. 2001; Lanki et al. 2007; Baxter et al. 2007). In this context, the present study has apportioned vehicular emissions as the major source of observed PAH levels. Interestingly, vehicular source contributions exhibited excellent association (r = 0.94, p < 0.001) with daily inhalation exposure levels in school children. This strongly supports our previous hypothesis that vehicular emission is the chief source of PAHs inhalation exposure for school children. The two ring roads in Delhi, outer ring road (ORR) and ring road (RR), carrying heavy traffic lie in close proximity to the study site. Portions of these roads adjacent to the study site have a number of congested intersections due to very heavy traffic on ORR and RR (Delhi Govt. 2012). The disproportionate growth of vehicles and available road network has resulted in heavy traffic congestions in Delhi in recent years (Planning Department 2008). Also, Kumar and Tyagi (2006) have reported that markers of vehicular emissions such as benzene and toluene register high concentrations in the morning and evening peak periods than the noon lean period in Delhi.
Foster and Kumar (2011) have observed that although radical interventions to improve the air quality of Delhi resulted in considerable improvements in respiratory health of its citizens, the effect varied significantly by gender, income, and, most importantly, from a causal point of view among those individuals who spend a disproportionate share of their time out-of-doors, such as school children. However, it is important to note that health hazards posed by various sources such as vehicular pollution are born disproportionately by children attending certain schools based on the location of the school (Rickwood and Knight 2009).
Seasonal differences in daily exposure level of total PAHs in school children were characterized by extremely high exposure levels during winters (439.43 ng day−1) followed by monsoon (232.59 ng day−1) and summer (171.08 ng day−1). However, the contribution during school hours, commuting time, and resting time in home to daily exposure level exhibited the same trend in all the seasons as exhibited in the mean annual levels. Similar highest winter time exposure levels to PM10, PM2.5, and PM1 in school children have been reported by Goyal and Khare (2011). Chithra and Shiva Nagendra (2012) also reported poorest indoor air quality during winter in classrooms.
Incremental lifetime cancer risk (ILCR) for inhalation in children
ILCR was calculated for the school children of Delhi with the observed mean daily exposure level of all the seasons (283.93 ng day−1).
ILCR for inhalation exposure in children was calculated as follows:
where R is the incremental individual lifetime cancer risk, E I is the daily exposure level for inhalation (ng day−1), CSF is the inhalation carcinogenic slope factor (mg kg−1 day−1)−1, EF is the exposure frequency (day year−1), ED is the exposure duration (14 years for children), AT is the averaging time for carcinogens (day), BW is the body weight (kg), and CF is the conversion factor (10−6) (Chen and Liao 2006).
The estimated ILCR for inhalation exposure of PAHs in school-going children was found to be 3.18 × 10−6. ILCR between 10−6 and 10−4 indicates potential risk. The value of ILCR in the present study fell within the range of 10−6–10−5, being higher than the acceptable risk level (10−6) and lower than the priority risk level (10−4). This risk transforms into ∼11 extra cancer cases every year in school children of Delhi (calculated by multiplying individual ICRs by Delhi’s school children population, i.e., 3.5 million; Planning Department 2011) due to inhalation exposure to the observed PAHs concentrations during childhood days. The estimate is considered to be conservative because, apart from inhalation, dermal and ingestion routes are also potential pathways for exposure and consequent risk in children during childhood days. Further, if the observed concentrations of PAHs are inhaled by 17 million people of Delhi for lifetime (70 years), then for a unit risk of 8.7 × 10−5 (ng m−3)−1 (WHO 2000), an estimated 652 excess cancer cases due to inhalation exposure of PAHs was calculated as follows:Annual number of cancer cases(persons per million) = (Unit risk * sum B[a]P * Resident(million)/(Life expectancy) (Pengchai et al. 2009).
Conclusions
High levels of PM10 and associated PAHs were observed at a school site located in close proximity of industrial areas and high traffic roads. Significantly higher wintertime PAHs was observed. Source apportionment of PAHs indicated vehicular (59.5 %) and coal combustion (40.5 %). Atmospheric distribution of total PAHs were heavily influenced by the carcinogenic species and the B[a]P equivalent concentrations, through both TEF and MEF approach, exhibited highest exposure risks during winter. Extremely high daily inhalation exposure was observed during winter > monsoon > summer. Furthermore, exposure during school hours was the highest in all the seasons followed by same during commuting to school and least during resting period. Also, good correlation (r = 0.94, p < 0.001) between daily vehicular source contributions to PAHs levels and daily PAHs inhalation exposure levels in school children pointed towards the risks imposed by vehicular emissions to school children in commuting to and from the school. A conservative estimate of ∼11 excess cancer cases in children during childhood and ∼652 cases for a lifetime inhalation exposure of PAHs at the observed concentration have been calculated in Delhi.
References
Akyüz M, Çabuk H (2009) Meteorological variations of PM2.5/PM10 concentrations and particle-associated polycyclic aromatic hydrocarbons in the atmospheric environment of Zonguldak, Turkey. J Hazard Mater 170:13–21
Anderson LM, Diwan BA, Fear NT, Roman E (2000) Critical windows of exposure for children’s health: cancer in human epidemiological studies and neoplasms in experimental animal models. Environ Health Perspect 108:573–594
American Thoracic Society (1996) Health effects of outdoor air pollution. Part 2. Committee of the Environmental and Occupational Health Assembly of the American Thoracic Society. Am J Respir Crit Care Med 153:477–498
Baxter LK, Clougherty JE, Laden F, Levy JI (2007) Predictors of concentrations of nitrogen dioxide, particulate matter, and particle constituents inside of lower socioeconomic status urban homes. J Expo Sci Environ Epidemiol 17:433–444
Beckerman B, Jerrett M, Brook JR, Verma DK, Arain MA, Finkelstein MM (2008) Correlation of nitrogen dioxide with other traffic pollutants near a major expressway. Atmos Environ 42:275–290
Bonn PJA, Driscoll K (1996) Particles, inflammation and respiratory tract carcinogenesis. Toxicol Lett 88:109–113
Caricchia AM, Chiavarini S, Pezza M (1999) Polycyclic aromatic hydrocarbons in the urban atmospheric particulate matter in the city of Naples (Italy). Atmos Environ 33:3731–3738
Census of India (2011) Government of India, Office of the Registrar General and Census Commissioner, New Delhi
Chen SC, Liao CM (2006) Health risk assessment on human exposed to environmental polycyclic aromatic hydrocarbons pollution sources. Sci Total Environ 366:112–123
Chithra VS, Shiva Nagendra SM (2012) Indoor air quality investigations in a naturally ventilated school building located close to an urban roadway in Chennai, India. Build Environ 54:159–167
Dejmek J, Solansky I, Beneš I, Leníček J, Šrám RJ (2000) The impact of polycyclic aromatic hydrocarbons and fine particles on pregnancy outcome. Environ Health Perspect 108:1159–1164
Delgado-Saborit JM, Aquilina N, Baker S, Harrad S, Meddings C, Harrison RM (2010) Determination of atmospheric particulate-phase polycyclic aromatic hydrocarbons from low volume air samples. Anal Methods 2:231–242
Delhi Govt. (2012) http://delhi.gov.in/wps/wcm/connect/fac089804f846492b2e0b2132654ee81/59+−+79+Transport+.pdf?MOD=AJPERES
DeMarini D, Brooks L, Warren S, Kobayashi T, Gilmour M, Singh P (2004) Bioassay-directed fractionation and salmonella mutagenicity of automobile and forklift diesel exhaust particles. Environ Health Perspect 112:814–819
Dickhut RM, Canuel EA, Gustafson KE, Liu K, Arzayus KM, Walker SE, Edgecombe G, Gaylor MO, MacDonald EH (2000) Automotive sources of carcinogenic polycyclic aromatic hydrocarbons associated with particulate matter in Chesapeake Bay region. Environ Sci Technol 34:4635–4640
Dockery DW, Skerrett PJ, Walters D, Gilliland F (2005) Development of lung function. In: Effects of air pollution on children’s health and development: a review of the evidence. World Health Organization special programme on health and environment. European Centre for Environment and Health, Bonn, pp 108–133
Durant J, Busby W, Lafleur A, Penman B, Crespi C (1996) Human cell mutagenicity of oxygenated, nitrated and unsubstituted polycyclic aromatic hydrocarbons associated with urban aerosols. Mutat Res 371:123–157
Durant J, Lafleur A, Busby W, Donhoffner L, Penman B, Crespi C (1999) Mutagenicity of C24H14 PAH in human cells expressing CYP1A1. Mutat Res Genet Toxicol Environ Mutagen 446:1–14
Finlayson-Pitts BJ, Pitts JN Jr (2000) Chemistry of upper and lower atmosphere: theory, experiments and applications. Academic, San Diego
Foster A, Kumar N (2011) Health effects of air quality regulations in Delhi, India. Atmos Environ 45:1675–1683
Ganguly D, Jayaraman A, Rajesh TA, Gadhavi H (2006) Wintertime aerosol properties during foggy and non-foggy days over urban center Delhi and their implications for shortwave radiative forcing. J Geophys Res 111, D15217
Goyal R, Khare M (2011) Indoor air quality modelling for PM10, PM2.5, and PM1.0 in naturally ventilated classrooms of an urban Indian school building. Environ Monit Assess 176:501–516
Guo H, Lee SC, Ho KF, Wang XM, Zou SC (2003) Particle-associated polycyclic aromatic hydrocarbons in urban air of Hong Kong. Atmos Environ 37:5307–5317
Gurjar BR, Jain A, Sharma A, Agarwal A, Gupta P, Nagpure AS, Lelieveld J (2010) Human health risks in megacities due to air pollution. Atmos Environ 44:4606–4613
Hoek G, Pattenden S, Willers S, Antova T, Fabianova E, Braun-Fahrländer C, Forastiere F, Gehring U, Luttmann-Gibson H, Grize L, Heinrich J, Houthuijs D, Janssen N, Katsnelson B, Kosheleva A, Moshammer H, Neuberger M, Privalova L, Rudnai P, Speizer F, Slachtova H, Tomaskova H, Zlotkowska R, Fletcher T (2012) PM10 and children's respiratory symptoms and lung function in the PATY study. Eur Respir J 40:538–547
Hu B, Freihaut J, Bahnfleth W, Aumpansub P, Thran B (2007) Modeling the influence of human activity on particle resuspension and dispersion in a multizone indoor environment with HVAC system. J Archit Eng 13:187–193
Jung KH, Yan B, Chillrud SN, Perera FP, Whyatt R, Camann D, Kinney PL, Miller RL (2010) Assessment of benzo(a)pyrene equivalent carcinogenicity and mutagenicity of residential indoor versus outdoor polycyclic aromatic hydrocarbons exposing young children in New York City. Int J Environ Res Public Health 7:1889–1900
Khalili NR, Scheff PA, Holsen TM (1995) PAH source fingerprints for coke ovens, diesel and gasoline engines, highway tunnels, and wood combustion emissions. Atmos Environ 29:533–542
Khillare PS, Sarkar S (2012) Airborne inhalable metals in residential areas of Delhi, India: distribution, source apportionment and health risks. Atmos Pol Res 3:46–54
Koistinen KJ, Hanninen O, Rotko T, Edwards RD, Moschandreas D, Jantunen MJ (2001) Behavioral and environmental determinants of personal exposures to PM2.5 in EXPOLIS—Helsinki, Finland. Atmos Environ 35:2473–2481
Kolokotroni M, Ge YT, Katsoulas D (2002) Monitoring and modelling indoor air quality and ventilation in classrooms within a purpose-designed naturally ventilated school. Indoor Built Environ 11:316–326
Kumar A, Tyagi SK (2006) Benzene and toluene profiles in ambient air of Delhi as determined by active sampling and GC analysis. J Sci Ind Res India 65:252–257
Lanki T, Ahokas A, Alm S, Janssen NAH, Hoek G, De Hartog JJ, Brunekreef B, Pekkanen J (2007) Determinants of personal and indoor PM2.5 and absorbance among elderly subjects with coronary heart disease. J Expo Sci Environ Epidemiol 17:124–133
Larsen RK, Baker JE (2003) Source apportionment of polycyclic aromatic hydrocarbons in the urban atmosphere: a comparison of three methods. Environ Sci Technol 37:1873–1881
Lee RGM, Jones KC (1999) The influence of meteorology and air masses on daily atmospheric PCB and PAH concentrations at a UK location. Environ Sci Technol 33:705–712
Levy JI, Bennett DH, Melly SJ, Spengler JD (2003) Influence of traffic patterns on particulate matter and polycyclic aromatic hydrocarbon concentrations in Roxbury, Massachusetts. J Expo Sci Environ Epidemiol 13:364–371
Li CS, Ro YS (2000) Indoor characteristics of polycyclic aromatic hydrocarbons in the urban atmosphere of Taipei. Atmos Environ 34:611–620
Li J, Zhang G, Li XD, Qi SH, Liu GQ, Peng XZ (2006) Source seasonality of polycyclic aromatic hydrocarbons (PAHs) in a subtropical city, Guangzhou, South China. Sci Total Environ 355:145–155
Lim SS, Vos T, Flaxman AD, Danaei G et al (2012) A comparative risk assessment of burden of disease and injury attributable to 67 risk factors and risk factor clusters in 21 regions, 1990–2010: a systematic analysis for the Global Burden of Disease Study 2010. Lancet 380:2224–2260
Liu Y, Zhu L, Shen X (2001) Polycyclic aromatic hydrocarbons (PAHs) in indoor and outdoor air of Hangzhou, China. Environ Sci Technol 35:840–844
Ludykar D, Westerholm R, Almén J (1999) Cold start emissions at +22, −7 and −20°C ambient temperatures from a three-way catalyst (TWC) car: regulated and unregulated exhaust components. Sci Total Environ 235:65–69
Masih J, Masih A, Kulshrestha A, Singhvi R, Taneja A (2010) Characteristics of polycyclic aromatic hydrocarbons in indoor and outdoor atmosphere in the North central part of India. J Hazard Mater 177:190–198
Masih J, Singhvi R, Kumar K, Jain VK, Taneja A (2012) Seasonal variation and sources of polycyclic aromatic hydrocarbons (PAHs) in indoor and outdoor air in a semi arid tract of Northern India. Aerosol Air Qual Res 12:515–525
Menichini E, Monfredini F, Merli F (1999) The temporal variability of the profile of carcinogenic polycyclic aromatic hydrocarbons in urban air: a study in a medium traffic area in Rome, 1993–1998. Atmos Environ 33:3739–3750
MoEF (2009) Environment (Protection) Seventh Amendment Rules. Ministry of Environment and Forests. Government of India Press, New Delhi
Hernández S, Mugica V, Torres M, García R (2010) Seasonal variation of polycyclic aromatic hydrocarbons exposure levels in Mexico City. J Air Waste Manage Assoc 60:548–555
Naumova YY, Eisenreich SJ, Turpin BJ, Weisel CP, Morandi MT, Colome SD, Totten LA, Stock TH, Winer AM, Alimokhtari S, Kwon J, Shendell D, Jones J, Maberti S, Wall SJ (2002) Polycyclic aromatic hydrocarbons in the indoor and outdoor air of three cities in the U.S. Environ Sci Technol 36:2552–2559
Nielsen T, Jorgensen H, Larsen J, Poulsen M (1996) City air pollution of polycyclic aromatic hydrocarbons and other mutagens: occurrence, sources and health effects. Sci Total Environ 189–190:41–49
Nisbet ICT, LaGoy PK (1992) Toxic equivalency factors (TEFs) for polycyclic aromatic hydrocarbons (PAHs). Regul Toxicol Pharm 16:290–300
Norramit P, Cheevaporn V, Itoh N, Tanaka K (2005) Characterization and carcinogenic risk assessment of polycyclic aromatic hydrocarbons in the respirable fraction of airborne particles in the Bangkok metropolitan area. J Health Sci 51:437–446
Northridge ME, Yankura J, Kinney PL, Santella RM, Shepard P, Riojas Y, Agarwal M, Stickland P (1999) Diesel exhaust exposure among adolescents in Harlem: a community-driven study. Am J Public Health 89:998–1002
Pandey JS, Rakesh K, Devotta S (2005) Health risks of NO2, SPM and SO2 in Delhi (India). Atmos Environ 39:6868–6874
Park JS, Wade TL, Sweet ST (2002) Atmospheric deposition of PAHs, PCBs, and organochlorine pesticides to Corpus Christi Bay, Texas. Atmos Environ 36:1707–1720
Pengchai P, Chantara S, Sopajaree K, Wangkarn S, Tengcharoenkul U, Rayanakorn M (2009) Seasonal variation, risk assessment and source estimation of PM10 and PM10-bound PAHs in the ambient air of Chiang Mai and Lamphun, Thailand. Environ Monit Assess 154:197–218
Perera FP (1997) Environment and cancer: who are susceptible? Science 278:1068–1073
PereraFP RV, Whyatt RM, Tsai WY, Tang D, Diaz D, Hoepner L, Barr D, Tu YH, Camann D, Kinney P (2006) Effect of prenatal exposure to airborne polycyclic aromatic hydrocarbons on neurodevelopment in the first 3 years of life among inner-city children. Environ Health Perspect 114:1287–1292
Planning Department (2008) Economic survey of Delhi 2007–2008. Government of NCT of Delhi, Delhi
Planning Department (2011) Economic survey of Delhi 2010–2011. Government of NCT of Delhi, Delhi
Quiteiro S, Arbilla G, Bauerfeld G, Moreira J (2007) Polycyclic aromatic hydrocarbons and their molecular diagnostic ratios in airborne particles PM10 collected in Rio de Janeiro, Brazil. Water Air Soil Pollut 179:79–92
Rajarathnam U, Seghal M, Nairy S, Patnayak RC, Chhabra SK, Kilnani KV, Ragavan S (2011) Time-series study on air pollution and mortality in Delhi. In: Public health and air pollution in Asia (PAPA): coordinated studies of short-term exposure to air pollution and daily mortality in two Indian cities. Research Report 157. Health Effects Institute, Boston, MA
Rajput N, Lakhani A (2010) Measurements of polycyclic aromatic hydrocarbons in an urban atmosphere of Agra, India. Atmosfera 23:165–183
Reponen T, Grinshpun SA, Trakumas S, Martuzevicius D, Wang ZM, LeMasters G, Lockey JE, Biswas P (2003) Concentration gradient patterns of aerosol particles near interstate highways in the Greater Cincinnati airshed. J Environ Monit 5:557–562
Rickwood P, Knight D (2009) The health impacts of local traffic pollution on primary school age children. State of Australian Cities 2009 conference proceedings
Saarnio K, Sillanpää M, Hillamo R, Sandell E, Pennanen AS, Salonen RO (2008) Polycyclic aromatic hydrocarbons in size-segregated particulate matter from six urban sites in Europe. Atmos Environ 42:9087–9097
Sahu SK, Beig G, Parkhi NS (2011) Emissions inventory of anthropogenic PM2.5 and PM10 in Delhi during commonwealth games 2010. Atmos Environ 45:6180–6190
Sarkar S, Khillare PS, Jyethi DS, Hasan A, Parween M (2010) Chemical speciation of respirable suspended particulate matter during a major firework festival in India. J Hazard Mater 184:321–330
Schwartz J (2004) Air pollution and children's health. Paediatrics 113:1037–1043
Sequeira J (2008) A toxic issue: air pollution in New Delhi. Harv Int Rev 30:3
Shi GL, Feng YC, Wu JH, Li X, Wang YQ, Xue YH, Zhu T (2009) Source identification of polycyclic aromatic hydrocarbons in urban particulate matter of Tangshan, China. Aerosol Air Qual Res 3:309–315
Simcik MF, Eisenreich SJ, Lioy PJ (1999) Source apportionment and source/sink relationship of PAHs in the coastal atmosphere of Chicago and Lake Michigan. Atmos Environ 33:5071–5079
Spira-Cohen A, Chen LC, Kendall M, Sheesley R, Thurston GD (2010) Personal exposures to traffic-related particle pollution among children with asthma in the South Bronx, NY. J Expo Sci Environ Epidemiol 20:446–456
Tan JH, Bi XH, Duan JC, Rahn KA, Sheng GY, Fu JM (2006) Seasonal variation of particulate polycyclic aromatic hydrocarbons associated with PM10 in Guangzhou, China. Atmos Res 80:250–262
Tuntawiroon J, Mahidol C, Navasumrit P, Autrup H, Ruchirawat M (2007) Increased health risk in Bangkok children exposed to polycyclic aromatic hydrocarbons from traffic-related sources. Carcinogenesis 28:816–822
USEPA (U.S. Environmental Protection Agency) (2010) Development of a relative potency factor (RPF) approach for polycyclic aromatic hydrocarbon (PAH) mixtures (external review draft). EPA/635/R-08/012A. USEPA, Washington DC
Wada M, Kido H, Kishikawa N, Tou T, Tanaka M, Tsubokura J, Shironita M, Matsui M, Kuroda N, Nakashima K (2001) Assessment of air pollution in Nagasaki city: determination of polycyclic aromatic hydrocarbons and their nitrated derivatives and some metals. Environ Pollut 115:139–147
Wang X, Cheng H, Xu X, Zhuang G, Zhao C (2008) A wintertime study of polycyclic aromatic hydrocarbons in PM2.5 and PM2.5–10 in Beijing: assessment of energy structure conversion. J Hazard Mater 157:47–56
World Health Organization (2000) Air Quality Guidelines for Europe, 2nd ed., WHO, Regional Office for Europe, Copenhagen
Xue WL, Warshawsky D (2005) Metabolic activation of polycyclic and heterocyclic aromatic hydrocarbons and DNA damage: a review. Toxicol Appl Pharmacol 206:73–93
Yassaa N, Meklati BY, Cecinato A, Marino F (2001) Particulate n-alkanes, n-alkanoic acids and polycyclic aromatic hydrocarbons in the atmosphere of Algiers City Area. Atmos Environ 35:843–1851
Yunker MB, MacDonald RW, Vingarzan R, Mitchell RH, Goyette D, Sylvestre S (2002) PAHs in the Fraser River basin: a critical appraisal of PAH ratios as indicators of PAH source and composition. Org Geochem 33:489–515
Zeiger E (2001) Mutagens that are not carcinogens: faulty theory or faulty tests? Mutat Res Genet Toxicol Environ Mutagen 492:29–38
Zhang Y, Tao S (2009) Global atmospheric emission inventory of polycyclic aromatic hydrocarbons (PAHs) for 2004. Atmos Environ 43:812–819
Zhou B, Zhao B (2012) Population inhalation exposure to polycyclic aromatic hydrocarbons and associated lung cancer risk in Beijing region: contributions of indoor and outdoor sources and exposure. Atmos Environ 62:472–480
Zhou Y, Levy JI (2007) Factors influencing the spatial extent of mobile source air pollution impacts: a meta-analysis. BMC Publ Health 7:89
Acknowledgments
Fellowship awarded by Indian Council of Medical Research (ICMR) to DSJ is duly acknowledged. The permission given by Kulachi Hansraj Model School, New Delhi to use the school building for operating the air sampler is acknowledged. The authors are thankful to the anonymous reviewers for their constructive comments and suggestions.
Author information
Authors and Affiliations
Corresponding author
Additional information
Responsible editor: Gerhard Lammel
Rights and permissions
About this article
Cite this article
Jyethi, D.S., Khillare, P.S. & Sarkar, S. Risk assessment of inhalation exposure to polycyclic aromatic hydrocarbons in school children. Environ Sci Pollut Res 21, 366–378 (2014). https://doi.org/10.1007/s11356-013-1912-6
Received:
Accepted:
Published:
Issue Date:
DOI: https://doi.org/10.1007/s11356-013-1912-6