Abstract
To better understand the driving forces of changes in streamflow (Q), this study analyzed the changes in the hydro-meteorological series by the Mann-Kendall, Pettitt’s test and the flow duration curve (FDC) in the Wuding River Basin (WRB), which is a typical river basin in the Loess Plateau. The response of Q variability to climate change and human activities were also quantified by the elasticity method and decomposition method based on the Budyko framework. The results showed that Q exhibited an obvious downward trend at the rate of 0.44 mm/y with a changing point occurred in 1980. Compared with 1961–1980, the greatest reduction in monthly Q during 1981–2007 was found in April (41 %) and the low flows have more distinct decrease than high flows. The precipitation (P), potential evapotranspiration (E 0 ) and catchment characteristics parameter n elasticity of Q are 2.40, −1.40 and −2.51, respectively, indicating that Q variability is most sensitive to human activities. The contribution of climate change and human activities to changes in Q from the two methods are 35 and 65 %, respectively. The ecological restoration (ER) measures, including channel measures and slope measures, were found to be the dominant factors responsible for the decreased Q. Furthermore, changes in Q in 1970–1990 could be mainly ascribed to channel measures while slope measures have played more important roles after 1999 when the Grain-for-Green (GFG) project was implemented. This study could provide scientific basis for how to mitigate effectively and efficiently changes in water resources and guide measures to be implemented in the region under the future climate change.
Similar content being viewed by others
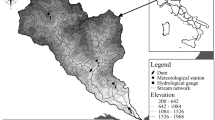
Avoid common mistakes on your manuscript.
1 Introduction
Water resources are widely regarded as the most essential natural assets for humans, wildlife and ecosystems (Oki and Kanae 2006). Presently, the majority of scientists and the international public consider the cause of water resources variability can be divided into two categories: climate change and human activities (Xu 2011; Van Ty et al. 2012; Gain and Wada 2014). Climate change includes the changes of precipitation (P) and potential evapotranspiration (E 0 ) while human activities mainly include ecological restoration (ER) measures, water diversion and water consumption (Zhao et al. 2014). Expedient water resources management strategies are essential when planning for water resource consumption, industrial and agricultural production, and ecosystem sustainability that rely heavily on surface and ground water. Programme for potential future changes in water resources requires an understanding about basin responsiveness-the response of water resources change to climate change and human activities (Donohue et al. 2011; Yang et al. 2012).
Numerous methods have been developed for assessing the impacts of climate change and human activities on streamflow (Q) variability, such as those by using physical based hydrological models (Zhang et al. 2008b, 2012a; Tang et al. 2014). However, hydrological models are always limited due to the fundamental challenges in calibration and validation, requirement of high resolution datasets and uncertainty in model structure and parameter estimation (Wang et al. 2013). Alternatively, methods that use simple hydro-meteorological data and interpolators of an appropriate form based on simple theory, such as the Budyko hypothesis (Budyko 1974), have provided a more general approach to attribute changes in Q in a more transparent way (Dooge et al. 1999; Choudhury 1999; Arora 2002; Yang et al. 2008; Roderick and Farquhar 2011; Donohue et al. 2011). Based on the Budyko framework, Xu et al. (2014) recently reported an attribution analysis in 33 selected mountainous basins in the Haihe River Basin using an elasticity method, which calculates the sensitivity and contributions of P, E 0 changes and human activities on Q variability independently. Besides, the decomposition method for attribution of Q variability proposed recently by Wang and Hejazi (2011) is also based on the Budyko framework. Comparison studies have shown that these simple methods based on the Budyko framework could perform as good as the more complex hydrological models (Zuo et al. 2014; Zhan et al. 2014), suggesting the robustness and effectiveness of the Budyko framework-derived methods in hydrological analysis.
Wuding River is a typical river in the Loess Plateau, where is characterized with a high sediment loading. Changes in Q and sediment discharge have exerted a great influence on the hydrological cycle in the Yellow River Basin (Zhang et al. 2002). To prevent soil erosion, Chinese government has implemented a series of ER measures in the basin since 1950s (Xu 2004; She et al. 2014), including channel measures such as building check-dams and slope measures such as building terraces, afforestation and planting pastures. These types of measures are commonly used around the world so as to control erosion and sediment transport (Myronidis et al. 2010). In 1999, a large reforestation campaign — the Grain-for-Green (GFG) project, which can be regarded as one of the slope measures, was implemented by converting farmland to forest and grassland mainly in areas where slopes exceed 15° (Zhang et al. 2012b). Although the effect of these ER measures on regional vegetation recovery has been shown positive (Cao et al. 2009), it is still unclear how, and to what extent, the intensive intervention has influenced the water resources in the region.
Previous studies have reported a dramatic decreasing trend of Q in the Wuding River Basin (WRB) during recent years. Although by no mean universal, it is generally believed that climate change coupled with ER measures is the major reason for the reduction of Q. For example, Li et al. (2007) reported that ER measures accounts for 87 % of the total reduction in mean annual Q in the period of 1972 to 1997 by using sensitivity-based method and rainfall–runoff model in the WRB. Zhang et al. (2008a) concluded that ER measures accounted for 57 % of Q reduction by applying the sensitivity-based method. Xu (2011) adopted multiple regression analysis to discuss the contributions of water diversion (37.5 %) and ER measures (11.8 %) to the reduction of water resources in this basin. As can be seen, different conclusions could be mainly due to the different study periods and methods being used. Xu et al. (2014) stated that attributing the remaining effect (apart from climate change) to human activities would overestimate the impact of human activities on changes in Q. Therefore, there is a need to independently determine the contribution of climate change and human activities to Q variability. Furthermore, the key issue of water resources management and ecological construction is to understand which factors (channel measures or slope measures) is the major force to changes in Q in the basin. Huang et al. (2003) evaluated Q responses to afforestation in a watershed on the Loess Plateau with an area of 1.15 km2 using a paired watershed approach. Zhang et al. (2014) quantified the effect of individual ER measures on Q and stated that terraced and check-dam present a much larger effect of reducing Q than sloped lands. However, these studies mainly focus on the small watershed based on observed data and it is unclear whether these results can be generalized to large-scale basins, which involves complicated interactions among soil, vegetation and climate. Zhang et al. (2008a) suggested that the ER measures have become important influencing factors on Q variability in the Loess Plateau, supporting the results by Zhao et al. (2014). Nevertheless, detailed information about channel measures (e.g., the number, types and timing of check-dams) is unavailable, making the effect of each ER measure unclear. In addition, few studies focused on the comprehensive effect of channel measures and slope measures on changes in Q at the monthly and daily time scale, which is very important to assess the effectiveness of ecological constructions, especially after the launch of the GFG project, which has resulted in considerable increases in vegetation coverage.
The aims of this study, therefore, are: (1) to detect statistically variability trends in meteorological and hydrological series and the changing point in annual Q; (2) to examine daily Q change by contrasting the shape of the flow duration curve (FDC) and mean monthly Q in pre-change period with post-change period; (3) to explore the sensitivity and contributions of climate change and human activities to Q variability by the elasticity method and the decomposition method based on the Budyko framework; and (4) to analyse the spatial and temporal effects of ER measures on Q variability by using detailed document information about ER measures.
2 Study Area and Data
2.1 Study Area
The WRB, draining an area of ~30,261 km2, is located in the northern part of the Loess Plateau and is a tributary of the Yellow River (Fig. 1). The outlet hydrometric station is the Baijiachuan station, above which the drainage area is ~29,662 km2. The area-averaged mean annual precipitation over the basin is about 400 mm and 75 % of the precipitation falls between June and September. The sandy region located in the northwestern part of the basin that covers 54.3 % of the total basin area and the southeastern part is a hilly, gullied area covered by loess and is the major water yield region (Xu 2011). Due to adverse natural conditions (e.g., thick and highly erodible loess, weak vegetation cover, relatively high intensity of rainstorms and anabatic human activities (e.g., steep-slopeland cultivation, fell trees and overgrazing), its soil erosion rate is the highest in the Loess Plateau (Shi et al. 2013).
2.2 Data
The Q data including daily Q from 1961 to 2007 and annual Q from 1961 to 2009, and sediment data from 1961 to 2009 in Baijiachuan hydrological station were obtained from the Water Resources Committee of the Yellow River Conservancy Commission. Meteorological data including daily precipitation, air temperature, sunshine hours, relative humidity and wind speed were obtained from the National Climatic Centre of the China Meteorological Administration. Daily E 0 was calculated using the Penman model (Penman 1948), and then accumulated to annual totals. All the meteorological data were spatially averaged across the study area. The ER measures data from 1960 to 2006 of the WRB used in the present study were from Zhang et al. (2002) and Yao et al. (2011). The information on numbers, types and timing of check-dams was obtained from the Soil and Water Conservation of the Shaanxi Provincial Bureau. Crop yield data from 1990 to 2010 were provided by Water Statistical Year Book of Shaanxi. The semi-monthly normalized difference vegetation index (NDVI) data (8 km spatial resolution) over 1982–2009 was obtained from the Global Inventory Modeling and Mapping Studies (Tucker et al. 2005). The maximum value composite method was used to reduce the noise in NDVI data and to generate monthly NDVI series (Yang et al. 2014). Regions with NDVI > 0.1 are considered as vegetated areas (Liang et al. 2015; Yang et al. 2015).
3 Methods
3.1 Trend Test and Break Point Analysis Method
The non-parametric Mann-Kendall test (Mann 1945; Kendall 1975) is a widely used method to detect trends in hydro-climatic series (Shadmani et al. 2012; Myronidis et al. 2012). In this study, it is applied to analyze change in trends of P, Q and E 0 . The Pettitt’s test (Pettitt 1979) is a rank-based and distribution-free test for detecting a significant change in the mean of a time series (Meddi et al. 2010). In this study, the Pettitt’s test was used to identify the changing point in the annual Q series and consequently divided the study period into pre-change period and post-change period.
3.2 Flow-Duration Curve
The flow-duration curve (FDC) (Vogel and Fennessey 1994) was plotted here to examine the relationship between the magnitude and frequency of Q such that to analyze the differences between Q in the same time with different frequency. Smakhtin (1999) suggested that the basin size, terrain characteristic, land use/cover and precipitation pattern can affect the shape of a FDC. Therefore, the FDC provides a graphical view that can reflect the Q characteristics of the complete range of Q and Q variability under changed land cover conditions over a time interval of interest by considering changes in percentile flows. Each value of Q has a corresponding exceedance probability p, and an FDC is simply a plot of Q p , the pth quantile or percentile of Q, versus exceedance probability p, where p is defined by
where the quantile Q p is a function of the observed Q, and it is often termed as the empirical quantile function (Vogel and Fennessey 1994). Usually, Q 5 (Q 95 ) indicates high flow (low flow) that flows exceed 5 % (exceed 95 %) and Q 50 is the median flow.
3.3 Attributing the Impact of Climate Change and Human Activities on Q Variability
3.3.1 Budyko Hypothesis
Budyko hypothesis (Budyko 1974) stated that the annual water balance can be expressed as a function of available water and energy. Among various forms of solutions to the Budyko hypothesis, the Mezentsev-Choudhury-Yang equation has been used most often and is also used here (Mezentsev 1955; Choudhury 1999; Yang et al. 2008).
where E, P, and E 0 are the mean annual actual evaporation, precipitation and potential evapotranspiration, respectively. The parameter n encodes the catchment characteristics which are principally connected with soil type, topography and vegetation (Yang et al. 2008). In this study, therefore, we assumed that changes in n over periods are mainly caused by human activities and can represent the impacts of human activities on Q change. Given P, Q and E 0 , we estimated the parameter n by modelling E (Eq. (2)) and at the same time minimizing the difference between modelled E and E estimated from catchment water balance while neglecting the change in soil water storage.
We assumed that the total changes in Q (∆Q) could be partitioned into those caused by climate change (∆Q c = ∆Q P (precipitation induced) + ∆Q E0 (potential evapotranspiration induced), and by human activities (∆Q h ). ∆Q was calculated as the difference between mean annual Q for the pre-change period that during the post-change period.
3.3.2 Elasticity Method
Schaake (1990) first proposed the concept of climate elasticity and then improved continually (e.g., Fu et al. 2007; Roderick and Farquhar 2011). The elasticity method determines the regression relationship between changes in Q and its influencing factors. Assuming P, E 0 and n in Eq. (2) are independent variables, the average annual Q can be expressed as Q = f(P, E 0,. n) and the total differential of Q can be written as (Xu et al. 2014):
Then based on the definition of elasticity \( \left({\varepsilon}_X=\frac{\partial Q/Q}{\partial X/X}\right) \), we can get the following form:
in which the elasticity of Q is given as:
where ε P , \( {\varepsilon}_{{\mathrm{E}}_0} \) and ε n represent the P, E 0 and catchment landscape elasticity of Q, respectively.
As a result, changes in Q caused by climate change (∆Q c ) and human activities (∆Q h ) can be calculated as:
3.3.3 Decomposition Method
Wang and Hejazi (2011) showed that the impact of climate change on Q can induce both horizontal and vertical components on Budyko curve, but direct human activities only can induce a vertical component on Budyko curve. Thus, the contribution of human activities to changes in Q can be calculated first:
where P 2 is the mean annual P during the post-change period, E′ 2 is the mean annual E, which can be calculated by the Eq. (2) using mean annual P and E 0 during the post-change period and the parameter n during the pre-change period. E 2 is computed by water balance equation using the average annual P and E 0 during the post-change period.
Then the climate change contribution to Q change can be calculated as:
4 Results
4.1 Changes in Hydro-climatic Series in the WRB
Figure 2 shows the variability of annual observed Q, P, and E 0 over the study period of 1961–2009. As mentioned above, the whole study period was split into two sub-periods (pre-change period (1961–1980) and post-change period (1981–2009)) based on the Pettitt’s test (Fig. 2d) on annual Q series. Annual Q showed a significant decreasing trend (0.44 mm/y, p < 0.01) during the entire period (Fig. 2a), whereas P and E 0 appeared to be more stationary (Fig. 2b and c and Table 1). Compared with the pre-change period, the relative Q, P and E 0 changes were about −29 −8 % and 2 % for the post-change period, respectively (Table 1).
4.2 Intra-Annual Variability of Q
As shown in Fig. 3, there have been considerable changes in mean monthly Q between the pre-change period and post-change period, with the percentage reduction being 9–41 %. The greatest reduction occurred in April (41 %) whereas the smallest reduction was found in June (9 %). Our results are different from those by Li et al. (2007), where that the absolute and relative reductions in Q were found greatest in July and August and smallest in the winter months in period 1972–1997 compared with 1961–1971 in the WRB. The discrepancy may result from different study period. Vegetation coverage has been largely increased since 1999 when the GFG project was implemented, resulting in alternations of the hydrological processes within the basin during growing season. This may lead to the higher Q changes in spring and autumn (Fig. 3). For both pre-change and post-change periods, Q peaked in March and August. Large Q occurred in early spring as a result of snowmelt and in flood-season due to high intensity rainfall events. In contrast, Q in both periods have low peak flows in winter due to less high intensity rainfall and Q is mainly consisted of baseflow (Li et al. 2007) and in the spring as a result of water being in need of vegetation growth.
4.3 Changes in Q Regime
Figure 4a shows the daily FDCs for the whole Q record in the pre-change period and post-change period. Compared with the pre-change period, it was evident that each part of FDC decreased in the post-change period. Especially for low flow Q 95 , it deceased by 60.04 %, which is more than twice of what high flow Q 5 did (Table 2). The trend of FDC in flood season is similar with that for the whole period, while it decreased relatively evenly in the non-flood season (Fig 4b and c). The rate of change of Q 5 , Q 50 and Q 95 is −25.95, −40.56 %, −82.69 % in flood season and −33.38, −27.12, −44.89 % in non-flood season, respectively (Table 2). In general, changes in Q in flood season were much more dramatic than that in non-flood season and the rate of change was reversed for the Q 5 case.
4.4 Effects of Climate Change and Human Activities on Q
The P and E 0 elasticity of Q is 2.40 and −1.40, respectively, suggesting that a 10 % increase in P (or E 0 ) would increase Q by 24 % (or decrease Q by 14 %). The parameter n elasticity of Q is −2.5, implying that a 10 % increase in n would decrease Q by 25 % (Table 3). It also indicates that Q is positively correlated with P but negatively correlated with E 0 and parameter n. In addition, changes in Q are most sensitive to human activities, followed by changes in P and E 0 in the WRB.
Table 3 also shows the relative contributions of climate change and human activities to changes in Q using the improved elasticity method and the decomposition method. The elasticity method reveals that climate change and human activities accounted for 32.80 and 68.85 % of changes in Q, respectively. Changes in P dominated the impact of climate change on Q (i.e., 33.76 %), whereas changes in E 0 exerted only a minor effect on changes in Q but with an opposite sign to the P effect (i.e., −0.96 %). Similar results were obtained by using the decomposition method, with the contributions of climate change and human activities to changes in Q being 36.68 and 63.62 %, respectively. Our results align well with previous studies (Li et al. 2007; Zhang et al. 2008a; Zhao et al. 2014) and further demonstrate the importance of human activities on hydrological cycle in this basin.
5 Discussion
5.1 Sensitivity of Q to P, E 0 and n
Due to the complex interaction between climate, human activities and hydrological processes, the response of Q is expected to vary with changes in P, E 0 and n, and presented a non-linear relationship (Yan et al. 2013). A large number of ER measures were implemented to control soil erosion and to improve vegetation coverage, resulting in large-scale landuse changes in the WRB. Consequently, Q showed the highest sensitive to human activities, followed by changes in P and E 0 . This result is similar to Donohue et al. (2011) in the Murray-Darling Basin in southeast Australia. However, Liang and Liu (2014) indicated that Q change was more sensitive to P, followed by catchment land surface conditions and E 0 in the Yellow River Basin, which is different from the findings in this study. This discrepancy might relate to differences in basin size, vegetation coverage, soil water holding capacity and topography analyzed in different studies.
5.2 Relative Effect of Channel Measures and Slope Measures on Q
Figure 5a was generated to further investigate the relationship between Q variability and ER measures. It was found that Q coefficient had a negative correlation with the percentage of total measured area in the basin (R 2 = 0.47 and p < 0.05). Some previous studies have focused on the influences of ER measures on Q variability (Cao et al. 2009; Shi et al. 2013), but these studies did not analyze the impacts of the individual measure on Q change. Zhang et al. (2014) stated that channel measures affect Q mainly through intercepting the overland flow, whereas slope measures affect primarily the water consumption and soil infiltration. Zhan et al. (2014) suggested that channel measures affect Q by reducing flood peaks and storing water within check-dams and reservoirs while the slope measures may have delayed these effects on Q because the plants take up water and accelerate its evapotranspiration with their growth. The effect of channel measures on Q variability is more direct and immediate (Huang and Zhang 2004), whereas the establishment of terraces may have a strong additional potential to lower water resources (Zhang et al. 2014).
The total number of check-dams peaked in 1970–1980 and presented a decreasing trend after 1980 (Fig. 5b), and the quantity of key dams (storage capacity is 0.5–5 million m3) had increased during 1960–1990 and started to decrease after 1990. In contrast, slope measures have significantly strengthened in the past 50 years. Although check-dams can reduce Q greatly, it would deposit in 5–10 years and change into farmland or be destroyed by flood, which would lose efficacy and have less effect on Q reduction (Xu 2011). A preliminary achievement has made in the implementation of GFG project after 1999 and vegetation coverage has increased significantly, particularly in the southeastern part of the WRB (Fig. 6). Therefore, it may lead to more infiltration and more water retained in the soil for evaporation (Zhang et al. 2008a). These changes caused the decrease of surface Q and deep drainage (Zhang et al. 2012b), making the amount of water into channel decreased. Hence, channel measures may mainly affect Q variability in 1970–1990 and slope measures have played more important roles in Q variability in the WRB after 1999.
5.3 Implication for Water Resources Management
At present, the implementations of channel and slope measures are managed by different departments. It is difficult to make policy comprehensively and hence may lead to many problems that cause changes in the hydrological cycle. To better manage water resources and guarantee production and livelihood, it is necessary to integrate different ER measures and conduct comprehensive assessment and management, especially under the current revegetation conditions.
Previous studies regarded the decreasing Q in the Yellow River Basin as a reduction of water resources. However, Xu (2012) indicated that the reduction of Q by these ER measures does not necessarily mean a “loss”, in that the green water, or the water consumption is reasonable utilization of water resources to enhance the vegetation and increase crop yield. Thus, the reduction of Q is compensated by the utilization of green-water resources. Harris et al. (2006) also considered that the implementation of these measures could contribute to the improvement of ecosystem services and adaptability to environmental change. Lü et al. (2012) pointed out that these measures were found to have resulted in enhancing soil conservation and carbon sequestration.
In the WRB, crop yield showed a gradual increasing trend with the enhanced total conservation area, from 0.32 million tons in 1990 to 0.81 million tons in 2010 (Fig. 7). The strengthened check-dam will accumulate field area, more than that, water input augmented by the ER measures produced an increase in crop productivity (Chen et al. 2007). Furthermore, sediment yield also decreased dramatically during the study period (Fig. 7) and it would lighten siltation in downstream, and diminish the danger from the aboveground river (Xu 2004). However, the interactions among water resources variability, climate change and human activities is complicated and remains a challenge for further studies.
5.4 Uncertainties
Uncertainties of this study are mainly raised from three aspect. Firstly, quantification of the impacts of climate change and human activities on Q variability is based on the assumption that human activities are independent of climate change, which may deviate from the fact that interactions are usually strong between the land and the climate system. Secondly, meteorological data used in this study is spatially averaged across the study area by using the ArcGIS Kriging interpolation method based on limited observations, which may potentially result in uncertainties in model inputs. Thirdly, water diversion is neglected due to the unavailable data. Ground-based observations are needed to further explore reciprocal relationship of climate change, human activities and hydrological change exclusively.
6 Conclusion
In this study, we chose a typical river basin in the Loess Plateau-the Wuding River Basin to analyze annual and inter-annual Q variability. The impacts of climate change and human activities on Q were quantified independently by the elasticity method and the decomposition method. Final conclusions are as follows:
-
(i)
Annual Q showed a significant declining trend during 1961–2009 with a changing point found in 1980. In contrast, neither P nor E 0 declined significantly during the same period. Compared with 1961–1980, there have been considerable changes in mean monthly Q during 1981–2007. Regime analysis showed that Q change more dramatically in flood season and the rate of change was reversed for the high flow.
-
(ii)
Q variability was most sensitive to changes in parameter n, followed by those in P and E 0 in the WRB. Climate change and human activities accounted for 32.80 and 68.85 % of the changes in Q based on the elasticity method, and these two numbers are 36.38 and 63.62 % from the decomposition method. Both methods suggest that the ER measures served as the dominant influencing factor on Q variability.
-
(iii)
Analysis of channel and slope measures variability showed that channel measures may mainly affect Q variability in 1970–1990 whereas slope measures have played more important roles in Q variability after 1999. The implementation of ER measures has benefited the local ecosystem and socioeconomic by improving the vegetation cover and crop yield and reducing soil erosion in the region. More efforts are needed to understand the integrated effect of each ER measure on water resources and ecosystem in the region.
References
Arora VK (2002) The use of the aridity index to assess climate change effect on annual runoff. J Hydrol 265(1):164–177
Budyko MI (1974) Climate and life. Academic, New York, p 508
Cao SX, Chen L, Yu XX (2009) Impact of China’s grain for green project on the landscape of vulnerable arid and semi-arid agricultural regional: a case study in northern Shaanxi province. J Appl Ecol 46(3):536–543
Chen H, Guo SL, Xu CY, Singh VP (2007) Historical temporal trends of hydro-climatic variables and runoff response to climate variability and their relevance in water resource management in the Hanjiang basin. J Hydrol 344(3):171–184
Choudhury B (1999) Evaluation of an empirical equation for annual evaporation using field observations and results from a biophysical model. J Hydrol 216:99–110
Donohue RJ, Roderick ML, McVicar TR (2011) Assessing the differences in sensitivities of runoff to changes in climatic conditions across a large basin. J Hydrol 406(3):234–244
Dooge J, Bruen M, Parmentier B (1999) A simple model for estimating the sensitivity of runoff to long-term changes in precipitation without a change in vegetation. Adv Water Resour 23:153–163
Fu GB, Charles SP, Chiew FHS (2007) A two‐parameter climate elasticity of streamflow index to assess climate change effects on annual streamflow. Water Resour Res 43(11), W11419
Gain AK, Wada Y (2014) Assessment of future water scarcity at different spatial and temporal scales of the Brahmaputra river basin. Water Resour Manag 28:999–1012
Harris JA, Hobbs RJ, Higgs E, Aronson J (2006) Ecological restoration and global climate change. Restor Ecol 14:170–176
Huang MB, Zhang L (2004) Hydrological responses to conservation practices in a catchment of the Loess Plateau, China. Hydrol Process 18:1885–1898
Huang MB, Zhang L, Gallichand J (2003) Runoff responses to afforestation in a watershed of the Loess Plateau, China. Hydrol Process 17(13):2599–2609
Kendall M (1975) Rank correlation measures. Charles Griffin, London
Li LJ, Zhang L, Wang H, Wang J, Yang JW, Jiang DJ, Qin DY (2007) Assessing the impact of climate variability and human activities on streamflow from the Wuding River basin in China. Hydrol Process 21:3485–3491
Liang LQ, Liu Q (2014) Streamflow sensitivity analysis to climate change for a large water‐limited basin. Hydrol Process 28(4):1767–1774
Liang W, Yang YT, Fan DM, Guan HD, Zhang T, Long D, Zhou Y, Bai D (2015) Analysis of spatial and temporal patterns of net primary production and their climate controls in China from 1982 to 2010. Agric For Meteorol 204:22–36
Lü YH, Fu BJ, Feng XM, Zeng Y, Liu Y, Chang RY, Sun G, Wu BF (2012) A policy-driven large scale ecological restoration: quantifying ecosystem services changes in the Loess Plateau of China. PLoS ONE 7(2), e31782
Mann HB (1945) Non-parametric test against trend. Econometrika 13:245–259
Meddi M, Assani A, Meddi H (2010) Temporal variability of annual rainfall in the Macta and Tafna Catchments, northwestern Algeria. Water Resour Manag 24:3817–3833
Mezentsev VS (1955) More on the calculation of average total evaporation. Meteorol Gidrol 5:24–26
Myronidis D, Emmanouloudis D, Mitsopoulos I, Riggos E (2010) Soil erosion potential after fire and rehabilitation treatments in Greece. Environ Model Assess 15(4):239–250
Myronidis D, Stathis D, Ioannou K, Fotakis D (2012) An integration of statistics temporal methods to track the effect of drought in a shallow Mediterranean lake. Water Resour Manag 26(15):4587–4605
Oki T, Kanae S (2006) Global hydrological cycles and world water resources. Science 313(5790):1068–1072
Penman HL (1948) Natural evaporation from open water, bare soil and grass. Proc Roy Soc Lond A 193:120–145
Pettitt A (1979) A non-parametric approach to the change-point problem. Appl Stat 28(2):126–135
Roderick ML, Farquhar GD (2011) A simple framework for relating variations in runoff to variations in climatic conditions and catchment properties. Water Resour Res 47(12), W00G07
Schaake JC (1990) In: Waggone PE (ed) Climate change and US water resources. John Wiley, New York, pp 77–206
Shadmani M, Marofi S, Roknian M (2012) Trend analysis in reference evapotranspiration using Mann-Kendall and Spearman’s Rho tests in arid regions of Iran. Water Resour Manag 26:211–224
She DL, Liu DD, Xia YQ, Shao MA (2014) Modeling effects of land use and vegetation density on soil water dynamics: implications on water resource management. Water Resour Manag 28:2063–2076
Shi CX, Zhou YY, Fan XL, Shao WW (2013) A study on the annual runoff change and its relationship with water and soil conservation practices and climate change in the middle Yellow River basin. Catena 100:31–41
Smakhtin V (1999) Generation of natural daily flow time‐series in regulated rivers using a non‐linear spatial interpolation technique. Regul Rivers: Res Manag 15:311–323
Tang J, Yin X-A, Yang P, Yang Z (2014) Assessment of contributions of climatic variation and human activities to streamflow changes in the Lancang River, China. Water Resour Manag 28:2953–2966
Tucker CJ, Pinzon JE, Brown ME, Slayback DA, Pak EW, Mahoney R, Vermote EF, Nazmi ES (2005) An extended AVHRR 8-km NDVI data set compatible with MODIS and SPOT vegetation NDVI data. Int J Remote Sens 26:4485–4498
Van Ty T, Sunada K, Ichikawa Y, Oishi S (2012) Scenario-based impact assessment of land use/cover and climate changes on water resources and demand: a case study in the Srepok River Basin, Vietnam—Cambodia. Water Resour Manag 26:1387–1407
Vogel RM, Fennessey NM (1994) Flow-duration curves. I: New interpretation and confidence intervals. J Water Res Pl-Asce 120:485–504
Wang DB, Hejazi M (2011) Quantifying the relative contribution of the climate and direct human impacts on mean annual streamflow in the contiguous United States. Water Resour Res 47(10), W00J12
Wang WG, Shao QX, Yang T, Peng SZ, Xing WQ, Sun FC, Luo YF (2013) Quantitative assessment of the impact of climate variability and human activities on runoff changes: a case study in four catchments of the Haihe River basin, China. Hydrol Process 27(8):1158–1174
Xu JX (2004) Response of erosion and sediment produce processes to soil and water conservation measures in Wuding River Basin. Acta Geograph Sin 59:972–981 (in Chinese)
Xu JX (2011) Variation in annual runoff of the Wudinghe River as influenced by climate change and human activity. Quatern Int 244(2):230–237
Xu JX (2012) Effects of climate and land-use change on green-water variations in the Middle Yellow River, China. Hydrol Sci J 58:106–117
Xu XY, Yang DW, Yang HB, Lei HM (2014) Attribution analysis based on the Budyko hypothesis for detecting the dominant cause of runoff decline in Haihe basin. J Hydrol 510:530–540
Yan Y, Yang ZF, Liu Q (2013) Nonlinear trend in streamflow and its response to climate change under complex ecohydrological patterns in the Yellow River Basin, China. Ecol Model 252:220–227
Yang HB, Yang DW, Lei ZD, Sun FB (2008) New analytical derivation of the mean annual water-energy balance equation. Water Resour Res 44(3), W03410
Yang YT, Shang SH, Jiang L (2012) Remote sensing temporal and spatial patterns of evapotranspiration and the responses to water management in a large irrigation district of North China. Agric For Meteorol 164:112–122
Yang YT, Long D, Guan HD, Scanlon B, Simmons C, Jiang L, Xu X (2014) GRACE satellite observed hydrological controls on interannual and seasonal variability in surface greenness over mainland Australia. J Geophys Res-Biogeosci 119:2245–2260
Yang YT, Guan HD, Shen MG, Liang W, Jiang L (2015) Changes in autumn vegetation dormancy onset date and the climate controls across temperate ecosystems in China from 1982 to 2010. Glob Chang Biol 21:652–665
Yao WY, Xu JH, Ran DC (2011) Assessment of changing trends in streamflow and sediment fluxes in the Yellow River basin. Yellow River Conservancy Press, Zhengzhou (in Chinese)
Zhan CS, Zeng SD, Jiang SS, Wang HX, Ye W (2014) An integrated approach for partitioning the effect of climate change and human activities on surface runoff. Water Resour Manag 28(11):3843–3858
Zhang JJ, Ji WH, Feng XD (2002) Study of runoff and sediment load changes in Wuding River basin: Present, causes and future trends. In: Wang G, Fan Z (eds) Study of changes in runoff and sediment load in the yellow river, vol 2. Yellow River Water Conservancy Press, Zhengzhou, pp 393–429, in Chinese
Zhang XP, Zhang L, Zhao J, Rustomji P, Hairsine P (2008a) Responses of streamflow to changes in climate and land use/cover in the Loess Plateau, China. Water Resour Res 44(7), W00A07
Zhang ZQ, Wang SP, Sun G, McNulty SG, Zhang HY et al (2008b) Evaluation of the MIKE SHE model for application in the Loess Plateau, China. J Am Water Resour Assoc 44(5):1108–1120
Zhang A, Zhang C, Fu G, Wang B, Bao Z, Zheng H (2012a) Assessments of impacts of climate change and human activities on runoff with SWAT for the Huifa River Basin, Northeast China. Water Resour Manag 26:2199–2217
Zhang BQ, Wu P, Zhao XN, Wang YB, Gao XD (2012b) Changes in vegetation condition in areas with different gradients (1980–2010) on the Loess Plateau, China. Environ Earth Sci 68(8):2427–2438
Zhang LL, Podlasly C, Ren Y, Feger KH, Wang YH, Schwarzel K (2014) Separating the effects of changes in land management and climatic condition on long-term streamflow trends analyzed for a small catchment in the Loess Plateau region, NW China. Hydrol Process 28(3):1284–1293
Zhao GJ, Tian P, Mu X, Jiao J, Wang F, Gao P (2014) Quantifying the impact of climate variability and human activities on streamflow in the middle reaches of the Yellow River basin, China. J Hydrol 519(Part A):387–398
Zuo D, Xu Z, Wu W, Zhao J, Zhao F (2014) Identification of streamflow response to climate change and human activities in the Wei River Basin, China. Water Resour Manag 28(3):833–851
Acknowledgments
We would like to thank the National Climatic Centre of the China Meteorological Administration for providing the meteorological data. This work was funded by the National Natural Science Foundation of China (No. 41390464, 41401027) and the Open Research Fund of the State Key Laboratory of Hydraulics and Mountain River Engineering (No. SKHL1405). We also thank two anonymous reviewers and editor for their constructive comments which improve greatly the overall quality of the manuscript.
Author information
Authors and Affiliations
Corresponding author
Rights and permissions
About this article
Cite this article
Liang, W., Bai, D., Jin, Z. et al. A Study on the Streamflow Change and its Relationship with Climate Change and Ecological Restoration Measures in a Sediment Concentrated Region in the Loess Plateau, China. Water Resour Manage 29, 4045–4060 (2015). https://doi.org/10.1007/s11269-015-1044-5
Received:
Accepted:
Published:
Issue Date:
DOI: https://doi.org/10.1007/s11269-015-1044-5