Abstract
This paper follows the research mainstream aimed to link the efficiency frontier approaches and the composite indicators (CI) methods. More in detail, the main drawbacks of the CI methods based on Benefit of the Doubt (BoD) approach are the sensitivity to the outliers, the perfect compensability and the lack of consideration about the marginal rate of substitution between simple indicators. Following Simar and Vanhems (J Econ 166(2):342–354, 2012) results, we propose a weighting method that bypassing all previous shortcomings suggests a comprehensive approach to construct robust and non-compensatory composite indicators. This approach is based on the integration of BoD by a directional distance function. In order to better highlight the advantages and limitations of our approach we present two applications: in the first one we test our approach on simulated data, while in the second one we consider the supply level of the Italian national health service with the aim to analyse the regional differences and verify the robustness of the results.
Similar content being viewed by others
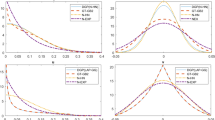
Avoid common mistakes on your manuscript.
1 Introduction
Composite indicators (CI) methods are increasingly recognized as a useful tool in policy analysis and public communication Nardo et al. (2005) for a variety of policy matters such as public units benchmark, health systems, industrial competitiveness, sustainable development, quality of life assessment, intensity of innovation.
Nevertheless the Joint Research Centre of European Commission asserts that “no uniformly agreed methodology exists to weight individual indicators before aggregating them into a composite indicator”.
Several weighting techniques, in fact, have been proposed in literature, derived both from statistical methodologies, such as factor analysis, data envelopment analysis (DEA) and unobserved components models (UCM), and from more specific methods like multi-criteria evaluation (see e.g. Munda 2013), budget allocation processes (BAP), analytic hierarchy processes (AHP), conjoint analysis (CA), method of penalty in the coefficient of variation (MPCV) De Muro et al. (2010) or via Pearson’s correlation ratio Paruolo et al. (2013).
These weighting techniques have been applied in many socio-economic areas, like the evaluation of public policies (see e.g. Prieto and ZofIo 2001; Zafra-Gomez et al. 2010; Klaveren and Witte 2014 for local government performance evaluation; Smith 2002; Kwon 2003, for national health system performance; Storrie and Bjurek 2000, for the European labor market analysis; Cherchye et al. 2004 for social inclusion policies at EU level and Takamura and Tone 2003 for government agencies evaluation). They have been used also in analysis of country performance through macroeconomic indicators (see e.g. Lovell 1995; Lovell et al. 1995; Cherchye 2001; Cherchye et al. 2005 for internal market policies), for the construction of a environmental and ecological performance indicator (see e.g. Zhou et al. 2007; Bellenger and Herlihy 2009; Lo 2010; Sahoo et al. 2011; Rogge 2012; Zanella et al. 2013) and finally for the calculation of the Human Development Index (see e.g. Mahlberg and Obersteiner 2001; Despotis 2005, 2005; Cherchye et al. 2008).
Several research papers concerning the comparison among different weighting methods provide interesting results, but these findings cannot be generalized especially for the specific domains of the applications and because these approaches often produce closed systems in which the conclusion is the argument that has to be demonstrate.
In general, in the CI framework, there are no functional relationships among single indicators covering different aspects of a specific economic or social phenomenon and it cannot be assumed nomic causality Born (1949), meaning that cannot be assumed certain or probabilistic general function covering relationship among instances. Moreover, in a nonparametric perspective, it is not even useful to introduce constraints, parametric functional forms or penalties which may be linked to a specific theoretical model because results would be clearly linked to the theoretical model that has generated them.
From a logical perspective, therefore, the claim of “back to the details” suggested by Nardo et al. (2005) appears misleading, because the resulting composite indicator is clearly linked to the model that generated it.
This happens because, especially in this context, “in the mathematical knowledge the consideration [the assumptions] is an operation that, for the objects, comes from outside; so it follows that the real object is altered”Footnote 1Hegel (1995). Knowledge is considered as a bias with respect to the expected measure and the measure itself influence the analysis, for which there are no neutral actions without consequences: or the thought explains the evolution of the object (adaequatio intellectus ad rem), or the object itself is deformed by the thought (adaequatio rei to intellectus).
Given that premise, the choice among different weighting functions, models or evaluation frameworks can be done or in an axiomatic way or according to the required statistical properties: in our paper, we suggest to highlight the statistical properties in general terms rather than compare it with the most common weighting methods.
Aware that “all models are wrong, some are useful” Box and Draper (1987), therefore, we propose a data driven framework rather than a parametric method or a model linked to the definitions of specific causalities among different dimensions. In particular, we propose a flexible framework where the non-compensability is not imposed in a fixed direction, but is linked to the data themselves and can be imposed in many different directions.
The paper is structured as follows: the methodological proposal and the properties of the CI weighting method are described in Sect. 2, results based on simulated data are explained in Sect. 3.1, while the supply level in Italian national health system is analysed in Sect. 3.2. Concluding remarks are summarized in Sect. 4.
2 A Methodological Proposal
In the present section we first show the foundations of the BoD and directional BoD models illustrated in Vidoli and Mazziotta (2013) and Fusco (2013) and, afterwards, we present a comprehensive model that adds the properties of the robust frontier models to the directional ones; finally, our proposed model is further specified in the particular case when outliers affect the direction.
2.1 The Nonparametric Framework
Our proposal is based on the logical union of two different proposals that aim, in the context of BoD nonparametric frontier models, to bypass two crucial drawbacks: on the one hand the lack of estimates robustness—see Robust BoD proposed by Vidoli and Mazziotta (2013)—and, on the other hand, the full compensability among simple indicators—see directional BoD models proposed by Fusco (2013).
As highlighted by Witte and Rogge (2009), the classical BoD model can be regarded as an output-oriented CRS–DEAFootnote 2 model, with all questionnaire items considered as outputs (\(Y_q \in \mathbb {R}_+ , \forall q=1 \ldots Q\)) and a single input equal to one for all observations.
Therefore the production set of a CRS–DEA model, in the BoD framework as a particular case, can be written as:
where \(\varPsi \) is the support of \(H(1\!{\rm l},{\mathbf {y}})\):
Having defined the support \(\varPsi \) and the random set \(H(1\!{\rm l},{\mathbf {y}})\) it is possible to calculate the Farrel–Debreu (output) efficiency score as:
and, consequently, the BoD CI as the reciprocal of \(\lambda (1\!{\rm l},{\mathbf {y}})\).
Composite indicators, calculated through Eq. (3), satisfy the following desirable properties:

BoD model is usually based on the strong assumption of compensability among different simple indicators, but in practical applications very often exists a preference structure. In order to avoid this drawback, Fusco (2013) suggests to include in the classical BoD model—equation (3)—a directional penalty using a directional distance function.
Using the BoD notation, the composite indicator can be calculated as the reciprocal of the directional distance function \(D(1\!{\rm l},{\mathbf {y}};g_y)\):
where \(g_y\) is the directional vector.
The directional BoD adds the following properties to the classical BoD model:

2.2 Robust Directional BoD with a Generic Direction
Models highlighted in Sect. 2.1 still suffer of a serious drawback: the lack of robustness with respect to outliers. To overcome this significant shortcoming the production set \(\varPsi \) can be translated in a probabilistic framework following Daraio and Simar (2005)’s proposal; considering a sample of \(m\) random variables with replacement \(\varvec{S}_{m}=\{{\mathbf{Y}_{\mathbf{i}}}\}_{\mathbf{i=1}}^{\mathbf{m}}\) drawn from the density of \({\mathbf {Y}}\), we define the random set \(\widetilde{\varPsi }_m\) as:
Therefore, the effect of an abnormal or outlier unit is damped given that the single unit is not compared to all the others, but to a sample subset of size \(m\).
This generalization allows us to calculate iteratively the sample subset of size \(m\) (for \(b=1,...,B\) times) and for each \(b\) iteration the directional distance for the single unit from the maximum values can be defined as:
More specifically Eq. (6) can be practically computed, in a multivariate setting, as suggested by Daouia and Gijbels (2010), by using the dimensionless transformation by minimum for the \({\mathbf {y}}^{*}= g_y \cdot {\mathbf {y}}\):
and the order-\(m\) directional distance estimator \(D_m(1\!{\rm l},{\mathbf {y}};g_y)\) as:
Following Cazals et al. (2002), finally, we can approximate the order-\(m\) directional distance estimator–even in a Shepard formulation—by computing the empirical mean over \(B\):
The Robust directional BoD adds the following property to the classical BoD model and to directional BoD:

For seek of clarity, Sect. 3.1 illustrates a graphical representation of the method using simulated data, in order to highlight that units’ scores are not affected by outliers.
2.2.1 Robust PCA Directional BoD
In the previous section a generic direction \(g_y\) has been included in the robust directional method proposed.
For practical computation, however, the direction \(g_y\), can be seen, for example, as the marginal rate of substitution among indicators; following this criteria, in case of additional information, it may be imposed by the researcher or derived directly from the data, for example through a principal components analysis (PCA) as in Fusco (2013).
PCA, in fact, lets to calculate the first principal component with the largest variance showing the internal structure and the main pattern of the data with the advantages of ease of calculation.
Despite this attractive feature, however, PCA models have several shortcomings; among others, all the classical PCA algorithms—based on least squares techniques—are set up on the assumption that outliers are not included in the dataset.
In order to bypass PCA outliers drawback, several robust versions of PCA have been developed by a modification of the covariance matrix (see e.g. Campbell 1980), by Projection Pursuit (see e.g. Li and Chen 1985) or by weighting singular value decomposition (SVD) (see e.g. Gabriel and Zamir 1979).
In particular, in this application, we propose to use Robust PCA by Projection Pursuit because the more robust covariance matrix can be computationally intensive, especially if the involved covariance matrices must be estimated in a robust way (see in particular Croux et al. 2007; Filzmoser et al. 2006).
This particular choice of \(g_y\), based on PCA by Projection Pursuit, adds the following property to the Robust directional BoD:

Simulation in Sect. 3.1 highlights the robustness of the main direction estimated in presence of outliers.
3 Application
In order to better describe the advantages and limitations of our approach, in this section we present two applications: the first one is based on simulated data, to better illustrate, in a cross-sectional framework, the robustness and the non-compensability with respect to a main direction. In the second application based on panel data, instead, we consider the supply level offered by the Italian national health service for the years 1998–2010 with the aim to highlight the regional divergence and to verify the robustness of the results changing the weighting model.
3.1 Simulated Data
In the present subsection multiple simulationsFootnote 3 are introduced with the aim to test the validity of our approach, to illustrate the required properties of the suggested methods and to proof the stability towards another ones.
More specifically, we have generated a two-dimensional dataset of 300 units \(i\) with a marginal rate of substitution between first and second indicator equal to 0.5.
In order to test the robustness of our measures, we have added to dataset an outlier set with a marginal rate of substitution between first and second indicator higher than 1.5.
More specifically, in the following figures, we have plotted the simulated simple indicators \(I_1\) and \(I_2\) with an increasing size and colour for higher values of CI; the composite indicator isoquants (light green for lower values of the composite indicator, dark green for higher values), are also displayed.
Figure 1 confirms that the selected approach is able to satisfy the requested properties outlined in Sect. 2; in particular, it can be highlighted that—without imposing a priori compensability or non-compensability among indicators—directional measure lets to obtain a monotonic increasing of CI when \(I_1\) or \(I_2\) increase.
At the same time, it can be observed how outliers clearly influence (estimated rate of substitution equal to 0.71) the estimated direction \(g_y\) (internal robustness property) when classical PCA is used, while they don’t have any impact on the frontier estimation (external robustness property) and on the relative ranking.
Finally, it can be noted that the CI is greater than 1 for the outlier units; this finding (in agreement with the order-\(m\) model) doesn’t affect the scores of the other units and it is indicative of its characteristic.
With the aim to correctly estimate the real direction, we have applied the Robust PCA by Projection Pursuit to the same dataset obtaining an estimated rate of substitution equal to 0.51 (see Fig. 2) very similar to the real one (0.5).
Construction of CI, in general terms, involves stages in which subjective judgements have to be made: selection of indicators, treatment of missing values, choice of aggregation model, estimation of the optimal weights assigned to the simple indicators and so on.
For these reasons, it is important to identify the sources of subjective assessment and/or data errors and use uncertainty and sensitivity analysisFootnote 4 (see Saltelli et al. 2008, 2004; Saisana and D’Hombres 2008) to gain useful insights during the process of CI building, including an appraisal of the reliability of units’ ranking.
In our paper, we have applied uncertainty analysis only with respect to changes in the proposed frontier models—BoD, RBoD, directional BoD and Robust PCA (by Projection Pursuit) directional BoD—in order to obtain informations about the introduction of robust and directional measures. More specifically, we have compared the individual units scores varying method not in terms of rank, since this would have hidden the impact of outliers, but in terms of pure scores given that all models were based on the same underlying assumptions.
Figure 3, showing the average score among the four models and the relative standard deviation, is very informative: in fact, it should be noted that, for the regular units (orange points) increasing the average score (close to the frontier) the variation of the model has increasingly less impact, while the outlier units (blue points) show an higher standard deviations.
Finally, to assess the impact of the direction, we have analysed, even in terms of mean and standard deviation only two models: the Robust directional BoD and the Robust PCA (by Projection Pursuit) directional BoD model. Figure 4 shows that the direction has no impact on the regular units scores, while strongly penalizes outliers.
3.2 Supply Levels in Italian Health System
The evaluation and the improvement of the national health system performance has become a key policy subject in most developed nations; in the last years many national authorities, as the United Kingdom National Health Service (NHS) and the Canadian Institute for Health Information, or research analysis on national health system (see e.g Jencks et al. 2000; Kwon 2003; Nuti et al. 2011 for the Italian health care system), have put in place.
Many subjects and objectives may be considered behind an health system evaluation framework: the scope of performance indicators, for this reason, can be considerable ranged for examining the state of a nation’s health system to reflect the experiences of the individual patients. Performance, efficiency or analysis on the supply side could be carried out at international, national, regional, local or institutional level Ibrahim (2001).
So a key question, especially in the complex healthcare system is: what should be measured? In general, health care systems can be evaluated with respect to the quality/quantity of care, to the access to care or to the cost (which, however, are not the only analytical dimensions); Paakkonen and Seppala (2014), for example, takes into account in its analysis the accessibility, the efficiency and equality.
But the real challenge in the evaluation models is the multidimensionality of health systems closely linked with the development of composite measures; in order to assess, compare and improve performance, quality or supply between or inside countries it is crucial to dispose of: (1) a set of measurable and reliable indicators built up from a good information system, (2) a robust and stable method to integrate indicators in a composite one and to set benchmarks.
Smith (2002), more specifically, discusses three methodological issues in the health sector related to composite indices: (1) the development of a set of weights, (2) the treatment of exogenous influences on system performance and (3) the modelling of efficiency; he notes that there isn’t a wide consensus regarding methodology issues, such as the weights to be used to form the composite index. In addition, composite measures of health system performance “lack precision and combine uncertain weighting systems, imprecision arising from the potential non-comparability of component measures, and misleading reliability in the form of whole-population averages that mask distribution issues” Bankauskaite and Dargent (2007).
Moreover, in our opinion, there are other issues related with the correct choice of the weights not yet fully highlighted in the literature; these matters are related to the public nature of the service: (1) optimal weights may not coincide with the marginal utility of the citizen and (2) weights can not be assumed as invariant to the increase of the Local Authorities dimension (see the results of Tiebout 1956).
Given these premises, it seems even more appropriate to propose a method with this particular characteristics: (1) weight endogeneity (property #1), variance of optimal weights among units (linked to the property #3), (3) robustness to outliers (property #6) and easily extension to a model that also control the effect of contextual variables (see Vidoli and Mazziotta 2013).
From an economic point of view, in this paper research questions are linked to administrative changes occurred in the last 15 years in Italy; we focus, therefore, on structural changes and on the different trends—only on the supply side of the Italian regions—due to the administrative devolution from the central government to the single local authority. More specifically, the aim of our application is the measurement of the variations on the supply side and the evaluation of territorial differences between richest regions (northern ones) and the less developed regions (southern ones).
For this purpose, we used the database of indicators regarding the health system in ItalyFootnote 5, provided by ISTAT for the years 1998–2010, containing more than 4,000 indicators on the socio-demographic aspects, lifestyles, disabilities and dependencies, monetary and input resources and health care supply.
Specifying that the accurate analysis on the whole health system is beyond the scope of this paper, we focus our attention, as previously mentioned, exclusively on the per capita outputs evaluating if the regional spending and legislative autonomy of each region has brought the healthcare system supply towards a territorial balance or not.
In order to avoid collinearity among the elementary indicators, linked to the multidimensionality nature of the informative setting, in a first step we get two main independent and informative factors through principal component analysisFootnote 6 (see Table 1).
Principal component analysis highlights two factors: the first one can be interpreted as the dimensional factor, while the second one appears to be more dependent to the proxies of the quality of the health system.
Figures 5 and 6 show a different temporal evolution among different regions: in fact, while supply, in terms of dimension, remains fairly stable or slightly decreasing (due to the national spending review laws), quality seems to be very differentiated.
In Fig. 7 we plot the main [\(g_y=(1,1.24)\)] and the compensative [\(g_y=(1,1)\)] direction on Factor 1 (dimension) and Factor 2 (quality) by regional code (lower values = northern regions, higher values = southern regions); given this plot, we expect that, introducing the main direction respectively in the BoD and in RBod models, the overall composite indicators remain stable.
Table 2 confirms this conjecture showing how, at least in ranks, the composite indicator is very robust to changes in the estimation model.
Even if in most regions there was an increase of the provided output—in terms of composite indicator—from a relative point of view, we observe an highest level of performance in the northern regions (Lombardia, Veneto, Emilia Romagna and Piemonte, with a maximum in Lombardia, see Fig. 8). The chosen dataset highlights one of the limitations of our approach: not being able to disentangle the increase due to technical progress (frontier shifting) and due to the improvement of a single region; in this setting, in other terms, all regions are analysed in a cross section framework, i.e. all units are compared to a single frontier.
From an economic point of view, it is interesting verify if the major level of service provided by the northern regions in the last year (2010) is due to better initial conditions or whether it is the result of better management of resources over the time; for this purpose we have computed, for each region \(i\), the main trend of the composite indicator \(\beta _i\) regressing the directional robust composite indicator on years \(t\):
In Fig. 9 is straightforward note that northern regionsFootnote 7 have increased their supply level while the southern ones have, even more, decrease their services both in qualitative and quantitative terms.
Last research question concerns the robustness and reliability of the results; in fact, even if there is a good correlation between methods in global terms (see Table 2), Fig. 10 shows that this result is not always verified for all regions; more specifically, in terms of rank differences (between the Directional RBoD and BoD), the variation of the weighting scheme seems to have a lower impact in northern regions (closer to the frontier), while it is more consistent for the southern ones.
4 Concluding Remarks
This paper is included in the wide theme of the composite indicators construction. The authors have before dealt with this research sector by suggesting several proposals of CI, in which characteristics of robustness and non-compensability have been continually pursued. In this context a method based on the Benefit of the Doubt technique has been developed and applied overall on infrastructure simple indicators. In progress, some improvements have been experimented; in particular: the order-\(m\) approach has been proposed in order to face the problem of outliers, and the directional approach has been integrated in the original BoD in order to enhance the characteristic of non-compensability of simple indicators.
The specific aim of this paper is to proceed on this research direction through the formulation of a comprehensive method of CI construction able to strengthen the mentioned characteristics, limiting as much as possible the usual drawbacks of CI: the sensitivity to the outliers, the non-compensability and the problem of the marginal rate of substitution between simple indicators. For this purpose, the paper has proposed a comprehensive model that adds the properties of the robust frontier with the directional ones. The paper is essentially methodological even if a simulation and an application are presented in order to illustrate the properties of the proposed method.
In summary, the method maintains the same peculiarity of previous works, namely to be a data driven approach and to be based on data variability as principal criteria of construction. More specifically, the proposed method is characterized by the following properties: 1. Weights are endogenously determined; 2. The weighting function is weakly positive monotone; 3. The weighting scheme is the highest as possible; 4. May be imposed non-compensability among simple indicators; 5. The obtained CI is invariant to the normalization method; 6. Outliers influence is removed (robustness); 7. Robustness by means of directional BoD based on PCA is improved.
The illustrated simulation introduces, beside the initial two-indicators dataset, an outliers set characterized by the abnormal rate of substitution between those two indicators. The construction of the composite indicator by the way of the Robust PCA Directional BoD confirms that the proposed method assures, as much as possible, the characteristics of robustness and non-compensability.
Finally, health system in Italy has been analysed for the years 1998–2010; results show that differences between richer regions with respect to the other ones are increased in terms of level of service for a better management of resources over time rather than different initial conditions.
This application, in particular, highlights two very important issues still to be considered in our approach: i) the ability to include the temporal dimension in the model, estimating how the composite measure changes with respect respectively to the frontier and to the main direction over the years and ii) the inclusion of contextual variables, also in a non-parametric way, in order to control the influence of different external conditions in the computation of the composite indicator.
Notes
In Italian “Nel conoscere matematico la considerazione è un operare che, per la cosa, vien da fuori; ne segue quindi che la cosa vera viene alterata”.
CRS–DEA: Constant Return to Scale Data Envelopment Analysis— see Charnes et al. (1978).
Simulations have been carried out thanks to Compind R package, available from the authors.
The purpose of uncertainty analysis is to determine the uncertainty in estimates for dependent variables of interest, while the purpose of sensitivity analysis is to determine the relationships between the uncertainty in the independent variables used in an analysis and the uncertainty in the resultant dependent variables.
This database is named “Health for all”, available at http://www.istat.it/it/archivio/14562.
Total variance explained by the two factors: 77 %; printed values are multiplied by 100 and rounded to the nearest integer; values greater than 0.6 are marked with “*”; values less than 0.3 are not printed.
Except for Trentino-Alto Adige—regional code 4—since it’s a autonomous status region.
References
Bankauskaite, V., & Dargent, G. (2007). Health systems performance indicators: Methodological issues. Presupuesto y gasto publico, 49, 125–137.
Bellenger, M. J., & Herlihy, A. T. (2009). An economic approach to environmental indices. Ecological Economics, 68, 2216–2223.
Born, M. (1949). Natural philosophy of cause and chance. New York: Dover Publications.
Box, G. E. P., & Draper, N. R. (1987). Empirical model building and response surfaces. New York, NY: Wiley.
Campbell, N. A. (1980). Robust procedures in multivariate analysis i: Robust covariance estimation. Applied Statistics, 29(3), 231–237.
Cazals, C., Florens, J., & Simar, L. (2002). Nonparametric frontier estimation: A robust approach. Journal of Econometrics, 106(1), 1–25.
Charnes, A., Cooper, W., & Rhodes, W. (1978). Measuring the efficiency of decision making units. European Journal of Operational Research, 2(4), 429–444.
Cherchye, L. (2001). Using data envelopment analysis to assess macroeconomic policy performance. Applied Economics, 33(3), 407–416.
Cherchye, L., Lovell, K., Moesen, W., Puyenbroeck, & T. V. (2005). One market, one number? A composite indicator assessment of eu internal market dynamics. In Technical report, working paper series ces0513, Katholieke Universiteit Leuven, Centrum voor Economische Studien.
Cherchye, L., Moesen, W., & Puyenbroeck, T. (2004). Legitimately diverse, yet comparable: On synthesizing social inclusion performance in the eu. Journal of Common Market Studies, 42(5), 919–955.
Cherchye, L., Ooghe, E., & Van Puyenbroeck, T. (2008). Robust human development rankings. Journal of Economic Inequality, 6, 287–321.
Croux, C., Filzmoser, P., & Oliveira, M. (2007). Algorithms for projection-pursuit robust principal component analysis. Chemometrics and Intelligent Laboratory Systems, 87, 218–225.
Daouia, A., & Gijbels, I. (2010). Robustness and inference in nonparametric partial-frontier modeling. Journal of Econometrics, 161, 147–165.
Daraio, C., & Simar, L. (2005). Introducing environmental variables in nonparametric frontier models: A probabilistic approach. Journal of Productivity Analysis, 24(1), 93–121.
De Muro, P., Mazziotta, M., & Pareto, A. (2010). Composite indices of development and poverty: An application to mdgs. Social Indicators Research, 104(1), 1–18.
Despotis, D. K. (2005). Measuring human development via data envelopment analysis: The case of asia and the pacific. Omega, 33(5), 385–390.
Despotis, D. K. (2005). A reassessment of the human development index via data envelopment analysis. Journal of the Operational Research Society, 56, 969–980.
Filzmoser, P., Serneels, S., Croux, C., & Van Espen, P. J. (2006). Robust multivariate methods: The projection pursuit approach, chap. In M. Spiliopoulou, R. Kruse, C. Borgelt, A. Nürnberger & W. Gaul (Eds.), From data and information analysis to knowledge engineering (pp. 270–277). Berlin: Springer.
Fusco, E. (2013). Enhancing non compensatory composite indicators: A directional proposal. In XXXIV AISRe conference, Palermo.
Gabriel, K., & Zamir, S. (1979). Lower rank approximation of matrices by least squares with any choice of weights. Technometrics, 21, 489–498.
Hegel, G. (1995). Fenomenologia dello spirito (Phänomenologie des Geistes). Bompiani: Milano.
Ibrahim, J. (2001). Performance indicators from all perspectives. International Journal for Quality in Health Care, 13, 431–432.
Jencks, S., Cuerdon, T., Burwen, D., Fleming, B., Houck, P. M., Kussmaul, A. E., Nilasena, D. S., Ordin, D. L., & Arday, D. R. (2000). Quality of medical care delivered to medicare beneficiaries: A profile at state and national levels. Journal of the American Medical Association, 284(13), 1670–1676.
Kwon, S. (2003). Health and health care. Social Indicators Research, 62–63(1–3), 171–186.
Li, G., & Chen, Z. (1985). Projection-pursuit approach to robust dispersion matrices and principal components: Primary theory and monte carlo. Journal of American Statistical Association, 80(391), 759–766.
Lo, S. F. (2010). The differing capabilities to respond to the challenge of climate change across annex parties under the kyoto protocol. Environmental Science & Policy, 13(1), 42–54.
Lovell, C. K. (1995). Measuring the macroeconomic performance of the taiwanese economy. International Journal of Production Economics, 39, 165–178.
Lovell, C. K., Pastor, J. T., & Turner, J. A. (1995). Measuring macroeconomic performance in the oecd: A comparison of european and non-european countries. European Journal of Operational Research, 87(3), 507–518.
Mahlberg, B., & Obersteiner, M. (2001). Remeasuring the hdi by data envelopment analysis. In Technical report, IIASA, interim report IR-01-069, Laxemburg, Austria.
Munda, G. (2013). Beyond gdp: An overview of measurement issues in redefining “wealth”. Journal of Economic Surveys. doi:10.1111/joes.12057.
Nardo, M., Saisana, M., Saltelli, A., Tarantola, S., Hoffman, A., & Giovannini, E. (2005). Handbook on constructing composite indicators: Methodology and user guide. In OECD statistics working papers 2005/3, OECD, Statistics Directorate.
Nuti, S., Daraio, C., Speroni, C., & Vainieri, M. (2011). Relationships between technical efficiency and the quality and costs of health care in italy. International Journal for Quality in Health Care, 23(3), 324–330.
Paakkonen, J., & Seppala, T. (2014). Using composite indicators to evaluate the efficiency of health care system. Applied Economics, 46(19), 2242–2250.
Paruolo, P., Saisana, M., & Saltelli, A. (2013). Ratings and rankings: Voodoo or science? Journal of the Royal Statistical Society: Series A (Statistics in Society), 176(3), 609–634.
Prieto, A., & ZofIo, J. (2001). Evaluating effectiveness in public provision of infrastructure and equipment: The case of spanish municipalities. Journal of Productivity Analysis, 15, 41–58.
Rogge, N. (2012). Undesirable specialization in the construction of composite policy indicators: The environmental performance index. Ecological Indicators, 23, 143–154.
Sahoo, B. K., Luptacik, M., & Mahlberg, B. (2011). Alternative measures of environmental technology structure in dea: An application. European Journal of Operational Research, 215(3), 750–762.
Saisana, M., & D’Hombres, B. (2008). Higher education rankings: Robustness issues and critical assessment. How much confidence can we have in higher education rankings. In Technical report, JRC scientific and technical reports, European Commission.
Saltelli, A., Ratto, M., Andres, T., Campolongo, F., Cariboni, J., Gatelli, D., et al. (2008). Global sensitivity analysis: The primer. New York: Wiley.
Saltelli, A., Tarantola, S., Campolongo, F., & Ratto, M. (2004). Sensitivity analysis in practice. A guide to assessing scientific models. New York: Wiley.
Simar, L., & Vanhems, A. (2012). Probabilistic characterization of directional distances and their robust versions. Journal of Econometrics, 166(2), 342–354.
Smith, P. (2002). Measuring health system performance. European Journal of Health Economics, 3(3), 145–148.
Smith, P. (2002). Measuring Up: Improving Health System Performance in OECD Countries, chap. Developing Composite Indicators for Assessing Health System Efficiency, pp. 295–316. OECD Publishing.
Storrie, D., & Bjurek, H. (2000). Benchmarking european labour market performance with efficiency frontier techniques. Tech. rep.: CELMS Discussion papers, Goteborg University.
Takamura, Y., & Tone, K. (2003). A comparative site evaluation study for relocating japanese government agencies out of tokyo. Socio-Economic Planning Sciences, 37, 85–102.
Tiebout, C. (1956). A pure theory of local expenditures. Journal of Political Economy, 64(5), 416–424.
Van Klaveren, C., & De Witte, K. (2014). How are teachers teaching? A nonparametric approach. Education Economics, 22(1), 3–23.
Vidoli, F., & Mazziotta, C. (2013). Robust weighted composite indicators by means of frontier methods with an application to european infrastructure endowment. Statistica Applicata—Italian Journal of Applied Statistics, 23(2), 259–282.
Witte, K. D., Rogge, N. (2009). Accounting for exogenous influences in a benevolent performance evaluation of teachers. Tech. rep., Working Paper Series ces0913, Katholieke Universiteit Leuven, Centrum voor Economische Studien.
Zafra-Gomez, J., Antonio, M., & Perez, M. (2010). Overcoming cost-inefficiencies within small municipalities: Improve financial condition or reduce the quality of public services? Environment and Planning C: Government and Policy, 28(4), 609–629.
Zanella, A., Camanho, A. S., & Dias, T. G. (2013). Benchmarking countries’ environmental performance. Journal of the Operational Research Society, 64(3), 426–438.
Zhou, P., Ang, B., & Poh, K. (2007). A mathematical programming approach to constructing composite indicators. Ecological Economics, 62(2), 291–297.
Author information
Authors and Affiliations
Corresponding author
Rights and permissions
About this article
Cite this article
Vidoli, F., Fusco, E. & Mazziotta, C. Non-compensability in Composite Indicators: A Robust Directional Frontier Method. Soc Indic Res 122, 635–652 (2015). https://doi.org/10.1007/s11205-014-0710-y
Accepted:
Published:
Issue Date:
DOI: https://doi.org/10.1007/s11205-014-0710-y