Abstract
Background
The SF36 Version 2 (SF36V2) is a revision of the SF36 Version 1, and is a widely used health status measure. It is important that guidelines for interpreting scores are available.
Method
A population sample of Australians (n = 3015) weighted to achieve representativeness was administered the SF36V2. Comparisons between published US weights and sample derived weights were made, and Australian population norms computed and presented.
Major findings
Significant differences were observed on 7/8 scales and on the mental health summary scale. Possible causes of these findings may include different sampling and data collection procedures, demographic characteristics, differences in data collection time (1998 vs. 2004), differences in health status or differences in cultural perception of the meaning of health. Australian population norms by age cohort, gender and health status are reported by T-score as recommended by the instrument developers. Additionally, the proportions of cases within T-score deciles are presented and show there are important data distribution issues.
Principal conclusions
The procedures reported here may be used by other researchers where local effects are suspected. The population norms presented may be of interest. There are statistical artefacts associated with T-scores that have implications for how SF36V2 data are analysed and interpreted.
Similar content being viewed by others
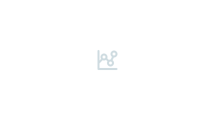
Avoid common mistakes on your manuscript.
Introduction
The SF-36 Version 1 [SF36V1, 1], released in 1988, is a widely used health status measure; a search of PubMed (May 2006) identified 5,405 references. Of these, many are local translation or validation studies examining its psychometric properties. For example, there were 130 Australian studies, including several validation studies of the Australian version of the SF-36 Version 1 [2–6].
In response to identified shortcomings of the SF-36 Version 1, the “international version–of the SF-36–the SF-36 Version 2 (hereafter SF36V2) [7]–was developed between 1996 and 2000. These shortcomings included cross-cultural non-equivalence, difficulties with some word meanings, possible double negatives, floor and ceiling effects, poor performance of the two role function scales, confusion caused by the standard layout and insensitivity in some population settings [7–16].
The changes were aimed at making it easier to understand, reducing missing data, improving the two role function scales–sensitivity, and to simplifying the response categories for the mental health and vitality scales. An important reason for these changes was the finding from the International Quality of Life Assessment (IQOLA) project that the SF36V1 had cultural inconsistencies following translation [17–19]. The changes may also have been influenced by the development of the US Veterans SF36 in 1996, which replaced the dichotomous item responses for the two role scales (Role Physical (RP) and Role Emotion (RE)) with multiple choice scales [20]. A key aim was to ensure that the SF36V2 has stronger cross-cultural validity defined as improved comparability in relation to translation and cultural adaptation [7]. A further change involved presenting the eight scale scores as T-scores [21], whereas in SF36V1 these were presented as percentage scores [1].
A further difference between the SF36V1 and the SF36V2 is the licensing and costs. The original RAND 36-Item Short Form Health Survey is available free to all users. It differs from the proprietary SF36V1 instrument only in the scoring algorithms. The proprietary SF36 instruments are copyrighted and users must be registered. The original licences for the SF36V1 were to research teams, which could use it indefinitely. With the SF36V2, licences are granted on a user-pays per project basis. Full details can be found at: http://www.sf-36.org/.
Since publication of the SF36V2, in addition to the US population norms provided by the developers, there have been three population validation studies. Regarding data from the US, Ware et al. [7] administered the SF36V1 and SF36V2 to a population sample (n = 6742), with random instrument allocation. The increased response choices in the RP and RE scales increased their sensitivity and mean scores (for RP the mean score on the SF36V1 was 75.1 and on SF36V2 it was 80.8, for the RE scale these were 83.7 and 86.3, respectively), and somewhat reduced floor and ceiling effects (RP from 62% to 47% and 14% to 2%, and RE from 74% to 60% and 9% to 1%, respectively). The reliability of these two scales also increased (RP from Cronbach α = 0.88 to 0.95, and RE 0.82 to 0.93). There were no substantial effects on any of the other scales. In general, the revisions aimed to improve the measurement properties of the SF36 without any change to internal structure.
Whether these changes make the SF36V2 a superior measure to the SF36V1 has not yet been sufficiently studied. Kazis et al. [20], based on an analysis of 2737 US veterans who completed both the SF36V1 and the Veterans SF36, reported that the modifications to the RP and RE scales improved their measurement properties and lowered scores on these scales by about 30% of a standard deviation thus alleviating some of the ceiling effects. The improvement in measurement efficiency for these scales was about 11% when compared with the SF36V1. Taft et al. [22] administered the SF36V2 to 2185 18–5 year-old randomly chosen national sample of Swedes and reported similar better measurement results to those reported by Ware et al. Jenkinson et al. [23] collected responses from 8889 Britons of working age, again finding improved measurement properties of the SF36V2. Unlike the Taft et al. study, however, Jenkinson et al. also published factor score coefficients which could be used as British weights for scoring of the summary scales (PCS and MCS) rather than the US weights published by Ware et al. None of these studies, however, reported population norms by gender and age group. A recent population-based study suggested there was no meaningful difference between the two versions [24].
Alongside the US version of the SF36V2, an Australia version was also released and reviewed by Sansoni and Costi [25]. Table 1 describes the descriptive system differences between the Australian SF36V1, the US SF36V2 and the Australian SF36V2. The two key points are the increased response categories for questions 4, 5 and 9 and that US version uses imperial units while the Australian version uses metric units. For 3G, for example, the response categories might suggest that respondents using the US version would have to be able to walk 60% further than their Australian counterparts to indicate equivalent health status (1 mile equals 1.6 km). Whether these differences matter is unknown, although for people with specific common diseases such as arthritis or chronic obstructive pulmonary disease this difference may mean being unable to do their own shopping or other daily activities. One study of the SF36V1 has suggested that respondents find it difficult to interpret these questions [14].
The international and Australian versions of the SF36V2 differ only on questions 3G, 3H, and 3I. There are also slight differences in the instructions as the Australian version includes a practice question.
Although Sansoni and Costi acknowledged some advantages of the descriptive system of the SF36V2 over the SF36V1, they also noted that the absence of Australian normative data limited its use by Australian researchers. This paper rectifies this situation by providing normative data based on Australian weights derived from similar analysis procedures used to derive the US weights reported by Ware et al. [7] and the Australian population norms for the SF36V1 published by the Australian Bureau of Statistics (ABS) [26]. The norms reported in this paper may be used by Australian researchers as benchmarks for the interpretation of their SF36V2 data, and they provide valuable insights for other researchers using the SF36V2.
Methods
Participants
Data were collected from 3015 South Australians who participated in the 2004 South Australian Health Omnibus Survey (SAHOS) [27]. The SAHOS is a population-based researcher-pays health survey which has been carried out annually since 1991. A full description of the methodology can be found in Wilson et al. [28]. In brief, the 2004 survey involved face-to-face interview with sampled households throughout South Australia, including all rural towns with a population of 1000 or greater. For the metropolitan sample, the ABS collectors districts for the 2001 Census were sampled based on probability of selection proportional to size (n = 363 districts). Within districts, using a ‘skip–pattern of every 4th household, 10 dwellings were chosen and one person (aged 15 or more years) from each dwelling interviewed, based on closest last birthday to interview day. Similar procedures were also used to select the rural sample, based on 107 districts.
Materials
Thirteen different research groups participated in the SAHOS sponsoring the use of 32 different measures. This study reports the use of the SF36V2, questions on demographics and question 16 from the HUI-3 (see below). The SF36V2 was the first instrument in the battery of measures.
Demographics
The demographic items used in this study were gender, age, birth country, relationship status, education attainment, and workforce participation.
The SF36V2
The SF36V2 [7] is a health status (function) measure comprising 36 items which are organised into 8 scales (Physical Functioning (PF), Role Physical (RP), Bodily Pain (BP), General Health (GH), Vitality (VT), Social Functioning (SF), Role Emotion (RE) and Mental Health (MH). There is a health transition item (Compared to one year ago, how would you rate your health in general now?). The 8 scales can be combined into 2 summary measures, providing overall estimates of physical health (Physical component score, PCS) and mental health (Mental component score, MCS).
The most important change between the SF36V1 and the SF36V2 relates to how the scale scores are presented. In the SF36V1, scale scores are presented on percentage scales (0–00), whereas for SF36V2 although percentage scores are computed, Ware et al. recommend presenting scale scores as T-scores. This extension of the T-score presentation from the PCS and MCS summary scales to the 8 scales means that each of the eight scales forming the SF36V2 can be reported as either percentage or T-scores. T-scores are described in the SF36V2 manual as “norm-based–scores [7]. An advantage of T-scores is that interpretation is made easy because the mean and standard deviation are always known.
The two summary scores, PCS and MCS, use the sum of the eight dimension z-scores weighted by factor score coefficients. The factor score coefficients are derived from US 1990 general population estimates [7, 29].
In the present study, the US weights described above were replaced with Australian weights derived from the SAHOS dataset. These procedures are consistent with those used by the ABS when it reported Australian population weights for the SF36V1 [26].
HUI-3
The HUI-3 is a multi-attribute utility measure comprising 15 items [30]. A 16th item asks respondents Overall, how would you rate your usual health in the past 4 weeks? Excellent/ Very good/ Good/ Fair/Poor. This is the only item from the HUI-3 used in this study.
Data analysis
All data were double-entered and verified prior to analysis. Missing data were collected by follow up telephone interview wherever possible. Consistent with Jenkinson et al. [23] missing data have not been imputed. Standard weighting procedures were used, i.e., the actual numbers were weighted by the inverse of the probability of selection and then re-weighted to benchmarks from the 2002 Estimated Resident Population [31] and the 2001 Census of Population to achieve representativeness [32]. The combination of small numbers of missing data (< 1%) with weighting accounts for the slightly different numbers in the tables.
To replicate methods used in deriving the SF36V2 scores, exploratory factor analysis with orthogonal rotation (varimax) was used to extract factor coefficients (Ware et al. [7] report extracting the US coefficients with a two-stage factor analysis; a principal components analysis to confirm the higher order two-factor structure, and an orthogonal rotation to identify the coefficients. For obvious reasons we have not replicated the principal component analysis). For comparison between US and Australian data, given that only summaries were available for the US data, 95% confidence intervals were computed or bootstrapped where bootstrapping was carried out by randomly sampling 10% of respondents with replacement 50 times. As data were non-normally distributed, Kruskall-Wallis χ2 was used to compare groups and the Wilcoxon rank sum test to compare matched pairs.
The data were analysed in SPSS V13.0 [33].
Results
Participants
Four thousand seven hundred dwellings were selected, 127 were vacant, 366 dwellings were non-contactable after six visits, 39 dwellings could not be accessed, in 82 dwellings the respondent was unable to speak English, 58 cases were absent during the data collection phase, 62 were incapacitated due to illness, and 945 refused to participate. The total number of participants was 3015, giving a within scope response rate of 72% (3015/(4700–66)). This sample size is consistent with the IQOLA project recommendations on sample size for population norming studies to be between 2500 and 3000 representative participants to allow for examination by gender and 10-year age cohorts [34].
Females comprised 50.9% of the sample, and the mean age was 45.29 years (SD = 18.69 years). For region of birth, 75.3% were born in Australia or New Zealand, 12.2% the UK or Ireland, 4.2% in North-West or Other European countries, 2.4% in South-East Europe, 3.4% in Asia and 2.5% in other parts of the world. For relationship status, 61.9% had a partner (married, de facto), 24.1% had never married, 8.5% were separated or divorced, and 5.6% were widowed. Primary school education only was reported by 18.4%, 32.5% had completed high school, 12.6% held a trade qualification, 22.6% a certificate or diploma and 13.9% a university degree. Full time employment was reported by 38.8%, part-time employment by 16.9%, being unemployed by 2.1%, home duties by 11.0%, being retired by 18.7%, 9.5% were studying and 3.1% were not employment status classified.
SF36V2 weights
Table 2 presents the percentage score scale means, standard deviations, 95% confidence intervals and the standard T-scores with 95% confidence intervals from both the US 1998 general survey and the Australian SAHOS survey, for each of the 8 scales. The key features are that on every scale the SAHOS mean percentage scores and the T-scores are higher than the US scores and that the Australian standard deviations for the percentage scores are smaller, with the exception of the GH and VT scales. That there are so few overlapping 95%CIs (they occur only for the PF and GH scales) suggests that there are significant differences between the US and SAHOS samples.
Table 3 shows the factor score coefficients for both the US and SAHOS data. The 95%CIs for the Australian coefficients were computed through bootstrapping as described in the Methods section. The results indicate that the US-reported coefficients all fall outside the bootstrapped 95%CIs for the Australian coefficients. This finding suggests that, although the differences appear to be small, they are important. When these two sets of factor coefficients were used to compute the summary scales, there were statistically significant differences in the resulting z-scores. The median for the US-weighted PCS was 0.33 (IQR: 1.08) compared with 0.30 (IQR: 0.14) for the Australian-weighted z-score (Wilcoxon z = −6.13, P < 0.01), while for the MCS the values were 0.53 (IQR: 0.92) and 0.29 (0.90), respectively (Wilcoxon z = −8.14, P < 0.01). The differences in the coefficients imply the relative weighting of the 8 scales within the PCS and MCS scoring systems may be different between the two countries. There are also differences in RP and VT coefficients used in scoring the MCS, and for BP and VT coefficients used in scoring the PCS.
Table 2 compares T-scores from the Australian SAHOS with the US data, based on using the US weights reported in Table 3. The differences in T-scores ranged from 0.50 (GH scale) to 3.18-points (MH scale). That there is no overlap in the 95%CIs for 8/10 scales suggests there are differences between Australian and US data on the SF36V2. The two scales where there is overlap are GH and the PCS summary scale.
Population norms
The findings reported in Tables 2 and 3 suggest there are differences between the US and Australia, therefore the Australian population normed T-scores for the 8 scales were derived from the Australian percentage score data in Table 2, and the two summary scales were weighted with the Australian coefficients reported in Table 3. This has been done for the reporting of Australian population norms in Tables 4–6.
Table 4 provides Australian normed mean T-scores for the 8 SF36V2 scales, by gender and the same 10-year age groups reported in the SF36V1 population norms study [26]. A key feature of the table is that on 7/8 scales, males obtained significantly higher scores, indicating better health, when compared with females (Kruskall–Wallis χ2 range = 26.72–4.69, all P < 0.001). The exception was for GH where there was no significant difference by gender (χ2 = 1.80, P = 0.18). For age group there were significant differences on all 8 scales (χ2 range = 13.28–06.74, all P < 0.05).
For the two summary scales, there were significant gender effects on both the PCS and MCS with males obtaining significantly higher scores than females (χ2 PCS = 37.83, χ2MCS= 20.89 both P < 0.001). When examined by age group, there were significant differences on both the PCS and MCS (χ2 PCS = 477.99, χ2 MCS = 182.97, both P < 0.001).
Table 5 shows SF36V2 T-scores by deciles and the proportion of cases which fell within each decile, where a decile is defined as 10% of the actual obtained T-score range. As shown, there were substantial ceiling effects. For example, for RE 79% of all cases fell within the top decile; indeed, on 4 of the 8 scales more than 50% of cases fell within the top decile. Conversely, there is almost no evidence of a floor effect. No scale had more than 3% of cases in the bottom decile. Finally, no cases fell within the 4th and 9th deciles for BP and within the 6th decile for SF. The reason is that these two scales have very limited scoring ranges (the ranges are 10 and 8 points, respectively (Table 6.11 in [7])).
Table 5 also shows deciles for the two summary scales. Although the data were skewed (33% of cases on the PCS and 36% of cases on the MCS fell within the 7th decile), there was no evidence of a floor effect and there was little evidence of ceiling effects.
The effect of health status by gender is reported in Table 6. This reveals a monotonic decline for both males and females across all 8 SF36V2 scales and on the two summary scales. It should be noted that the magnitude of decline increases with poorer health levels.
Discussion
This paper presents Australian weighted population norms for the SF36V2 using the identical methods to those of the instrument developers. Although there are good reasons to suggest alternative methods of scoring the SF36 instruments [16], the methods used here ensure compatibility with the US data published by Ware et al. [7].
This work advances the field as other recent population-based validation studies did not report norms or proportions within deciles for the SF36V2 nor comment on issues arising from scoring it. These data are pertinent for researchers as current SF36V2 data that are available for researchers and policy makers are based on US values derived in 1990. There may be good reasons for the derivation of local weights in other countries or communities because ‘health–assessment is a function of the cultural milieu within which a person lives and their own health expectations as well as their actual health status [35]. Where there is evidence of such emic effects, the collection of self-report data without adjustment for these effects will lead to misleading estimates of health. Comparisons of such estimates between countries or communities will be misleading since variations may be due to cultural factors rather than real health differences. Where researchers have good reason to suspect such local effects, the use of local weights may assist because the weights will adjust the data for emic effects. An example is the recent papers describing differences between the UK and the US weights for the EQ5D: the mean difference in health state values was 10% of a life-death scale. Consequently it was recommended that when used in the US, US-derived weights should be used [36–39].
In the current paper, it has been assumed that there is a degree of cultural homogeneity among Australians regarding the concept of health. It may be that this assumption is flawed and that different groups within Australian society have different understandings of health (e.g., some ethnic groups or the Aborigines). Where this is the case, it may be appropriate to derive community weights and norms rather than use those presented in this paper.
The results have been presented in considerable detail, by age group, gender, and respondent health status, and also by percentage scores as well as the recommended T-scores and by T-score decile. The reason for this is to assist researchers as much as possible by illuminating the distribution of SF36V2 scores.
As shown in Tables 2 and 3 there are small but potentially important differences between the percentage scores, factor coefficients and normed scores obtained in the SAHOS survey when compared with the US norms published in the SF-36V2 manual [7]. If there were no differences in Tables 2 and 3 then the standard US scoring algorithm could be accepted. The source of these differences is unknown and the critical issue is to correctly interpret these differences with respect to both the use of the SF26V2 and the computation of population norms. Although the SF36V2 developers have argued that it is an international version, the weights behind both the items and scales were derived from US samples. Of concern is the current use in the SF36V2 of the 1990 factor weights for computing the PCS and MCS summary scales given that Ware et al. report there were significant differences between the 1990 and 1998 surveys, including sampling bias in 1990 [7].
Whether these US weights should be accepted as the international standard is open to discussion. Generally, there are two approaches to the problem of cross-cultural equivalence. On the one hand it has been argued that rigorous approaches to translation and reliability across cultures ensure cross-cultural equivalence. This is essentially the position adopted by the IQOLA group and the SF36 developers [9, 34, 40]. The difficulty is that the descriptive system itself and the scoring weights may be culture-bound and therefore inappropriate in some societies (e.g., where walking is the transportation norm). The alternative position is that for cross-culture validity the descriptive system of a measure must be internationally developed, but perhaps scored with local variations. This is the position behind the World Health Organization’s WHOQOL instruments [41–43].
From these arguments it seems that ’health status–may be a social construct in which a person’s health function assessment is made within a cultural understanding of health [44]. Where a common descriptive system is used across countries, cultural differences in the understanding of health can be adjusted through the use of locally derived population-based weights. (This argument only applies where population-based weights are available, it would not apply where, say, weights were derived from a clinical sample.)
Although there may be theoretical reasons for local population weights, the more practical issue is whether the differences between countries are sufficient to warrant using locally derived weights. Ware et al. [45] argued that for the SF36V1, based on correlations between US-weighted and local country weighted scores, US weights should be used. In the present study, although the correlations between scores (US versus Australian weighted PCS and MCS; r s = > 0.98) were similar to those reported by Ware et al. for the SF36V1 the mean score 95% confidence intervals did not overlap for 8/10 SF36V2 scales (Table 2) suggesting that correlation analysis may not be the best test for demonstrating equivalence. In the current study the absolute differences, based on using US weights and the percentage scale, range from 1.05 (General Health) to 5.64 points (Mental Health) (Table 2). Depending upon health condition, the range of clinically important differences reported in the literature on the percentage scale is between 2 and 20 points for the SF36V1 [46–52]. If Ware et al.’s standard of 5-points for the SF36V1 is used as a criterion [1], there would be clinically important differences on the BP (5.12 point difference) and MH scales. It would seem, therefore, that the differences between the US and Australian norms are probably minimal on some scales (e.g., the GH scale) but important on other scales (e.g., the MH scale).
These findings suggest there is a prima facie case for local weights to be used in scoring the SF36V2, at least in Australian samples, although more research is needed on this matter. This is also the position that Jenkinson et al. implicitly supported in their UK validation study of the SF36V2 where British factor coefficients were presented [23].
With regards to individual item response weights, referred to as ‘recalibration–in the SF36V2 manual, the procedures and values were replicated in the IQOLA international studies [17]. These weights have been accepted in the present analyses in the interest of consistency with previous ABS population norms for the SF36V1 [26].
For the coefficient weights, the data suggest there are differences between the US and Australian samples (Table 3). The source of these differences is unknown and it could be any of the following (or several in combination): differences in the population samples (quota sampling based on age, gender and income in the US versus list sampling based on geographic location in the SAHOS), demographic characteristics (e.g., gender, age, birth country, race, education); differences in data collection procedures (mail administration in the US versus interview in the SAHOS); differences in time when the data were collected (1998 vs. 2004); differences in health status; or differences in cultural perception of the items and the meaning of health. Collectively, these suggest there may be emic differences between the US and Australia that, although small, are important in assessing health function status.
The use of local means and standard deviations, however, does have ramifications for the calculation of T-scores. Although T-scores enable the comparison of different tests on a common metric, they do not remove skew from data. T-scores are based on estimates of data distribution, as such the range of T-values are a function of the variance expressed as the standard deviation. Whilst this is not a problem where data are normally distributed, it artificially inflates reported score ranges where there are ceiling or floor effects which limit the standard deviation. This is the case with several of the SF36V2 scales (see Table 5). This may relate to the health status of a sample (e.g., most people are actually well placed at the healthiest end of the scale) or it may relate to inadequate item response options (such that respondents are forced to score at the ends of scales irrespective of their health status). These artefacts may lead to a high proportion of respondents obtaining a ceiling-score, leading to an artificially low spread of the data (i.e., a narrow standard deviation) and a consequent inflated score range when presented as a T-score. Because it is the convention to report mean SF36V2 scores (see Tables 2, 4 and 6), these effects (ceiling restricted standard deviations and skew) are largely hidden whereas they are overt in the decile table (Table 5). The ceiling effects and non-normal distributions compromise the usefulness of T-scores and may render scales unresponsive to change and group comparison–two key uses of the SF36V2.
In the case of the Australian scores presented in this paper, based on the means and standard deviations presented in Table 2, it was observed that the standard deviations were smaller than those reported by Ware et al. [7]. This is almost certainly due to the higher mean scores obtained from the SAHOS restricting the standard deviations because of ceiling effects. Conversion to T-scores may cause the scores of a substantial proportion of individuals with moderately poor health to be so low that they are interpreted as suffering poor health because these cases–scores are based on the number of standard deviations away from the mean. In this setting, individuals might be a small distance from the population mean in terms of original item endorsement, but a long distance in T-scores. Comparison of T-scores in pre-post studies may lead to overestimates of intervention effect. This is of concern as clinical and public policy decisions may well be influenced by conclusions based on these scales.
This statistical phenomenon partly explains the highly skewed data distributions reported in Table 5 which are not obvious when means are reported, as in Tables 2 and 4. When these results are compared with the results for two other patient-outcome measures which have had Australian norms reported recently, the WHOQOL-Bref [53] and the Assessment of Quality of Life [AQoL, 54] instruments, skewed data and ceiling effects appear to be more of a problem for the SF36V2 scales (the proportion of cases in the top decile was between 6% and 79%; half of the SF36V2 scales had > 50% of cases in the top decile): for the WHOQOL-Bref the proportion in the top decile ranged from 14% to 17%, and it was 45% for the AQoL. Researchers and policy makers looking for more reliable data at healthy ends of the spectrum might consider these other measures.
Australian population norms are presented in Table 4. To enhance the usefulness of the data, they have been presented by age group and gender, in accordance with the IQOLA recommendations [34]. Broadly, the SF36V2 scales map what is expected and known about the Australian population health status. Overall, physical health declines with age and mental health follows a slight U-shape where the youngest and oldest reported the highest scores. A potential limitation is that South Australia receives fewer migrants than the eastern Australian states (Victoria, New South Wales and Queensland) and thus has a more homogenous population [55]. Any generalizability threat arising from this, however, is unlikely given that the data were weighted to achieve representativeness by 2001 Census and 2002 Estimated Resident Populations as described in the Methods section.
Table 6 presents mean (SDs) scores by self-reported health status, based on the health status question from the HUI-3 [30]. In all cases there was a monotonic decline, which accelerated with poorer health status.
Conclusion
Although many researchers may still be using the SF36V1, particularly in longitudinal studies, the use of the SF36V2 may rise rapidly. Given that this is claimed to be an international instrument, it is important that the descriptive system and weights used can be demonstrated to be independent of cultural effects. If there are emic effects, then local population weights should be published and used.
Although this study was not a validation of the SF36V2 per se the findings suggest that there are important differences between the US samples used for the SF36V2 weights and the Australian sample reported here. Consequently, local weights were derived and have been used to report local population norms. A computer algorithm with the Australian weights is available from the authors. It should also be noted that the SF36V2 is copyright, and that researchers must purchase a licence from the developers.
Population norms provide guidelines for interpreting SF36V2 scores. When available by age group, gender or instrument decile, these are particularly useful for describing populations, providing benchmarks for the proportion of cases returned to good or full health, or they may provide yardsticks against which the effectiveness of interventions can be assessed.
References
Ware, J. E., Snow, K. K., Kosinski, M., & Gandek, B. (1993). SF-36 health survey: Manual and interpretation guide. Boston: The Health Institute, New England Medical Centre.
Sanson-Fisher, R. W., & Perkins, J. J. (1998). Adaptation and validation of the SF-36 health survey for use in Australia. Journal of Clinical Epidemiology, 51, 961–67.
McCallum, J. (1994). The new ‘SF-36–health status measure: Australian validity tests. Canberra: National Centre for Epidemiology and Population Health. 2.
McCallum, J. (1995). The SF-36 in an Australian sample: Validating a new, generic health status measure. Australian Journal of Public Health, 19, 160–66.
Perkins, J. J., & Sanson-Fisher, R. W. (1998). An examination of self- and telephone-administered modes of administration for the Australian SF-36. Journal of Clinical Epidemiology, 51, 969–73.
Watson, E., Firman, D., Baade, P., & Ring, I. (1996). Telephone administration of the SF-36 health survey: Validation studies and population norms for adults in Queensland. Australian and New Zealand Journal of Public Health, 20, 359–63.
Ware, J. E., Kosinski, M. A., & Dewey J. E. (2000). How to score version 2 of the SF-36 health survey. Lincoln: Quality Metric Inc.
Andresen, E., Patrick, D., Carter, W., & Malmgren, J. (1995). Comparing the performance of health status measures for healthy older adults. Journal of the American Geriatrics Society, 43, 1030–034.
Bullinger, M., Alonso, J., Apolone, G., Leplège, A., Sullivan, M., Wood Dauphinee, S., Gandek, B., Wagner, A., Aaronson, N., Bech, P., Fukuhara, S., Kaasa, S., & Ware, J.E. Jr. (1998). Translating health status questionnaires and evaluating their quality: The IQOLA Project approach. International Quality of Life Assessment. Journal of Clinical Epidemiology, 51, 913–23.
Hayes, V., Morris, J., Wolfe, C., & Morgan, M. (1995). The SF-36 health survey questionnaire: Is it suitable for use with older adults? Age and Ageing, 24, 120–25.
O’Mahoney, P. G., Rodgers, H., Thomson, R. G., Dobson, R., & James, O. F. W. (1998). Is the SF-36 suitable for assessing health status of older stroke patients? Age and Ageing, 27, 19–4.
Parker, S. G., Peet, S. M., Jagger, C., Farhan, M., & Castleden, C. M. (1998). Measuring health status in older patients. The SF-36 in practice. Age and Ageing, 27, 13–8.
Jenkinson, C. (1995). Evaluating the efficacy of medical treatment: possibilities and limitations. Social Science & Medicine, 41, 1395–401.
Adamson, J., Gooberman-Hill, R., Woolhead, G., & Donovan, J. (2004). ‘Questerviews– Using questionnaires in qualitative interviews as a method of integrating qualitative and quantitative health services research. Journal of Health Services Research & Policy, 9, 139–45.
Ziebland, S. (1995). The short form 36 health status questionnaire: Clues from the Oxford region’s normative data about its usefulness in measuring health gain in population surveys. Journal of Epidemiology and Community Health, 49, 102–05.
Wilson, D., Parsons, J., & Tucker, G. (2000). The SF-36 summary scales: Problems and solutions. Sozial- und Präventivmedizin, 45, 239–46.
Keller, S. D., Ware, J. E. Jr., Gandek, B., Aaronson, N. K., Alonso, J., Apolone, G., Bjorner, J. B., Brazier, J., Bullinger, M., Fukuhara, S., Kaasa, S., Leplège, A., Sanson Fisher, R. W., Sullivan, M., & Wood Dauphinee, S. (1998). Testing the equivalence of translations of widely used response choice labels: Results from the IQOLA Project. International Quality of Life Assessment. Journal of Clinical Epidemiology, 51, 933–44.
Bjorner, J. B., Damsgaard, M. T., Watt, T., & Groenvold, M. (1998). Tests of data quality, scaling assumptions, and reliability of the Danish SF-36. Journal of Clinical Epidemiology, 51, 1001–011.
Razavi, D., & Gandek, B. (1998). Testing Dutch and French translations of the SF-36 health survey among Belgian angina patients. Journal of Clinical Epidemiology, 51, 975–81.
Kazis, L. E., Lee, A., Spiro, A. 3rd, Rogers, W., Ren, X. S., Miller, D. R., Selim, A., Hamed, A., & Haffer, S. C. (2004). Measurement comparisons of the medical outcomes study and veterans SF-36 health survey. Health Care Financing Review, 25, 43–8.
McCall, W. A. (1922). How to measure in education. New York: Macmillan.
Taft, C., Karlsson, J., & Sullivan, M. (2004). Performance of the Swedish SF-36 version 2.0. Quality of Life Research, 13, 251–56.
Jenkinson, C., Stewart-Brown, S., Petersen, S., & Paice, C. (1999). Assessment of the SF-36 version 2 in the United Kingdom. Journal of Epidemiology and Community Health, 53, 46–0.
Morfeld, M., Bullinger, M., Nantke, J., & Brahler, E. (2005). Die version 2.0 des SF-36 Health Survey–Ergebnisse einer bevölkerungsrepräsentativen studie. Sozial- und Präventivmedizin, 50, 292–00.
Sansoni, J., & Costi, J. (2001). SF-36: Version 1 or Version 2: The need for Australian normative data. Proceedings of Health Outcomes 2001: The Odyssey Advances Conference. Canberra: Australian Health Outcomes Collaboration.
ABS (1997). National Health Survey: SF-36 Population Norms, Australia. Canberra: Australia Bureau of Statistics.
Harrison Health Research (2004). Findings from Autumn 2004 Health Omnibus Survey. Adelaide, Harrison Health Research.
Wilson, D., Wakefield, M., & Taylor, A. (1992). The South Australian Health Omnibus Survey. Health Promotion Journal of Australia, 2, 47–9.
Ware, J., Kosinski, M., & Keller, S. (1994). SF-36 physical and mental health summary scales: A user’s manual Boston: The Health Institute, New England Medical Centre.
Feeny, D., Furlong, W., & Torrance, G. (1996). Health Utilities Index Mark 2 and Mark 3 (HUI2/3) 15-item questionnaire for self-administered, self-assessed usual health status. Hamilton: Centre for Health Economics and Policy Analysis, McMaster University.
ABS (2005). 3235.4.55.001–Population by age and sex, South Australia Canberra: Australian Bureau of Statistics.
ABS (2003). 2001.0–Census of population and housing: Basic community profiles, 2001 Canberra.
SPSS (2004). SPSS for Windows, Version 13.0 Chicago: SPSS Inc.
Gandek, B., & Ware, J. E. Jr. (1998). Methods for validating and norming translations of health status questionnaires: The IQOLA Project approach. International Quality of Life Assessment. Journal of Clinical Epidemiology, 51, 953–59.
Patrick, D. L., Bush, J. W., & Chen, M. M. (1973). Toward an operational definition of health. Journal of Health and Social Behavior, 14, 6–3.
Shaw, J. W., Johnson, J. A., & Coons, S. J. (2005). US valuation of the EQ-5D health states: Development and testing of the D1 valuation model. Medical Care, 43, 203–20.
Havranek, E. P., & Steiner, J. F. (2005). Valuation of health states in the US versus the UK: Two measures divided by a common language? Medical Care, 43, 201–02.
Johnson, J. A., Luo, N., Shaw, J. W., Kind, P., & Coons, S. J. (2005). Valuations of EQ-5D health states: Are the United States and United Kingdom different? Medical Care, 43, 221–28.
Fryback, D. G. (2005). A US valuation of the EQ-5D. Medical Care, 43, 199–00.
Ware, J., Gandek, B., & Keller, S. (1996). Evaluating instruments used cross-nationally: Methods from the IQOLA project. In B. Spilker (Ed.), Quality of Life and Pharmacoeconomics (pp. 681–92). Philadelphia: Lippincott-Raven Publishers.
Skevington, S. M., Sartorius, N., & Amir, M. (2004). Developing methods for assessing quality of life in different cultural settings. The history of the WHOQOL instruments. Social Psychiatry and Psychiatric Epidemiology, 39, 1–.
Szabo, S., Orley, J., Saxena, S., & The WHOQOL Group. (1997). An approach to response scale development for cross-cultural questionnaires. European Psychologist, 2, 270–76.
WHOQoL Group (1995). The World Health Organization Quality of Life Assessment (WHOQOL): Position paper from the World Health Organization. Social Science & Medicine, 41, 1403–409.
Wee, H. L., Li, S. C., Cheung, Y. B., Fong, K. Y., & Thumboo, J. (2006). The influence of ethnicity on health-related quality of life in diabetes mellitus: A population-based, multiethnic study. Journal of Diabetes and its Complications, 20, 170–78.
Ware, J. E. Jr., Gandek, B., Kosinski, M., Aaronson, N. K., Apolone, G., Brazier, J., Bullinger, M., Kaasa, S., Leplege, A., Prieto, L., Sullivan, M., & Thunedborg K. (1998). The equivalence of SF-36 summary health scores estimated using standard and country-specific algorithms in 10 countries: Results from the IQOLA Project. International Quality of Life Assessment. Journal of Clinical Epidemiology, 51, 1167–170.
Angst, F., Aeschlimann, A., & Stucki, G. (2001). Smallest detectable and minimal clinically important differences of rehabilitation intervention with their implications for required sample sizes using WOMAC and SF-36 quality of life measurement instruments in patients with osteoarthritis of the lower extremities. Arthritis Care and Research, 45, 384–91.
Kosinski, M., Zhao, S. Z., Dedhiya, S., Osterhaus, J. T., & Ware, J.,E. Jr. (2000). Determining minimally important changes in generic and disease-specific health-related quality of life questionnaires in clinical trials of rheumatoid arthritis. Arthritis and Rheumatism 43, 1478–487.
Ahroni, J. H., & Boyko, E. J. (2000). Responsiveness of the SF-36 among veterans with diabetes mellitus. Journal of Diabetes and its Complications, 14, 31–9.
Spiegel, B. M., Younossi, Z. M., Hays, R. D., Revicki, D., Robbins, S., & Kanwal, F. (2005). Impact of hepatitis C on health related quality of life: A systematic review and quantitative assessment. Hepatology, 41, 790–00.
Wiebe, S., Matijevic, S., Eliasziw, M., & Derry, P. A. (2002). Clinically important change in quality of life in epilepsy. Journal of Neurology, Neurosurgery, and Psychiatry 73, 116–20.
Quintana, J. M., Escobar, A., Bilbao, A., Arostegui, I., Lafuente, I., & Vidaurreta, I. (2005). Responsiveness and clinically important differences for the WOMAC and SF-36 after hip joint replacement. Osteoarthritis and Cartilage, 13, 1076–083.
Bessette, L., Sangha, O., Kuntz, K. M., Keller, R. B., Lew, R. A., Fossel, A. H., & Katz, J. N. (1998). Comparative responsiveness of generic versus disease-specific and weighted versus unweighted health status measures in carpal tunnel syndrome. Medical Care, 36, 491–02.
Hawthorne, G., Herrman, H., & Murphy, B. (2006). Interpreting the WHOQOL-Brèf: Preliminary population norms and effect sizes. Social Indicators Research, 77, 37–9.
Hawthorne, G., & Osborne, R. (2005). Population norms and meaningful differences for the Assessment of Quality of Life (AQoL) measure. Australian and New Zealand Journal of Public Health, 29, 136–42.
ABS (2002). Year Book Australia. Canberra: Australian Bureau of Statistics.
Acknowledgements
We would like to thank Mr Nick Marosszeky for his valuable comments on the manuscript.
This project was supported by a grant from the Community Care Branch, Australian Commonwealth Department of Health and Ageing, as part of the National Continence Management Strategy. The collection of data was carried out by the Population Research and Outcome Studies Unit, South Australian Department of Health. Dr Richard Osborne is supported in part by the Baker Trust, Buckland Foundation, the Arthritis Foundation of Australia, and by the National Health and Medical Research Council Career Development Award Population Health Fellowship.
Finally, our thanks go to those South Australians who gave their time to complete the questionnaire.
Author information
Authors and Affiliations
Corresponding author
Rights and permissions
About this article
Cite this article
Hawthorne, G., Osborne, R.H., Taylor, A. et al. The SF36 Version 2: critical analyses of population weights, scoring algorithms and population norms. Qual Life Res 16, 661–673 (2007). https://doi.org/10.1007/s11136-006-9154-4
Received:
Accepted:
Published:
Issue Date:
DOI: https://doi.org/10.1007/s11136-006-9154-4