Abstract
Cities and towns along the tidal Hudson River are highly vulnerable to flooding through the combination of storm tides and high streamflows, compounded by sea level rise. Here a three-dimensional hydrodynamic model, validated by comparing peak water levels for 76 historical storms, is applied in a probabilistic flood hazard assessment. In simulations, the model merges streamflows and storm tides from tropical cyclones (TCs), offshore extratropical cyclones (ETCs) and inland “wet extratropical” cyclones (WETCs). The climatology of possible ETC and WETC storm events is represented by historical events (1931–2013), and simulations include gauged streamflows and inferred ungauged streamflows (based on watershed area) for the Hudson River and its tributaries. The TC climatology is created using a stochastic statistical model to represent a wider range of storms than is contained in the historical record. TC streamflow hydrographs are simulated for tributaries spaced along the Hudson, modeled as a function of TC attributes (storm track, sea surface temperature, maximum wind speed) using a statistical Bayesian approach. Results show WETCs are important to flood risk in the upper tidal river (e.g., Albany, New York), ETCs are important in the estuary (e.g., New York City) and lower tidal river, and TCs are important at all locations due to their potential for both high surge and extreme rainfall. The raising of floods by sea level rise is shown to be reduced by ~ 30–60% at Albany due to the dominance of streamflow for flood risk. This can be explained with simple channel flow dynamics, in which increased depth throughout the river reduces frictional resistance, thereby reducing the water level slope and the upriver water level.
Similar content being viewed by others
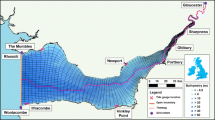
Avoid common mistakes on your manuscript.
1 Introduction
Both storm tides and rainfall can cause floods along the tidal Hudson River (Orton et al. 2012). Rainfall and storm surge frequently co-occur, and this frequency has been increasing at some US locations including New York City (Wahl et al. 2015). Hurricane Irene made landfall in August 2011 at New York City at only tropical storm intensity, with a maximum sustained wind speed of 28 m s−1. However, its inland impacts were severe, due to extremely heavy rainfall totals of 0.10–0.25 m in the Hudson River Valley (Orton et al. 2012; Strachan 2012). Hurricane Sandy caused record-breaking storm tides in 2012 for New Jersey and New York. At the seaward end of the Hudson (the Battery, New York City), the peak storm surge was 2.74 m and the peak storm surge plus tide was 3.39 m above mean sea level (e.g., Orton et al. 2016a; Wang et al. 2014). Sandy’s storm surge at that location was exceeded by Category-3 hurricanes in 1788 (3.0 m) and 1821 (3.4 m) (Orton et al. 2016a), and Irene’s peak streamflow of 3300 m3 s−1 from the Mohawk River into the Hudson was exceeded by an undated 1860s flood estimated at 5700 m3 s−1 (U.S. Geological Survey 2016), showing that history has proven that worse events can occur. However, no comprehensive flood hazard assessment has been conducted to evaluate probabilities from rainfall and storm tides along the Hudson.
As a result of the severe impacts of Irene and Sandy, there has been a strong interest in State and Federal government to develop better forecasting capabilities and a better understanding of flood probabilities for the New York region. This information can help the state prepare for coastal flood emergencies, plan coastal protection measures, and develop long-term plans for increasing the climate change resilience (e.g., Gibbs and Holloway 2013; Sobel 2014).
The three-dimensional hydrodynamic model and setup behind the operational New York Harbor Observing and Prediction System (NYHOPS; Georgas and Blumberg 2009; Georgas et al. 2016a, b) was applied by Orton et al. (2012) to study Hurricane Irene and examine the role of various physical processes in controlling peak water levels across the region. One of the most important findings was that model experiments with and without tributary freshwater inputs to the Hudson showed that omitting freshwater inputs led to a low bias in peak flood levels of 2% at New York Harbor (NYH; the Battery tide gauge), growing to 10% in areas only 20 km upriver, and above 50% in the upper tidal river (Orton et al. 2012). Experiments involving omission of estuary and coastal ocean stratification in the model also led to a low bias of 6–13% in the Hudson’s estuarine region, including a 6% low bias at NYH. The results showed that, in order to develop an accurate model of Hudson River flooding, freshwater inputs and ocean and estuarine stratification should be taken into account.
Here, we extend the recent flood modeling and hazard assessment work by Orton et al. (2012, 2016a) for NYH up the Hudson River estuary and its tidal river reach (Fig. 1) by merging storm tides and storm-driven streamflows in the model simulations. The modeling is validated by comparing results with 76 historical events and is applied to calculate peak water levels produced by tropical and extratropical cyclones. For tropical cyclone rainfall-driven freshwater inputs to the Hudson, a quantile regression/Bayesian multivariate approach is used, translating the storm attributes (e.g., track, SST, wind speed) directly into tributary streamflows. The primary goal of the project is to compute flood hazards for the Hudson River floodplain for today and future decades, including storm surge, rainfall flooding and sea level rise. Once the climatology of storms is represented and the flood inundation hazard itself is quantified, this opens the door to adapting this multivariate approach to forecasting and adaptation studies (e.g., Cioffi and Gallerano 2003).
Hudson River study area, including a the modeled streamflow watersheds on a map of the Northeastern United States, b expanded view of the black box showing the tidal portion of the Hudson, from New York City to Troy, and c observed tide datums along the Hudson (Georgas et al. 2013). Red dots in b show water elevation measurement locations (Albany, Poughkeepsie, Battery) and the lead author’s research institution (Hoboken, at Castle Point). The tide datums shown are mean sea level for the 1983–2001 period (MSL), mean lower-low water (MLLW), and mean higher-high water (MHHW)
2 Background
2.1 Flood hazards in tidal river estuaries
Studies of storm surges have typically focused on estuarine regions and ocean-facing shorelines. Tidal river reaches have received far less attention. Tidal river reaches are defined here as the tidal portions of river systems. To perform a dynamic model-based flood hazard assessment in a river with a tidal reach, a model is required with both hydrological and physical oceanographic boundary conditions.
Dynamic modeling of flooding in tidal river estuary systems is becoming more common (e.g., Dresback et al. 2013; Georgas et al. 2016a; Golding 2009; Martyr et al. 2012), but there have been only a few studies that have assessed flood risk from merged storm-driven streamflows and storm tides. A study of Taiwan’s Gaoping River (Chen and Liu 2016) used an observation-based return period analysis of recent historical events (1989–2011) to develop a small set of storm scenarios (up to the 200-year flood) for simulation and flood mapping. A study for the Rhine River showed that there is a far greater chance of floods overtopping defenses with combined streamflow/surge events, versus separate streamflow or surge events (Zhong et al. 2013). In this case, a simplified approach was used with a joint probability analysis and a 1D channel model.
A 1D/2D coupled flood inundation model (FloodMap) was used to predict the river flow and flood inundation, and to investigate the interaction between sea level rise, land subsidence and storm tide induced fluvial flooding in the Huangpu river floodplain (Yin et al. 2013). A three-dimensional estuarine and coastal ocean model with an unstructured-grid framework and wetting–drying capability was applied to simulate the inundation processes and the dynamic interactions between the estuarine and river floodplain regimes in the Skagit River estuary and its upstream river floodplain of Puget Sound along the northwest coast of North America (Yang et al. 2012).
The present study of the Hudson River builds upon this prior work by leveraging a preexisting extremely detailed hydrodynamic model-based flood forecasting system and a probabilistic flood hazard assessment for the coastal region. The resulting detailed and thorough flood assessment demonstrates how different types of storms control flood risk at a range of upriver sites, as well as how sea level rise will influence these flood levels.
2.2 The Hudson tidal river estuary and its historical floods
The tidal Hudson River (Fig. 1) stretches 225 km from the Battery, NYH, to the Federal Dam at Troy, NY, with the estuarine portion typically covering the lower 30–90 km (up to latitude 41.0°–41.5°N), and tidal river reaches extending northward from there. The Hudson is a drowned river valley in a former fjord that was filled in with sediment as sea level rose during the Holocene (Geyer and Chant 2006). As such, it is topographically bounded with high ground, and the head of tide at Troy is at a lock/spillway system. The mean water level slope in the tidal Hudson is 2.5 mm per kilometer from Battery to Poughkeepsie, but steepens near Albany to 5.6 mm per kilometer (from Schodack Island to Albany) (Georgas et al. 2013), in either case a relatively low gradient. The Hudson’s daily tide range (MHHW-MLLW) is largest at the Battery and at Albany, and smaller in the middle regions as shown in Fig. 1c and previously observed and summarized by Geyer and Chant (2006). Mean annual minimum and maximum streamflows into the tidal Hudson at Troy are 90 and 2340 m3 s−1, respectively (Orton and Visbeck 2009), but storms have driven flow rates of up to 6100 m3 s−1.
In this study, the largest flood events from 1931 to 2013 at NYH and Albany are taken into account—the southern and northern ends of the tidal Hudson, respectively. The historical data are chosen to start at 1931 because that is the first year after the statewide system of reservoirs in New York was completed, most notably the Great Sacandaga Lake and the Conklingville Dam. Seven of the top-20 flood events at the Battery (NYH; Fig. 2, left panel) were of tropical cyclone (TC) origin. Extratropical cyclones (ETCs) cause more frequent flood events, but the historical rankings show an apparent ceiling value of about 2.2 m. The Albany record (Fig. 2, right panel) shows TCs to have been responsible for four of the top 20 events. A different type of event was defined based on the observation that many events were not TCs but were also not surge-inducing ETC events at the seaward end of the river system. (The storm surge was below 1 m at the Battery.) These events typically were rain-on-snow or rain-on-ice events in the cool season (December through May). They account for the other 16 of the top 20 events at Albany and are referred to hereafter as wet extratropical cyclones (WETCs).
Top-20 highest historical flood events from 1931 to 2013 at (left) Battery (New York Harbor, NYH) and (right) Albany. These have been a mixture of three different storm types: tropical cyclones (TCs), high-surge extratropical cyclones (ETCs) and high-streamflow “wet extratropicals” (WETCs). Annual mean sea level was subtracted from data at NYH, but is not available for each year at Albany
2.3 Tropical cyclone rainfall and streamflow modeling
As shown in Fig. 2, TCs occur only rarely in this study area, such that synthetic events must be simulated to assess flood risk (Orton et al. 2016a). Another challenge is that they have a very wide range of storm surge and rainfall. Rainfall prediction for landfalling tropical cyclones is complex since it requires linking tropical cyclone attributes (e.g., track, intensity, size), which depend on large-scale atmospheric circulation conditions, to local factors at the basin scale (e.g., orography, land cover). Most prior studies have focused on rainfall rather than the associated streamflow. The direct modeling of freshwater inputs, as a function of tropical cyclone attributes, has received less attention.
Konrad et al. (2002) analyzed how maximum mean precipitation amounts are related to the tropical cyclone attributes of strength, size, and speed of movement; Cerveny and Newman (2000) used a satellite-derived daily oceanic precipitation dataset, together with hurricane and tropical storm observations, to construct climate logical relationships between precipitation and common variables associated with tropical cyclones such as number of days since the origin of the tropical cyclone, latitudinal gradient in average tropical cyclonic rainfall, ratio of the center grid cell rainfall to the average tropical cyclonic rainfall, and maximum surface wind speed. Field and Wood (2007) analyzed composite mean fields and probability distribution functions (PDFs) of rain rate, cloud type and cover, cloud top temperature, surface wind velocity, and water vapor path (WVP) using satellite observations of mid-latitude cyclones from four oceanic regions. They found that, as vertical motions increase with cyclone strength, the rain rate and high-cloud fraction are both positively correlated with cyclone strength due to the increased flux of moisture upward in the cyclone. Rain rate is also strongly correlated with atmospheric moisture, which is in turn strongly correlated with the sea surface temperature. A Tropical Cyclone Rainfall Climatology and Persistence (R-CLIPER) Model has been developed by Marks and De Maria (2003). In such model, the rainfall climatology was reduced to a linear fit of the mean rainfall rates by radius (r) and time (t) after TC landfall. The statistical parameters were deducted by using hourly rain gages within 500 km of the storm center and global satellite-based TC rainfall climatology based on rain estimates from the NASA Tropical Rain Measurement Mission (TRMM) satellite (Lonfat et al. 2004), in particular the microwave imager (TMI).
The references in the previous paragraph are mainly attempting to forecast rainfall. However, rainfall forecasting is just a part of the problem of the quantification of the spatial structure of flooding over a region, since simulated rainfall fields have to be combined with hydrological and hydraulic models of runoff production and transport through the drainage network. This approach requires the implementation of hydrological models for flood hazard characterization which can be difficult to apply, especially in large regions. Beyond the difficulty in implementing hydrological models, inaccuracy in results may occur due to the paucity of rainfall data.
To overcome these limitations of hydrological models (Czajkowski et al. 2013) statistical data-driven approaches to flood hazard characterization based on discharge observations from streamflow measurements have to be devised (Villarini et al. 2014). Furthermore, probabilistic models for computing river discharge need to be integrated, exploring the causal mechanisms and main processes in the atmosphere, catchment and river system (Merz et al. 2014). Following the above examples, in this paper, a Bayesian Statistical Approach is developed consisting of the evaluation of (1) the peak discharge and its PDF as a function of TC characteristics; (2) the temporal trend of the hydrograph as a function of temporal evolution of the cyclone track, its intensity and the response characteristics of the specific basin. The approach is developed and applied to the New York region and to five sub-basins of the Hudson River (see Fig. 1).
3 Methods
A detailed operational ocean forecasting system was leveraged to run three-dimensional ocean simulations for the flood hazard assessment (Sect. 3.2). The general statistical framework for the study is the same framework that was used in the separate study of NYH (Orton et al. 2016a), but adding the additional storm type of WETCs (Fig. 2, right panel). That paper focuses on the oceanic end of the Hudson, where streamflows have a very small effect on flood risk. Here, we also explain our methods for streamflows that are not summarized in Orton et al. (2016b).
The statistical framework builds an annual rate distribution of water elevations for every location, using model simulations of observed historical events for ETCs and WETCs, and simulated synthetic extreme events for TCs so that rare hurricane events are included in the assessment (Sect. 3.1). The historical events are used to represent the climatology of ETCs and WETCs because they occur frequently and are relatively well represented by the historical events (e.g., FEMA 2014; Orton et al. 2016a). A joint probability method is used to build a representative climatology of TC events to represent the climatology and probabilities of TCs (Orton et al. 2016a). For WETCs, a Generalized Extreme Value distribution is fitted to the WETC rate distributions so that the flood elevations or map contours for a specific return period (from 5- to 1000-year return periods) can be interpolated (e.g., FEMA 2014). For ETCs, the empirical distribution is used, because rate distributions are relatively smooth and the tail is well represented by simulating each storm with many random tide scenarios. The above statistical treatments for TCs and ETCs are described in detail in Orton et al. (2016a). One difference is that, in that paper, the TC rate distribution was smoothed with a filter that varied with flood level, an approach that was tailored to one location (NYH) and based on 600 random storm and tide simulations. Here, we smooth the TC distribution with a Gaussian curve that has a spatially varying standard deviation, representing different random storm and tide variations for every grid cell along the Hudson, based on the results of the same set of random simulations.
A new addition to the TC assessment here beyond that prior paper is that there is one new free variable, streamflow, which is represented with three cases (Sect. 3.4): the 10th, 50th and 90th streamflow percentiles. The model runs are redone for several sea level rise scenarios, to study how sea level rise raises the flood levels and probabilities (Sect. 3.3). Validation is provided for each storm type, based on historical storms and measured water levels at NYH, Poughkeepsie and Albany, spaced along the Hudson, and presented in Sect. 4.1.
3.1 Storm climatologies and wind, pressure and tide forcing
A climatology of all possible TCs affecting the Hudson River was built using a statistical stochastic model of the complete life cycle of North Atlantic (NA) TCs (Hall and Yonekura 2013). It creates a set of synthetic TCs using the statistics of historical North Atlantic TCs (1900–2010). A comparison of the modeled and observed historical TC landfall return periods for classes of TCs up to Category-3 hurricanes (no Cat-4 TCs have occurred) shows that the TC model is unbiased—the model’s return period curve for landfall wind speed falls inside the 95% confidence range of observed historical return periods (Orton et al. 2016a). We note here that the prior paper utilized a TC simulation trained on TC data from 1950 to 2013 and a derived climatology of 606 storms (Orton et al. 2016a), whereas here we use a simulation trained on TC data from 1900 to 2010 and a climatology of 637 storms. The two studies results are nearly identical at NYH, with a difference of only 2 cm in the 100-year TC water level.
We utilize simple parametric equations to represent each storm’s wind and pressure forcing for our ocean model—the Holland pressure model (Holland 1980) and SLOSH wind model (Jelesnianski et al. 1992) (Fig. 3). A set of 30 historical ETCs was utilized to represent the ETC flood hazard, and meteorological reanalysis data were available for these storms from a prior FEMA study (FEMA 2014). Detailed summaries of the TC and ETC storm sets, methods for obtaining wind and pressure fields and other details are given in the separate paper (Orton et al. 2016a).
The WETC storm set was derived by ranking historical streamflows from 1931 to 2013 at Troy, New York, and choosing the top-41 events that have occurred in “cool season” which is from December through May. The number 41 was chosen to be half the dataset duration of 82 years, such that the ~ 2-year flood would be included, with the goal of resolving WETC floods with 5-year return periods and worse. The resulting minimum streamflow entering the tidal Hudson at Troy is 2700 m3 s−1 for these chosen events (total streamflow, including gaged flow plus ungagged flow estimates). Meteorology was not imposed, as the streamflows dominate the water elevations for these storms, and high-resolution meteorological data for the entire period are not available.
Tides were randomly selected from a time series of tides from 1900 to 2010, with one simulation with random tide for each TC, one for each WETC, and ten simulations with random tides for each ETC, where tides are a larger proportion of the total water level. Tides were included in the hydrodynamic model, imposed at the edge of the continental shelf as is done with the NYHOPS forecasting system (Georgas and Blumberg 2009).
3.2 Hydrodynamic modeling
The Stevens ECOM (sECOM) three-dimensional hydrodynamic model (Blumberg et al. 1999; Georgas and Blumberg 2009; Georgas et al. 2014; Orton et al. 2012) has been providing highly accurate operational storm surge forecasts on its NYHOPS grid for over a decade (New York Harbor Observing and Prediction System; http://stevens.edu/NYHOPS), with typical water level RMS errors of ~ 0.10 m (Georgas and Blumberg 2009), 0.15 m for Tropical Storm Irene (Orton et al. 2012, #5970), and 0.17 m for Hurricane Sandy (Georgas et al. 2014). The NYHOPS grid includes the Mid-Atlantic and Northeastern U.S. coastline from Maryland to Rhode Island and for flood hazard assessment studies is nested inside a NW Atlantic model grid captures the large-scale influence of winds from Nova Scotia to Cape Hatteras and out to ~ 2000 km distance offshore. Details of the ocean modeling, including drag coefficient parameterization, wave model coupling and tide forcing, are all summarized in Orton et al. (2016a).
3.3 Sea level rise
Three future sea level scenarios were evaluated, for 0.61, 1.22 and 1.83 m (2, 4 and 6 feet) above the base mean sea level of 1983–2001. In the model simulations, these are imposed with the tide and offshore surge time series as a sum, at the NYHOPS model grid offshore boundaries. Current projections from the updated New York State ClimAID report still show great uncertainty in future rates of sea level rise, with projections for the year 2100 ranging from 0.38 to 1.91 m (Horton et al. 2015); these are the 10th and the 90th percentile values at NYH. Our top value of 1.83 m approximately matches the high-end (90th percentile) projections of sea level rise at the year 2100.
3.4 Tributary streamflows
TC streamflow hydrographs were modeled using a statistical Bayesian approach (summarized below) to create streamflows for five tributaries spaced along the Hudson from north-to-south, and across it east-to-west (Table 1; Fig. 1). The chosen tributaries were the Upper Hudson (above lock 1; 11,966 km2), Mohawk (8837 km2), Wappinger (469 km2), Rondout (2849 km2) and Croton (935 km2). The TC streamflows are added to monthly median flows for each tributary, to capture base flow. Different approaches, such as ignoring base flow, make little difference to the flood levels of interest, as median base flow to the tidal Hudson in hurricane season is 150–190 m3 s−1, whereas the floods of interest are above 2000 m3 s−1.
For ETCs and WETCs, we used available historical streamflow data along the Hudson, including the Mohawk, Ft Edward, Hackensack, Passaic, Saddle, Raritan, Manalapan, Esopus, Rondout, Wallkill, Wappinger, Rahway, Croton and Hoosic Rivers. Where only daily data were available (typically prior to 1990), the USGS peak flow estimates for major flood events were inserted into the time series on the day of the peak, to avoid underestimating peak flows during the storms. For all three storm types, ungaged or unmodeled small-to-medium tributaries (the remainder of a total of 52 Hudson River and NYH freshwater inputs to the model) are estimated using the standard NYHOPS system of estimating streamflows based on nearest similar-sized watersheds and scaled by watershed area (Georgas 2010; Georgas and Blumberg 2009).
As mentioned in Sect. 2.2, the tidal Hudson is topographically bounded with high ground. Therefore, the main tributaries in the Hudson north of NYH come down relatively steep watersheds, and the model’s injection of streamflows from these watersheds is very similar to the actual physical system—tides should not influence their hydrological characteristics.
3.5 Statistical Bayesian approach to translate TC attributes to streamflows
The effects of TCs are not limited to coastal regions, but affect large areas away from the coast, and often away from the center of the storm; as a consequence, heavy rainfall associated with North Atlantic TCs can be an important ingredient in flood generation. Here, a statistical model is constructed that utilizes the parametric attributes of a TC that have been simulated by the TC model (Hall and Yonekura 2013) to create streamflow hydrographs due to runoff at specific points of the tributaries of the Hudson River, for use as inputs in flood simulations by the hydrodynamic model. A hydrograph is identified by the following variables (see Fig. 4): (a) the initial discharge Qinit, (b) the peak discharge, (c) the hydrograph shape, and (d) the timing of the peak discharge relative to TC timing. The latter is the time in which the TC low pressure center is at the minimum distance from the center of a given tributary basin. While the first variable is known, the others have to be obtained by modeling their link with TC attributes as described next.
A Bayesian simultaneous quantile regression approach (Reich and Smith 2013) is used to translate the TC attributes (storm track, sea surface temperature—SST, maximum wind speed) into discharge peaks; a multivariate normal distribution is used to determine the time shift associated with the TC track; and lastly a k-nearest neighbor (KNN) method is employed to determine the hydrograph shape. To construct the statistical model, we used HURDAT 2 data (http://www.aoml.noaa.gov/hrd/hurdat/Data_Storm.html) with climatological along-track SST data for 1938–2012 TCs. For each 6-h step, the HURDAT 2 dataset provides longitude and latitude of the TC low-pressure center and the maximum sustained wind speed, among other variables. From the dataset, we identified 103 hurricanes whose track passed within a radial distance of 400 km from NYH. Beyond this distance, the TC rainfall contribution drops significantly and can be assumed to be negligible (Frank 1977; Rodgers et al. 1994). Flow rate data are taken from USGS gauges, and only the streamflow data associated with TCs in the HURDAT dataset were used to build the statistical model.
3.5.1 Discharge peak evaluation
Empirical analysis of data, together with inferences deduced by the physical mechanisms of genesis, development and lysis of TCs (Emanuel 1991), suggest that the discharge peaks for a TC are higher if the track is closer to a river basin and if the energy content of the cyclone is higher. Qualitatively, TC energy can increase only if a TC is over the ocean, and it is more likely to increase if there are high SSTs along the storm track. Therefore, if part of a TC storm track is over land, the discharge peaks may be lower, and this decrease may be related to the total time the TC stays over land. Here, we assume TC energy is related to the integral of SST over the time interval when the track is over the ocean, from the time of generation until the time of minimum distance from the center of the river basin, D.
Thus, we can identify a set of TC variables significantly affecting the peak discharge:
-
(a)
the minimum distance D from the TC track to the center of the basin;
-
(b)
the maximum sustained wind speed Vmax at the time the TC reaches the minimum distance
-
(c)
the integral of SST along the time interval in which the track is over the ocean, from the time of generation until the time of minimum distance, ISST;
-
(d)
the total time over land along the TC track, “over-ground duration” (OGD).
The qualitative picture of the dependence of hydrograph peak discharge on the above identified TC attributes is shown in Fig. 5. From the 103 TCs of the HURDAT dataset, we selected only those corresponding to hydrographs whose peak discharge occurred within three days before the time of minimum distance of TC track from the watershed. Furthermore, we removed from the dataset incomplete TC tracks or hydrographs that do not show a clear dependence on TCs. After this analysis, the following numbers of TCs survived: 75 for the Croton watershed, 76 for the Mohawk, 82 for the Rondout, 61 for the Upper Hudson, 80 for the Wappinger.
Scatterplots of the relationship between normalized peak discharge and TC attributes. a Minimum distance from the TC track to the center of the watershed, b TC maximum sustained wind speed, c the sum of SST from t = 0 to t = tD, with 6-h time steps (iSST), and d over-ground duration, the sum of the days over the ground for the TC track from t = 0 to t = tD, with 6-h time step
Then, the discharge peak values of each basin were normalized to the maximum discharge peak of each basin, merged with the data of other basins, obtaining a single, more numerous and significant, dataset. Initially, a linear regression analysis was performed between the normalized discharge peaks and the variables quantifying the TC attributes, standardized by mapping each variable’s mean to zero and standard deviation to one. In Table 2, the slope of the regression line for each of TC variables is shown which confirms the qualitative trends shown in Fig. 5.
To model the dependence of peak discharge on the TC attributes, the TC variables are combined in a unique variable X:
where weights and exponents wi are all positive, the numerator contains quantities whose increase produces an increase in peak discharge, and in the denominator the contrary. According to such definition, increases in X lead to increases in the peak discharge. A Bayesian simultaneous quantile regression (Reich and Smith 2013) is applied to find the statistical relationship between the covariate X and the response y = ln(Q) (Q being the peak discharge). A quantile function \(q(\tau |X)\) defined as the function satisfying \(P(y < q(\tau |X)) = \tau\) is linearly related to the covariate \(X\;by\;q(\tau |X) = X\;\beta \left( \tau \right)\;{\text{where}}\;\beta \left( \tau \right)\) is a continuous function over quantile level \(\tau\), modeled as a linear combination of L basis functions
A prior for αi ensures that the quantile function corresponds to a valid density function, denoted \(f(y|X,a)\).
The Markov Chain Monte Carlo (MCMC) algorithm (Besag et al. 1995; Gelfand and Smith 1990; Tanner and Wong 1987) is then applied to identify the parameters (\(w_{i} |i\) = 1:10) and the \(\beta (\tau )\) as a function of a number of basis and density functions assumed a priori.
The results of the Bayesian simultaneous quantile regression for the Mohawk River are shown in Fig. 6. In the left panel is the relationship between parameter X (normalized between − 1 and 1) and peak discharge for the regression interpolation for the 10th (black), 50th (red) and 90th (green) percentile values of peak discharge. In the right panel of Fig. 6 is the probability distributions peak discharge as functions of the assigned values of X, where X = − 0.8 (black), X = 0 (red) and X = 0.8 (green). The left panel shows a positive correlation between X and the peak discharge for all quantile levels, so the variable X is a good predictor for peak discharge in the Mohawk. Similar results are found for the other four river basins. Furthermore, the highest quantile has a steeper slope. Therefore, the combined variable X is a stronger predictor of extreme peak discharge events than the median and other quantiles.
3.5.2 Hydrograph shape and timing of peak discharge
We assume that the hydrograph could be divided into two branches, an ascending branch with flow rate that increases till the peak, and a decreasing branch. The former depends on both the TC track and basin characteristics, while the latter mainly depends on basin characteristics. Next, the normalized flow rate vector and distance vector for each historical cyclone are calculated as:
here k = (1, T) is the time step and j = (1, N) is the jth cyclone.
Next, the steps of the procedure for the calculation of hydrograph shape are the following:
-
1.
A conditional distribution of the peak discharge qpeaksim is generated for each basin by Bayesian quantile regression as a function of the TC attributes and its track rsim(t);
-
2.
A peak discharge qpeaksim—having a predetermined percentile—from which the conditional distribution is sampled;
-
3.
From the dataset of historical peak discharges, Nc nearest neighbors hydrographs \(\tilde{q}_{{{\text{hist}},k,j}}\), whose peak discharge is closer to qpeaksim, are identified;
-
4.
The temporal axes of the Nc normalized historical cyclone tracks \(\tilde{r}_{{{\text{hist}},k,j}}\) and hydrographs \(\tilde{q}_{{{\text{hist}},k,j}}\) are shifted to have a common origin relative to the peak discharge;
-
5.
The normalized ascending branch of the hydrograph corresponding to qpeaksim is assumed equal to the trend of the normalized historical hydrograph \(\tilde{q}_{{{\text{hist}},k,j}}\) having the peak discharge qhist closer to qpeaksim;
-
6.
The normalized decreasing branch of the hydrograph after the peak—which depends mainly on the characteristics of the basin—is represented by a negative exponential function whose parameters are obtained by a best fitting of the normalized hydrographs of the Nc nearest neighbors;
The steps for the calculation of the peak discharge time shift are the following:
-
1.
The 25th, 50th and 75th percentiles of historical peak flows qhist are calculated, and four peak discharge classes are defined: qhist < 25th, 25th ≤ qhist ≤ 50th, 50th ≤ qhist ≤ 75th, and qhist > 75th;
-
2.
The class of peak discharge qpeaksim is identified as well as the TC normalized tracks and corresponding normalized hydrographs belonging to that class;
-
3.
Assuming that \(\tilde{q}_{{{\text{hist}},k,j}} ,\tilde{r}_{{{\text{hist}},k,j}}\) are normally distributed with, respectively, mean μ and v, standard deviation σ and τ, and correlation ρ = corr(\(\tilde{q}_{{{\text{hist}},k,j}} ,\tilde{r}_{{{\text{hist}},k,j}}\)), a joint probability function can be identified as:
$$f\left( {\tilde{q}_{{{\text{sim}},k}} ,\tilde{r}_{{{\text{sim}}.k}} } \right) = \frac{1}{{2\pi \sigma \tau \sqrt {1 - \rho^{2} } }}e^{{\left\{ { - \frac{1}{{2\left( {1 - \rho^{2} } \right)}}\left[ {\frac{{^{{\left( {\tilde{q}_{{{\text{sim}},k}} - \mu } \right)^{2} }} }}{{\sigma^{2} }} - 2\rho \frac{{\left( {\tilde{q}_{{{\text{sim}},k}} - \mu } \right)\left( {\tilde{r}_{{{\text{sim}},k}} - \upsilon } \right)}}{\sigma \tau } + \frac{{\left( {\tilde{r}_{{{\text{sim}},k}} - \upsilon } \right)^{2} }}{{\tau^{2} }}} \right]} \right\}}}$$(5)
For a known sequence of \(\tilde{r}_{{{\text{sim}}.k}}\), at each time step the joint probability distribution of \(\tilde{q}_{{{\text{hist}},k,j}}\) can be calculated and the time step k corresponding to max(\(\tilde{q}_{{{\text{hist}},k,j}}\)) obtained.
A small set of individually out-of-sample validations of the above-cited methods are shown in Fig. 7 for the Mohawk Watershed, with the observed (green) and simulated (red) hydrographs for TCs that have produced varying levels of peak discharge. In most of the cases and across a wide range of TC characteristics, the model captures both the correct temporal trend of the hydrograph as a function of the position in time of the TC center, and the shape of hydrograph, both important determinants of the flood volume during the event.
Out-of-sample validations were created for the hydrograph shape model using comparisons to four historical TC streamflow/discharge events from the Mohawk River, ranging from moderate (top) to high (bottom) discharge. The observed discharge is shown in green and modeled shown in red. The black dashed line is the distance of the TC from the center of the basin. Discharge is normalized by the peak during each event, and distance is normalized by the maximum distance from the watershed
3.5.3 Application of model to hydrodynamic modeling of TCs
Using the procedure described in the previous paragraph, we generate a large number of synthetic hydrographs to accompany the TC storm set. As an example, the synthetic series of hydrographs related to the 637 TCs for the Mohawk River is shown in Fig. 8.
Complete time series of synthetic Mohawk River (at Cohoes) storm-driven discharge for 637 synthetic storms, shown back-to-back, with flow rates for each percentile. Low (10th percentile) discharge events (red) typically involve no rainfall and no additional streamflow above the base flow. All these TC-driven flows are added to the median monthly flow for each tributary, to form a total streamflow for input to the hydrodynamic model
Three model runs are performed for each TC, each with a different percentile streamflow peak (10th, 50th and 90th percentile). In order to scale the annual rates and enter the modeled flood elevation results into the TC annual rate distributions, we consider these as mean values of flows belonging to three classes of probability (100-80, 80-20, 20-0). As a result, the storm annual rates are scaled by 0.2, 0.6 and 0.2, respectively, for the three classes. This should be a reasonable approximation to the complete spectrum of streamflows for each TC, balancing computational time (3 model runs per storm representing the range of possible TC streamflows) against possible error, and is similar to our representation of other variables with three classes (e.g., landfall angle, storm size, storm speed). The total number of storms then is multiplied by 3, equaling a total of 1911 TCs.
4 Results
Below, we show that our model gives accurate reproduction of historical flood levels for ETCs, TCs and WETCs (Sect. 4.1). We also present the probability distributions and exceedance probabilities (inverse return periods) for various levels of flooding, as well as the importance of each type of storm for the three locations along the Hudson (Sect. 4.2). Water levels are converted to be relative to a common datum, the NYH 1983–2001 mean sea level.
4.1 Water level modeling validations
Comparisons of historical observed and modeled temporal maximum water levels are shown for each storm type in Fig. 9, generally showing good agreement and helping quantify model error for our uncertainty analysis (described in Sect. 4.2). In each case, the actual streamflows and meteorological forcing methods used in the probabilistic assessment are utilized. In the case of the TCs, synthetic streamflows at the same percentile of the actual historical event for each river basin were used, and these were created using out-of-sample statistical modeling. The modeling is validated using a set of 30 ETC events (1950–2009), 12 historical TC events (1788–2012) and 41 WETC events (1940–2012). However, there is a limited number of validation data available at Albany due to relative unavailability of historical data prior to 2000 except for the peaks for the most extreme events, mainly WETCs. Details of the observational data and sources of parametric TC meteorological data and simplified radial wind and pressure models are discussed in Orton et al. (2016a).
Describing validation results in Fig. 9, for ETCs the Battery modeled mean bias is − 0.03 m and root-mean-square error (RMSE) is 0.19 m (30 events), whereas Albany bias is 0.02 m and RMSE is 0.14 m (5 events). For TCs, the Battery bias is 0.01 m and RMSE is 0.32 m (12 events), whereas at Albany bias is 0.21 m and RMSE is 0.54 m (5 events). At Battery, a majority of the storms is simulated very accurately, within 0.20 m, but Hurricane Sandy is overestimated by 0.55 m (15%) (Orton et al. 2016a). The events at Albany include two high-streamflow events, the hurricanes in 1938 and 2011 (Irene), with excellent model-observation agreement. The validation for the WETC water levels at Albany shows a mean bias of + 0.10 m and RMSE of 0.33 (18 events). Taking into account the uncertainty in observations—especially for the most intense storms—overall, the results show that the model is reliable and able to represent and quantify the complex hydrodynamics of the storm induced flows.
4.2 Probability distributions and flood/return period curves
Raw annual rate distributions were constructed from model results at each model grid cell for TCs, ETCs and WETCs (Fig. 10; blue bars). Using Albany as an example of the distribution shapes and densities, these show a raw TC rate distribution that is long-tailed, with many tail events from 4 to 6 m with annual rates of ~ 10−4 year−1. The raw ETC rate distribution has a narrow but short tail truncated with many events from 3 to 4 m but higher rates at 10−3 year−1. The raw WETC distribution has a long tail that is not as well resolved, with only two large events at rates of 0.012 year−1 (one event in the 83-year period from 1931 to 2013) with floods from 4.5 to 5.5 m. Rate distributions for the estuarine portion of the Hudson (NYH) are described in Orton et al. (2016a) as being resolved fairly smoothly with many events in the tails for both TCs and ETCs. The ETCs have a high rate of moderate flood events (~ 1–2 m) and a relatively short tail ending at ~ 2.7 m. The TCs have a bulk of events in the 1–2 m range, lower probabilities in general, but a very long tail which includes floods of nearly 6 m. The historical WETCs caused low peak water elevations at NYH (never in the top-20 events shown in Fig. 2), so modeled WETCs for that location are not described here. Smoothed TC and fitted WETC distributions are also shown in red in Fig. 10, and used below, but the raw ETC rate distribution is used here as with Orton et al. (2016a) and discussed at the top of Sect. 3.
Annual water level rate distributions at Albany for a ETCs, b TCs and c WETCs. Raw distributions are shown with blue bars, and the smoothed TC and fitted WETC distributions are shown with red lines. Note the y-axis range in the middle (TC) panel is different from the other two cases. Water levels are all relative to a common datum, NYH mean sea level
Exceedance probabilities (inverse cumulative rate distributions) for each storm type and location are merged to create the combined flood/return period curves. Figure 11 shows examples for three locations. Similar data are available for all grid cells within the model domain. Monte Carlo methods were used to assess the propagation of model error through the analysis, forming 95% confidence intervals on these results (methods described in Orton et al. 2016a). Bootstrapping was used for re-sampling storms to incorporate the uncertainty of the relatively limited ETC and WETC storm sets. Still-water elevation (SWE) map data for various return periods are created by interpolating data like that shown in Fig. 11 (black line) at every model grid cell.
At Albany, the return period curve for WETCs is nearly the same as for the combined curve (flooding from any type of storm), indicating the WETCs are the dominant storm type defining flood risk. The TC curve has a slightly larger slope than the WETC curve, however, and therefore, TCs have increasing importance with increasing return period. The ETC curve is far below the WETC curve, suggesting a low importance for ETCs at this location. Uncertainty grows large for longer return periods, and this is discussed in Sect. 5.2. The 10-year flood is 3.50 m (2.97–3.86 at 95% confidence), the 100-year flood is 5.07 m (4.03–6.28 m), and the 1000-year flood is 6.66 m (4.17–9.38 m), all relative to the Battery mean sea level datum
At NYH, flooding is defined by TCs and ETCs, with ETCs primarily controlling flood risk of shorter return periods, a cross-over at the 50-year return period, and return periods of 100 years and longer defined exclusively by TCs. The WETC curve is not shown here, as they have a negligible effect at NYH. The 10-year flood is 1.96 m (1.77–2.22 at 95% confidence), the 100-year flood is 2.75 m (2.50–3.10 m), and the 1000-year flood is 4.12 m (3.86–4.50 m).
At Poughkeepsie, flood risk is also defined by TCs and ETCs, with ETCs dominating the shorter return period events, and TCs dominating only for long return periods of 500 years and longer. However, uncertainty is large throughout and the curves have irregular bends as does the uncertainty shading. This suggests that it may be useful to incorporate more storms into the ETC climatology, or more random tide simulations, or smoothing of the rate distribution, which was not used here. The 10-year flood is 1.63 m (1.49–1.85 at 95% confidence), the 100-year flood is 2.70 m (2.19–3.08 m), and the 1000-year flood is 3.52 m (3.28–3.79 m). As mentioned in Sect. 2.2, the Hudson’s tides are actually smaller in the middle region than they are at Albany and NYH, and this helps explain why 10-year floods are lowest at Poughkeepsie (Geyer and Chant 2006).
4.3 Impacts of sea level rise
The same modeling and statistical analyses are used with results for each of the sea level rise scenarios, apart from one difference with WETCs. In the fitting of GEV distributions to the WETC rate distributions, we found that the fitting of the tail was not robust, and “wagged” with sea level rise, leading to non-robust results. As a solution, we fitted linear regressions to the model results with (y-axis) and without (x-axis) sea level rise, then applied the resulting slope with the baseline curve to estimate future floods at each return period.
Results for Poughkeepsie and NYH generally show linear superposition of sea level rise within some slight variation (Fig. 12). That is, a 3-m flood with 1 m of sea level rise results in approximately a 4-m water level. On the other hand, the results at Albany show large deviations from simple linear superposition of sea level rise (Fig. 13). Water levels for WETCs are overall lower than linear superposition with deviations from linear superposition increasing with the rate of SLR and with event magnitude. Water levels for ETCs are higher than superposition for shorter return periods but become similar to linear superposition for larger events, regardless of the SLR rate tested.
Change in water level for various amounts of sea level rise for (left) Poughkeepsie for ETCs, and (right) for NYH for TCs. In both cases, model results are very close to the static assumption (simple superposition of water level and sea level rise), and this was also the case for TCs at Poughkeepsie and ETCs at NYH
4.4 Still-water elevations and the online flood mapper/information tool
Final results for the flood zones with sea level rise are created for each model grid cell and mapped. These maps are available in an online flood and sea level rise mapper called the Hudson River Flood Impact Decision Support System (http://www.ciesin.columbia.edu/hudson-river-flood-map). A set of impact estimates are provided for each of the flood scenarios presented in the mapping tool. The impacts are divided into 3 categories: critical infrastructure, social vulnerability and natural resilience features. Detailed mapping methods and other components of that website are summarized in a technical report linked therein. Tools such as this can help inform municipal and regional planners in the face of high uncertainty in flood probabilities and future sea level rise.
5 Discussion and conclusions
Below, we discuss what the results indicate for various regions of the Hudson River and tidal river reaches and estuaries in general. We also contrast our results with other studies of the Hudson. Section 5.1 broadly considers the influence of sea level rise on tidal river flood hazards, examining prior studies, our evidence, and presenting dynamical explanations for our results. Section 5.2 discusses the application, accuracy and simplifications of our approach for flood hazard assessment from streamflow and storm tides. Section 5.3 concludes with examples of the utility of this type of analysis for decision-makers, in terms of adaptation designs, risk management and planning for climate change.
At Albany, the assessment results (Fig. 11) suggest a dominance of WETCs, where TCs contribute a small 0.025 year−1 probability to the 5-year (0.2 year−1) flood, and about 0.0017 year−1 to the probability of a 100-year (0.01 year−1) flood. The historical events (Fig. 2) suggest that TCs contribute a slightly larger fraction of the top-20 events in the past 84 years (4/20). This difference between roughly a 1/5 and 1/8 contribution is well within the large error bounds conveyed in the results, however.
For NYH and Poughkeepsie, our assessment results show ETCs and TCs are both important. The results suggest ETCs dominate shorter return periods, and TCs are more important for the longer return periods. For NYH, the historical events show ETCs to account for the most top-20 events, but TCs to account for the top two. Therefore, the assessment results are qualitatively consistent with historical observations, as demonstrated quantitatively at NYH with a deeper analysis of historical events that included the 1700s and 1800s (a).
Another recent study has evaluated the flood hazard for Albany—FEMA’s flood maps there show a 100-year flood elevation of 6.2 m, about 0.9 m higher than our result. Several studies have evaluated flood probabilities at NYH, finding widely diverging exceedance curves, as previously noted (Orton et al. 2016a). The 100-year water level at NYH has had estimates ranging from 2.44 m (Zervas 2013) to 3.50 m (FEMA 2014). Our result here of 2.72 m falls between these studies and is also within the large 95% confidence range (2.2–5.2 m) of an estimated exceedance curve based on historical data (Orton et al. 2016a).
5.1 Tidal river flood hazards and sea level rise
Most fundamentally, this paper demonstrates that sea level rise can impact tidal river reaches, extending its effects hundreds of kilometers inland (Fig. 12). Yet, the results also show that a simple assumption that sea level rise increases flood elevations equivalently in all locations (“static superposition”) may not be accurate at upriver locations, though this assumption is very good from Poughkeepsie southward. Importantly, sea level rise effects on storm-driven flooding may be ameliorated in areas and for storms when streamflows and the resulting hydraulic head control flood levels (Fig. 13). The raising of floods by sea level rise is shown to be reduced by ~ 30–60% at Albany due to the dominance of streamflow for flood risk.
A small number of prior studies have looked at climate change effects on tidal river flood risk. A study for the Rhine River (Zhong et al. 2013) used a simplified approach for flood hazard assessment with a joint probability analysis and a 1D channel model. They simulated three types of flood events in a Monte Carlo analysis, incorporated climate change effects on both peak streamflows and on sea levels, and found a worsening of future flood levels and frequencies. A study of Taiwan’s Gaoping River (Chen and Liu 2016) used an observation-based return period analysis of recent historical events (1989–2011) to develop a small set of storm scenarios (up to the 200-year flood) for simulation and flood mapping. They also concluded that sea level rise led to a larger flood area and depths in low-lying regions, but not in upriver locations where water levels are only influenced by the freshwater discharges. However, to our knowledge, no study has discussed the dynamics of sea level rise in tidal river reaches.
Here, we have also shown the range of influences of sea level rise along a tidal river system, but we will also discuss the dynamical reasons for these findings. At the seaward end (NYH), sea level rise has a nearly linear superposition effect on flood levels from storm surge events (Fig. 12). This result refers only to the harbor area around Manhattan and northward up the Hudson, not for the nearby New Jersey tributaries such as the Passaic and Hackensack Rivers, where streamflows can contribute to flooding much like in the Hudson (Saleh et al. 2016). The static (linear) superposition of sea level rise on flood levels at New York Harbor is unsurprising, because water depths are deep and thus a small change in depth (and therefore, frictional effects) should have little effect on storm surges and tides near the open ocean, in a deep-channel Harbor. Two prior studies have shown a nearly static effect of sea level rise in New York Harbor (Lin et al. 2012; Orton et al. 2015). At Poughkeepsie, there is a nearly static effect of sea level rise, though subtly higher water levels on average versus static (Fig. 12). This likely occurs because ocean tides (and surge) are propagating over 100 km up the Hudson through significantly deepened water due to sea level rise and therefore have less frictional damping and are larger once they reach Poughkeepsie.
In contrast, at the upriver end of our system, results at Albany show large deviations from a static sea level rise effect (Fig. 13), with water levels for WETCs being below the static assumption for all events, and water levels for ETCs being above the static assumption for smaller events. The likely explanation for the WETC result is that a deeper river has less of a frictional effect on a flood, which favors the escape toward the ocean of the river floodwater. That is, the sea level rise may cause higher water, but it also ameliorates the hydraulic head (water level slope) that is set up by the high streamflow, and in the end the total water level is less than the sum of the two. The ETC storm tide flood enhancement result described in the paragraph above for Poughkeepsie is more pronounced at Albany (Fig. 13), again due to the reduced frictional damping over the 200-km propagation of tides and surges up the Hudson.
The amelioration of sea level rise at Albany can also be explained with simple channel flow dynamics, in which increased depth throughout the river reduces frictional resistance and therefore reduces the water level slope and the upriver water level. In a vertically integrated dynamic balance for channel flow, the along-channel water level gradient is inversely proportional to the bottom stress over water depth (τ/h), so an increase of 10% in h (~ 1 m of sea level rise for the Hudson) reduces the gradient by 10%. The along-channel gradient during a 100-year WETC flood is about 3 m over the upper 50 km of the river, and assuming a fixed sea level at the seaward end, reducing this gradient by 10% ameliorates 0.6 m of flood level near Albany. This is 60% of the imposed sea level rise and is qualitatively consistent with our dynamic model-based findings of a 30–60% mitigation of sea level rise.
5.2 Novel methods for flood hazard assessment from streamflow and storm tides
Our hazard assessment approach holds promise for many coastal regions where storm surge and fluvial flooding merge, avoiding the possible low bias caused by evaluating each separately based on an assumption of statistical independence. Other noteworthy river systems with mixed surge and rain flood hazards exist, including river estuaries of North Carolina (Dresback et al. 2013), Texas (Ray et al. 2011), Chesapeake Bay (Wang et al. 2015), and other global river estuaries (Chen et al. 2012), and these methods may also prove useful for hazard and risk assessment in these locations. Moreover, integrated coastal flood forecast models are advancing rapidly (e.g., Georgas et al. 2016a). The methods developed herein may also be used for TC-related flood forecasting, absent hydrological models, to provide streamflow hydrographs to integrated coastal flood models.
A new statistical probabilistic model was developed for TC-driven streamflows, useful for flood risk assessment in estuaries and tidal river reaches. The Bayesian modeling of TC streamflows has been highly useful in this study, whereas a great deal more effort would be required to set up a comprehensive domain-wide system for TC rainfall and hydrological modeling. The hydrograph model is simple, direct and efficient in reconstructing the discharge hydrographs at outlet sections of the tributaries of Hudson River induced by TC landfalls in the region. This approach allows for a detailed reconstruction of the main characteristics of the hydrograph—peak discharge, temporal trend and shape—as a function of identified TC attributes and of prefixed quantiles. The out-of-sample validation tests have demonstrated the capability of the Bayesian model, in most of the cases and for very different TC characteristics, to capture both the shape of hydrograph and the correct temporal trend of the hydrograph as a function of the position in time of the TC center, reasonably representing the total flood volume during the event. The peak flood timing, together with the peak discharge, plays an important role on the flooding phenomena along the Hudson River downstream of the tributary inlets. Benefits of using the statistical streamflow model included the simplicity of using observed streamflow records, and the model output in terms of percentiles of streamflow. A possible shortcoming of the proposed method is that it requires numerous TCs and consistent streamflow data to estimate the model parameters. If this requirement is satisfied, it can be applied to other hydrological systems.
The results for Albany have higher uncertainty at long return periods (above 50 years) than those at Poughkeepsie and NYH (Fig. 11), and this mainly results from uncertainty in the curve for WETCs. The rate distribution for Albany WETC water levels (Fig. 10 bottom panel) shows they are relatively erratic, somewhat more like TC floods than ETC floods. Yet, the storm set is limited only to 41 historical events. These results suggest that use of a larger historical event set, or development of a synthetic WETC storm set could be helpful for future studies. Alternatively, a study could quantify and utilize the dependence structure of extrema in coastal sea level and river streamflow (e.g., Wahl et al. 2015; Moftakhari et al. 2017) to build an event set that captures a wider range of possible events.
Some factors have been omitted in this first analysis of combined surge, tide, rain and sea level rise impacts on Hudson River flooding. We ignored potential climate change effects on rainfall, soil moisture and hydrology, and of warming on snowpack, which would require substantial additional hydrology and hydroclimate modeling. It would also be valuable to examine how streamflows from tropical cyclones and wet cool season storms will change, as heavy rainfall episodes have been increasing over the U.S. Northeast, and may continue to increase with climate change (e.g., New York City Panel on Climate Change 2013). Due to the dominance of streamflow over storm surge at upriver locations like Albany, this factor could control the future evolution of flood hazards in these areas. However, past changes in precipitation and flooding have not been closely connected and future impacts on storm-driven streamflows are highly uncertain (Peterson et al. 2013). In the past year, a hydrological model for the Hudson River has been set up by members of our research group (Saleh et al. 2016), raising possibilities of further testing the present study’s simplified approach, or creating a deeper analysis of climate change that includes rainfall changes.
Two other minor factors are omitted in the present study, likely with negligible effects. In the present study, we have treated riverbed roughness as a constant, yet riverbed roughness could be variable due to sediment movement. A recent study of the Mississippi River used a variable river bottom roughness that decreased for very high water speeds above 1.5 m s−1 (Martyr et al. 2012). The study showed accurate simulations for a recent hurricane, yet the Mississippi is a substantially larger river with higher water speeds and may not have similar conditions to the Hudson. Ice jams are not modeled, although they are known to have played a part in some of the worst historical floods (typically in the 1800s and early 1900s). It is very reasonable to ignore ice jams, for two reasons: (1) the river was increasingly straightened and channelized in the 1900s, and (2) since President Roosevelt signed the Ice Breaker clause, when ice forms on the modern tidal Hudson River, it is broken by ice-breaking ships (Georgas 2012). A final simplification is that our work assumes river control changes (e.g., through dams) in the future will be negligible. This appears reasonable, given that from the 1940s to present, the relationship between streamflow and flood level for WETCs has been fairly stable, suggesting no major water storage capacity changes.
5.3 Utility for decision-makers, adaptation design
Models and flood hazard assessments that include both streamflow and storm tides can be useful for understanding flood risk, improving disaster management through extreme flood scenarios, studying how climate change will impact future flooding, and quantitatively evaluating flood adaptation measures. For example, modeling for the Thames River in England showed that while the rainfall flood and storm tide flood for one storm peaked at different days, a better response plan could be formulated when using a forecasting system that also considers streamflows (Golding 2009).
The results of the study are being used to map flood zones along the Hudson River, for 5-year to 1000-year floods as part of an online mapping tool, as mentioned (with URL) in Sect. 4.4. The tool will enable planners and populations in the region to better understand and plan for possible flood events and worsening future flooding.
Lastly, flood mitigation measures can be comprehensively evaluated using our assessment. An important example is a cross-harbor storm surge barrier at NYH, which has been considered as a possible solution for the entire harbor region. The storm set gives a wide range of flood events from both storm tides and rainfall, so that the barrier’s influence on both types of flooding could be evaluated, including the potential for trapping of rainfall flooding and resulting duration it can be closed. Similarly, a set of nature-based flood mitigation strategies was developed for New York City’s Jamaica Bay, with higher-resolution modeling, and quantification of monetary benefits and costs using a similar flood assessment framework (Orton et al. 2016a, b; http://AdaptMap.info). Whereas small sets of storm scenarios may be used as basic tests of flood mitigation plans (Cobell et al. 2013), the present study enables the use of probabilistic information to compute annualized avoided damages (the monetary benefit) and other more comprehensive metrics for decision making. In conclusion, the present work demonstrates novel methods for improving emergency preparedness and for quantitative adaptation to future impacts of climate change.
References
Besag J, Green P, Higdon D, Mengersen K (1995) Bayesian computation and stochastic systems. Stat Sci 10(1):3–41
Blumberg AF, Khan LA, St John J (1999) Three-dimensional hydrodynamic model of New York Harbor region. J Hydraul Engin 125(8):799–816
Cerveny RS, Newman LE (2000) Climatological relationships between tropical cyclones and rainfall. Mon Weather Rev 128(9):3329–3336
Chen W-B, Liu W-C (2016) Assessment of storm surge inundation and potential hazard maps for the southern coast of Taiwan. Nat Hazards 82(1):591–616
Chen W-B, Liu W-C, Hsu M-H (2012) Comparison of ANN approach with 2D and 3D hydrodynamic models for simulating estuary water stage. Adv Eng Softw 45(1):69–79
Cioffi F, Gallerano F (2003) A two-dimensional self-adaptive hydrodynamic scheme for the assessment of the effects of structures on flooding phenomena in river basins. River Res Appl 19(1):1–26
Cobell Z, Zhao H, Roberts HJ, Clark FR, Zou S (2013) Surge and wave modeling for the Louisiana 2012 Coastal Master Plan. J Coast Res 67(sp1):88–108
Czajkowski J, Villarini G, Michel-Kerjan E, Smith JA (2013) Determining tropical cyclone inland flooding loss on a large scale through a new flood peak ratio-based methodology. Environ Res Lett 8(4):044056
Dresback KM, Fleming JG, Blanton BO et al (2013) Skill assessment of a real-time forecast system utilizing a coupled hydrologic and coastal hydrodynamic model during Hurricane Irene (2011). Cont Shelf Res 71:78–94
Emanuel KA (1991) The theory of hurricanes. Annu Rev Fluid Mech 23(1):179–196
FEMA (2014) Region II coastal storm surge study: overview. In M. Risk assessment, and planning partners (ed). Federal Emergency Management Agency, Washington, p 15
Field PR, Wood R (2007) Precipitation and cloud structure in midlatitude cyclones. J Clim 20(2):233–254
Frank WM (1977) The structure and energetics of the tropical cyclone II. Dynamics and energetics. Mon Weather Rev 105(9):1136–1150
Gelfand AE, Smith AF (1990) Sampling-based approaches to calculating marginal densities. J Am Stat Assoc 85(410):398–409
Georgas N (2010) Establishing confidence in marine forecast systems: the design of a high fidelity marine forecast model for the NY/NJ harbor estuary and its adjoining coastal waters, PhD dissertation, Department of Civil, Environmental and Ocean Engineering. Stevens Institute of Technology, Hoboken, New Jersey
Georgas N (2012) Large seasonal modulation of tides due to ice cover friction in a midlatitude estuary*. J Phys Oceanogr 42(3):352–369
Georgas N, Blumberg AF (2009) Establishing confidence in marine forecast systems: the design and skill assessment of the New York Harbor Observation and Prediction System, version 3 (NYHOPS v3). Paper presented at the Eleventh International Conference in Estuarine and Coastal Modeling (ECM11), Seattle, Washington, USA
Georgas N, Wen B, Zhao Y (2013) Calculation of vertical tidal datums for the tidal Hudson River north of Yonkers, New York. Technical report 2926, Stevens Institute of Technology, p 25
Georgas N, Orton P, Blumberg A, Cohen L, Zarrilli D, Yin L (2014) The impact of tidal phase on Hurricane Sandy’s flooding around New York City and Long Island Sound. J Extreme Events. https://doi.org/10.1142/S2345737614500067
Georgas N, Blumberg A, Herrington T et al (2016a) The Stevens Flood Advisory System: operational H3e flood forecasts for the Greater New York/New Jersey metropolitan region. Int J Saf Secur Eng 6(3):648–662
Georgas N, Yin L, Jiang Y et al (2016b) An open-access, multi-decadal, three-dimensional, hydrodynamic hindcast dataset for the Long Island Sound and New York/New Jersey Harbor Estuaries. J Mar Sci Eng. https://doi.org/10.3390/jmse4030048
Geyer WR, Chant R (2006) The physical oceanography processes in the Hudson River Estuary. In: Levinton J, Waldman JR (eds) The Hudson River estuary. Cambridge University Press, New York
Gibbs L, Holloway C (2013) Hurricane Sandy after action, reports and recommendations to Mayor Michael R. Bloomberg. Deputy Mayors of NYC, New York
Golding B (2009) Long lead time flood warnings: reality or fantasy? Meteorol Appl 16(1):3–12
Hall T, Yonekura E (2013) North American tropical cyclone landfall and SST: a statistical model study. J Clim 26(21):8422–8439
Hipel KW, McLeod AI (1994) Time series modelling of water resources and environmental systems, vol 45. Elsevier, Amsterdam
Holland GJ (1980) An analytic model of the wind and pressure profiles in hurricanes. Mon Weather Rev 108(8):1212–1218
Horton R, Bader D, Rosenzweig C, DeGaetano A, Solecki W (2015) Climate change in New York State: updating the 2011 ClimAID climate risk information, supplement to NYSERDA report 11-18 (responding to climate change in New York State). Albany, NY
Jelesnianski C, Chen J, Shaffer W (1992) SLOSH: sea, lake, and overland surges from hurricanes. US Department of Commerce, National Oceanic and Atmospheric Administration, National Weather Service, Silver Spring, MD, USA
Konrad CE, Meaux MF, Meaux DA (2002) Relationships between tropical cyclone attributes and precipitation totals: considerations of scale. Int J Climatol 22(2):237–247
Lin N, Emanuel K, Oppenheimer M, Vanmarcke E (2012) Physically based assessment of hurricane surge threat under climate change. Nat Clim Change 2(6):462–467
Lonfat M, Marks FD Jr, Chen SS (2004) Precipitation distribution in tropical cyclones using the Tropical Rainfall Measuring Mission (TRMM) microwave imager: a global perspective. Mon Weather Rev 132(7):1645–1660
Marks Jr FD, De Maria M (2003) Development of a tropical cyclone rainfall climatology and persistence (R-CLIPER) model. Final report, p 20
Martyr R, Dietrich J, Westerink JJ et al (2012) Simulating hurricane storm surge in the lower Mississippi River under varying flow conditions. J Hydraul Eng 139(5):492–501
Merz B, Aerts J, Arnbjerg-Nielsen K et al (2014) Floods and climate: emerging perspectives for flood risk assessment and management. Nat Hazards Earth Syst Sci 14(7):1921
Moftakhari HR, Salvadori G, AghaKouchak A, Sanders BF, Matthew RA (2017) Compounding effects of sea level rise and fluvial flooding. Proc Natl Acad Sci 114(37):9785–9790
New York City Panel on Climate Change (2013) Climate risk information 2013: observations, climate change projections, and maps. In Rosenzweig C, Solecki W (ed) Prepared for use by the city of New York special initiative on rebuilding and resiliency, New York
Orton PM, Visbeck M (2009) Variability of internally generated turbulence in an estuary, from 100 days of continuous observations. Cont Shelf Res 29(1):61–77
Orton P, Georgas N, Blumberg A, Pullen J (2012) Detailed modeling of recent severe storm tides in estuaries of the New York City region. J Geophys Res 117:C09030. https://doi.org/10.1029/2012JC008220
Orton P, Vinogradov S, Georgas N et al (2015) New York City panel on climate change 2015 report chapter 4: dynamic coastal flood modeling. Ann N Y Acad Sci 1336(1):56–66
Orton PM, Hall TM, Talke S, Blumberg AF, Georgas N, Vinogradov S (2016a) A validated tropical-extratropical flood hazard assessment for New York harbor. J Geophys Res. https://doi.org/10.1002/2016JC011679
Orton P, MacManus K, Sanderson E et al (2016b) Project final technical report: quantifying the value and communicating the protective services of nature-based flood mitigation using flood risk assessment. http://adaptmap.info/jamaicabay/technical_report.pdf. Accessed 01 Jan 2017
Peterson TC, Heim RR Jr, Hirsch R et al (2013) Monitoring and understanding changes in heat waves, cold waves, floods, and droughts in the United States: state of knowledge. Bull Am Meteorol Soc 94(6):821–834
Ray T, Stepinski E, Sebastian A, Bedient PB (2011) Dynamic modeling of storm surge and inland flooding in a Texas coastal floodplain. J Hydraul Eng 137(10):1103–1110
Reich BJ, Smith LB (2013) Bayesian quantile regression for censored data. Biometrics 69(3):651–660
Rodgers EB, Baik J-J, Pierce HF (1994) The environmental influence on tropical cyclone precipitation. J Appl Meteorol 33(5):573–593
Saleh F, Ramaswamy V, Georgas N, Blumberg AF, Pullen J (2016) A retrospective streamflow ensemble forecast for an extreme hydrologic event: a case study of Hurricane Irene and on the Hudson River basin. Hydrol Earth Syst Sci 20(7):2649–2667. https://doi.org/10.5194/hess-20-2649-2016
Sobel AH (2014) Storm surge: hurricane sandy, our warming planet, and the extreme weather of the past and future. Harper Wave, New York
Strachan J (2012) Tropical cyclones in 2011. Weather 67(2):31–34
Tanner MA, Wong WH (1987) The calculation of posterior distributions by data augmentation. J Am Stat Assoc 82(398):528–540
U.S. Geological Survey (2016) National Water Information System data available on the World Wide Web (USGS Water Data for the Nation). Retrieved 16 Dec 2016 from http://waterdata.usgs.gov/nwis/
Villarini G, Goska R, Smith JA, Vecchi GA (2014) North Atlantic tropical cyclones and US flooding. Bull Am Meteorol Soc 95(9):1381–1388
Wahl T, Jain S, Bender J, Meyers SD, Luther ME (2015) Increasing risk of compound flooding from storm surge and rainfall for major US cities. Nat Clim Change 5(12):1093–1097
Wang HV, Loftis JD, Liu Z, Forrest D, Zhang J (2014) The storm surge and sub-grid inundation modeling in New York City during Hurricane Sandy. J Mar Sci Eng 2(1):226–246
Wang HV, Loftis JD, Forrest D, Smith W, Stamey B (2015) Modeling storm surge and inundation inWashington, DC, during Hurricane Isabel and the 1936 Potomac River Great Flood. J Mar Sci Engin 3(3):607–629
Yang Z, Wang T, Khangaonkar T, Breithaupt S (2012) Integrated modeling of flood flows and tidal hydrodynamics over a coastal floodplain. Environ Fluid Mech 12(1):63–80
Yin J, Yu D, Yin Z, Wang J, Xu S (2013) Modelling the combined impacts of sea-level rise and land subsidence on storm tides induced flooding of the Huangpu River in Shanghai, China. Clim change 119(3–4):919–932
Zervas C (2013). Extreme water levels of the United States 1893–2010, NOAA technical report NOS CO-OPS 067, NOAA National Ocean Service Center for Operational Oceanographic Products and Services. Silver Spring, Maryland, p 200
Zhong H, Van Overloop P, Van Gelder P (2013) A joint probability approach using a 1-D hydrodynamic model for estimating high water level frequencies in the Lower Rhine Delta. Nat Hazards Earth Syst Sci 13(7):1841
Acknowledgements
We would like to acknowledge the vision and leadership of Mark G. Becker (1961–2014), who was an original Principal Investigator for the project. Amanda Stevens, Jane Mills and Dara Mendeloff also played important roles in the project.
Funding
This research was primarily funded by New York State Energy Research and Development Authority (NYSERDA; Agreement 28258A). Funding also came from the National Aeronautics and Space Administration (NASA) Centers call for support of the National Climate Assessment (Agreements NNX12AI28G and NNX15AD61G) and Research Opportunities in Space and Earth Sciences (NASA-ROSES-2012; grant NNX14AD48G). Modeling was made possible by a grant of computer time from the City University of New York High Performance Computing Center under NSF Grants CNS-0855217, CNS-0958379 and ACI-1126113.
Author information
Authors and Affiliations
Corresponding author
Ethics declarations
Conflict of interest
The authors declare that they have no conflict of interest.
Rights and permissions
About this article
Cite this article
Orton, P.M., Conticello, F.R., Cioffi, F. et al. Flood hazard assessment from storm tides, rain and sea level rise for a tidal river estuary. Nat Hazards 102, 729–757 (2020). https://doi.org/10.1007/s11069-018-3251-x
Received:
Accepted:
Published:
Issue Date:
DOI: https://doi.org/10.1007/s11069-018-3251-x