Abstract
We conducted a meta-analysis of case–control studies to determine whether SELP genetic polymorphisms contribute to the pathogenesis of coronary heart disease (CHD) and myocardial infarction (MI). A range of electronic databases were searched: MEDLINE (1966–2013), the Cochrane Library Database (Issue 12, 2013), EMBASE (1980–2013), CINAHL (1982–2013), Web of Science (1945–2013) and the Chinese biomedical database (1982–2013) without language restrictions. Meta-analysis was performed with the use of the STATA statistical software. Nine case–control studies with a total of 3,154 CHD patients, 1,608 MI patients and 17,304 healthy controls were involved in this meta-analysis. Six common polymorphisms in the SELE gene were assessed, including −1969G/A (rs1800805 G > A), −1817T/C (rs1800808 T > C), −2123C/G (rs1800807 C > G), Thr715Pro (rs6136 A > C), Leu599Val (rs6133 G > T), and Ser290Asn (rs6131 C > T). Our findings illustrated significantly positive associations of SELE genetic polymorphisms with the development of CHD and MI. The results of subgroup analysis by SNP type indicated that −1969G/A, −1817T/C, −2123C/G, Thr715Pro and Ser290Asn in the SELP gene might be strongly correlated with CHD and MI risk, but no similar results were found in SELP Leu599Val polymorphism. In the subgroup analysis by ethnicity, our results indicated significant relationships between SELE genetic polymorphisms and the pathogenesis of CHD and MI among Asians and Caucasians. However, we observed no significant associations between SELP genetic polymorphisms and the risk of CHD and MI among Africans. Our findings provide empirical evidence that SELE genetic polymorphisms may contribute to the pathogenesis of CHD and MI, especially among Asians and Caucasians. Thus, SELP genetic polymorphisms could be potential and practical biomarkers for early diagnosis of CHD and MI.
Similar content being viewed by others
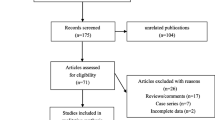
Avoid common mistakes on your manuscript.
Introduction
Coronary heart disease (CHD) is the most common type of heart disease induced by the narrowing or blockage of the coronary arteries resulting from atherosclerosis, which is characterized by gradual build-up of fatty material and plaque inside the wall of arteries [1]. Myocardial infarction (MI), another widely known heart attack, is the first clinical manifestation of CHD [2]. It has been reported that CHD is responsible for approximately 7.3 million deaths, with its highest prevalence in high or middle income countries [3]. Furthermore, MI is considered as the leading cause of death and disability all over the world [4]. During the past decades, a number of risk factors associated with CHD and MI have been identified including old age, tobacco smoking, hypertension, and unhealthy cholesterol levels [5, 6]. Currently, rapid advances have been made in the genetic analysis of CHD and MI and susceptibility genes such as SELP are hypothesized to be involved in the pathogenesis of CHD and MI [7, 8].
P-selectin, encoded by SELP gene, is a 140 kDa adhesion molecule belonging to the selectin family of cell adhesion molecules which are involved in the transient attachment of leukocytes to endothelial cells and platelets [9]. More specific, P-selectin, mainly stored in α-granules of platelets, could support leukocyte rolling on the endothelium, platelet rolling on venules and platelet-leukocyte adhesion, which may further facilitate the interaction of platelets, leukocytes and endothelial cells [10]. Human SELP gene is located on chromosome 1q21–24, regions that also shelters the SELE and SELL genes, and composed of 17 exons and 16 introns, spanning proximately 50 kb [11]. To date, polymorphic variants of SELP have been intensively postulated to be involved in the pathogenesis of atherosclerotic and inflammatory diseases, including CHD and MI [12–14]. Moreover, there is evidence demonstrating that P-selectin may act as a novel risk marker in both early and advanced stages of the atherosclerotic lesion development, and up-regulated expression of P-selectin on endothelial cells may promote the formation of atherosclerotic plaques, and increase the risk of atherosclerotic diseases [14, 15]. A number of polymorphisms in the SELP gene have been identified which may influence the peptide sequence of this protein and its function, thereby contributing to susceptibility to CHD and MI [10, 12]. Large quantities of evidence have demonstrated that SELP genetic polymorphisms may contribute to an increased risk of CHD and MI, but contradictory results were also reported [16, 17]. Given the conflicting evidence on this issue, we conducted a meta-analysis of all available case–control studies to determine whether SELP genetic polymorphisms contribute to the pathogenesis of CHD and MI.
Materials and methods
Literature search and selection criteria
A range of electronic databases were searched: MEDLINE (1966–2013), the Cochrane Library Database (Issue 12, 2013), EMBASE (1980–2013), CINAHL (1982–2013), Web of Science (1945–2013) and the Chinese Biomedical Database (CBM) (1982–2013) without language restrictions. We used the following keywords and MeSH terms in conjunction with a highly sensitive search strategy: [“single nucleotide polymorphism” or “SNP” or “polymorphism” or “mutation” or “mutant” or “variation” or “variant”] and [“myocardial infarction” or “MI” or “coronary artery diseases” or “coronary heart disease” or “CHD” or “CAD”] and [“P-selectin” or “CD62P” or “granule membrane protein” or “GMP140 “]. We also conducted a manual search to find other potential articles based on references identified in the individual articles.
The following criteria were for the eligibility of included studies: (1) the study design must be clinical case–control study that focused on the relationships of SELP genetic polymorphisms with the pathogenesis of CHD or MI; (2) all patients met the diagnostic criteria for CHD and MI; (3) the genotype frequencies of healthy controls should follow the Hardy–Weinberg equilibrium (HWE); (4) the study must provide sufficient information about the genotype frequencies. If the study could not meet the inclusion criteria, it would be excluded. The most recent or the largest sample size publication was included when the authors published several studies using the same subjects.
Data extraction and methodological assessment
Data were systematically extracted by two authors from each included study by using a standardized form. The form used for data extraction documented the most relevant items including language of publication, publication year of article, the first author’s surname, geographical location, design of study, sample size, the source of the subjects, genotype frequencies, source of samples, genotyping method, evidence of HWE, etc.
Methodological quality was evaluated separately by two observers using the Newcastle-Ottawa Scale (NOS) criteria [18]. The NOS criteria included three aspects: (1) subject selection: 0–4; (2) comparability of subject: 0–2; (3) clinical outcome: 0–3. NOS scores ranged from 0 to 9; and a score ≥7 indicate a good quality.
Statistical analysis
Meta-analysis was performed with the use of the STATA statistical software (Version 12.0, Stata Corporation, College Station, TX, USA). Odds ratios (OR) and 95 % confidence interval (95 % CI) were calculated as estimates of relative risk for TB under different genetic models. The Z test was used to estimate the statistical significance of pooled ORs. Heterogeneity among studies were estimated by the Cochran’s Q-statistic and I 2 tests [19]. If Q test shows a P < 0.05 or I 2 test exhibits >50 % which indicates significant heterogeneity, the random-effect model was conducted, or else the fixed-effects model was used. We also explored reasons for heterogeneity using meta-regression and subgroup analyses. In order to evaluate the influence of single studies on the overall estimate, a sensitivity analysis was performed. Funnel plots and Egger’s linear regression test were applied to investigate publication bias [20].
Results
Study selection and characteristics of included studies
Initially, the highly sensitive search strategy identified 169 articles. We reviewed the titles and abstracts of all articles and excluded 77 articles; then we systematically reviewed full texts and 79 articles were further excluded. Another 4 studies were also excluded due to lack of data integrity. Publication years of the eligible studies ranged from 2000 to 2012. Figure 1 shows the selection process of eligible articles. Finally, 9 case–control studies with a total of 3,154 CHD patients, 1,608 MI patients and 17,304 healthy subjects met our inclusion criteria for qualitative data analysis [10, 11, 14, 16, 17, 21–24]. Overall, 6 studies were conducted among Caucasians, 2 studies among Asians and only one study among Africans. Polymerase chain reaction–restriction fragment length polymorphism (PCR–RFLP), TaqMan assay, PCR-allele-specific oligonucleotide (PCR-ASO), and PCR-sequence-specific primers (PCR-SSP) were used for genotyping. Six common polymorphisms in the SELP gene were assessed, including −1969G/A (rs1800805 G > A), −1817T/C (rs1800808 T > C), −2123C/G (rs1800807 C > G), Thr715Pro (rs6136 A > C), Leu599Val (rs6133 G > T), and Ser290Asn (rs6131 C > T). None of the studies deviated from the HWE (all P > 0.05). NOS scores of all included studies were ≥ 5. We summarized the study characteristics and methodological quality in Table 1.
Quantitative data synthesis
Our findings illustrated significantly positive associations of SELE genetic polymorphisms with the development of CHD (allele model: OR = 1.29, 95 % CI 1.16–1.43, P = 0.019; dominant model: OR = 1.31, 95 % CI 1.16–1.48, P = 0.034; recessive model: OR = 1.47, 95 % CI 1.23–1.75, P < 0.001; homozygous model: OR = 8.38, 95 % CI 4.81–14.61, P < 0.001; heterozygous model: OR = 1.32, 95 % CI 1.09–1.61, P = 0.005; respectively) and MI (allele model: OR = 1.31, 95 % CI 1.12–1.54, P = 0.001; dominant model: OR = 1.27, 95 % CI 1.07–1.51, P = 0.006; recessive model: OR = 2.05, 95 % CI 1.28–3.30, P = 0.003; homozygous model: OR = 13.13, 95 % CI 8.47–20.33, P = 0.013; heterozygous model: OR = 1.82, 95 % CI 1.13–2.92, P = 0.013; respectively) (Fig. 2).
We also conducted subgroup analysis to investigate the influence of potential factors on individuals’ risk of CHD and MI. The results of subgroup analysis by SNP type indicated that −1969G/A, −1817T/C, −2123C/G, Thr715Pro and Ser290Asn in the SELP gene might be strongly correlated with CHD and MI risk, but no similar results were found in SELP Leu599Val polymorphism (as shown in Table 2). In the subgroup analysis by ethnicity, our results indicated significant relationships between SELE genetic polymorphisms and the pathogenesis of CHD and MI among Asians (allele model: OR = 1.47, 95 % CI 1.23–1.77, P < 0.001; dominant model: OR = 1.56, 95 % CI 1.22–2.00, P < 0.001; recessive model: OR = 1.80, 95 % CI 1.22–2.64, P = 0.003; homozygous model: OR = 7.43, 95 % CI 3.96–13.95, P < 0.001; respectively) and Caucasians (allele model: OR = 1.26, 95 % CI 1.16–1.37, P < 0.001; dominant model: OR = 1.26, 95 % CI 1.14–1.41, P < 0.001; recessive model: OR = 1.65, 95 % CI 1.27–2.14, P < 0.001; homozygous model: OR = 10.73, 95 % CI 6.48–17.76, P < 0.001; heterozygous model: OR = 1.47, 95 % CI 1.12–1.92, P = 0.005; respectively). However, we observed no significant associations between SELP genetic polymorphisms and the risk of CHD and MI among Africans (all P > 0.05). As shown in Table 2, SELP genetic polymorphisms also showed significant associations with an increased risk of CHD and MI in the PCR–RFLP and TaqMan assay subgroups (all P < 0.05), while similar results were not found in other subgroups (all P > 0.05) (Fig. 3, 4).
Univariate and multivariate meta-regression analyses indicated that SNP type, country and genotyping method might be predominant sources of heterogeneity (Table 3). Sensitivity analysis suggested that no single study could influence the pooled ORs (Fig. 5). Funnel plots demonstrated no evidence of obvious asymmetry existing (Fig. 6). Egger’s test also did not display strong statistical evidence for publication bias (allele model: t = 0.03, P = 0.978; dominant model: t = −0.54, P = 0.598).
Discussion
In the current meta-analysis, individuals with SELP genetic polymorphisms were found to have a higher risk of developing CHD and MI, suggesting that genetic variants in SELP gene may be strongly correlated with the etiology of CHD and MI. Although the exact role of SELP genetic polymorphisms in the occurrence of CHD and MI is not well elucidated, a possible interpretation for this finding might be that SELP genetic polymorphisms might increase its level and alter its function which was postulated to be implicated in the development of atherosclerosis [25]. It is widely accepted that P-selectin can mediate leukocyte rolling on the endothelium, and promote leukocyte adhesion to activated endothelial cells and platelets through various stimulation induced by oxidized low density lipoprotein, thrombin, and cytokines [26]. After activation, part of the P-selectin molecule is trans-located to the cell surface, and released into the plasma in soluble form, further facilitating the leukocyte-endothelial cell adhesion [27]. Furthermore, P-selectin is responsible for efficient recruitment of neutrophils in chronic and acute inflammation and has been demonstrated to be capable of binding T cells on vascular endothelial cells recently [28]. Consequently, P-selectin has been regarded to play a crucial role in the development of atherogenesis [29].
We also carried out subgroup analysis based on country, ethnicity, SNP type and genotyping method in consideration of obvious heterogeneity. The results of subgroup analysis by SNP type revealed that −1969G/A, −1817T/C, −2123C/G, Thr715Pro and Ser290Asn in the SELP gene might be closely linked to the pathogenesis of CHD and MI. Furthermore, our findings showed significant positive associations between common polymorphisms in the SELP gene and an increased risk of CHD and MI among Asians and Caucasians, but no similar associations were observed among Africans, implicating that ethnicity differences may play an important in modifying individual’s susceptibility to CHD and MI. A reasonable explanation may be that large differences in common SNPs that affect the risk of CHD and MI are mostly due to genetic drift and natural selection. Subgroup analysis based on genotyping method suggested that SELP genetic polymorphisms may be related with an increased risk of CHD and MI in the PCR–RFLP and TaqMan assay subgroups, but not in other subgroups. In short, our findings are consistent with previous studies that SELP genetic polymorphisms may increase the risk of CAD and MI.
The current meta-analysis also had several limitations that should be acknowledged. First, our results were lack of sufficient statistical power to assess the correlations of SELP genetic polymorphisms with the development of CHD and MI. Secondly, meta-analysis is a retrospective study that may inevitably induce subject selection bias, and thereby have an impact on the reliability of our results. Thirdly, our meta-analysis failed to obtain original data from the included studies, which may limit further evaluation of potential role of SELP genetic polymorphisms in the pathogenesis of CHD and MI. Although our study has some limitations, this is the first meta-analysis focusing on the relationships of SELP genetic polymorphisms with CHD and MI risk. Furthermore, we undertook a highly sensitive literature search strategy for electronic databases. A manual search of the reference lists from the relevant articles was also conducted to find other potential articles. The selection process of eligible articles was performed based on strict inclusion and exclusion criteria. Importantly, rigorous statistical analysis of SNP data provided a basis for pooling of information from individual studies.
In conclusion, our meta-analysis results confirmed the hypothesis that SELE genetic polymorphisms may contribute to the pathogenesis of CHD and MI, especially among Asians and Caucasians. Thus, SELP genetic polymorphisms could be potential and practical biomarkers for early diagnosis of CHD and MI. However, due to the limitations mentioned above, more reliable researches with larger sample size are still required to provide a more comprehensive and representative statistical analysis precisely.
References
Tehrani DM, Seto AH (2013) Third universal definition of myocardial infarction: update, caveats, differential diagnoses. Cleve Clin J Med 80:777–786. doi:10.3949/ccjm.80a.12158
Gerretsen SC, Kooi ME, Kessels AG, Schalla S, Katoh M, van der Geest RJ, Manning WJ, Waltenberger J et al (2010) Visualization of coronary wall atherosclerosis in asymptomatic subjects and patients with coronary artery disease using magnetic resonance imaging. PLoS One. doi:10.1371/journal.pone.0012998
Gaziano TA, Bitton A, Anand S, Abrahams-Gessel S, Murphy A (2010) Growing epidemic of coronary heart disease in low- and middle-income countries. Curr Probl Cardiol 35:72–115. doi:10.1016/j.cpcardiol.2009.10.002
Thygesen K, Alpert JS, White HD, Jaffe AS, Apple FS, Galvani M, Katus HA, Newby LK et al (2007) Universal definition of myocardial infarction. Circulation 116:2634–2653. doi:10.1161/CIRCULATIONAHA.107.187397
Torpy JM, Burke AE, Glass RM (2009) Jama patient page. Coronary heart disease risk factors. JAMA 302:2388. doi:10.1001/jama.302.21.2388
Anand SS, Islam S, Rosengren A, Franzosi MG, Steyn K, Yusufali AH, Keltai M, Diaz R et al (2008) Risk factors for myocardial infarction in women and men: insights from the interheart study. Eur Heart J 29:932–940. doi:10.1093/eurheartj/ehn018
Motawi T, Shaker O, Taha N, Abdel Raheem M (2012) Genetic variations in E-selectin and ICAM-1: relation to atherosclerosis. Med Sci Monit 18:CR381–CR389. http://www.ncbi.nlm.nih.gov/pubmed/22648254
Stellos K, Bigalke B, Stakos D, Henkelmann N, Gawaz M (2010) Platelet-bound P-selectin expression in patients with coronary artery disease: impact on clinical presentation and myocardial necrosis, and effect of diabetes mellitus and anti-platelet medication. J Thromb Haemost 8:205–207. doi:10.1111/j.1538-7836.2009.03659.x
Woollard KJ, Chin-Dusting J (2010) P-selectin antagonism in inflammatory disease. Curr Pharm Des 16:4113–4118. http://www.ncbi.nlm.nih.gov/pubmed/21247396
Ghazouani L, Abboud N, Khalifa SB, Perret C, Nicaud V, Almawi WY, Cambien F, Mahjoub T (2011) Contribution of SELP and PSGL-1 genotypes and haplotypes to the presence of coronary heart disease in Tunisians. Mol Biol Rep 38:495–501. doi:10.1007/s11033-010-0133-z
Barbaux SC, Blankenberg S, Rupprecht HJ, Francomme C, Bickel C, Hafner G, Nicaud V, Meyer J et al (2001) Association between P-selectin gene polymorphisms and soluble p-selectin levels and their relation to coronary artery disease. Arterioscler Thromb Vasc Biol 21:1668–1673
Ghazouani L, Abboud N, Khlifa SB, Perret C, Nicaud V, Cambien F, Almawi WY, Mahjoub T (2009) P-selectin gene polymorphisms and risk of coronary heart disease among tunisians. J Thromb Thrombolysis 28:314–319. doi:10.1007/s11239-008-0297-8
Pasquali A, Trabetti E, Romanelli MG, Galavotti R, Martinelli N, Girelli D, Gambaro G, Olivieri O et al (2010) Detection of a large deletion in the P-selectin (selp) gene. Mol Cell Probes 24:161–165. doi:10.1016/j.mcp.2009.11.006
Elmas E, Bugert P, Popp T, Lang S, Weiss C, Behnes M, Borggrefe M, Kalsch T (2010) The P-selectin gene polymorphism val168met: a novel risk marker for the occurrence of primary ventricular fibrillation during acute myocardial infarction. J Cardiovasc Electrophysiol 21:1260–1265. doi:10.1111/j.1540-8167.2010.01833.x
Sivapalaratnam S, Motazacker MM, Maiwald S, Hovingh GK, Kastelein JJ, Levi M, Trip MD, Dallinga-Thie GM (2011) Genome-wide association studies in atherosclerosis. Curr Atheroscler Rep 13:225–232. doi:10.1007/s11883-011-0173-4
Carter AM, Anagnostopoulou K, Mansfield MW, Grant PJ (2003) Soluble P-selectin levels, P-selectin polymorphisms and cardiovascular disease. J Thromb Haemost 1:1718–1723. http://onlinelibrary.wiley.com/store/10.1046/j.1538-7836.2003.00312.x/asset/j.1538-7836.2003.00312.x.pdf?v=1&t=hoaq2ete&s=7e7574582c227f653a1bfc9fc93499a593f418ef
Kee F, Morrison C, Evans AE, McCrum E, McMaster D, Dallongeville J, Nicaud V, Poirier O et al (2000) Polymorphisms of the P-selectin gene and risk of myocardial infarction in men and women in the ectim extension study. Etude cas-temoin de l’infarctus myocarde. Heart 84:548–552
Stang A (2010) Critical evaluation of the newcastle-ottawa scale for the assessment of the quality of nonrandomized studies in meta-analyses. Eur J Epidemiol 25:603–605. doi:10.1007/s10654-010-9491-z
Zintzaras E, Ioannidis JP (2005) Hegesma: genome search meta-analysis and heterogeneity testing. Bioinformatics 21:3672–3673. doi:10.1093/bioinformatics/bti536
Peters JL, Sutton AJ, Jones DR, Abrams KR, Rushton L (2006) Comparison of two methods to detect publication bias in meta-analysis. JAMA 295:676–680. doi:10.1001/jama.295.6.676
Liao CL, Huang MZ, Liu HY, Liang D, Pam GZ (2011) Association of the genotype and serum level of P-selectin with acute myocardial infarction. Chin J Arteriosclerosis 19:151–154. http://d.g.wanfangdata.com.cn/Periodical_zgdmyhzz201102016.aspx
Liao CL, Huang MZ, Liu HY, Liang D, Pan GZ (2012) Association P-selectin gene polymorphisms and haplotype with coronary heart disease. J Guangxi Med Univ 29:598–600
Rosner SA, Ridker PM, Zee RY, Cook NR (2005) Interaction between inflammation-related gene polymorphisms and cigarette smoking on the risk of myocardial infarction in the physician’s health study. Hum Genet 118:287–294. doi:10.1007/s00439-005-0052-6
Volcik KA, Ballantyne CM, Coresh J, Folsom AR, Wu KK, Boerwinkle E (2006) P-selectin Thr715Pro polymorphism predicts P-selectin levels but not risk of incident coronary heart disease or ischemic stroke in a cohort of 14595 participants: the Atherosclerosis Risk in Communities Study. Atherosclerosis 186:74–79. doi:10.1016/j.atherosclerosis.2005.07.010
van der Zee PM, Biro E, Ko Y, de Winter RJ, Hack CE, Sturk A, Nieuwland R (2006) P-selectin- and CD63-exposing platelet microparticles reflect platelet activation in peripheral arterial disease and myocardial infarction. Clin Chem 52:657–664. doi:10.1373/clinchem.2005.057414
Chen AY, Ha JN, Delano FA, Schmid-Schonbein GW (2012) Receptor cleavage and P-selectin-dependent reduction of leukocyte adhesion in the spontaneously hypertensive rat. J Leukoc Biol 92:183–194. doi:10.1189/jlb.0112010
Jaremo P, Milovanovic M, Buller C, Nilsson S, Winblad B (2013) P-selectin paradox and dementia of the alzheimer type: circulating P-selectin is increased but platelet-bound P-selectin after agonist provocation is compromised. Scand J Clin Lab Invest 73:170–174. doi:10.3109/00365513.2013.764572
Brown JB, Cheresh P, Zhang Z, Ryu H, Managlia E, Barrett TA (2012) P-selectin glycoprotein ligand-1 is needed for sequential recruitment of T-helper 1 (Th1) and local generation of Th17 T cells in dextran sodium sulfate (DSS) colitis. Inflamm Bowel Dis 18:323–332. doi:10.1002/ibd.21779
Kisucka J, Chauhan AK, Zhao BQ, Patten IS, Yesilaltay A, Krieger M, Wagner DD (2009) Elevated levels of soluble P-selectin in mice alter blood-brain barrier function, exacerbate stroke, and promote atherosclerosis. Blood 113:6015–6022. doi:10.1182/blood-2008-10-186650
Acknowledgments
This study was funded by the Department of Education Project of Liaoning Province (20072092). We would like to thank our colleagues at the Department of Cardiology, The Fourth Affiliated Hospital of China Medical University.
Disclosure
No competing financial interests exist.
Author information
Authors and Affiliations
Corresponding author
Additional information
The Publisher and Editor retract this article in accordance with the recommendations of the Committee on Publication Ethics (COPE). After a thorough investigation we have strong reason to believe that the peer review process was compromised.
About this article
Cite this article
Zhou, DH., Wang, Y., Hu, WN. et al. RETRACTED ARTICLE: SELP genetic polymorphisms may contribute to the pathogenesis of coronary heart disease and myocardial infarction: a meta-analysis. Mol Biol Rep 41, 3369–3380 (2014). https://doi.org/10.1007/s11033-014-3199-1
Received:
Accepted:
Published:
Issue Date:
DOI: https://doi.org/10.1007/s11033-014-3199-1