Abstract
To assess whether a measure of prenatal case management (PCM) dosage is more sensitive than a dichotomous PCM exposure measure when evaluating the effect of PCM on low birthweight (LBW) and preterm birth (PTB). We constructed a retrospective cohort study (N = 16,657) of Iowa Medicaid-insured women who had a singleton live birth from October 2005 to December 2006; 28 % of women received PCM. A PCM dosage measure was created to capture duration of enrollment, total time with a case manager, and intervention breadth. Propensity score (PS)-adjusted odds ratios (ORs), and 95 % confidence intervals (95 % CIs) were calculated to assess the risk of each outcome by PCM dosage and the dichotomous PCM exposure measure. PS-adjusted ORs of PTB were 0.88 (95 % CI 0.70–1.11), 0.58 (95 % CI 0.47–0.72), and 1.43 (95 % CI 1.23–1.67) for high, medium, and low PCM dosage, respectively. For LBW, the PS-adjusted ORs were 0.76 (95 % CI 0.57–1.00), 0.64 (95 % CI 0.50–0.82), and 1.36 (95 % CI 1.14–1.63), for high, medium, and low PCM dosage, respectively. The PCM dichotomous participation measure was not significantly associated with LBW (OR = 0.95, 95 % CI 0.82–1.09) or PTB (0.97, 95 % CI 0.87–1.10). The reference group in each analysis is No PCM. PCM was associated with a reduced risk of adverse pregnancy outcomes for Medicaid-insured women in Iowa. PCM dosage appeared to be a more sensitive measure than the dichotomous measure of PCM participation.
Similar content being viewed by others
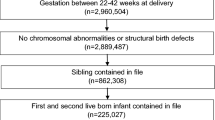
Explore related subjects
Discover the latest articles, news and stories from top researchers in related subjects.Avoid common mistakes on your manuscript.
Introduction
Over the years, numerous evaluations of prenatal case management (PCM) have been conducted. PCM is a community-based health related service offered to medically and/or socially vulnerable pregnant women to improve maternal and infant health outcomes [1]. Many of these studies have shown significant benefits for participants in mental health, substance use, employment attainment, parenting skills, and educational attainment [2–9]. Unfortunately, studies examining outcomes such as low birthweight (LBW) and preterm birth (PTB) have shown little or no benefit from PCM participation [4, 9, 10]. Studies that do show a significant improvement in birth outcomes seem to detect differences only in the extremes of the outcomes, very PTB (<34 weeks) and very LBW (<1,500 g) [4, 11]. Critiques of previous studies of PCM suggest that the failure to detect an effect may be due to a lack of statistical power resulting from a small sample size, [6, 10, 12] selection bias, [9, 12] or failure to assess PCM dosage [6, 10, 13–15].
Background
PCM is a collaborative process in which a case manager (CM) and a pregnant woman work together to develop an individualized plan of care to address the woman’s needs as identified through risk assessment [13, 15, 16]. Since health and social needs differ among women, the intervention breadth, amount of time spent with a CM, and duration of enrollment in PCM is unique to each woman [15]. Most studies evaluating the relationship between PCM and pregnancy outcomes have only assessed the effect of participation as a dichotomous exposure, giving no consideration to variations in intervention intensity, and the content of individualized care plans [10, 14, 15, 17]. This simple dichotomy fails to describe the amount of effort expended by both the CM and the enrolled woman to reach the targeted outcomes. Therefore, deriving a variable that captures this effort or “dosage” is necessary to more precisely measure the true effectiveness of PCM.
According to Slaughter and Issel [15], PCM dosage is characterized by three aspects: duration of enrollment, breadth of interventions, and amount of time spent with the CM. Duration of enrollment captures the length of time a woman is enrolled in PCM, beginning with the enrollment visit and ending with the targeted outcome or loss to follow up. Breadth of interventions describes the variety of intervention types received by the woman while enrolled. Finally, the amount of contact time measures the number of hours/minutes the woman spends with her CM during interventions delivered [15, 18].
In addition to a lack of attention to dosage, most studies of PCM may have been influenced by selection bias due to their study design. Like prenatal care (PNC) and the Special Supplemental Nutrition Program for Women, Infants and Children (WIC), different selection processes affect a woman’s likelihood to enter PCM. These processes do not occur at random, are often unobservable, and can be difficult to measure. Differences in selection processes may stem from variations in women’s and providers’ knowledge, attitudes, and perceptions surrounding the women’s health practices and health status [19, 20]. These factors are not only associated with women’s propensity to seek, enroll, and stay engaged in PCM, but also have an unknown contribution to the risk of having adverse pregnancy outcomes.
To control for selection bias, ideally researchers would conduct a randomized control trial (RCTs) and randomly assign women to varying levels of PCM dosage [21, 22]. Random allocation helps ensure that both unknown and known confounding and selection bias factors are evenly distributed between groups. Unfortunately, RCTs are expensive and may not be ethically feasible for high-risk pregnant women. Although some RCTs have been conducted in studies of PCM, most suffer from small sample sizes and lack of power to detect differences for rare outcomes (i.e., LBW and PTB) [10, 17]. The majority of PCM studies investigating pregnancy outcomes have been observational. In an observational study design groups are formed by “convenience” or due to a woman’s willingness to participate in the PCM program [22]. Consequently, the resulting treatment groups may have different distributions of maternal characteristics; direct comparison of these groups without accounting for differences could lead to biased estimates of treatment effect, selection bias [21, 23].
Researchers have traditionally used matching, stratification, and regression adjustment [23] to account for systematic differences in baseline characteristics and confounding. Unfortunately, these traditional methods have limits. Stratification and matching both suffer from dimensionality problems, [24, 25] while regression adjustment is limited by the amount of data available and degrees of freedom [23, 26]. This can lead to decreased statistical power and a failure to reject the null hypothesis (type II error).
Propensity score (PS) methods were first introduced by Rosenbaum and Rubin [27] as an alternative to traditional methods to account for selection bias in observational studies while examining the effect of a dichotomous treatment on a health outcome [23, 28, 29]. In recent years, PS methodology has been extended to assess dose–response relationships with health outcomes [26, 30].
To date, no study on the effectiveness of PCM has examined the dose–response relationship between PCM and pregnancy outcomes, while using PS methods to control for selection bias. The objective of this study was to assess whether PCM dosage is a more sensitive measure than a dichotomous participation measure when assessing the relationship between PCM and birth outcomes while controlling for selection bias using PS methods. We hypothesize that a measure of PCM dosage will have more power to detect a protective association with adverse birth outcomes than a dichotomous participation measure.
Methods
Iowa’s PCM Program
In Iowa, PCM is provided by twenty-one Maternal Health Service Agencies (MHSA) serving Iowa’s 99 counties. PCM interventions performed by MHSAs are paid for by Medicaid fees and Title V funding and include risk assessment, care coordination, health education, and nutrition education, assistance with tangibles, counseling, and workforce development. In 2010, approximately, 40 % of Iowa 38,514 births were reimbursed by Medicaid, and 28 % of these Medicaid births received PCM at a MHSA.
Study Population
We conducted a retrospective study of Medicaid-insured women residing in Iowa who had a singleton birth between October 2005 and December 2006. The cohort was constructed by linking Iowa’s birth certificate data, Medicaid claims, and Women’s Health Information System (WHIS) risk assessment data. Birth certificate and Medicaid claims data were linked a priori by the Iowa Department of Public Health. WHIS risk assessment data (90 %) were then probabilistically [31] linked to the birth certificate-Medicaid claims data by the authors using maternal date of birth; and maternal first, last, and maiden name. The constructed birth cohort consisted of 19,280 Medicaid births.
Based on variables from the birth certificate, women were excluded from analyses if maternal race was not White or Black (3.1 %), or if plurality >1 (2.7 %). To reduce bias from gestational age (GA) misclassification or underreporting of live births, women with GA <22 or >42 weeks (4.9 %) and/or birthweight <400 g (0.4 %) were excluded. GA was calculated from the date of last menstrual period (LMP). If the LMP-based gestation was missing or the absolute difference between the LMP-based gestation and the clinical estimate (CE) of GA >2 weeks, then the clinical estimate of gestation was used.
Women were also excluded if they had no WHIS risk assessment file but Medicaid claims data indicated that the women received PCM (5.6 %) since dates to determine enrollment into PCM were only available in the WHIS risk assessment file. After exclusions, there were 16,657 Medicaid births: 4,639 births (28.1 %) had PCM and 12,018 (72.1 %) had no PCM. This study was approved by the University of Illinois at Chicago, Office for the Protection of Research Subjects on October 13, 2009.
Dependent Variables
The dependent variables for this study were LBW and PTB. LBW was defined as birthweight <2,500 g and PTB was defined as GA <37 weeks among live births.
Maternal Characteristics (Covariates)
Covariates chosen for this analysis were maternal characteristics available on the birth certificate hypothesized to affect the association between PCM and the birth outcomes, LBW and PTB. Covariates were selected a priori based on published literature, biologic plausibility, and expert opinion [32–34]. Maternal characteristics were examined for their association with PCM and each birth outcome. Demographic variables from the birth certificate included maternal education (<high school, high school, >high school), marital status (married/unmarried), Hispanic ethnicity, maternal race (Black/White), maternal age (<18 years, 18–34 years, ≥35 years), and rural/urban residency (central city, metropolitan statistical area (MSA), rural adjacent to an urban area, and rural not adjacent to an urban area). Maternal medical conditions for LBW and PTB were defined as the following: diabetes, anemia, cardiac disease, lung disease, chronic hypertension, and renal disease; pregnancy associated hypertension, uterine bleeding, hydramnios, oligohydramnios, and incompetent cervix. Others considered for inclusion were parity (nulliparous, primiparous, 2, ≥3 live births), smoking during pregnancy, previous PTB (primiparous, multiparous with previous PTB, and multiparous no previous PTB), and adequacy of prenatal care entry. Month PNC began was defined by Kotelchuck’s Initiation of PNC Index [35] as inadequate (>6 months), intermediate (5–6 months), adequate (3–4 months), and adequate plus (1–2 months).
Independent Variables
PCM Any_Participation
PCM Any_Participation is a dichotomous measure of program participation. Women were classified as PCM participants if they had a WHIS risk assessment file. Non-participants were those women who had no WHIS risk assessment file and their Medicaid claims data indicated that they did not receive risk assessment, prenatal care coordination, or health education.
PCM Dosage
Based on the conceptual framework of Huber et al. [36] for case management, Slaughter and Issel [15] created a measure of dosage for PCM. Briefly, PCM dosage was defined as the average rank across 3 dimensions: duration of enrollment, breadth of intervention, and amount of contact time.
Duration of Enrollment
Since the outcomes for this study were LBW and PTB, duration of enrollment was measured as the number of weeks between the first PCM visit and the date of the infant’s birth or WHIS file discharge date. The first PCM visit was defined as the date of the initial risk assessment. Approximately, 2 % of PCM enrollees did not have an initial risk assessment; therefore, the date that their WHIS file was opened was used as a proxy for when contact with a case manager started. Duration was categorized into four groups: 0-none, 1-low (1–13 weeks), 2-medium (13–27 weeks), and 3-high (≥28 weeks). Category cutoffs were based on the number of weeks in a trimester.
Breadth of Intervention
The following interventions were included in the breadth dimension of PCM: (1) Medicaid Prenatal Risk Assessment (WHIS risk assessment data); (2) Care coordination visit (Medicaid Claims data); and (3) Health education (Medicaid Claims data). Breadth ranged from 0 to 3.
Amount of Contact Time
The amount of contact time (hours) spent with a CM during interventions was measured as the total amount of time billed to Medicaid for health education (15 min increments) or care coordination (30 min increments) visits. Time spent with a case manager during the initial risk assessment was not included since risk assessments were not billed in time increments. The amount of contact time was categorized as: 0-none, 1-low (0.1–1 h), 2-medium (1.1–3.25 h), and 3-high (≥3.25 h).
PCM dosage was categorized into 4 levels: none (0), low (0.1–1.4), medium (1.5–2.4), and high (2.5–3.0) based on the distribution of women’s scores.
Statistical Analysis
Descriptive statistics were used to summarize the distributions of the independent variables, dependent variables, and all maternal characteristics. Covariates were assessed for missing values. Although no single covariate exhibited more than 2 % missing values, exclusion of all records with missing data would have resulted in a loss of 6.6 % of the records. Since data were not missing at random, the expectation–maximization (EM) algorithm was used to impute missing values [37].
PS methods were used to control for overt selection bias: multiple PS methods for PCM dosage and binary PS methods for PCM Any_Participation. For PCM Dosage, we first checked for balance and comparability between each dosage level (none, low, medium, and high) on covariates related to both the exposure and the outcome, but not in the casual pathway; this was done by regressing each categorical maternal characteristic on PCM dosage via logistic regression (binary or multinomial). For continuous covariates, balance was checked using ANCOVA with PCM dosage as the fixed factor. Once balance was assessed, we estimated the multiple PS as specified by Spreeuwenberg et al. [25] Using multinomial logistic regression analysis we calculated a PS for each dosage level per individual in the dataset (four PS per individual). Next, to ensure compatibility between dosage groups we inspected the distributions of the multiple PS using box plots for extensive overlap between dosage groups. We also checked that balance was achieved (Table 1) on all covariates by regressing (binary or multinomial logistic) each covariate on the multiple PS and PCM dosage. The above analysis was repeated for the dichotomous measure PCM Any_Participation; however, we used binary logistic regression to calculate the PS (See Table 2 for balance check).
To estimate the effect of PCM dosage on the birth outcomes (LBW and PTB) while adjusting for covariates, a binary logistic regression model was used to regress PCM dosage and the multiple PS on each birth outcome. Next, binary logistic regression was used to regress PCM Any_Participation and the binary PS on each birth outcome. For each outcome, the PCM Dosage PS-adjusted odds ratios (OR) and 95 % confidence intervals (CI) were compared to the PCM Any_Participation Status PS-adjusted OR and CIs. The analysis was conducted using SAS V9.2.
Results
The maternal characteristics in relation to birth outcomes for the Iowa Medicaid birth cohort are presented in Table 3. A majority (89.8 %) of the women were between the ages of 18 and 34 years, White (93.0 %), had at least a high school education (71.2 %), and 88.7 % entered prenatal care by their fourth month of pregnancy. Approximately, 72 % of women had no PCM, while 6.1, 10.3, and 11.4 % had high, medium, or low dose PCM, respectively. Fewer than 7 % of the Medicaid-births were LBW, while 9.6 % were PTB.
PCM Dosage and PCM Any_Participation PS-adjusted ORs and 95 % CI for each adverse birth outcome are shown in Fig. 1. While controlling for maternal characteristics using multiple PS methods, the OR for the association between PTB and high PCM dosage was 0.88 (95 % CI 0.70–1.11), for medium PCM dosage was 0.58 (95 % CI 0.47–0.72), and for low PCM dosage was 1.43 (95 % CI 1.23–1.67). PS-adjusted OR for the dichotomous PCM Any_Participation measure was close to one and not statistically significant, (OR = 0.97, 95 % CI 0.82–1.09).
For LBW, the PS-adjusted OR was 0.76 (95 % CI 0.57–1.00) for high, 0.64 (95 % CI 0.50–0.82) for medium, and 1.36 (95 % CI 1.14–1.63) for low dosage PCM. Like PTB, the PS-adjusted OR for PCM Any_Participation was close to the null and not statistically significant (OR = 0.95, 95 % CI 0.82–1.09).
Discussion
Using an exploratory measure of PCM dosage, our study demonstrated a relationship between PCM and adverse pregnancy outcomes. On the other hand, no statistically significant associations were detected when using a dichotomous PCM exposure measure to evaluate the effect of PCM on adverse pregnancy outcomes. Receiving a moderate to high amount of PCM decreased a woman’s likelihood of having an adverse pregnancy outcome. In particular, women who received a medium dose of PCM showed a statistically significant reduction in the likelihood of having a LWB or PTB by 36 and 42 %, respectively.
Receiving a high dose of PCM corresponded with a 24 and 12 % reduction in the likelihood of having a LBW or PTB infant. Although the results for women who received a high dose of PCM were not statistically significant they do trend in a positive direction which has clinical significance. We hypothesize that the non-statistically significant results are due to differences in unmeasured health behaviors or other risk factors among “high dose” women that we were not able to control for using propensity score methods. Future research should examine the use of instrumental variables methods as a way to account for unmeasured selection bias.
In our study, a medium dose of PCM was related to the greatest reduction in risk followed by a high dose of PCM; this may be due to the fact that women with a medium dose of PCM had fewer risk factors than women in the high dose group and the intervention of health education and care coordination were able to better address the needs of the women who received a medium dose of PCM.To be included in the high dosage group women had to have been, at minimum, enrolled in PCM for at least 28 weeks, received all 3 types of interventions, and had at least 3.5 h of case manger time billed to Medicaid. In some respects, this characterization of a “high dose” seems more minimal than optimal. Further research is needed to investigate what would be an effective upper limit to a PCM dosage measure.
Women with a low dose of PCM had a 36 % higher risk of LBW and 43 % higher risk of PTB compared to women with no PCM. Women in the low dose category were enrolled in PCM for less than 13 weeks, only had the assessment intervention, and had less than 1 h of case manager time billed to Medicaid. A greater portion of the low PCM dose women were under 18 years of age, lived in rural areas and had inadequate PNC compared to women who did not receive PCM, as well compared to women in the high or medium dosage categories. These characteristics suggest that women with a low dosage of PCM may have a different set of issues and concerns (e.g., transportation, lack of financial resources) which compete for their attention and thus reduce their likelihood of staying engaged in PCM once enrolled [38]. A literature review focused on retention in PNC found that women with reduced resources who stay engaged in PNC appear to be on average healthier and more likely to engage in healthier lifestyle behaviors than those who are not retained [39]. This suggests that to optimize the effect of the dosage of PCM, motivational interventions may need to be incorporated into PCM program models to facilitate women’s engagement.
The success in finding a difference in PCM effects by dosage level constitutes a substantive advance in the evaluation of PCM. As noted by Issel et al. [17] in a literature review of 28 studies assessing the effectiveness of PCM on LBW and PTB, the dose of PCM received was not measured. In fact, only half of the studies indicated GA cutoff points for enrollment into PCM in their program descriptions. The measurement of PCM dosage is essential to understanding the relationship between PCM and birth outcomes. PCM programs engage in a complex process in which case managers match women’s needs to appropriate services for best outcomes. To measure PCM participation as a dichotomous exposure (e.g., participant vs. non-participant) implies a “one size fits all” approach and ignores important concepts such as timing of entry and program engagement. It also ignores the potential threshold that may exist, only above which a significant and beneficial relationship may develop. Examination of dose–response relationships allows us to ask four fundamental questions about interventions: (1) Is there evidence of an intervention effect? (2) Are there doses that lead a response different from that of the unexposed cohort? (3) What is the nature of the dose–response relationship? (4) What is the optimal dose? [40]
Using an exploratory dosage measure in studies of PCM in lieu of a dichotomous measure of PCM would allow researchers, providers, and policy makers to demonstrate the importance of the amount and type of interventions provided in PCM. For example, such a measure can be valuable to state agencies receiving funding from the Patient Protection and Affordable Care Act’s Maternal, Infant, and Early Childhood Home Visiting (MIECV) Program. In their applications, states stipulated how their PCM program model was to be implemented in targeted at-risk communities and provided their criteria for evaluating whether their programs addressed the specific needs of families in the target communities [41]. Adding a measure of dosage to their toolkit will allow for increased power in their demonstration of an effect as well as a clear understanding of the nature of the effect. In addition, the use of a dosage measure helps explicate the impact of interventions that are not being tested in RCT’s, but are implemented in real world settings.
The results presented here need to be considered in the context of this study’s limitations. Multiple propensity score methodology is a rigorous analytical tool; however, propensity score estimates are based on observed measured characteristics. Selection bias may still be present as a result of unmeasured factors that influence a woman’s likelihood to enroll and engage in PCM [23, 27]. Also, we chose to perform regression analysis instead of matching to correct for the multiple propensity scores when estimating the treatment effect of PCM dosage. We did this since matching on all four multiple propensity scores would have proven difficult and resulted in small treatment samples [25]. For consistency, regression analysis was also used to estimate the treatment effect of PCM Any_Participation on the birth outcomes.
Additionally, data quality and registration completeness are always an issue when using vital records. Possible underreporting of maternal medical risk factors and behaviors could have occurred [42]. Furthermore, this study used administrative data (Medicaid claims data); and only interventions which were billable and paid to Medicaid or required to be reported to the state were recorded and an underestimation of breadth of interventions and the amount of time spent with a case manager may have occurred. A study by Kane and Issel [43] examining the estimated cost of Medicaid PCM found that case managers spent 20–67 % of their time providing interventions not reimbursable by Medicaid, e.g., emotional support, coaching, and assistance with tangibles. Consequently, non-differential misclassification of the exposure is a possibility. In addition, there is a potential for preterm delivery bias in our exploratory measure of PCM dosage since women who deliver at term may receive more PCM than women who deliver preterm simply due to the length of their pregnancy and unrelated to their risk status. Approaches that incorporate pregnancy length into a new measure of PCM dosage are needed.
Finally, our findings may have limited generalizability to more heterogeneous populations since our population consisted of White Medicaid women who had live births in Iowa, a predominantly rural state. The degree of rural isolation has been associated with increased occurrence of LBW and PTB due a to differences in socioeconomic characteristics and access to health services [39]. In addition, white women may have different risk factors for poor birth outcomes than women of color as they do not experience racism or its associated stress. However, the homogeneity of our population provided assurance that there were fewer confounders.
Conclusion
In this study we were able to show that demonstrating a program’s effectiveness can be hindered by inadequate representation of intervention intensity or dosage. By using a measure of dosage which accounts for the amount of time a woman spends with her case manager, the duration of enrollment in PCM, and the breadth of interventions a woman receives while enrolled in PCM we were able to show a significant and beneficial relationship between PCM dosage and birth outcomes in a sample of Iowa Medicaid births. This has implications for both future research and practice with respect to PCM.
References
Issel, L. M., Anderson, R. A., & Kane, D. J. (2003). Administrative characteristics of comprehensive prenatal case management program. Public Health Nursing, 20(5), 349–360.
American Academy of Pediatrics. (1998). The role of home-visitation programs in improving health outcomes for children and families. Pediatrics, 101(3), 486–489.
Bradley, P. J., & Martin, J. (1994). The impact of home visits on enrollment patterns in pregnancy-related services among low-income women. Public Health Nursing, 11(6), 392–398.
Carabin, H., Cowan, L. D., Beebe, L. A., Skaggs, V. J., Thompson, D., & Agbangla, C. (2005). Does participation in a nurse visitation program reduce the frequency of adverse perinatal outcomes in first-time mothers? Paediatric and Perinatal Epidemiology, 19(3), 194–205.
Hodnett, E. D., & Fredericks, S. (2003). Support during pregnancy for women at increased risk of low birthweight babies. The Cochrane Database of Systematic Reviews, 3.
McNaughton, D. B. (2004). Nurse home visits to maternal-child clients: A review of intervention research. Public Health Nursing, 21(3), 207–219.
Olds, D. L., Henderson, C. R., Kitzman, H., Eckenrode, J., Cole, R., & Tatelbaum, R. (1998). The promise of home visitation: Results of two randomized trials. Journal of Community Psychology, 26(1), 5–21.
Sangalang, B. B., Barth, R., & Painter, J. S. (2006). First-Birth outcomes and timing of second Births: A statewide case management program for adolescent mothers. Health and Social Work, 31(1), 54–63.
Silva, R., Thomas, M., Caetano, R., & Aragaki, C. (2006). Preventing low birth weight in Illinois: Outcomes of the family case management program. Maternal and Child Health Journal, 10(6), 481–488.
Ciliska, D., Mastrilli, P., Ploeg, J., Hayward, S., Brunton, G., & Underwood, J. (2001). The effectiveness of home visiting as a delivery strategy for public health nursing interventions to clients in the prenatal and postnatal period: A systematic review. Primary Health Care Research & Development, 2(1), 41–54.
Keeton, K., Saunders, S. E., & Koltun, D. (2004). The effect of the family case management program on 1996 Birth outcomes in Illinois. Journal of Women’s Health, 13(2), 207–215.
Wells, N., Sbrocco, T., Hsiao, C. W., Hill, L. D., Vaugh, N. A., & Lockley, B. (2008). The impact of nurse case management home visitation on birth outcomes in African-American women. Journal of the National Medical Association, 100(5), 547–552.
Issel, L. M. (1997). Measuring comprehensive case management interventions: Development of a tool. Nursing Case Management, 4, 132–138.
Issel, L. M., Slaughter, J. C., & Forrestal, S. G. (2011). Prenatal case management of pregnant women: What is the evidence for its contribution to a reduction of disparities in perinatal outcomes? In A. Handler, J. Kennelly, & N. Peacock (Eds.), Reducing racial/ethnic disparities in reproductive and perinatal outcomes: The evidence from population-based interventions (pp. 209–238). New York: Springer.
Slaughter, J. C., & Issel, L. M. (2012). Developing a measure of prenatal case management dosage. Maternal and Child Health Journal, 16(5), 1120–1130.
Case Management Society of America (CMSA). (2008). Definition of case management. Available at: http://www.cmsa.org/Consumer/GlossaryFAQs/tabid/102/Default.aspx. Accessed 23 August 2012.
Issel, L. M., Forrestal, S. G., Slaughter, J., Wiencrot, A., & Handler, A. (2011). A review of prenatal home-visiting effectiveness for improving birth outcomes. Journal of Obstetric, Gynecologic, and Neonatal Nursing, 40(2), 157–165.
Huber, D., Sarrazin, M. V., Vaughn, T., & Hall, J. A. (2003). Evaluating the impact of case management dosage. Nursing Research, 52(5), 276–288.
Bell, J. F., & Zimmerman, F. J. (2003). Selection bias in prenatal care use by Medicaid recipients. Maternal and Child Health Journal, 7(4), 239–252.
Frick, K. D., & Lantz, P. M. (1999). Commentary: How well do we understand the relationship between prenatal care and birth weight? Health Service Research, 34(5 Part I), 1063–1073.
Rothman, K. J. (2002). Epidemiology: An introduction (1st ed.). New York: Oxford University Press.
Szklo, M., & Nieto, F. J. (2000). Epidemiology: Beyond the basics (1st ed.). Gaithersburg: An Aspen Publication.
D’Agostino, R. B. (1998). Tutorial in biostatistics propensity score methods for bias reduction in the comparison of a treatment to a non-randomized control group. Statistics in Medicine, 2281(19), 2265–2281.
Oakes, J. M., & Johnson, P. J. (2006). Propensity score matching for social epidemiology. In J. M. Oakes & J. S. Kaufman (Eds.), Methods in social epidemiology (pp. 370–392). San Francisco: Jossey-Bass Inc Pub.
Spreeuwenberg, M. D., Bartak, A., Croon, M. A., Hagenaars, J. A., Busschbach, J. J. V., Andrea, H., et al. (2010). The multiple propensity score as control for bias in the comparison of more than two treatment arms: An introduction from a case study in mental health. Medical Care, 48(2), 166.
Yanovitzky, I., Zanutto, E., & Hornik, R. (2005). Estimating causal effects of public health education campaigns using propensity score methodology. Evaluation and Program Planning, 28(2), 209–220.
Rosenbaum, P. R., & Rubin, D. B. (1983). The central role of the propensity score in observational studies for causal effects. Biometrika, 70(1), 41–55.
Hernán, M. A., & Robins, J. M. (2006). Instruments for causal inference: An epidemiologist’s dream? Epidemiology, 17(4), 360.
Hernán, M. A., & Robins, J. M. (2006). Estimating causal effects from epidemiological data. Journal of Epidemiology and Community Health, 60(7), 578–586.
Wang, J., Donnan, P. T., Steinke, D., & MacDonald, T. M. (2001). The multiple propensity score for analysis of dose–response relationships in drug safety studies. Pharmacoepidemiology and Drug Safety, 10(2), 105–111.
Campbell, K. M., Deck, D., & Krupski, A. (2008). Record linkage software in the public domain: A comparison of Link Plus, The Link King, and a ‘basic’ deterministic algorithm. Health Informatics Journal, 14(1), 5–15.
Goldenberg, R. L., & Culhane, J. F. (2007). Low birth weight in the United States. American Journal of Clinical Nutrition, 85(2), 584S.
Kiely, J. L., Yu, S., & Rowley, D. L. (1995). Low birth weight and intrauterine fetal growth restriction. In L. S. Wilcox & J. S. Marks (Eds.), From data to action: CDC’s public health surveillance for women, infants, and children (pp. 185–202). Washington, DC: US Department of Health and Human Services.
Kramer, M. S. (2003). The epidemiology of adverse pregnancy outcomes: An overview. Journal of Nutrition, 133(5 Suppl 2), 1592S–1596S.
Kotelchuck, M. (1994). The Adequacy of Prenatal Care Utilization Index: Its US distribution and association with low birthweight. American Journal of Public Health, 84(9), 1486–1489.
Huber, D., Hall, J., & Vaughn, T. (2001). The dose of case management interventions. Lippincott’s Case Management, 6(3), 119–126.
Allison, P. D. (2001). Missing data. Thousand Oaks: Sage Publications.
Tandon, S. D., Parillo, K., Mercer, C., Keefer, M., & Duggan, A. K. (2008). Engagement in paraprofessional home visitation: Families’ reasons for enrollment and program response to identified reasons. Women’s Health Issues, 18(2), 118–129.
Hillemeier, M. M., Weisman, C. S., Chase, G. A., & Dyer, A.-M. (2007). Individual and community predictors of preterm birth and low birthweight along the rural-urban continuum in central Pennsylvania. The Journal of Rural Health, 23(1), 42–48.
Ruberg, S. J. (1995). Dose response studies I. some design considerations. Journal of Biopharmaceutical Statistics, 5(1), 1–14.
Health Resource and Service Administration. (2010). Announcing $90 million in affordable care act funding for maternal, infant and childhood home visiting program grants. Available from: http://mchb.hrsa.gov/programs/homevisiting/index.html.
Lydon-Rochelle, M. T., Holt, V. L., Cárdenas, V., Nelson, J. C., Easterling, T. R., Gardella, C., et al. (2005). The reporting of pre-existing maternal medical conditions and complications of pregnancy on birth certificates and in hospital discharge data. American Journal of Obstetrics and Gynecology, 193(1), 125–134.
Kane, D. J., & Issel, L. M. (2005). Estimating Medicaid prenatal case management costs: The provider’s perspective. Nursing Economics, 23(4), 181.
Acknowledgments
Special thanks to the Iowa Department of Public Health for making the data used in this study available for my dissertation. Additional thanks to Arden Handler, Leslie Stayner, Deborah Rosenberg, Deborah Kane and Kristin Rankin for feedback on earlier versions. This research was funded, in part, by the Illinois Public Health Research Pre-doctoral Fellowship, the MCHB funded Maternal and Child Health Epidemiology Program, and the Training Program in Perinatal Epidemiology Grant (T32 HD046377).
Author information
Authors and Affiliations
Corresponding author
Rights and permissions
About this article
Cite this article
Slaughter, J.C., Issel, L.M., Handler, A.S. et al. Measuring Dosage: A Key Factor When Assessing the Relationship Between Prenatal Case Management and Birth Outcomes. Matern Child Health J 17, 1414–1423 (2013). https://doi.org/10.1007/s10995-012-1143-3
Published:
Issue Date:
DOI: https://doi.org/10.1007/s10995-012-1143-3