Abstract
Although under-diagnosed, anxiety and depression are among the most prevalent psychiatric disorders in children and adolescents, leading to severe impairment, increased risk of future psychiatric problems, and a high economic burden to society. Universal prevention may be a potent way to address these widespread problems. There are several benefits to universal relative to targeted interventions because there is limited knowledge as to how to screen for anxiety and depression in the general population. Earlier meta-analyses of the prevention of depression and anxiety symptoms among children suffer from methodological inadequacies such as combining universal, selective, and indicated interventions in the same analyses, and comparing cluster-randomized trials with randomized trials without any correction for clustering effects. The present meta-analysis attempted to determine the effectiveness of universal interventions to prevent anxiety and depressive symptoms after correcting for clustering effects. A systematic search of randomized studies in PsychINFO, Cochrane Library, and Google Scholar resulted in 30 eligible studies meeting inclusion criteria, namely peer-reviewed, randomized or cluster-randomized trials of universal interventions for anxiety and depressive symptoms in school-aged children. Sixty-three percent of the studies reported outcome data regarding anxiety and 87 % reported outcome data regarding depression. Seventy percent of the studies used randomization at the cluster level. There were small but significant effects regarding anxiety (.13) and depressive (.11) symptoms as measured at immediate posttest. At follow-up, which ranged from 3 to 48 months, effects were significantly larger than zero regarding depressive (.07) but not anxiety (.11) symptoms. There was no significant moderation effect of the following pre-selected variables: the primary aim of the intervention (anxiety or depression), deliverer of the intervention, gender distribution, children’s age, and length of the intervention. Despite small effects, we argue for the possible clinical and practical significance of these programs. Future evaluations should carefully investigate the moderators and mediators of program effects to identify active program components.
Similar content being viewed by others
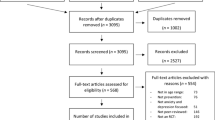
Avoid common mistakes on your manuscript.
Introduction
Anxiety and Depression
Anxiety and depression are very common in children and adolescents (Merry et al., 2011; Neil & Christensen, 2009). Costello, Mustillo, Erkanli, Keeler, and Angold (2003) found that the cumulative prevalence of these conditions in children up to 16 years old was 9.9 % for anxiety disorders and 9.5 % for depression. Anxiety and depression in children are associated with a variety of psychiatric diagnoses later in life (Bittner et al., 2007), are responsible for impairments in school performance and relationship difficulties with peers, and increase risk of suicidal behaviors and alcohol and substance abuse (Birmaher, Ryan, Williamson, Brent, & Kaufman 1996). In addition to high prevalence and severe consequences, only one in five of children and adolescents with anxiety or depression use mental health services (Essau, Conradt, & Petermann, 2000).
There is a strong relationship between anxiety and depression (Garber & Weersing, 2010). Anxiety predicts depression and vice versa (Costello et al. 2003), and there is a high rate of comorbidity between anxiety and depression in children and adolescents. Costello et al. (2003) found a comorbidity of approximately 30 % between anxiety and depression in children and adolescents. Several explanations have been suggested to elucidate the high comorbidity between these two conditions. Some evidence suggests that they are not completely distinct constructs and may share a common component, often referred to as negative affectivity, a general tendency to experience a wide range of negative emotions (Garber & Weersing, 2010; Seligman & Ollendick, 1998).
Prevention
The goal of prevention is to reduce the future appearance of negative outcomes, which is often attempted by reducing relevant risk factors and by strengthening relevant protective factors (Coie et al., 1993). In general, prevention programs for anxiety and depression are based on evidence-based cognitive-behavioral or interpersonal treatment programs (Donovan & Spence, 2000; Gladstone & Beardslee, 2009). When developed as prevention programs, these programs are designed to build skills as opposed to provide therapy, meaning strategies are learned for common situations that many people have either experienced or may experience, rather than specific situations derived from individual difficulties (Lowry-Webster, Barrett, & Dadds, 2001). The content of the program is often is very similar, irrespective whether the primary aim is to prevent anxiety or to prevent depression. The most extensively evaluated programs to prevent anxiety (FRIENDS for life; Barrett, 2004) and depression (Penn Resiliency Program; Gillham, Brunwasser, & Freres, 2008) contain basically the same components.
Several meta-analyses have found an overall positive effect of prevention strategies that target anxiety and depression (Fisak, Richard, & Mann 2011; Horowitz & Garber, 2006; Stice, Shaw, Bohon, Marti, & Rohde 2009; Teubert & Pinquart, 2011). Selective and indicated interventions, and those delivered by mental health professionals, have generally showed larger effect sizes than universal interventions and those delivered by school personnel (Stice et al., 2009; Teubert & Pinquart, 2011). Studies with younger children and a lower percentage of girls have yielded larger effect sizes in prevention for anxiety (Teubert & Pinquart, 2011), whereas studies of older children and a higher percentage of girls have been associated with larger effect sizes in prevention for depression (Horowitz & Garber, 2006; Stice et al., 2009).
Preventive efforts are classified into different categories, based on the characteristics of the target group. Universal prevention is defined as interventions that are implemented in a population that is not identified on the basis of potential risk or symptoms (Mrazek & Haggerty, 1994). There are several benefits associated with universal relative to selective and targeted interventions. Universal interventions provide the opportunity to reach individuals with limited access to treatment (Barrett & Pahl, 2006), and no one will be omitted as in some selective interventions due to inadequacies in screening tools for anxiety in the normal population (Donovan & Spence, 2000). In addition, although parents and teachers seem to readily identify depression in children, only a few consider psychiatric care (Puura et al., 1998). Universal interventions also show low dropout rates and might be one way to avoid stigma associated with participation in selected or targeted interventions (Fisak et al., 2011; Horowitz & Garber, 2006).
Focus of Our Study
Our study provides a unique contribution to research, concerning universal prevention for anxiety and depression, in several ways. First, the majority of universal prevention trials for anxiety and depression have allocated participants using randomization at the cluster level, which typically comprises schools or school classes (Fisak et al., 2011; Horowitz & Garber, 2006). Analyzing data from cluster-randomized trials using methods intended for individual randomization is, according to a famous quote, self-deception (Cornfield, 1978). Among others, Hedges (2007) has showed that clustering could have a considerable effect on the weights of effect sizes in meta-analyses. Recent meta-analyses (Fisak et al., 2011; Horowitz & Garber, 2006; Stice et al., 2009; Teubert & Pinquart, 2011) have typically included both randomized and cluster-randomized trials without any correction for clustering effects. In contrast, our study takes account of cluster randomization, and presents a more precise estimate of the effect of universal interventions. Second, moderators of outcome have been examined for universal, selective and indicated interventions in the same analyses (Fisak et al., 2011; Stice et al., 2009; Teubert & Pinquart, 2011). Combining the outcomes of different levels of prevention (universal, selected and indicated) will not provide a methodologically sound and accurate estimate of specific moderators of universal prevention. Our study examines five previously studied moderators (primary aim of the intervention, deliverer of the intervention, gender distribution, participants’ age, and length of the intervention), together with three additional moderators (follow-up duration, Penn Resiliency Program [for depressive symptoms], and FRIENDS for life [for anxiety symptoms]). Consequently, our study provides a more accurate examination of the moderators of outcomes in universal prevention than have previous meta-analyses. Third, recent meta-analyses have typically examined prevention targeting anxiety and depression separately (Fisak et al., 2011; Horowitz & Garber, 2006; Stice et al., 2009; Teubert & Pinquart, 2011). However, there are several reasons to investigate the efficacy of such programs in regards to both conditions. Much of the content of interventions to prevent anxiety and depression are obviously the same, and it is not uncommon that interventions aimed at preventing depression also affect anxiety and vice versa (Garber & Weersing, 2010; Lock & Barrett, 2003).
Our study addresses the following three questions: What is the weighted mean effect size of universal preventive interventions regarding (1) anxiety and (2) depressive symptoms? (3) Does the primary aim of the intervention, deliverer of the intervention, gender distribution, participants’ age, length of the intervention, follow-up duration, or using a specific program moderate effects on anxiety or depressive symptoms?
Method
Literature Search
We conducted a literature search in three steps in July of 2012. The first step comprised an electronic search on PsychINFO, Cochrane Library, and Google Scholar that included several search terms in different combinations to ensure the comprehensiveness of the search. We used the following search terms; prevent*, school-based, universal, community-based, anxiety, depress*, child* and youth*. We examined titles, abstracts or full texts depending on relevance, in order to exclude articles non-relevant to the research question. At the second step we examined relevant reviews (Briesch, Sanetti, & Briesch, 2010; Brunwasser, Gillham, & Kim, 2009; Calear & Christensen, 2010; Fisak et al., 2011; Horowitz & Garber, 2006; Jané-Llopis, Hosman, Jenkins, & Anderson, 2003; Merry et al., 2011; Neil & Christensen, 2009; Stice et al., 2009; Teubert & Pinquart, 2011). At the third step we did a hand search of all volumes since 1980 in journals occasionally publishing universal preventive intervention trials; Advances in School Mental Health Promotion (2007–2012), Behaviour Change (1999–2012), International Journal of Mental Health Promotion (1998–2012), Journal of Consulting and Clinical Psychology (1980–2012), Prevention & Treatment (1997–2003), School Mental Health (2009–2012), and the Journal of Primary Prevention (1980–2012).
Selection of Studies
As illustrated in Fig. 1 by a QUOROM flow chart (Moher et al., 1999), there were a total of 59 potentially relevant studies considered for a more detailed evaluation.
To be included in our meta-analysis, studies had to be (1) randomized controlled trials (RCTs) or cluster randomized controlled trials (C-RCTs); (2) peer-reviewed articles (in English) that were published in scholarly journals; (3) universal interventions, i.e. studies where participants or groups were not identified as at risk; (4) interventions primarily targeting anxiety or/and depression; and (5) interventions targeting school-aged children 6–18 years. We chose to include only randomized or cluster-randomized trials, because random assignment is crucial for generating unbiased estimates effects (Flay et al., 2005). According to the Cochrane handbook for systematic reviews of interventions (Higgins, 2008), non-randomized trials are only to be considered when effects cannot be adequately studied in randomized trials, or when interventions cannot be randomized. Including non-randomized trials simply to supplement existing randomized trials may, as argued in the Cochrane Handbook (2008), replace undesirable uncertainty with unacceptable error. We chose to include only peer-reviewed articles (in English), published in scholarly journals, to ensure the quality of the studies.
Based on the inclusion criteria, we excluded: (1) cohort and quasi-experimental studies; (2) studies not published in scholarly journals; (3) studies of selective and indicated interventions; (4) studies including programs targeting suicide-prevention, the prevention of externalizing symptoms, and the promotion of social-emotional development and well-being; and (5) interventions targeting pre-school children and young adults. The first and the second author separately examined these 59 studies relative to the inclusion and exclusion criteria and were unanimous in their decisions regarding 55 of the studies (92 %). After discussion with the third author we reached consensus for the remaining studies, which resulted in a total of 30 studies that met criteria for inclusion.
Coding Procedure
We coded studies on several variables: Mean age, gender distribution (percentage of girls), primary aim of the intervention (anxiety, depression or both), number of sessions, total length of the intervention, number of participants, intervention deliverer (teachers or mental health professionals), level of randomization (cluster or individual), participants blind to allocation, reports of intention to treat analyses, reports of adherence to manual, attrition-rate, and follow-up duration. To ensure reliability, the first and the second authors coded all studies separately. The inter-rater reliability was over .80 (kappa) for nominal variables and over .77 (ICC) for continuous variables. To reach consensus, the first and second authors recoded the studies for which they did not reach complete agreement.
Data Analysis
In studies with complex data structures, i.e., means and SDs provided for two or more subgroups within the sample, we calculated one composite mean and one composite SD. For the randomized trials, we estimated effect sizes and variances according to the approach suggested by Cohen (1987) using a standardized mean difference. For cluster-randomized trials, we calculated corrected effect sizes and variances. Hedges (2007) proposed that when comparing cluster-randomized studies to multisite studies using an individual assignment strategy, one should take both the variance within and between the clusters into account.
\( S_{Total}^{2} \) is the total variance, \( sd^{I} \) and \( sd^{C} \) the SDs for the intervention and control respectively. N I and N C the total number of participants in the intervention and control respectively, and N is the total number of participants in the intervention and condition combined. Finally, we estimated effect size (d) and variance \( (S_{d}^{2} ) \) for the cluster-randomized trials according to the following formulas:
where \( \overline{Y}^{I} \) and \( \overline{Y}^{C} \) are the means for the intervention and control respectively, S Total is the square root of the total variance \( (S_{Total}^{2} ) \), d 2 is the squared effect-size (d), and ρ is the intra cluster correlation (ICC) and n is the number of participants in each cluster. According to the What Works Clearinghouse Procedures and Standards Handbook, Version 3.0 (The Department of Education, 2014) ICCs reported in the trial should be used when controlling for clustering effects. However, only four of the cluster-randomized trials included in the present paper reported ICCs regarding anxiety or depression as outcomes, all ranging between .01 and .03 (Calear, Christensen, Mackinnon, Griffiths, & O’Kearney, 2009; Kraag, Van Breukelen, Kok, & Hosman, 2009; Miller et al., 2011a, b; Spence, Sheffield, & Donovan, 2003). The What Works Clearinghouse Procedures and Standards Handbook suggests an ICC of .10 as the default for behavioral or attitudinal outcomes in situations where ICCs are not reported, but also points out that different defaults could be used with explicit justifications dependent of the nature of the research. We made a broader search for ICCs for anxiety or depression as outcome studies focusing on children and adolescents, and found out three additional ICCs between .01 and .02 (Bond et al., 2004; Roberts, Kane, Bishop, Matthews, & Thomson, 2004; Trudeau, Spoth, Randall, & Azevedo, 2007). In order to avoid unduly conservative estimates of variance in CRTs where no ICCs were reported, we used an ICC of .03 (highest ICC of the identified studies) as a default. To ensure the validity of the results, we also performed a sensitivity analysis using the recommended default of .10.
There are several ways to estimate an aggregated effect size within a study when several dependent variables are used to report outcomes of the same theoretical construct. In order to present the typical effect size, and to control for the different number of dependent variables in the different studies, we calculated aggregated effect sizes and variances according to a recommendation by Borenstein, Hedges, and Higgins (2007) for studies that had two or more measures of the same construct. Lastly, we adjusted effect sizes and variances for sample size, according to Hedges (1981) for an unbiased estimate (Hedges g).
When calculating mean effect sizes, we used a random effect model (Borenstein et al., 2007), motivated by differences regarding design, participants’ characteristics, and implementation between studies. Effect sizes were therefore weighted by the inverse of the variance, taking both the variance between and within studies into account. We calculated a 95 % CI and a z value in order to determine the significance of the mean effect, and estimated the variance of the true effect size and conducted a significance test of the heterogeneity using the Q-statistics (Borenstein et al., 2007). We additionally assessed heterogeneity using the I2 statistic, which describes the percent of variability that is due to true variances in effect sizes rather than sample error. We interpreted the results of the I2 according to guidelines (Higgins, 2008).
Based on results from previous meta-analyses, we selected five variables a priori for moderation analyses: (1) primary aim of the intervention, (2) deliverer of the intervention (school personnel, mental health professionals), (3) gender distribution (percent of girls), (4) children’s age, and (5) the length of the intervention. In addition, we examined the most frequent interventions, namely the FRIENDS for life and the Penn Resiliency Program as moderators for anxiety and depressive symptoms respectively. For the dichotomous characteristics, we calculated summary effects within subgroups using a random-effects model with pooled estimates of Tau2 (Borenstein et al., 2007) and applied a Q-test based on analysis of variance to investigate differences between subgroups. For the continuous characteristics, we used random-effects meta-analysis regression to investigate any possible relationship between these characteristics and effect size using MetaAnalyst software (Wallace, Schmid, Lau, & Trikalinos, 2009).
Regarding follow-up, studies varied markedly in the duration of the follow-up periods. Several procedures have been suggested when examining follow-up periods in meta-analyses (Higgins, 2008). In order to include all studies reporting follow-up data, and to avoid entering only a subgroup of the studies in different analyses and thereby losing power, we chose the longest follow-up for each study. Although this procedure has been used in several recent meta-analyses (e.g., Stice et al., 2009; Teubert & Pinquart, 2011) it is somewhat controversial because it may give rise to heterogeneity (Higgins, 2008). To address this concern, we also examined follow-up duration as a moderator in the subgroup analyses. To address possible selection bias regarding studies reporting follow-up data, we calculated separate mean effect-sizes for studies reporting follow-up data, and for those not reporting follow-up data.
We used the Optimal Design Software (Spybrook, Raudenbush, Liu, Congdon, & Martínez, 2008), to calculate the power to detect a small effect size of d = .20 for the cluster-randomized trials.
Results
Study Characteristics
Table 1 shows a summary of included studies in the present meta-analysis. Of the total 30 studies included in this meta-analysis, 19 studies reported outcome data on anxiety symptoms and 26 reported outcome data on depressive symptoms. Fifteen studies reported data both on anxiety and depressive symptoms. One study (Tomba et al., 2010) was excluded from the analyses, because it only compared two active interventions.
The total number of participants in the 30 studies was 21,439. Girls constituted 52 % of the total sample, ranging from zero to 78 % across studies. The mean age of the total sample was 12.77 years (SD = 1.58). The primary aim of the intervention was to prevent depression in 13 studies, to prevent anxiety in ten studies, and to prevent both anxiety and depression in seven. The total number of sessions ranged from 3 to 30 sessions (Mdn = 10 sessions), and the total length of the interventions ranged from 2.3 to 22.5 h (Mdn = 10.9 h). School-personnel delivered the intervention in 15 studies, mental health professionals in 12 studies, both school personal and mental health professionals in two studies, and in one study the intervention was Internet-based. Twenty-one studies conducted randomization at a cluster level, leaving nine studies using randomization at an individual level. Six studies conducted an intent to treat analysis, 14 studies reported results of adherence to manual, and four studies blinded participants to allocation. Only three studies of the C-RCTs were adequately powered to identify a small effect size of d = 0.2.
Effect Sizes
Anxiety Symptoms: Mean Effect Size
There was no significant difference in anxiety symptoms at pre-intervention between the intervention and the control group N = 9635, g = .03 (95 % CI −.02 to .09), p = .19. At post-intervention, effect sizes ranged between −.31 and 1.73 (18 studies). Thirteen studies had a positive effect size and five studies a negative effect size. Relative to the control group, the intervention group showed significantly lower anxiety symptoms post-intervention: N = 9242, g = .13 (95 % CI .01–.26), p = .03. An assessment of heterogeneity indicated substantial heterogeneity, Tau 2 = .04; Q = 53.08, df = 17, p < .001; I 2 = 68 %. Follow-up measurements ranged from 3 to 36 months (Mdn = 10.5). Outcome data at follow-up were available in 12 of the 18 studies. At longest follow-up, effect sizes ranged between −.17 and .69. Seven studies had a positive effect size and five studies a negative effect size. There was no significant difference in anxiety symptoms at follow-up between the groups: N = 5797, g = .14 (95 % CI −.02 to .31), p = .08. Once again, we found substantial heterogeneity, Tau 2 = .06; Q = 51.23, df = 11, p < .001; I 2 = 79 %. When making calculations of effect sizes without any corrections for clustering, the effect-sizes were slightly larger (at post g = .14, p = .03; at follow up g = .15, p = .08).
Depressive Symptoms: Mean Effect Size
There was no significant difference in depressive symptoms at pre-intervention between the intervention and the control group N = 18,981, g = .02 (95 % CI −.04 to .07), p = .52. Effect sizes ranged between −.30 and 1.04 at post-intervention (25 studies). Nineteen studies had a positive effect size, one study had zero effect size, and five studies had a negative effect size. The intervention group showed significantly lower depressive symptoms post-intervention, relative to the control group: N = 17,254 g = .11 (95 % CI .03–.20), p = .008. An assessment of heterogeneity indicated substantial heterogeneity, Tau 2 = .02; Q = 63.42, df = 24, p < .001; I 2 = 62 %. Follow-up measurements ranged from 3 to 48 months (Mdn = 8.5). Outcome data at follow-up were available in 20 of the 25 studies. At longest follow-up, effect sizes ranged between −.23 and .68. Fifteen studies had a positive effect size, one study had zero effect size, and four studies had a negative effect size. The intervention group showed significantly lower depressive symptoms at follow-up, compared to the control group: N = 11,735, g = .10 (95 % CI .01–.18), p = .02. There was indication of moderate heterogeneity, Tau 2 = .02; Q = 44.87, df = 19, p < .001; I 2 = 58 %. None of the negative effect sizes was significantly different from zero, according to a 95 % CI of the effect size. When making calculations of effect sizes without any corrections for clustering, the effect-size was slightly smaller at post-intervention (g = .10, p = .006) and slightly larger at follow-up (g = .11, p = .01).
Sensitivity Analyses
When we performed mean effect size calculations using an ICC of .10, we found a significant difference in anxiety symptoms at post-intervention: g = .13 (95 % CI .01–.26), p = .04, but not at follow-up: g = .13 (95 % CI −.01 to .28), p = .06. Similarly, there was a significant difference in depressive symptoms at post intervention: g = .13 (95 % CI .03–.22), p = .01, but not at follow-up: g = .07 (95 % CI −.001 to .15), p = .051.
Moderation Analyses
Anxiety Symptoms
Table 2 shows summary effects and Q-test for the dichotomous variables. Based on the Q-test, we found no significant differences between interventions primarily targeting anxiety and interventions targeting both anxiety and depression or depression only; between interventions delivered by mental health professionals and those delivered by school personnel; or between interventions using the FRIENDS for life program and other programs. There was no significant difference in anxiety symptoms between intervention and control groups for any of the subgroups examined as dichotomous variables. Regarding the continuous characteristics investigated, neither the percent of girls (β = −.01, k = 18, p = .19) nor age moderated the effect of the intervention (β = .02, k = 13, p = .42). Similarly, the length of the intervention did not moderate the effect of the intervention (β = .00, k = 18, p = .20).
Depressive Symptoms
Table 2 shows summary effects and Q-tests for the dichotomous variables. Based on the Q-test, we found no significant differences between interventions primarily targeting depression and those targeting both anxiety and depression or anxiety only; between interventions delivered by mental health professionals and interventions delivered by school personnel; or between interventions using the Penn Resilience Program and other programs. The intervention group showed significantly lower depressive symptoms than the control group for interventions primarily targeting depression, interventions delivered by school personnel, and interventions not using the Penn Resilience Program at post-intervention, but not at follow-up. There was no significant difference in depressive symptoms between intervention and control groups for the other subgroups examined as dichotomous variables. Neither the length of the intervention (β = .00, k = 25, p = .42), age (β = .03, k = 25, p = .24), nor percent of girls moderated the effects of the intervention (β = −.01, k = 23, p = .56).
Publication Bias
A funnel plot is often used to identify publication bias in meta-analyses. For a non-biased selection of studies, the plot should be symmetric regarding the distribution of studies. The funnel plots (Fig. 2) in our study showed no obvious signs of publication bias, but a possible asymmetry with a smaller proportion of studies than expected reporting negative or zero effect sizes. The Egger test (Egger, Smith, Schneider, & Minder, 1997) showed no evidence of publication bias regarding anxiety intercept = .79 (95 % CI −.80 to 2.47), p = .33, or depression intercept = .77 (95 % CI −.61 to 2.14), p = .26.
Discussion
Summary
The purpose of our study was to undertake a meta-analysis on RCTs and C-RCTs of universal prevention for anxiety and depressive symptoms in school-aged children, 6–18 years old. Our meta-analysis shows that universal interventions displayed significantly lower anxiety and depressive symptoms at post-intervention, and lower depressive symptoms at follow-up, as compared to the control groups even after correcting for the clustering effects in C-RCTs. Subgroup analyses in recent meta-analyses have found seemingly smaller effect-sizes regarding depressive symptoms (Stice et al., 2009), and similar or somewhat larger effect-sizes regarding anxiety (Fisak et al., 2011; Teubert & Pinquart, 2011) for universal trials. These possible differences might partly be due to the failure to control for clustering.
There was no significant difference in anxiety symptoms between groups at follow-up. Our findings indicate that universal interventions do not reduce anxiety symptoms in the longer term. However, even though non-significant, the change from post to follow-up is very small and analyses are based on only 12 studies, almost all of them with cluster-randomized design, which tend to be characterized by low power. In contrast, subgroup analyses did not show any evidence of smaller effect sizes for long-term follow-ups relative to short-term follow-ups. A sensitivity analysis using a more conservative ICC revealed no significant difference in either anxiety symptoms or depressive symptoms at follow-up. Future reviews with larger samples of studies are needed to fully answer this question, and ICCs needs to be further evaluated in order to conduct more precise analyses. We found no evidence of a selection bias regarding what studies reported follow-ups when examining post-intervention effects for studies reporting follow-up and those that not reported follow-ups.
To explore heterogeneity, we performed several moderation analyses. Regarding anxiety symptoms, there was no indication of larger effects at post-intervention regarding interventions specifically aiming anxiety. In contrast, Teubert and Pinquart’s (2011) meta-analysis indicated that interventions primarily targeting anxiety produced larger effect sizes than those with anxiety prevention as a secondary purpose. However, Teubert and Pinquart analyzed both targeted and universal interventions together in the same moderation analysis. Since targeted interventions more often are specified and generally show larger effects (Stice et al., 2009; Teubert & Pinquart, 2011), their results might be partially explained by whether studies were targeted or universal. Regarding depressive symptoms, the effect of interventions primarily targeting depression was significantly larger than zero, but not significantly different from interventions targeting both anxiety and depression, or anxiety alone. According to our meta-analysis, there is no clear and well-defined moderation effect regarding the primary aim of the intervention in universal trials.
Other meta-analyses (Fisak et al., 2011; Stice et al., 2009; Teubert & Pinquart, 2011) have reported larger effect sizes for interventions delivered by psychologists. However, Stice et al. (2009) found a positive correlation between risk-status (targeted interventions) and professional deliverer. The reported larger effect sizes for interventions delivered by psychologists in other meta-analyses might therefore be partially explained by the risk-status of participants. In other words, there is more room for improvement among those with more symptoms (i.e., those participating in targeted intervention), and that might to some extent explain the larger effects noted. Even though not significantly different on the Q-test, effect sizes in our study seemed to be larger when mental health professionals delivered the intervention.
Earlier studies show contradictory results regarding percentage of girls, length of the intervention or age as moderating factors. In the present meta-analysis we did not find that they supported the outcome in universal interventions. Regarding percentage of girls, the null result is somewhat expected because the distribution of gender in universal interventions is about 50/50 in most studies.
The societal costs of anxiety and depression are very high (Johnston, Westerfield, Momin, Phillippi, & Naidoo, 2009; Rice & Miller, 1998). Universal interventions, especially shorter ones, implemented at relatively low cost (such as part of the school-curriculum) might be cost-effective and worthy of consideration. The effect sizes at post (.13 and .11 for anxiety symptoms and depressive symptoms respectively) might seem trivial, but must be interpreted with respect to the whole population, and thus may be of importance. Among others, Wilson and Lipsey (2007) argue for the clinical and practical significance of seemingly trivial effect sizes in large populations. Following their reasoning, suppose that anxiety scores, 1 SD above the mean, are of clinical interest, as suggested by several researchers (e.g., Reynolds & Richmond, 1978; Simon & Bögels, 2009). Applied on the most frequently used anxiety measure in this meta-analysis (i.e., the Revised Children’s Manifest Anxiety Scale), this suggests a recommended cut-off score of 19 out of 28 (Stallard, Velleman, Langsford, & Baldwin, 2001). The effect size describes the disparity between populations (intervention and control). Assuming normal distribution, the post effect size of .13 hypothetically means that about 12.9 % of the intervention group would score above the cutoff of 19 as compared to 15.9 % of the control group (i.e., dependent on the disparity of the populations, estimated by calculating the area under the curve above 19 for the two populations). This represents a reduction of 18.9 % individuals who score over the cut-off in the intervention group. However, the above estimation assumes that the effect size is the same throughout the whole population. Evidence suggests that small effect sizes in universal trials may involve larger effects for children and adolescents showing high levels of symptomatology (Chaplin et al., 2006; Horowitz et al., 2007; Lock & Barrett, 2003; Pössel, Baldus, Horn, Groen, & Hautzinger, 2005). This would imply an even larger reduction of children and youth at levels of clinical interest. Such a reduction would clearly be of practical significance for children, families, and schools, and of clinical significance as a supplement to evidence-based treatment.
Study Limitations and Future Directions
Our meta-analysis is limited insofar as it contains relatively few studies, which presents a problem particularly when calculating effect sizes at follow-up and when performing subgroups analyses. Another possible limitation is the exclusion of unpublished studies. There has been a debate as to whether to include unpublished trials in meta-analyses as a way of addressing publication bias (Moher et al., 1999). For example, Hopewell, McDonald, Clarke, and Egger (2007) found that published trials might overestimate the effect size, relative to informally published trials.
In view of the small effect sizes, an important future research question is to explore the possible enhancement of these programs. Mediating factors, and the implementation factors improving the effect, have not been thoroughly examined in this area of research. Another future research question is to better understand what strategies in an intervention affect anxiety relative to depression.
Lastly, when conducting future C-RCTs investigators should calculate power accurately because a small number of randomized units (often schools) decrease power. Many of the studies included in this meta-analysis were greatly underpowered. Several studies also lacked adequate statistical analyses with respect to clustering effects. To avoid spurious precision, future C-RCTs need to carefully report how clustering was taken into account in the analyses.
References
Aune, T., & Stiles, T. C. (2009). Universal-based prevention of syndromal and subsyndromal social anxiety: A randomized controlled study. Journal of Consulting and Clinical Psychology, 77, 867–879.
Baker, S. B., & Butler, J. N. (1984). Effects of preventive cognitive self-instruction training on adolescent attitudes, experiences, and state anxiety. Journal of Primary Prevention, 5, 17–26.
Barrett, P. M. (2004). Friends for life: Group leaders manual for children. Bowen Hills: Australian Academic Press.
Barrett, P. M., Farrell, L. J., Ollendick, T. H., & Dadds, M. (2006). Long-term outcomes of an Australian universal prevention trial of anxiety and depression symptoms in children and youth: An evaluation of the friends program. Journal of Clinical Child and Adolescent Psychology, 35, 403–411.
Barrett, P. M., & Pahl, K. M. (2006). School-based intervention: Examining a universal approach to anxiety management. Australian Journal of Guidance and Counselling, 16, 55–75.
Barrett, P., & Turner, C. (2001). Prevention of anxiety symptoms in primary school children: Preliminary results from a universal school-based trial. British Journal of Clinical Psychology, 40, 399–410.
Beck, A. T., Ward, C. H., Mendelson, M., Mock, J., & Erbaugh, J. (1961). An inventory for measuring depression. Archives of General Psychiatry, 4, 561–571.
Beidel, D. C., Turner, S. M., & Morris, T. L. (1995). A new inventory to assess childhood social anxiety and phobia: The Social Phobia and Anxiety Inventory for Children. Psychological Assessment, 7, 73–79.
Birmaher, B., Khetarpal, S., Brent, D., Cully, M., Balach, L., Kaufman, J., & Neer, S. M. (1997). The screen for child anxiety related emotional disorders (SCARED): Scale construction and psychometric characteristics. Journal of the American Academy of Child and Adolescent Psychiatry, 36, 545–553.
Birmaher, B., Ryan, N. D., Williamson, D. E., Brent, D. A., & Kaufman, J. (1996). Childhood and adolescent depression: A review of the past 10 years. Part II. Journal of the American Academy of Child and Adolescent Psychiatry, 35, 1575–1583.
Bittner, A., Egger, H. L., Erkanli, A., Jane Costello, E., Foley, D. L., & Angold, A. (2007). What do childhood anxiety disorders predict? The Journal of Child Psychology and Psychiatry, 48, 1174–1183.
Bond, L., Patton, G., Glover, S., Carlin, J. B., Butler, H., Thomas, L., & Bowes, G. (2004). The Gatehouse Project: Can a multilevel school intervention affect emotional wellbeing and health risk behaviours? Journal of Epidemiology and Community Health, 58, 997–1003.
Borenstein, M., Hedges, L. V., & Higgins, J. P. T. (2007). Introduction to meta-analysis. Hoboken, NJ: Wiley.
Briesch, A. M., Sanetti, L. M. H., & Briesch, J. M. (2010). Reducing the prevalence of anxiety in children and adolescents: An evaluation of the evidence base for the FRIENDS for Life program. School Mental Health, 2, 155–165.
Brunwasser, S. M., Gillham, J. E., & Kim, E. S. (2009). A meta-analytic review of the Penn Resiliency Program’s effect on depressive symptoms. Journal of Consulting and Clinical Psychology, 77, 1042–1054.
Calear, A. L., & Christensen, H. (2010). Systematic review of school-based prevention and early intervention programs for depression. Journal of Adolescence, 33, 429–438.
Calear, A. L., Christensen, H., Mackinnon, A., Griffiths, K. M., & O’Kearney, R. (2009). The YouthMood Project: A cluster randomized controlled trial of an online cognitive behavioral program with adolescents. Journal of Consulting and Clinical Psychology, 77, 1021–1032.
Chaplin, T. M., Gillham, J. E., Reivich, K., Elkon, A. G. L., Samuels, B., Freres, D. R., et al. (2006). Depression prevention for early adolescent girls: A pilot study of all girls versus co-ed groups. The Journal of Early Adolescence, 26, 110–126.
Chorpita, B. F., Yim, L., Moffitt, C. E., Umemoto, L. A., & Francis, S. E. (2000). Assessment of symptoms of DSM-IV anxiety and depression in children: A Revised Child Anxiety and Depression Scale. Behaviour Research and Therapy, 38, 835–855.
Clarke, G. N., Hawkins, W., Murphy, M., & Sheeber, L. (1993). School-based primary prevention of depressive symptomatology in adolescents: Findings from two studies. Journal of Adolescent Research, 8, 183–204.
Cohen, J. (1987). Statistical power analysis for the behavioral sciences. Hillside, NJ: Lawrence Erlbaum Associates.
Coie, J. D., Watt, N. F., West, S. G., Hawkins, J. D., Asarnow, J. R., Markman, H. J., et al. (1993). The science of prevention: A conceptual framework and some directions for a national research program. American Psychologist, 48, 1013–1022.
Cornfield, J. (1978). Randomization by group: A formal analysis. American Journal of Epidemiology, 108, 100–102.
Costello, E. J., Mustillo, S., Erkanli, A., Keeler, G., & Angold, A. (2003). Prevalence and development of psychiatric disorders in childhood and adolescence. Archives of General Psychiatry, 60, 837–844.
Donovan, C. L., & Spence, S. H. (2000). Prevention of childhood anxiety disorders. Clinical Psychology Review, 20, 509–531.
Döpfner, M., & Lehmkuhl, G. (2000). DISYPS-KJ: Diagnostik-system für psychische storungen im kindes- und jugendalter nach ICD-10 und DSM-IV. Bern: Huber.
Egger, M., Smith, G. D., Schneider, M., & Minder, C. (1997). Bias in meta-analysis detected by a simple graphical test. British Medical Journal, 315, 629–634.
Essau, C. A., Conradt, J., & Petermann, F. (2000). Frequency, comorbidity, and psychosocial impairment of anxiety disorders in German adolescents. Journal of Anxiety Disorders, 14, 263–279.
Essau, C. A., Conradt, J., Sasagawa, S., & Ollendick, T. H. (2012). Prevention of anxiety symptoms in children: Results from a universal school-based trial. Behavior Therapy, 43, 450–464.
Fisak, B. J, Jr, Richard, D., & Mann, A. (2011). The prevention of child and adolescent anxiety: A meta-analytic review. Prevention Science, 12, 255–268.
Flay, B. R., Biglan, A., Boruch, R. F., Castro, F. G., Gottfredson, D., Kellam, S., et al. (2005). Standards of evidence: Criteria for efficacy, effectiveness and dissemination. Prevention Science, 6, 151–175.
Garber, J., & Weersing, V. R. (2010). Comorbidity of anxiety and depression in youth: Implications for treatment and prevention. Clinical Psychology: Science and Practice, 17, 293–306.
Gillham, J. E., Brunwasser, S. M., & Freres, D. R. (2008). Preventing depression in early adolescence: The Penn Resiliency Program. In J. Z. Abela, B. L. Hankin, J. Z. Abela, & B. L. Hankin (Eds.), Handbook of depression in children and adolescents (pp. 309–322). New York, NY: Guilford Press.
Gillham, J. E., Reivich, K. J., Freres, D. R., Chaplin, T. M., Shatté, A. J., Samuels, B., et al. (2007). School-based prevention of depressive symptoms: A randomized controlled study of the effectiveness and specificity of the Penn Resiliency Program. Journal of Consulting and Clinical Psychology, 75, 9–19.
Gladstone, T. R. G., & Beardslee, W. R. (2009). The prevention of depression in children and adolescents: A review. The Canadian Journal of Psychiatry, 54, 212–221.
Hains, A. A. (1992). Comparison of cognitive-behavioral stress management techniques with adolescent boys. Journal of Counseling and Development, 70, 600–605.
Hains, A. A., & Ellmann, S. W. (1994). Stress inoculation training as a preventative intervention for high school youths. Journal of Cognitive Psychotherapy, 8, 219–232.
Hains, A. A., & Szyjakowski, M. (1990). A cognitive stress-reduction intervention program for adolescents. Journal of Counseling Psychology, 37, 79–84.
Hedges, L. V. (1981). Distribution theory for Glass’s estimator of effect size and related estimators. Journal of Educational Statistics, 6, 107–128.
Hedges, L. V. (2007). Effect sizes in cluster-randomized designs. Journal of Educational and Behavioral Statistics, 32, 341–370.
Higgins, J. P. (Ed.). (2008). Cochrane handbook for systematic reviews of interventions (Vol. 5). Chichester: Wiley-Blackwell.
Hopewell, S., McDonald, S., Clarke, M., & Egger, M. (2007). Grey literature in meta-analyses of randomized trials of health care interventions. Cochrane Database of Systematic Reviews, 2(2), 1–17.
Horowitz, J. L., & Garber, J. (2006). The prevention of depressive symptoms in children and adolescents: A meta-analytic review. Journal of Consulting and Clinical Psychology, 74, 401–415.
Horowitz, J. L., Garber, J., Ciesla, J. A., Young, J. F., & Mufson, L. (2007). Prevention of depressive symptoms in adolescents: A randomized trial of cognitive-behavioral and interpersonal prevention programs. Journal of Consulting and Clinical Psychology, 75, 693–706.
Jané-Llopis, E., Hosman, C., Jenkins, R., & Anderson, P. (2003). Predictors of efficacy in depression prevention programmes: Meta-analysis. The British Journal of Psychiatry, 183, 384–397.
Johnston, K., Westerfield, W., Momin, S., Phillippi, R., & Naidoo, A. (2009). The direct and indirect costs of employee depression, anxiety, and emotional disorders—An employer case study. Journal of Occupational Environmental Medicine, 51, 564–577.
Kellner, R. (1987). A symptom questionnaire. Journal of Clinical Psychiatry, 48, 268–274.
Kovacs, M. (1985). The children’s depression inventory (CDI). Psychopharmacology Bulletin, 21, 995–998.
Kraag, G., Van Breukelen, G. J. P., Kok, G., & Hosman, C. (2009). ‘Learn Young, Learn Fair’, a stress management program for fifth and sixth graders: Longitudinal results from an experimental study. Journal of Child Psychology and Psychiatry, 50, 1185–1195.
Lock, S., & Barrett, P. M. (2003). A longitudinal study of developmental differences in universal preventive intervention for child anxiety. Behaviour Change, 20, 183–199.
Lowry-Webster, H. M., Barrett, P. M., & Dadds, M. R. (2001). A universal prevention trial of anxiety and depressive symptomatology in childhood: Preliminary data from an Australian study. Behaviour Change, 18, 36–50.
Lowry-Webster, H. M., Barrett, P. M., & Lock, S. (2003). A universal prevention trial of anxiety symptomology during childhood: Results at 1-year follow-up. Behaviour Change, 20, 25–43.
March, J. S., Parker, J. D. A., Sullivan, K., & Stallings, P. (1997). The Multidimensional Anxiety Scale for Children (MASC): Factor structure, reliability, and validity. Journal of the American Academy of Child and Adolescent Psychiatry, 36, 554–565.
Meijer, P. G., Mellenbergh, G. J., & de Wit, C. A. (1986). De Korte Depressie-Vragenlijst voor Kinderen (KDVK). Nederlands Tijdschrift voor de Psychologie en haar Grensgebieden, 41, 364–368.
Merry, S., McDowell, H., Wild, C. J., Bir, J., & Cunliffe, R. (2004). A randomized placebo-controlled trial of a school-based depression prevention program. Journal of the American Academy of Child and Adolescent Psychiatry, 43, 538–547.
Merry, S. N., Hetrick, S. E., Cox, G. R., Brudevold-Iversen, T., Bir, J. J., & McDowell, H. (2011). Psychological and educational interventions for preventing depression in children and adolescents. Cochrane Database of Systematic Reviews. doi:10.1002/14651858.CD003380.pub3/abstract.
Messer, S. C., Angold, A., Costello, E. J., & Loeber, R. (1995). Development of a short questionnaire for use in epidemiological studies of depression in children and adolescents: Factor composition and structure across development. International Journal of Methods in Psychiatric Research, 5, 251–262.
Miller, L. D., Laye-Gindhu, A., Bennett, J. L., Liu, Y., Gold, S., March, J. S., et al. (2011a). An effectiveness study of a culturally enriched school-based CBT anxiety prevention program. Journal of Clinical Child and Adolescent Psychology, 40, 618–629.
Miller, L. D., Laye-Gindhu, A., Liu, Y., March, J. S., Thordarson, D. S., & Garland, E. J. (2011b). Evaluation of a preventive intervention for child anxiety in two randomized attention-control school trials. Behaviour Research and Therapy, 49, 315–323.
Miller, L. D., Short, C., Garland, E. J., & Clark, S. (2010). The ABCs of CBT (cognitive behavior therapy): Evidence-based approaches to child anxiety in public school settings. Journal of Counseling and Development, 88, 432–439.
Moher, D., Cook, D. J., Eastwood, S., Olkin, I., Rennie, D., & Stroup, D. F. (1999). Improving the quality of reports of meta-analyses of randomised controlled trials: The QUOROM statement. The Lancet, 354, 1896–1900.
Mrazek, P. J., & Haggerty, R. J. (1994). Reducing risks for mental disorders: Frontiers for preventive intervention research. Washington, DC US: National Academy Press.
Neil, A. L., & Christensen, H. (2009). Efficacy and effectiveness of school-based prevention and early intervention programs for anxiety. Clinical Psychology Review, 29, 208–215.
Pattison, C., & Lynd-Stevenson, R. M. (2001). The prevention of depressive symptoms in children: The immediate and long-term outcomes of a school based program. Behaviour Change, 18, 92–102.
Pössel, P., Baldus, C., Horn, A. B., Groen, G., & Hautzinger, M. (2005). Influence of general self-efficacy on the effects of a school-based universal primary prevention program of depressive symptoms in adolescents: A randomized and controlled follow-up study. Journal of Child Psychology and Psychiatry, 46, 982–994.
Pössel, P., Horn, A. B., Groen, G., & Hautzinger, M. (2004). School-based prevention of depressive symptoms in adolescents: A 6-month follow-up. Journal of the American Academy of Child and Adolescent Psychiatry, 43, 1003–1010.
Pössel, P., Seemann, S., & Hautzinger, M. (2008). Impact of comorbidity in prevention of adolescent depressive symptoms. Journal of Counseling Psychology, 55, 106–117.
Puura, K., Almqvist, F., Tamminen, T., Piha, J., Kumpulainen, K., Rasanen, E., et al. (1998). Children with symptoms of depression—What do the adults see? Journal of Child Psychology and Psychiatry, 39, 577–585.
Radloff, L. S. (1977). The CES-D Scale: A self-report depression scale for research in the general population. Applied Psychological Measurement, 1, 385–401.
Reynolds, C. R. (1980). Concurrent validity of What I Think and Feel: The Revised Children’s Manifest Anxiety Scale. Journal of Consulting and Clinical Psychology, 48, 774–775.
Reynolds, W. M., & Mazza, J. J. (1998). Reliability and validity of the Reynolds Adolescent Depression Scale with young adolescents. Journal of School Psychology, 36, 295–312.
Reynolds, C. R., & Richmond, B. O. (1978). What I think and feel: A revised measure of children’s manifest anxiety. Journal of Abnormal Psychology, 6, 271–280.
Rice, D. P., & Miller, L. S. (1998). Health economics and cost implications of anxiety and other mental disorders in the United States. The British journal of Psychiatry. Supplement, 34, 4–9.
Rivet Duval, E., Heriot, S., & Hunt, C. (2011). Preventing adolescent depression in Mauritius: A universal school-based program. Child and Adolescent Mental Health, 16, 86–91.
Roberts, C. M., Kane, R., Bishop, B., Cross, D., Fenton, J., & Hart, B. (2010). The prevention of anxiety and depression in children from disadvantaged schools. Behaviour Research and Therapy, 48, 68–73.
Roberts, C., Kane, R., Bishop, B., Matthews, H., & Thomson, H. (2004). The prevention of depressive symptoms in rural school children: A follow-up study. International Journal of Mental Health Promotion, 6, 4–16.
Ruini, C., Ottolini, F., Tomba, E., Belaise, C., Albieri, E., Visani, D., et al. (2009). School intervention for promoting psychological well-being in adolescence. Journal of Behavior Therapy and Experimental Psychiatry, 40, 522–532.
Sawyer, M. G., Harchak, T. F., Spence, S. H., Bond, L., Graetz, B., Kay, D., et al. (2010a). School-based prevention of depression: A 2-year follow-up of a randomized controlled trial of the beyondblue schools research initiative. Journal of Adolescent Health, 47, 297–304.
Sawyer, M. G., Pfeiffer, S., Spence, S. H., Bond, L., Graetz, B., Kay, D., et al. (2010b). School-based prevention of depression: A randomised controlled study of the beyondblue schools research initiative. Journal of Child Psychology and Psychiatry, 51, 199–209.
Seligman, L. D., & Ollendick, T. H. (1998). Comorbidity of anxiety and depression in children and adolescents: An integrative review. Clinical Child and Family Psychology Review, 1, 125–144.
Sheffield, J. K., Spence, S. H., Rapee, R. M., Kowalenko, N., Wignall, A., Davis, A., & McLoone, J. (2006). Evaluation of universal, indicated, and combined cognitive-behavioral approaches to the prevention of depression among adolescents. Journal of Consulting and Clinical Psychology, 74, 66–79.
Simon, E., & Bögels, S. M. (2009). Screening for anxiety disorders in children. European Child and Adolescent Psychiatry, 18, 625–634.
Spence, S. H. (1997). Structure of anxiety symptoms among children: A confirmatory factor-analytic study. Journal of Abnormal Psychology, 106, 280–297.
Spence, S. H., Sheffield, J. K., & Donovan, C. L. (2003). Preventing adolescent depression: An evaluation of the problem solving for life program. Journal of Consulting and Clinical Psychology, 71, 3–13.
Spence, S. H., Sheffield, J. K., & Donovan, C. L. (2005). Long-term outcome of a school-based, universal approach to prevention of depression in adolescents. Journal of Consulting and Clinical Psychology, 73, 160–167.
Spielberger, C. D., Edwards, C. D., Montouri, J., & Lushene, R. (1973). State-trait anxiety inventory for children. Palo Alto, CA: Consulting Psychology Press.
Spielberger, C. D., Gorsuch, R. L., & Lushene, R. (1970). Manual for the state-trait anxiety inventory. Palo Alto, CA: Consulting Psychologist Press.
Spybrook, J., Raudenbush, S. W., Liu, X. F., Congdon, R., & Martinez, A. (2008). Optimal design for longitudinal and multilevel research: Documentation for the “Optimal Design” software (Version 1.76) [Software manual]. Ann Arbor: University of Michigan.
Stallard, P., Velleman, R., Langsford, J., & Baldwin, S. (2001). Coping and psychological distress in children involved in road traffic accidents. British Journal of Clinical Psychology, 40, 197–208.
Stice, E., Shaw, H., Bohon, C., Marti, C. N., & Rohde, P. (2009). A meta-analytic review of depression prevention programs for children and adolescents: Factors that predict magnitude of intervention effects. Journal of Consulting and Clinical Psychology, 77, 486–503.
Teubert, D., & Pinquart, M. (2011). A meta-analytic review on the prevention of symptoms of anxiety in children and adolescents. Journal of Anxiety Disorders, 25, 1046–1059.
Tomba, E., Belaise, C., Ottolini, F., Ruini, C., Bravi, A., Albieri, E., et al. (2010). Differential effects of well-being promoting and anxiety-management strategies in a non-clinical school setting. Journal of Anxiety Disorders, 24, 326–333.
Trudeau, L., Spoth, R., Randall, G. K., & Azevedo, K. (2007). Longitudinal effects of a universal family-focused intervention on growth patterns of adolescent internalizing symptoms and polysubstance use: Gender comparisons. Journal of Youth and Adolescence, 36, 725–740.
Wallace, B. C., Schmid, C. H., Lau, J., & Trikalinos, T. A. (2009). Meta-analyst: Software for meta-analysis of binary, continuous and diagnostic data. BMC Medical Research Methodology, 9, 80.
Wilson, S. J., & Lipsey, M. W. (2007). School-based interventions for aggressive and disruptive behavior: Update of a meta-analysis. American Journal of Preventive Medicine, 33, 130–143.
Author information
Authors and Affiliations
Corresponding author
Ethics declarations
Conflict of interest
The authors have no conflict of interest to declare, financial or otherwise.
Appendix: Measures
Appendix: Measures
Several different measures were used in the studies. Measures of anxiety were in descending order, the Revised Children’s Manifest Anxiety Scale (RCMAS; Reynolds, 1980); State-Trait Anxiety Inventory for Adults (STAI; Spielberger, Gorsuch, & Lushene, 1970); Spence Children’s Anxiety Scale (SCAS; Spence, 1997); the Multidimensional Anxiety Scale for Children (MASC; March, Parker, Sullivan, & Stallings, 1997); the Screen for Child Anxiety Related Emotional Disorders (SCARED; Birmaher et al., 1997); State-Trait Anxiety Inventory for Children (STAI-C; Spielberger, Edwards, Montouri, & Lushene, 1973); in one study the Social Phobia and Anxiety Inventory for Children (SPAI-C; Beidel, Turner, & Morris, 1995); Symptom Questionnaire (SQ; Kellner, 1987).
Measures of depression were: in descending order, Children’s Depression Inventory (CDI; Kovacs, 1985); Center for Epidemiologic Studies-Depression Scale (CES-D; Radloff, 1977); Reynolds Adolescent Depression Scale (RADS; Reynolds & Mazza, 1998); Beck Depression Inventory (BDI; Beck, Ward, Mendelson, Mock, & Erbaugh, 1961); Symptom Questionnaire (SQ; Kellner, 1987); in one study, Mood and Feelings Questionnaire –short form (SMFQ; Messer, Angold, Costello, & Loeber, 1995); Revised Child Anxiety and Depression Scale (RCADS; Chorpita, Yim, Moffitt, Umemoto, & Francis, 2000); Short Depression Inventory for Children (SDIC; Meijer, Mellenbergh, & de Wit, 1986); The Self-Report Questionnaire-Depression (SBB-DES; Dopfner & Lehmkuhl, 2000).
Rights and permissions
About this article
Cite this article
Ahlen, J., Lenhard, F. & Ghaderi, A. Universal Prevention for Anxiety and Depressive Symptoms in Children: A Meta-analysis of Randomized and Cluster-Randomized Trials. J Primary Prevent 36, 387–403 (2015). https://doi.org/10.1007/s10935-015-0405-4
Published:
Issue Date:
DOI: https://doi.org/10.1007/s10935-015-0405-4