Abstract
On time start of the first case of the day is an important operating room (OR) efficiency metric, in which delays can have effects throughout the day. Although previous studies have identified various causes of first case start delays, none have attempted to evaluate the effect anesthesia staffing ratios have on first case start times. We performed a single-center retrospective analysis at an academic teaching hospital. Data was collected and analyzed over a period of 4 years and on more than 8,700 cases. We examined whether staffing ratios of attending only (solo staffing ratio), attending working with 1 resident/certified registered nurse anesthetist (CRNA) (1 to 1), or attending covering 2 residents/CRNAs (1 to 2) had a significant effect on first patient in room time (FPIR) and first case on time start (FCOTS). In addition, we examined whether staffing ratios had an effect on start times in various surgical subspecialties. We performed a univariate logistic regression analysis to determine if age, anesthesia base units, American Society of Anesthesiologists Physical Status (ASA PS) classification score, and staffing ratio was associated with FPIR and FCOTS being on time. Then, we performed a multivariate logistic regression analysis to determine if staffing ratio was associated with these outcomes, utilizing age, anesthesia base units, and ASA PS class as covariates. A decreased odds for FPIR being on time were seen in general and orthopedic surgeries when staffed 1 to 1, and cardiac surgery when staffed 1 to 2, when compared to solo staffing. FCOTS showed statistically significant differences when looking at all services with solo staffing having the highest odds for FCOTS being on time. This effect was seen also when analyzing only oncologic and orthopedic surgeries. Hospitals should consider using different staffing ratios in different surgical specialties to minimize delays and maximize OR efficiency.
Similar content being viewed by others
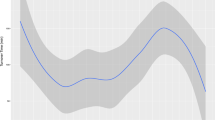
Avoid common mistakes on your manuscript.
Introduction
Operating rooms (OR) are expensive to run and in the recent healthcare landscape of decreasing reimbursement and budget restraints, OR efficiency is becoming an ever-increasing priority for hospitals [1–4]. The on-time start of the first case of the day is often used as a metric for measuring efficiency in the OR [5]. Delays in the first cases of the day often can result in subsequent case delays [6, 7]. These delays may interrupt workflow in the OR, thereby decreasing patient and surgeon satisfaction [8, 9]. In addition, the effects are felt throughout the hospital as post-surgical bed availability on the inpatient floors and in the intensive care unit are also interrupted by OR case delays. Two important time metrics to monitor include first case on-time start (FCOTS), defined as time at which anesthesia induction was marked as complete, and first patient in room (FPIR), defined as the time the patient entered the OR.
Numerous studies have examined ways to improve timeliness of first case starts [8, 10–12] by proposing solutions that involve streamlining the pre-operative process [13] and utilizing peri-operative checklists and facilitators [5]. In a previous study, multiple causes for delays were identified including issues with consent, late arrival of surgeons, and nursing issues, among others [14]. Several systematic changes were implemented, including earlier completion of preoperative surgical tasks in patients receiving regional and neuraxial anesthesia, encouraging surgical consents to be signed by patients in clinic as opposed to on the day of surgery, and changing the circulator nurse workflow, which resulted in a 12 % improvement in FCOTS [14].
One area that has not been addressed in the efficiency of on time first case starts is the effect of the staffing ratio (the number of ORs an attending anesthesiologist is managing). At academic medical centers, anesthesiologists often work in the anesthesia care team model, where they are responsible for supervising multiple rooms staffed by residents and/or certified registered nurse anesthetists (CRNAs) [15]. In one Dutch study of academic medical centers, having extra anesthesiologists available to help with induction improved FCOTS [16]. Both prediction models [17] and previous studies have shown that staffing ratios have a large effect on supervision lapses during first-case starts [18], which could potentially impact patient safety and contribute to adverse outcomes. However, no previous study to our knowledge has attempted to evaluate the effect staffing ratios have had on first case timeliness. The purpose of this study was to analyze the effect staffing ratios have on FPIR and FCOTS at an academic institution. In addition, we examined whether staffing ratios had an effect on these metrics within various surgical subspecialties.
Materials and methods
Data collection
Each operating room has a computer for the circulating nurse to log perioperative times, including patient arrival into the operating room, induction start and induction complete. First case start times were excluded if the case was added on that morning or if the order of cases was changed. Only cases originally scheduled for 7:30 am (9:30 am on Wednesdays) were included in the study. The Center for Clinical Excellence, an already established group that focuses on patient quality and safety at our hospital, was responsible for data acquisition, analysis, and reporting. FPIR on time was defined as the patient entering the OR by 7:30, except for Wednesdays when elective cases start at 9:30. FCOTS was defined as the time from patient in room to induction complete. The cutoff was 20 min for all cases except for cardiac and neurosurgery, which had a cutoff of 35 min.
Statistical analysis
R Project for Statistical Computing (R version 3.1.2) was used to perform all statistical analysis. FPIR and FCOTS metrics between the years 2010 to 2014 were collected and analyzed. Data for FPIR and FCOTS were available for 33,034 and 38,673 cases, respectively. For each case in FPIR dataset, data included surgical specialty, time in room, expected first case to start time, binary value for whether case was in room on time (yes or no), staffing ratio, American Society of Anesthesiologists Physical Status Classification (ASA PS), age, and anesthesia base units. For the FCOTS dataset, similar data was collected except it included time in which induction was marked complete and excluded any FPIR-related data. All cases that had missing data for any of these data points were excluded from the analysis. We analyzed three different staffing ratios: solo staff anesthesiologists, 1 to 1 ratio (one staff and one resident or CRNA), and 1 to 2 ratio (one staff covering two operating rooms with a resident or CRNA in each room). All cases with unknown staffing ratio or a 1 to 3 staffing ratio (defined as one staff attending covering three operating rooms) were excluded from the study. The latter was excluded because of the small number of such cases with this ratio performed at our institution. A univariate logistic regression model was fitted to the data to determine if ASA PS, age group, base units, or staffing ratios were associated with FPIR and FCOTS. The following reference groups were chosen for each group: ASA PS I for ASA PS, age less than 50 in the age group (compared to 50–64 years old, 65–79 years old, and > 80 years old), base units less than or equal to 5 in the base units group (compared to 6–10, 11–15, or > 15), and solo staffing ratio (compared to 1 to 1 and 1 to 2). All were associated with FCOTS and FPIR outcomes and were therefore included in the subsequent multivariate logistic regression analysis. For both FPIR and FCOTS, a multivariate logistic regression model was fitted to the data to determine if staffing ratios were associated with FPIR and FCOTS. ASA PS, age group, and anesthesia base units (ABU) were used as covariates for this model. This analysis was done for all surgeries and then separately for each surgery group. Results were reported as odds ratios (OR) with their corresponding 95 % confidence interval (CI). ORs were considered statistically significant if the 95 % CI did not include 1.00 within its range and if p values <0.05. Billing data consisting of the ABU value was used to control for case complexity. Base units range from 3 to 25 per anesthesia CPT code.
Results
Intraoperative data was collected from the years 2010–2014. There were 33,034 and 38,673 cases available with data for FPIR and FCOTS, respectively. After exclusion criteria, as described in the methods, there were 32,608 cases included for FPIR analysis and only 8,727 available for FCOTS. The reduced number of cases with FCOTS data was due to lack of ABU data available in this group.
Univariate analysis
Higher ASA PS class was associated with a decreased likelihood for both FPIR and FCOTS. Patient age greater than 80 was associated with a decreased likelihood of FPIR and FCOTS. Age 65–79 was only associated with decreased FCOTS. Higher ABU was associated with increased likelihood of FPIR on time. Only ABU 6–10 showed a similar trend for FCOTS times. High staffing ratios was associated with decreased probability of both FPIR and FCOTS (Table 1).
FPIR
An analysis across all surgical services combined demonstrated no statistically significant differences in FPIR time based on staffing ratios, while controlling for ASA PS, age and ABUs (Table 2). However, there were a few specific staffing ratios and services that showed statistically significant FPIR times. Pain service had a statistically significant increased likelihood of FPIR on time when staffed 1 to 2 compared to solo and 1 to 1 staffing. Cardiac surgery had a significantly decreased likelihood of FPIR on time when staffed 1 to 2 compared to solo and 1 to 1 staffing. General and orthopedic surgeries had a decreased likelihood of FPIR on time when staffed 1 to 1 compared to solo and 1 to 2 staffing.
FCOTS
Overall, there was statistically significant difference in FCOTS across all services (Table 3) when controlling for ASA PS, age and ABUs. Solo staffing ratio had the highest likelihood of FCOTS compared to 1 to 1 and 1 to 2 staffing ratios. Within surgical services, oncologic and orthopedic surgeries showed significant decrease in likelihood of on-time start when staffed 1 to 1 and 1 to 2 compared to solo attendings. Cardiac and general surgeries had a decreased likelihood of on-time start when staffed 1 to 2 compared to 1 to 1 and solo attendings.
Discussion
There is limited published data analyzing the effects of anesthesia staffing ratios on OR time metrics. Here, we reported our institutional data related to how these various ratios affected FPIR and FCOTS. By understanding how variations in an anesthesia team model can change efficiency measures, institutions can develop better strategies to improve surgical workflow.
Rooms staffed 1 to 2 had a lower FPIR on-time percentage for cardiac surgeries compared to solo and rooms staffed 1 to 1. A likely reason for the delay is due to preoperative procedures. For example, the vast majority of our cardiac patients receive an arterial line prior to their surgery. In a study by Koenig et al. at a British teaching hospital, they found that induction time correlated with the number of invasive lines and procedures done [19]. At their institution, most of their procedures were done in the OR either immediately before or after induction. At our institution, because we do a portion of our procedures in the preoperative area, delays may be reflected more in FPIR times. If an attending has 2 patients that both need arterial lines and the resident or CRNA encounters difficulty placing either line, one or both rooms may have a delayed FPIR time.
For general and orthopedic surgeries, rooms staffed 1 to 1 had a lower likelihood of patient arriving in room on time compared to solo attendings and 1 to 2 staffing ratios. New anesthesia residents are often assigned 1 to 1 to a room for 2 months at the beginning of their training. This is done to acclimate the residents to the new OR environment and help them with the steep learning curve of basic anesthesia. In this scenario, if additional procedures, such as arterial lines, epidurals, or peripheral nerve blocks need to be done pre-operatively, the inexperience of the new residents may result in delay in patient arrival into the OR [19].
When we examined time starts across all specialties, cases staffed solo were more likely to start on time than those staffed 1 to 1 and 1 to 2 (Table 3). This is not surprising and previous studies have shown similar results [20, 21]. Similar to the reasons mentioned above for FPIR, experience with procedures can have an effect on induction times as oftentimes invasive lines are placed post-induction. In addition, for cases staffed 1 to 2, an attending has to induce in 2 rooms sequentially. If induction in the first room takes longer than anticipated, due to an unanticipated difficult airway or hemodynamic instability, or if the patient does not arrive into the room on time due to aforementioned reasons, then the start time of the second room will also be delayed.
Given the recent changes in healthcare and focus on cost-cutting measures, the hospital may start paying more attention to the anesthesia staffing ratios especially if efficiency can be preserved while increasing the number of rooms covered by the attending anesthesiologist. Still, the decision to hire fewer anesthesiologists and thus have a higher staffing ratio must be balanced against the potential delays and missed earning opportunities that may result. Conversely, based on decision-analysis models, having an anesthesiologist work every case solo is not cost effective [22]. Given the results of our study, it may be worthwhile to consider different staffing ratios for different surgical services to optimize cost and productivity without compromising patient safety.
There are several limitations of our study. First, the times used for calculation of FPIR and FCOTS are manually entered by the OR circulator nurse. As a result, there is likely a small amount of variability in the accuracy of those times due to human error. Also, there may be some subjectivity in regards to the induction complete time used to calculate FCOTS as what one nurse considers induction complete may not be the case for another nurse or the anesthesia provider. Second, there are supervisees with a wide variety of anesthesia experience at our institution—from very experienced CRNAs to senior level residents to relatively inexperienced first year anesthesia residents and interns. Our data does not include the type of supervisee in each staffing ratio. The experience of the supervisee likely plays a role in the FPIR and FCOTS times, especially for cases that require pre-induction procedures such as lines and/or blocks as more experienced providers are likely more efficient at doing procedures. Lastly, while we were able to analyze FPIR and FCOTS times by surgical subspecialty while controlling for certain variables such as ABUs and patient age and ASA status, the specific type of case was not included in the data. For example, in our analysis of cardiac surgery cases we included all types of procedures performed by cardiac surgeons and covered by anesthesiologists. We believe that the ABU can serve as a reasonable proxy for case complexity which can influence anesthesia staffing assignments. Given the wide variability in case complexity in most surgical subspecialties at our institution, it may be worthwhile to investigate whether there are significant differences in FPIR and FCOTS times for specific types of cases within each subspecialty.
References
Malapero, R. J., et al., An anesthesia medication cost scorecard–concepts for individualized feedback. J. Med. Syst. 39:48, 2015.
Eijkemans, M. J., et al., Predicting the unpredictable: a new prediction model for operating room times using individual characteristics and the surgeon’s estimate. Anesthesiology 112:41–49, 2010.
Krupka, D. C., and Sandberg, W. S., Operating room design and its impact on operating room economics. Curr. Opin. Anaesthesiol. 19:185–191, 2006.
Kodali, B. S., Kim, D., Bleday, R., Flanagan, H., and Urman, R. D., Successful strategies for the reduction of operating room turnover times in a tertiary care academic medical center. J. Surg. Res. 187:403–411, 2014.
Panni, M. K., et al., Improving operating room first start efficiency - value of both checklist and a pre-operative facilitator. Acta Anaesthesiol. Scand. 57:1118–1123, 2013.
Wong, J., Khu, K. J., Kaderali, Z., and Bernstein, M., Delays in the operating room: signs of an imperfect system. Can J Surg J Can de chirurgie 53:189–195, 2010.
Mazzei, W. J., Operating room start times and turnover times in a university hospital. J. Clin. Anesth. 6:405–408, 1994.
Harders, M., Malangoni, M. A., Weight, S., and Sidhu, T., Improving operating room efficiency through process redesign. Surgery 140:509–514, 2006. discussion 514–506 2006.
Vitez, T. S., and Macario, A., Setting performance standards for an anesthesia department. J. Clin. Anesth. 10:166–175, 1998.
Overdyk, F. J., Harvey, S. C., Fishman, R. L., and Shippey, F., Successful strategies for improving operating room efficiency at academic institutions. Anesth. Analg. 86:896–906, 1998.
Gabriel, R. A., Gimlich, R., Ehrenfeld, J. M., and Urman, R. D., Operating room metrics score card-creating a prototype for individualized feedback. J. Med. Syst. 38:144, 2014.
Peccora, C. D., et al., Anesthesia report card - a customizable tool for performance improvement. J. Med. Syst. 38:105, 2014.
Wright, J. G., Roche, A., and Khoury, A. E., Improving on-time surgical starts in an operating room. Can J Surg J Can de chirurgie. 53:167–170, 2010.
Saw, N., et al. Process Redesign to Improve First Case Surgical Starts in an Academic Institution. Journal of investigative surgery : the official journal of the Academy of Surgical Research 2014.
Kodali, B. S., Kim, K. D., Flanagan, H., Ehrenfeld, J. M., and Urman, R. D., Variability of subspecialty-specific anesthesia-controlled times at two academic institutions. J. Med. Syst. 38:11, 2014.
van Veen-Berkx, E., Elkhuizen, S. G., Kalkman, C. J., Buhre, W. F., and Kazemier, G., Successful interventions to reduce first-case tardiness in Dutch university medical centers: results of a nationwide operating room benchmark study. Am. J. Surg. 207:949–959, 2014.
Paoletti, X., and Marty, J., Consequences of running more operating theatres than anaesthetists to staff them: a stochastic simulation study. Br. J. Anaesth. 98:462–469, 2007.
Epstein, R. H., and Dexter, F., Influence of supervision ratios by anesthesiologists on first-case starts and critical portions of anesthetics. Anesthesiology 116:683–691, 2012.
Koenig, T., et al., Estimating the time needed for induction of anaesthesia and its importance in balancing anaesthetists’ and surgeons’ waiting times around the start of surgery. Anaesthesia 66:556–562, 2011.
Urman, R. D., Sarin, P., Mitani, A., Philip, B., and Eappen, S., Presence of anesthesia resident trainees in day surgery unit has mixed effects on operating room efficiency measures. The Ochsner J. 12:25–29, 2012.
Davis, E. A., et al., Resident teaching versus the operating room schedule: an independent observer-based study of 1558 cases. Anesth. Analg. 103:932–937, 2006.
Glance, L. G., The cost effectiveness of anesthesia workforce models: a simulation approach using decision-analysis modeling. Anesth. Analg. 90:584–592, 2000.
Author information
Authors and Affiliations
Corresponding author
Additional information
This article is part of the Topical Collection on Systems-Level Quality Improvement
Rights and permissions
About this article
Cite this article
Chen, Y., Gabriel, R.A., Kodali, B.S. et al. Effect of Anesthesia Staffing Ratio on First-Case Surgical Start Time. J Med Syst 40, 115 (2016). https://doi.org/10.1007/s10916-016-0471-z
Received:
Accepted:
Published:
DOI: https://doi.org/10.1007/s10916-016-0471-z