Abstract
The objective of this study is to examine risk factors of physical aggression against a partner in a large representative Active Duty Air Force sample. A stratified sample of 128,950 United States Active Duty members were invited to participate in an Air Force-wide anonymous online survey across 82 bases. The final sample (N = 52,780) was weighted to be representative of the United States Air Force. Backward stepwise regression analyses were conducted to identify unique predictors of partner physical aggression perpetration within and across different ecological levels (individual, family, organization, and community levels). Relationship satisfaction, alcohol problems, financial stress, and number of years in the military were identified as unique predictors of men’s and women’s perpetration of violence against their partner across ecological levels. Parental status, support from neighbors, personal coping, and support from formal agencies also uniquely predicted men’s but not women’s perpetration of violence across ecological levels. This study identified specific risk factors of partner violence that may be targeted by prevention and intervention efforts aimed at different levels of impact (e.g., family interventions, community-wide programs).
Similar content being viewed by others
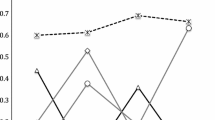
Avoid common mistakes on your manuscript.
Introduction
Intimate partner violence is a major public health problem, affecting millions of American families each year. Prevalence of physically aggressive acts toward partners in the United States is estimated at approximately 16% [1]. Partner aggression is associated with major depressive episodes and posttraumatic stress disorder in women, poorer health, substance abuse, depressive symptoms, and injury among men and women [2–5]. Although both women and men are injured by their aggressive partners, injury is more likely for women than men [5, 6]. Longitudinal studies also show that physical aggression against women and men is associated with marital discord and divorce [7].
Partner violence is as much of a problem in military communities as civilian ones [8]. Each year, the United States Air Force substantiates approximately 2,900 partner maltreatment incidents. Over $30 million is spent each year on the Air Force Family Advocacy Program to prevent, assess, and treat family maltreatment and to maintain a central registry of substantiated cases. Concern about partner violence in the military led to the formation of a congressionally mandated task force on domestic violence in the Department of Defense. Not unlike in civilian communities, both perpetrators and victims are motivated to keep maltreatment incidents private. Recent data suggest that fewer than 10% of individuals involved in a severely partner abusive relationship in the Air Force have come to anyone’s formal or informal attention for these problems, which makes outreach and prevention efforts targeting the continuum of aggression critical [9].
The risk and protective factor literature on partner aggression is extensive [10, 11]. Most of this work has focused on a few factors drawn from specific theoretical approaches to partner abuse. Dutton argued that we could improve our understanding of partner abuse by examining a broader range of potential causes that vary in their ecological level of origin and level of influence with an eye to understanding how these factors fit together [12]. Levels described by Bronfenbrenner included macrosystem (societal), exosystem (contextual such as workplace stressors), microsystem (household or relationship) and ontogenetic (individual) factors [13]. Integration of many potential causes assessed across multiple ecological levels might be a necessary step in the development of more effective approaches to preventing partner aggression. Some multivariate models have been tested that evaluated how subsets of predictor variables from specific theoretical approaches relate to one another and to partner aggression [14–19]. However, this research remains relatively new and further research is necessary to elucidate how factors might operate across levels.
In this paper, we use a large, representatively sampled survey data set of active duty Air Force members to investigate a series of hypotheses about risk factors for perpetration of partner violence across the full continuum of aggression, ranging from milder acts (e.g., pushing, slapping) to more serious, potentially injurious acts (e.g., punching, kicking hard, burning). Based on the existing literature a variety of variables reflecting individual functioning (e.g., depressive symptoms, general self-efficacy), relationship/family functioning (e.g., relationship satisfaction, family coping ability), workplace functioning (e.g., workgroup cohesion), and community functioning (e.g., community safety, community cohesion) were selected for examination in this study [10, 11]. Because of the military nature of this sample, and the relative lack of information about military-related risk factors, a number of factors that could plausibly be related to partner abuse but that had not previously been examined in the literature, were also included (e.g., support from leaders, spouse’s ability to cope with deployment) as hypothesized predictors.
We hypothesized several variables at each ecological level would be significantly related to partner aggression perpetration. Because ecological analyses have implications helping guide both for integrated theory development as well as prevention and outreach activities, which can occur at any or all of the ecological levels, after testing the specifically hypothesized bivariate relations, we then developed regression models of uniquely additive risk factors both within and across ecological levels. This allows theoreticians and program developers alike to understand, for example, all (a) all the significant community risk factors for partner aggression, (b) the unique community risk factors for partner aggression, and (c) community risk factors that account for unique variance in partner aggression when considered in the context of risk factors from all ecological levels.
Parallel analyses were conducted separately for men and women. Women’s perpetration has been less well studied; however, the literature that does exist on partner aggression suggests the factors that place women at risk for acting aggressively are not noticeably different than the factors that place men at risk for such acts [17, 20]. Thus, we did not hypothesize gender effects but did keep the genders separate to allow different patterns to be detectable.
Method
Participants
Active duty (AD) members (N = 128,950) of the United States Air Force (AF) were invited to complete the 2006 Community Assessment (CA), a biennial, anonymous survey conducted at 82 Air Force sites worldwide. Sampling occurred via sophisticated stratification procedures; technical details of the sample selection process are available from the RAND Corporation [21]. The sample was representative at the base level and, with appropriate weighting, at the AF level. Unweighted demographic information is presented in Table 1.
Procedure
The 2006 CA was administered online by Caliber Associates; it launched on 27 April and closed on 23 June and assessed individual, family, organizational, and community functioning as well as secretive behavioral health problems (including partner aggression). Each selected participant was e-mailed an invitation containing the Web link to the survey and an access code. From launch date to survey close, weekly e-mails were sent reminding the selected AD members to participate; each base also conducted its own community-wide campaign encouraging participation from anyone who had been invited to do so. The survey took approximately 45 min–1 h to complete and could be completed across multiple sessions.
Measures
The CA included brief scales measuring relevant individual, family, organization, and community constructs. At the individual level, the CA assessed depressive symptoms (seven items), financial stress (five items), personal coping (nine items), physical health (six items) alcohol problems (ten items), spirituality/religiosity (five items), and years in the military [22–29]. Family-level constructs included length of marriage, parental status, family income (estimated from salary statistics available from the US Air Force), relationship satisfaction (four items), career support from significant other (three items), partner physical aggression (frequency of perpetration and victimization; 15 items each), family coping (three items), ability of spouse to cope with deployment (two items), parent–child relationship satisfaction (three items, and parent–child physical aggression (variety of acts endorsed; 18 items per child) [30–33]. Work-related variables included total hours worked (per week), length of commute, weeks deployed (in past year), satisfaction with the air force (as a way of life; five items), work group cohesion/preparedness (six items), work relationships (three items), and support from leadership (17 items). Finally, at the community level, the survey assessed community safety (six items), community stressors (13 items), community unity (21 items), community support for youth (three items), support from formal agencies (six items), support from neighbors (seven items), and social support (five items) [34–37].
Although based on existing literature, the CA was largely designed by a working group with the help of additional outside consultants. Thus, many of the scales were modified in some manner. The composition and psychometrics of the CA scales have been reported in detail elsewhere [9]; factor analyses confirmed the structure of each scale, and all internal consistency coefficients were acceptable (mean = .83, range = .63–.95).
Data Management
Missing Data
The data from all individuals who logged into the survey (N = 54,543) were examined. A few individuals (n = 1,369) ended their participation without answering even the first few questions asking for basic demographics; these were considered non-respondents and were removed from the dataset. Of those remaining, over 75% (n = 42,215) completed the survey, while the rest (n = 10,959) ended their participation at some point in between. Of these, all who had entered “Other” as their location rather than selecting a base and could therefore not be weighted (n = 305) were removed from the dataset. Also, because it was likely that both members of at least a few dual-AD couples would have participated (creating possible problems of non-independence), we used certain data points (gender, length of marriage, number and ages of children in the home) to “match up” these couples (n = 55 couples). One member of each such couple was then randomly chosen and removed from the dataset, as were 34 individuals whose responses were suspect (e.g., who endorsed every possible act of parent–child physical assault).
Although the amount of missingness was lower than expected given the nature of the survey, it could not be ignored [38]. After scoring, significantly non-normal variables were transformed appropriately, and five multiply imputed datasets were then created using SAS-callable IVEware [39].
Weighting
We developed post-stratification weights to adjust for Air Force base populations, which varied from the Air Force population because (a) smaller bases were oversampled; and (b) non-response differed across bases. Given the multiple variables used in the sampling, we used raking to adjust the cells of all the variables considered in the sampling design. WesVar 4.2 was used to create the survey weights and rake on marital status, base, ethnicity, religion, gender, paygrade, and job type. Raking uses iterative proportional fitting to match marginal distributions of a survey sample to known population margins. In sample surveys, weights—especially for those in very rare categories, such as Hispanic female officers—can become very large. Extreme weights (four times greater than or less than the size of the mean weight) were trimmed. This is typically done so that extreme weights do not overly influence results and do not result in large sampling variances [40].
Results
The sample was randomly split into a development sample and validation sample to permit cross-validation analyses. This resulted in a development sample of 17,247 men and 4,016 women and a validation sample of 17,466 men and 4,015 women. There were no statistically significant differences between the development and validation samples on any relevant study variables (P > .01). Approximately 5% of men and 9% of the women in the sample reported partner aggression. Rather than using an excessively large comparison group (~91 to 95%), we randomly selected a comparison group from the participants that did not endorse partner aggression [41]. This resulted in 5,397 men and 1,866 women in the development and 5,640 men and 1,902 women in the validation samples. The randomly selected comparison groups did not significantly differ from the rest of the sample on any of the study variables (P > .01).
Bivariate Results
Correlation analyses of bivariate relationships between partner aggression and each of the predictor variables were conducted first. Descriptive statistics and bivariate correlations are in Table 2. As hypothesized, nearly all of the study variables were significantly related to partner aggression for men. The associations between partner aggression and the more proximal levels (individual and family) tended to be larger in magnitude than the more distal levels (community and organization). For women, there were a smaller number of significant predictors of partner aggression, but those with the largest correlation coefficients also tended to individual and family level predictors. Only two organization variables and one community variable were significant predictors of women’s partner aggression.
Backward Stepwise Regressions: Relationship Sample
Backward stepwise linear regression analyses predicting partner aggression were conducted separately for each ecological level on the development subsamples. Analyses were first conducted for those individuals in relationships excluding variables only available for parents or married individuals (family coping, child physical aggression, parent child relationships, marital length, and spouse deployment support). The specific predictor variables entered for each ecological level are listed in Table 2. Variables were entered into a backward stepwise regression and those that made a unique contribution to prediction of partner aggression were retained in the final models. The final models of the variables that made a unique contribution to predicting partner aggression within their ecological level in the development subsamples are presented in Table 3 (men) and Table 4 (women).
Next, all the significant unique predictors from each ecological level were entered into a backward stepwise regression equations to test which made a unique contribution overall. The unique overall predictors are also presented in Tables 3 and 4.
After the backward stepwise regressions were performed on the development subsamples, the final models were tested with the validation subsamples. These results are also presented in Tables 3 and 4. As can be seen from the tables, the final models were largely replicated in the validation subsamples for men and women.
Backward Stepwise Regressions: Married and Parent Samples
Additional family level analyses were conducted with the subset of participants that were married, married with children, or in relationships with children to test whether any of the specific marital or parental variables made unique contributions. For women with children, none of the variables specific to parenthood made unique contributions. For married women, spouse deployment support, marital length, family coping, and family income all made unique contributions to predicting women’s aggression in addition to relationship satisfaction and parental status. Although marital length, family coping, and family income all made unique contributions at the family level for married women, they did not make unique contributions in the context of the other ecological level predictors. Spouse deployment support replaced support from significant other as a unique predictor across ecological levels and was the only marriage-specific predictor retained in the final overall model for married women. The final model for married women was tested with the cross-validation subsample and all predictors remained significant (spouse deployment support, relationship satisfaction, alcohol problems, military years, and financial stress).
For men, both marital and parental variables made unique contributions in the context of other family level variables. The only unique parental variable retained was child physical aggression and the only unique marital variables retained were marital length and spouse deployment support. This resulted in the following models for (a) men with children: family income, relationship satisfaction, support from significant other, and child physical aggression; (b) married men with children: relationship satisfaction, spouse deployment support, marital length, and child physical aggression; and (c) married men: relationship satisfaction, spouse deployment support, and parental status. It should be noted that when marital length was added to the models, family income was no longer retained. It is likely that they are accounting for similar variance related to family stability.
Backward stepwise regressions were conducted to determine unique overall predictors across levels for men with children, married men with children, and married men. The final overall models in the development subsample for men were as follows: (a) men with children: relationship satisfaction, alcohol problems, financial stress, support from neighbors, and child physical aggression, (b) married men with children: relationship satisfaction, alcohol problems, financial stress, support from neighbors, marital length, and spouse deployment support,(c) married men: relationship satisfaction, alcohol problems, financial stress, support from neighbors, marital length, spouse deployment support, parental status, community unity, and support from formal agencies. These final models were tested in the validation subsamples and all predictors were retained except alcohol problems and spouse deployment support in the married with children sample and community unity in the married men sample. In comparison to the results presented in Table 3, the inclusion of marital length in the married samples resulted in military years no longer remaining a significant unique predictor. Similar to the findings for women, this is likely to due to marital length accounting for similar variance as military years in predicting partner aggression. A factor analysis of study variables confirmed that marital length, military years, and family income indeed formed one factor that can be interpreted as a family stability construct.
Generalizability Analyses
The models that cross-validated in the validation samples (see Tables 3, 4) were tested to see whether the results generalized across geographical regions (Northeast United States, Midwest United States, South United States, West United States, Asia, and Europe), different city sizes (urban area of 1,000,000 or more, urban area of 250,000–1,000,000, urban area of less than 250,000, rural area of 20,000 or more, rural area of 20,000 or less), and for different marital statuses (married versus unmarried). The development and validation samples were combined for these analyses resulting in 11,037 men and 3,768 women for generalizability analyses of region and marital status. The generalizability analyses for city size combined across development and validation subsamples were with 9,200 men and 1,032 women in cities in the United States. Mplus 5.1 statistical software was used for the analyses [42]. The final logistic regression models were tested constraining the regression coefficients to be equal across generalizability groups (e.g., married versus unmarried). The models were run a second time with the regression coefficients free to vary across groups. The Chi-square difference test was used to calculate whether the Chi-square from the constrained analyses differed significantly from the Chi-square of the unconstrained analyses. The unconstrained models were not a significantly better fit than the constrained models (the Chi-square difference test was not significant, P > .05) for any of the models for women. There were also no significant differences across region and city size for men in the constrained versus unconstrained models. However, the unconstrained model was a significantly better fit than the constrained model for the unique family level predictors when examining marital status (mean Δχ2 (3) = 11.51, Rubin’s F = 3.96, P < .01). The family level models for marital status were compared in order to explain the significant Chi-square differences found. As noted in Table 3, the family level model consisted of parental status, family income, and relationship satisfaction as significant unique predictors. The difference in models for married and unmarried men was due to parental status only predicting abuse for married men. Among the unmarried dating men, being a parent (coefficient = −.03, z score = −.17, P > .05) was not a significant unique predictor of less partner abuse whereas it was for the married men (coefficient = −.49, z score = −.10.74, P < .05). Family income and relationship satisfaction were both retained as unique predictors of abuse for married and unmarried men.
Discussion
This study is the first to examine risk factors from four ecological levels as they relate to men’s and women’s physical partner aggression in a large, military sample. Generally, nearly all hypothesized risk factors from each of the four levels were significantly related to men’s aggression. A subset of these were related to women’s aggression. With the exception of those at the community level, predictors that were significant for women were those with the strongest effect sizes for men. Among the community level predictors, only community safety was related to women’s aggression; community safety’s effect size was in the middle of effects for men’s aggression.
Individual and family predictors for both men’s and women’s aggression are generally consistent with the existing literature [10, 11] and emphasize self-regulatory (e.g., personal coping, depressive symptoms) and relationship functioning (e.g., relationship satisfaction, family coping) variables.
Of note, the majority of the workplace factors for men, and nearly half for women, were significantly related to aggression. This may suggest that program and policy developers can gain additional leverage in targeting partner physical aggression indirectly by targeting workplace-based factors. Generally, these factors may be more palatable to target and may be attractive to target for other reasons (e.g., possible increased productivity, retention), perhaps making it easier to garner institutional support than for work-based programs directly aimed at partner physical aggression. Furthermore, several community level predictors accounted for unique variance in men’s physical partner aggression even in the context of variables from all other levels, which may suggest that targeting community factors might help reduce men’s partner aggression. Examples of prevention frameworks that use community-level intervention aimed at target problems indirectly through targeting risk factors include Communities that Care and NORTH STAR [43, 44].
Because of the very large size of this data set, we were able to examine the generalizability of the results across region, urbanicity, and marital status. With one exception, all results generalized across each of these factors. The one exception was for men’s models with respect for marital status.
Some important limitations of this study must be noted. First, all data are self-report; it is likely that perpetration of physical partner aggression is underreported (as found in other surveys; e.g., [45, 46]. Second, although a wide selection of putative risk factors were included in the survey, no instrument can comprehensively assess all potential risk factors, and some were not included in this survey. Finally, although the sample is large and generalizable to AF active duty members generally, results cannot be generalized to specific subsamples (e.g., female, Hispanic officers) or to groups generally outside of the AF population (e.g., couples over 50 years of age, or where neither partner is employed).
In sum, this study elucidates how individual, family, workplace, and community factors relate to men’s and women’s physical partner aggression among members of the AF. These results can help inform the development of integrated etiological theories [17] and innovative outreach and prevention initiatives.
References
Schafer, J., Caetano, R., & Clark, C. L. (1998). Rates of intimate partner violence in the United States. American Journal of Public Health, 88, 1702–1704.
Campbell, J. C., & Lewandowski, L. A. (1997). Mental and physical effects of intimate partner violence on women and children. Psychiatric Clinics of North America, 20, 353–374.
Cascardi, M., Langhinrichsen, J., & Vivian, D. (1992). Marital aggression: Impact, injury, and health correlates for husbands and wives. Archives of Internal Medicine, 152, 1178–1184.
Cascardi, M., O’Leary, K. D., & Schlee, K. A. (1999). Co-occurrence and correlates of post traumatic stress disorder and major depression in physically abused women. Journal of Family Psychology, 14, 227–249.
Coker, A. L., Davis, K. E., Arias, I., Desai, S., Sanderson, M., Brandt, H. S., et al. (2002). Physical and mental health effects of intimate partner violence for men and women. American Journal of Preventive Medicine, 24, 260–268.
Stets, J. E., & Straus, M. A. (1990). Gender differences in reporting marital violence and its medical and psychological consequences. In M. A. Straus & R. J. Gelles (Eds.), Physical violence in American families (pp. 151–165). New Brunswick, NJ: Transaction Publishers.
Lawrence, E., & Bradbury, T. N. (2001). Physical aggression as a predictor of marital dysfunction: A longitudinal analysis. Journal of Family Psychology, 15, 135–154.
Heyman, R. E., & Neidig, A. (1999). Comparison of spousal aggression prevalence rates in U.S. army and civilian representative samples. Journal of Consulting and Clinical Psychology, 2, 239–242.
Snarr, J. D., Heyman, R. E., & Slep, A. M. S. (2006). The 2006 Air Force behavioral health problem review: Assessing the prevalence of force health challenges.
Schumacher, J. A., Feldbau-Kohn, S., Slep, A. M. S., & Heyman, R. E. (2001). Risk factors for male-to-female partner physical abuse. Aggression and Violent Behavior, 6, 281–352.
Stith, S. M., Smith, D. B., & Penn, C. E. (2004). Intimate partner physical abuse perpetration and victimization risk factors: A meta-analytic review. Aggression and Violent Behavior, 10, 65–98.
Dutton, D. G. (1985). An ecologically nested theory of male violence toward intimates. International Journal of Women’s Studies, 8, 404–413.
Bronfenbrenner, U. (1979). The ecology of human development: Experiments by design and nature. Cambridge, MA: Harvard University Press.
Capaldi, D. M., Shortt, J. W., & Kim, H. K. (2005). A life span developmental system perspective on aggression toward a partner. In W. M. Pinsoff & J. L. Lebow (Eds.), Family psychology: The art of the science (pp. 141–167). New York: Oxford University Press.
Leonard, K. E., & Senchak, M. (1996). Prospective prediction of husband marital aggression within newlywed couples. Journal of Abnormal Psychology, 105, 369–380.
O’Leary, K. D., Malone, J. E., & Tyree, A. (1994). Physical aggression in early marriage: Pre-relationship and relationship effects. Journal of Consulting and Clinical Psychology, 62, 594–602.
O’Leary, K. D., Slep, A. M. S., & O’Leary, S. G. (2007). Multivariate models of men’s and women’s partner aggression. Journal of Consulting and Clinical Psychology, 75, 752–764.
Riggs, D. S., & O’Leary, K. D. (1996). Aggression between heterosexual dating partners: An examination of a causal model of courtship aggression. Journal of Interpersonal Violence, 11, 519–540.
White, J. W., Merrill, L. L., & Koss, M. P. (2001). Prediction of premilitary courtship violence in a navy recruit sample. Journal of Interpersonal Violence, 16, 910–927.
Stith, S. M., Green, N. M., Smith, D. B., & Ward, D. B. (2008). Marital satisfaction and marital discord as risk markers for intimate partner violence: A meta-analytic review. Journal of Family Violence, 23, 149–160.
Bigelow, J. H. (2006). Using linear programming to design samples for a complex survey. Unpublished manuscript, DRR-3992-AF, RAND Project AIR FORCE, Arlington, VA.
Mirowsky, J., & Ross, C. E. (1992). Age and depression. Journal of Health and Social Behavior, 33, 187–205.
Radloff, L. S. (1977). The CES-D Scale: A self-report depression scale for research in the general population. Applied Psychological Measurement, 1, 385–401.
Krause, N., & Baker, E. (1992). Financial strain, economic values, and somatic symptoms in later life. Psychology and Aging, 7, 4–14.
Vinokur, A. D., Price, R. H., & Caplan, R. D. (1996). Hard times and hurtful partners: How financial strain affects depression and relationship satisfaction of unemployed persons and their spouses. Journal of Personality and Social Psychology, 71, 166–179.
Conger, R. D., Conger, K. J., Elder, G. H., Lorenz, F. O., Simons, R. L., & Whitbeck, L. B. (1993). Family economic stress and adjustment of early adolescent girls. Developmental Psychology, 29, 206–219.
Scholz, U., Gutierrez, D. B., Sud, S., & Schwarzer, R. (2003). Is general self-efficacy a universal construct? European Journal of Psychological Assessment, 18, 242–251.
Ware, J. E., Jr., Kosinski, M., Dewey, J. E., & Gandek, B. (2001). How to score and interpret single-item health status measures: A manual for users of the SF-8™ health survey. Lincoln, RI: QualityMetric Incorporated.
Rumpf, H., Hampke, U., Meyer, C., & John, U. (2002). Screening for alcohol use disorders and at-risk drinking in the general population: Psychometric performance of three questionnaires. Alcohol and Alcoholism, 37, 261–268.
Norton, R. (1983). Measuring marital quality: A critical look at the dependent variable. Journal of Marriage and the Family, 45, 141–151.
Straus, M. A., Hamby, S. L., Boney-McCoy, S., & Sugarman, D. B. (1996). The revised Conflict Tactics Scales (CTS2): Development and preliminary psychometric data. Journal of Family Issues, 17, 283–316.
Simons, R. L., Beaman, J., Conger, R. D., & Chao, W. (1993). Childhood experience, conceptions of parenting, and attitudes of spouse as determinants of parental behavior.
Straus, M. A., Hamby, S. L., Finkelhor, D., Moore, D. W., & Runyan, D. (1998). Identification of child maltreatment with the parent–child conflict tactics scales: Development and psychometric data for a national sample of American parents. Child Abuse and Neglect, 22, 249–270.
U. S. Army Community Support Center. (1989). 1989 Army Soldier and Family Survey: Soldier Survey (SCN: ATNC-AO-89-10A). Cary, NC: Research Triangle Institute, Caliber Associates, and Human Resources Research Organization.
Princeton Survey Research Associates. (1999). The community indicators survey—national: Topline results. Retrieved April 10, 2008 from http://www.knightfoundation.org/research_publications/community_indicators/nat/topline.pdf.
The Social Capital Benchmark Survey. (2000). Retrieved April 8, 2008, from http://www.cfsv.org/communitysurvey/docs/survey_instrument.pdf.
Research Triangle Institute. (1990). AFRP analysis plan (Volume II: Appendices). Research Triangle Park, NC: Author.
Allison, P. D. (2001). Missing data (Sage University papers series on quantitative applications in the social sciences no. 07-136). Thousand Oaks, CA: Sage Publications.
Raghunathan, T. E., Solenberger, P., & Van Hoewyk, J. (2002). IVEware: Imputation and variance estimation software installation instructions and user guide. Ann Arbor: The University of Michigan.
Potter, F. (1988). Survey of procedures to control extreme sampling weights. In Proceedings of the survey research methods section of the American Statistical Association, pp. 453–458.
Graubard, B. I., & Korn, E. L. (1996). Survey inference for subpopulations. American Journal of Epidemiology, 144(1), 102–106.
Muthén, L. K., & Muthén, B. O. (2007). Mplus Version 5.1. Los Angeles, CA: Muthén & Muthén.
Fagan, A. A., Hawkins, J. D., & Catalano, R. F. (2008). Using community epidemiologic data to improve social settings: The communities that care prevention system. In M. Shinn & H. Yoshikawa (Eds.), Toward positive youth development: Transforming schools, community programs (pp. 292–312). New York, NY, USA: Oxford University Press.
Slep, A. M. S., & Heyman, R. E. (2008). Public health approaches to family maltreatment prevention: Resetting family psychology’s sights from the home to the community. Journal of Family Psychology, 22(3), 518–528.
Heyman, R. E., & Schlee, K. A. (1997). Toward a better estimate of the prevalence of partner abuse: Adjusting rates based on the sensitivity of the Conflict Tactics Scale. Journal of Family Psychology, 11, 332–338.
Schafer, J., Caetano, R., & Clark, C. L. (2002). Agreement about violence in U.S. couples. Journal of Interpersonal Violence, 17, 457–470.
Acknowledgments
This research was supported by U.S. Department of Defense grant W81XWH0710328. The opinions expressed in this article are solely those of the authors and do not necessarily represent the official views of the U.S. Government, the Department of Defense, or the Department of the Air Force. We wish to acknowledge Maj. David Linkh his assistance and support, which made this project viable. We would also like the thank the personnel at the local Air Force site who were responsible for promoting the survey, to Caliber Associates (especially Dr. Chris Spera) for administering the web survey and to the active duty members and their spouses who took time to complete it.
Author information
Authors and Affiliations
Corresponding author
Rights and permissions
About this article
Cite this article
Slep, A.M.S., Foran, H.M., Heyman, R.E. et al. Unique Risk and Protective Factors for Partner Aggression in a Large Scale Air Force Survey. J Community Health 35, 375–383 (2010). https://doi.org/10.1007/s10900-010-9264-3
Published:
Issue Date:
DOI: https://doi.org/10.1007/s10900-010-9264-3