Abstract
A number of studies have demonstrated that individuals with autism spectrum disorders (ASDs) are faster or more successful than typically developing control participants at various visual-attentional tasks (for reviews, see Dakin and Frith in Neuron 48:497–507, 2005; Simmons et al. in Vis Res 49:2705–2739, 2009). This “ASD advantage” was first identified in the domain of visual search by Plaisted et al. (J Child Psychol Psychiatry 39:777–783, 1998). Here we survey the findings of visual search studies from the past 15 years that contrasted the performance of individuals with and without ASD. Although there are some minor caveats, the overall consensus is that—across development and a broad range of symptom severity—individuals with ASD reliably outperform controls on visual search. The etiology of the ASD advantage has not been formally specified, but has been commonly attributed to ‘enhanced perceptual discrimination’, a superior ability to visually discriminate between targets and distractors in such tasks (e.g. O’Riordan in Cognition 77:81–96, 2000). As well, there is considerable evidence for impairments of the attentional network in ASD (for a review, see Keehn et al. in J Child Psychol Psychiatry 37:164–183, 2013). We discuss some recent results from our laboratory that support an attentional, rather than perceptual explanation for the ASD advantage in visual search. We speculate that this new conceptualization may offer a better understanding of some of the behavioral symptoms associated with ASD, such as over-focusing and restricted interests.
Similar content being viewed by others
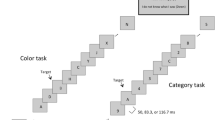
Avoid common mistakes on your manuscript.
Introduction
Alongside the well-known social and communicative deficits of autism spectrum disorder (ASD) stands an ‘ASD advantage’ characterized by superior performance on many perceptual and attentional tasks, including the embedded figures task (Shah and Frith 1983) and the block design task (BDT) (Shah and Frith 1993; for reviews, see Dakin and Frith 2005; Simmons et al. 2009). While most of the research and the public’s awareness have been focused on the impairments in ASD, some scientists (e.g. Mottron 2011), non-profit companies and many individuals living with autism are actively fighting this one-sided view. This perspective has been featured in the popular press, most recently, for example, in The New York Times (Cook: The Autism advantage, Nov. 29, 2012).
Our goal in this paper is twofold. First, we provide a targeted ‘mini-review’ of the empirical findings from a paradigm where the ASD advantage has been studied most extensively: visual search. Second, we present the two most influential theories that have been proposed to explain the ASD advantage in these search tasks. One of them asserts that the cause is primarily perceptual: that bottom-up, low-level processes are functioning atypically in ASD. The other explanation suggests that it is the attention system that is atypical in ASD; a tendency to ‘over-focus’, while perhaps disadvantageous in some contexts, is beneficial to visual search. While not mutually exclusive, these explanations can be hard to tease apart, as both predict reductions in reaction times (RTs) and increases in accuracy for finding hidden targets in visual search tasks. We will then introduce a recent result from our laboratory, using pupillometry as a measure of attentional engagement that supports an attention-based explanation. Finally, we show how differences in attentional processing can be related to clinical observations. Throughout this review, wherever possible, we will highlight developmental trends.
Visual Search
There are several psychophysical paradigms that have been developed to study search abilities. The most ubiquitous is Anne Treisman’s (Treisman and Gelade 1980). In this paradigm, two conditions have been contrasted: ‘single feature’, ‘pop-out’ (Julesz 1981) search, and ‘feature conjunction’ search. In a single feature search task an array of items is shown in which a ‘target’ item (the item that is to be searched for) has a unique feature that distinguishes it from a homogeneous set of distractors (e.g. a red disk target in a field of blue disk distractors). The classic signature of single feature search is that the amount of time it takes individuals to find the target is not significantly affected by the set size of the distractors (a red target in a field of 20 blue distractors is found as quickly as in a field of five); the target ‘pops out’ automatically and effortlessly. However, to find an item that is unique among the distractors by virtue of having a conjunction of two different feature dimensions (e.g. a red disk among a distractor set containing both blue disks and red triangles) typically requires an effortful search of the items in the display in a more or less serial fashion; the target no longer pops out. Therefore, search times in feature conjunction search tasks typically vary linearly with the number of distractors. While research suggests that the attentional mechanisms involved in single versus feature conjunction search are not categorically different (see e.g. Duncan and Humphreys 1989; Wolfe 1998), and that target-distractor similarity and the perceptual characteristics of the target itself together determine the efficiency of search (Wolfe and Horowitz 2004), the classic paradigm using shape and color as features is still often used as a way to systematically contrast easy and difficult attentional demands. In the current theory of visual search the distinction is simply between efficient and inefficient search (Wolfe and Horowitz 2004). For our purposes, the distinctions between easy vs. difficult and efficient vs. inefficient search map onto the single feature/feature conjunction distinction.
Visual Search in ASD: The Findings
Kate Plaisted and her colleagues were the first to demonstrate the ASD advantage in visual search in 1998 (Plaisted et al. 1998). They found that 7–10-year-old children with autism demonstrated faster RTs in feature-conjunction tasks than verbal-ability matched typically developing (TD) children. The group extended these findings to nonverbal IQ-matched and age-matched controls (O’Riordan 2000; O’Riordan et al. 2001) and adults (O’Riordan 2004). Since this seminal report and its follow-ups, a number of studies have contrasted the visual search skills of participants with and without ASD. We have summarized the participant characteristics, basic elements of the tasks employed, and main results of 22 experimental studies from the past 15 years (see Table 1).
Overwhelmingly, these studies demonstrate that compared to control participants, individuals with ASD are both faster and more accurate (though accuracy is typically just measured to ensure that shorter RTs do not just reflect a speed-accuracy tradeoff) at identifying a target hidden amongst a number of distractors (‘set size’). This finding has been consistent across ages (from 2.5-year-old children to adults) and the spectrum of symptom severity. In children, the ASD advantage is consistently present even in age-matched control groups. Even the presence of sub-clinical autism-like traits in normally developed adults (as measured by the Autism Quotient) correlates with better visual search performance. In terms of differences across task design, the ASD advantage is present at different difficulty levels (though more reliably so in ‘conjunction’ search and more difficult, inefficient feature searches) and for various perceptual features (color, shape, and orientation).
There are only a few exceptions to these findings. In Ashwin et al. (2006) task, the target and distractors in the search task consisted of threatening, happy, and neutral faces. In a series of four experiments, adults with ASD were slower than controls to find a discrepant face in an array of faces. However, these findings are in line with previous research demonstrating deficits related to facial and emotional recognition in individuals with ASD (see Harms et al. 2010, for a review).
In Baldassi et al. (2009), the search performance of the ASD group was not significantly different from TD controls. The paradigm they used though had some salient differences from the search task employed in the other studies reviewed here. Instead of RT or accuracy of target detection, they measured orientation discrimination thresholds in briefly presented (200 ms) displays presented centrally or peripherally. The target was either presented alone (set size 1) or with flankers (set size 6 or 9). Relative to their detection thresholds, both groups were equally slowed by the addition of flankers; no apparent ASD advantage for visual search. Intriguingly, in a different manifestation of the ASD advantage, when the set of items was presented in the periphery, children with ASD showed no crowding effect (a significant impairment in orientation discrimination of the target when it is surrounded by flankers, an effect that was present in TD children). These findings reveal the importance of certain task demands, such as employing brief (such as 200 ms) onset–offset stimuli.
Caron et al. (2006) tested typical adults and adults with high-functioning autism. Within each group, they compared a subgroup that was selected based on their exceptional score on the BDT. They found that those who did exceptionally well on the BDT were faster at conjunction search, independent of diagnosis; at face value, this would indicate a lack of an ASD advantage. However, BDT scores highly correlated with visual search performance in this study, so matching individuals by BDT score is a proxy for matching them for search; no differences would be expected. The ASD advantage is found in general because the base rate of individuals that score high on BDT and do well on search is (apparently, our survey supports) higher in ASD than TD populations.
Finally, Iarocci et al. (2006) found no significant difference between the performance of children with and without ASD in a local/global visual search task. Here, since the ASD group (mean age 8 years) was well above average in non-verbal IQ, the matching TD control group was almost a 1.5 years older. Similarly, Riby et al. (2012) found no group differences in a task where older children with and without ASD had to look for a specific object in an array of random objects. Since in this task the salience of the distractors was much more heterogeneous, it is possible that children with ASD got more distracted by particular objects in the displays.
In sum, even though some studies reviewed above have pointed out certain limitations, the ASD advantage in visual search stands on firm empirical ground. That said, it is clear that further investigation is required to pinpoint the task and stimulus parameters that have greatest influence over the expression of the ASD advantage. We will now turn to the discussion of the two prominent theories on the mechanisms underlying the ASD advantage in visual search.
Two Theories of the ASD Advantage in Visual Search
Perceptual Enhancement
The first theory of the ASD advantage in visual search was that individuals with ASD have enhanced perceptual discrimination (Plaisted et al., 1998; O’Riordan 2000; O’Riordan et al. 2001). Similarly, the Enhanced Perceptual Functioning model proposed by Mottron and his colleagues (Mottron and Burack, 2001; Mottron et al. 2006, for a more recent extension of the model, see Mottron et al. 2013) is based on the idea that both low-level (discrimination) and mid-level (pattern detection) perceptual processes are enhanced in ASD (this model has not been specifically applied to visual search performance (apart from Experiment 4 of Caron et al. 2006), but it is relevant to this discussion). Models based on perceptual enhancement have considerable intuitive appeal and face validity, after all, any manipulation—physical or perceptual—that increases target discriminability (decreasing target-distractor similarity) can increase performance; target–distractor discriminability is a principal rate-determining factor in visual search (Duncan and Humphreys 1989; Wolfe 1994).
While there is ample evidence to support that individuals with ASD show higher sensitivity in certain perceptual domains (for a summary, see Mottron et al. 2006), there is no reliable evidence for higher sensitivity to spatial or color contrast in children or adults with ASD (Koh et al. 2010; for a summary, see Simmons et al. 2009), nor evidence for higher visual acuity in general (Bölte et al. 2012; Falkmer et al. 2011). Regarding color perception in ASD, a recent study found reduced sensitivity for color in children with high-functioning autism compared to controls matched on age and nonverbal ability, and no significant differences were found in luminance sensitivity (Franklin et al. 2010).
Examining the studies in Table 1, it is clear that most invoke some form of perceptual enhancement to explain the ASD advantage. Most though do not claim to have direct evidence for perceptual enhancement per se, but instead choose it after a ‘process of elimination’ of competing explanations, or by analogy to similar studies that have also invoked it (as we ourselves did in Kaldy et al. 2011). Of the papers reviewed here, only two measured the discrimination thresholds for their visual search stimuli (in both cases, the parameters of Gabor patches), with one finding no correlation between thresholds and search performance (Brock et al. 2011), and another finding significantly higher thresholds in their Autism group (Baldassi et al. 2009). In particular, in the Brock et al. (2011) study, the authors tested whether visual search performance for a target defined by the conjunction of spatial frequency and orientation correlates with lower visual discrimination thresholds for the same features. Their sample was undergraduate students who completed the autism spectrum quotient (AQ) questionnaire (Baron-Cohen et al. 2001) before the psychophysical tests. Brock et al. (2011) found that adults with higher AQ were faster in visual search, but that visual search performance did not correlate with discrimination thresholds. To date, this has been the most direct test of the enhanced discrimination explanation behind visual search performance, which, if it can be replicated with individuals with and without ASD, can pose a problem for the enhanced perceptual discrimination model as an explanation for the ASD advantage in visual search.
Atypical Attention
Another theory attributes the superior skills in ASD to an atypically functioning attentional system. Under this view, a tendency to over-focus, and a resistance to disengagement is responsible for superior visual search.Footnote 1 In a recent review, Keehn et al. (2013) summarized the extant research on attentional functioning in ASD following Posner’s model that distinguishes the alerting, orienting and executive control networks of attention (Posner and Petersen 1990; Petersen and Posner 2012). The alerting network has two dissociable functions: tonic alertness provides a baseline sensitivity level to incoming information (equivalent to vigilance) and phasic alertness is the more transient state that the organism is in when it is actively engaged in a task. The orienting network, on the other hand, selects information from the sensory input by engaging, disengaging and re-engaging attention (whether covertly or overtly, endogenously or exogenously). Finally, the executive control network consists of set shifting (or task switching), working memory and inhibition.
Keehn et al. (2013) finds evidence for atypical function in the all three systems in ASD, but puts problems with attentional disengagement at the origin of the developmental cascade that leads to dysfunctional arousal regulation by the alerting system, which in turn engenders over-focused attention, which explains enhanced visual search abilities. With respect to the other systems, impairments of the executive control network appear later in development so are viewed as secondary to other symptoms of the disorder, and while there are ASD-related attentional disengagement problems in the orienting system as measured by the classic ‘gap-overlap’ task (Saslow 1967), it does not manifest itself in visual search paradigms. Landry and Bryson (2004) found that children with ASD were slower in the ‘overlap’ condition (in this condition a simple shape is presented centrally briefly, then another stimulus appears in one of two lateral positions. The dependent measure is the saccadic latency to the second stimulus. This is contrasted with the ‘gap’ condition, where the first stimulus disappears before the appearance of the second one) than mental age-matched controls, while there were no differences in the gap condition. Impaired disengagement in the same task was found in older children with ASD (Van der Geest et al. 2001) and in adults with autism (Kawakubo et al. 2007) and importantly, in infants at risk for developing autism (Zwaigenbaum et al. 2005, Elsabbagh et al. 2009). For example, Zwaigenbaum et al. (2005) found that slower disengagement of gaze at 12 months significantly predicted ASD outcome at 2 years of age, and notably, all the infants’ whose disengagement score declined between 6 and 12 months were on the spectrum by 2 years of age. In spite of these findings, children with ASD often show shorter fixation times than controls in visual search (Joseph et al. 2009), and they have been shown to disengage from items at a faster pace or at the same pace (Kaldy et al. 2011) as TD children. Whatever role the orienting network may play in ASD, it does not seem to impede attentional disengagement—as manifested in gaze behavior—in visual search.
An account where atypical function of the alerting system leads to (advantageously) overly focused attention finds independent support from work on the Locus Coeruleus (LC; the brainstem area that regulates noradrenergic activity in cortex). Tonic activity of the LC modulates a diffuse, exploratory attentional state that facilitates task switching, while phasic activity modulates a focused attentional state that facilitates performance on fixed, well-defined tasks (Sara 2009). This link can be seen during direct manipulation of the LC. In monkeys, local microinfusion of clonidine to increase LC phasic activity increases performance on a visual task, while a suppressive agent (pilocarpine) has the reduces it (Aston-Jones and Cohen 2005). In humans, administration of modafinil to increase LC phasic activity increases task-related activity in cognitive control areas (shown by fMRI) and improves performance on a visual task (Minzenberg et al. 2008). Recent work has implicated the LC in ASD etiology, in humans (Mehler and Purpura 2009) and rat models (Darling et al. 2011). In fact, it has been speculated that the LC may be in a persistent hyperphasic state in ASD (Aston-Jones et al. 2007). Indeed, administration of venlafaxine to regulate LC activity effectively treats some of the attention-related symptoms of ASD (Hollander et al. 2000).
It is possible, then, that a dysregulated, hyperphasic LC predisposes individuals with ASD to over-focused attention, thereby increasing performance on tasks that benefit from focused attention and reduced distractibility (like visual search), while potentially decreasing performance on tasks that require shifts of attentional engagement. Fortunately, there is a way to gain insight into LC activity (and therefore attentional state) during a task: pupil dilation is a biomarker of LC activity (Aston-Jones and Cohen 2005). Gradual changes in pupil size reflect the tonic activity of the LC while dilations time-linked to task events reflect its phasic activity.
Pupillometry focusing on the phasic, task-related response has long been used as a sensitive, real-time physiological measure of cognitive effort (Kahneman and Beatty 1966; Beatty 1982). For instance, when attempting to read an incongruent word in the Stroop task, the pupil dilates (Laeng et al. 2011). Porter et al. (2007) found a clear link between search difficulty and mental effort as shown by larger pupil dilations in inefficient (feature-conjunction) vs. efficient (single feature) search. (Recently, Nassar et al. (2012) demonstrated a causal link between mental effort (as measured by pupil dilations) and performance in a challenging cognitive task). This relationship to cognitive effort even holds in infants. For instance, 8-month-olds will show greater pupil dilation when presented with impossible events (Jackson and Sirois 2009). Pupil responses in ASD and TD groups have been investigated by Anderson, Colombo and their colleagues (Anderson et al. 2006; Anderson and Colombo 2009; Anderson et al. 2012). They found that tonic pupil size is significantly elevated in children with ASD. However, pupillometry has not been used to investigate the phasic, task-related responses in ASD or to elucidate the mechanisms behind the ASD advantage.
In our recent study (Kaldy et al. 2011), we found that the ASD advantage in visual search is present as early as 2.5 years of age. We developed a version of the classic visual search paradigm that contrasted single-feature search and feature-conjunction search with varying set sizes (using shape and color as features), that did not require following verbal instructions, making it ideal for toddlers with weak receptive language skills. We tested 17 toddlers with ASD (who tended to be on the severe end of the symptom spectrum—in fact, 15 of them met the criteria for autism) and 17 age-matched TD children. Using a Tobii T120 eye-tracker, we measured success rate: the percent of trials were toddlers able to find the target within the 4 s presentation period. Our main finding was that (especially in the more attention-demanding feature-conjunction task) toddlers with ASD outperformed controls (by up to a factor of two).
We analyzed pupil dilation from that study (Blaser et al. 2012; Blaser et al. 2013) and found that the toddlers with ASD had exaggerated task-related pupil dilations; the LC was indeed more frequently phasic during search than in controls—evidence of a focused attentional state. Toddlers with ASD are predisposed, in a sense, to be ‘on task’, for visual search. Our analysis showed that TD toddlers can focus too, but they tend to do so less frequently during a test session. In short, children with ASD do not search better than TD controls, they are simply more likely to search with effort in any given trial.
This is a parsimonious explanation that accounts for the ASD advantage observed in our study. It could conceivably account for similar effects in other studies (or at least account for some of the variance). After all, if an ASD group exerts cognitive effort more consistently in a visual search task, this will result in better performance—a result that would otherwise be tempting to attribute to enhanced perception. It is important to note that this greater attentional engagement need not be evident in other measures. In our study we examined gaze behavior alongside task performance, yet the ASD and the TD groups did not differ in the number of fixations they made during a trial, the percent of trials rejected for never having fixated an item, the total time spent dwelling on items, or even the amount of time it took for them to get to the target on successful trials. Without pupillometry, we would have lacked the insight to reject perceptual enhancement as the default explanation (which we had invoked in Kaldy et al. 2011). This attentional explanation has received some further support from a recent ERP study (Milne et al. 2013). In a sample of neurotypical adults, Milne and colleagues found that a late ERP component (P3b) in an attentional task significantly predicted visual search efficiency (while earlier components, that reflect perceptual processing, did not).
We hypothesize that this predisposition to intense attentional focus in ASD comes at the cost of resistance to task disengagement. This link is examined in our final section.
Over-Focusing and Resistance to Task Disengagement: Clinical Observations
Both retrospective parental reports on children with ASD (Baranek 1999) and prospective experimental studies of infants at genetic risk for ASD (Zwaigenbaum et al. 2009, Elsabbagh et al. 2009) demonstrate that attentional dysfunction is one of the most reliable early signs of ASD among affected children. Early atypical attentional symptoms in the social domain include limited initiation and maintenance of eye contact (e.g., Gillberg et al. 1990), reduced social orienting, reduced and atypical joint attention, including deficits in both (a) following others’ gaze or pointing and (b) initiating shared attention with others (e.g., showing) as well as unusual and repetitive object-oriented play that often involves atypical visual inspection of or peering at objects (Ozonoff et al. 2008). In many of these atypical attentional behaviors the social and the attentional substrates are deeply intertwined and it is not surprising that social reward circuitry is also implicated in understanding these early atypicalities (Dawson et al. 2001).
Consistent with the heterogeneity of presentations, Liss et al. (2006) reported that 43 % of parents of children with ASD (N = 144) who were surveyed about their child’s sensory, motor and attention regulation reported over-focused attention. Consistent with our hypothesis of heightened phasic alerting activity, these authors linked these parental observations of over-focused attention with hyper-arousal. Importantly, attention regulation is critical not only for social engagement and communication, but also for regulation of emotion, as gaze shifting is one of the earliest emerging emotion regulation strategies (Mangelsdorf et al. 1995).
Early differences in attention-modulation may contribute to dynamic, developmental cascades, in which brain and behavioral functioning may be constrained through limited age-typical social interactions (Dawson 2008; Elsabbagh and Johnson 2007, 2010). Specifically, if infants are more likely to be in heightened states of phasic alerting, which may be experienced by interactive partners as over-focusing their attention on (non-social) objects to the exclusion of social information in their environments, intrinsic susceptibilities that confer an Autism advantage in visual search and an Autism disadvantage in facial processing and social communication may be intensified over time.
To the extent that very young infants with or at risk for ASD choose to pursue repetitive object play (Ozonoff et al. 2008) and fail to acquire joint attention, imitation, and other social communication skills that support typical language acquisition (Mundy et al. 1990; Rogers et al. 2003), atypical development may be exacerbated. Ozonoff and colleagues (Ozonoff et al. 2008) documented the presence of elevated repetitive and restricted object play as early as 12 months of age and three other studies characterized atypical use of objects in the toddler/preschool period among children with ASD (Bruckner and Yoder 2007; Mottron et al. 2007; Wetherby et al. 2004). Across these studies, the kinds of behaviors observed included reduced exploration as well as rotating, spinning, twirling, rolling, tapping, banging, rubbing, lining up and unusual visual inspection of or peering at objects. Of great interest for understanding the impact of the ASD advantage in clinical presentation, Ozonoff and colleagues (Ozonoff et al. 2008) reported that the most common repetitive behavior was atypical visual inspection, which was present in seven of the nine 12-month-old infants later diagnosed with ASD. Moreover, there was stability in repetitive behaviors between 12 and 36 months of age and atypical repetitive behaviors at 12 months were associated with lower social-communication scores on the ADOS and lower developmental outcomes on the Mullen Scales of Early Learning.
Consistent with relations between restricted and repetitive object play and broader development, restricted object use, which was defined as the number of toys that children engaged with in a differentiated manner when a set array of toys was presented, was associated with poorer joint attention, social engagement, and imitation skills (Bruckner and Yoder 2007). Moreover, Wetherby et al. (2004) reported that the rate of repetitive behaviors in the second year of life was significantly correlated with ASD outcome at 36 months of age. Sasson and colleagues (Sasson et al. 2008) used a passive viewing task of social and non-social stimuli, and within the second set they included a subset of stimuli chosen to be of high interest to children with ASD (e.g., trains, planes). In both school-age and preschool-age children (Sasson et al. 2011), the stimuli selected for high interest in children in ASD were, in fact, more visually engaging for this group. Importantly, in the older children, longer exploration of non-social stimuli was associated with parent report of increased repetitive behaviors.
Thus, these studies not only highlight the early emergence of restricted and repetitive behaviors in children affected with ASD, but also provide preliminary evidence in support of the potentially important role that visual attention is playing in developmental outcomes.
Dynamic, developmental theories hold promise for early intervention efforts as it may be possible to use deeper understanding of emerging attentional proclivities in children at risk or evidencing early signs to redirect them toward more typical learning experiences that can promote optimal social and communicative development. Thus, just as visual search performance has been associated with concurrent symptom severity (e.g. Joseph et al. 2009), it might inform decisions regarding intervention response and/or aspects of developmental course.
Notes
It is important to note that an opposite trend, namely increased distractibility has also been reported in ASD. Burack (1994) tested a small group of extremely low-functioning (N = 12, mean IQ = 49.5) adults with ASD and suggested that there is a general selective attention deficit in autism. Many authors since this early report have demonstrated (e.g. Remington et al. 2009) evidence for the contrary. A general selective attention deficit is also in contrast with the findings of the large body of research on ASD advantage in visual search reviewed in this paper.
References
Almeida, R. A., Dickinson, J., Maybery, M. T., Badcock, J. C., & Badcock, D. R. (2010). Visual search performance in the autism spectrum II: The radial frequency search task with additional segmentation cues. Neuropsychologia, 48, 4117–4124.
Almeida, R. A., Dickinson, J., Maybery, M. T., Badcock, J. C., & Badcock, D. R. (2013). Visual search targeting either local or global perceptual processes differs as a function of autistic-like traits in the typically developing population. Journal of Autism and Developmental Disorders, 43, 1272–1286.
Anderson, C. J., & Colombo, J. (2009). Larger tonic pupil size in young children with autism spectrum disorder. Developmental Psychobiology, 51, 207–211.
Anderson, C. J., Colombo, J., & Shaddy, D. (2006). Visual scanning and pupillary responses in young children with autism spectrum disorder. Journal of Clinical and Experimental Neuropsychology, 28, 1238–1256.
Anderson, C. J., Colombo, J., & Unruh, K. E. (2012). Pupil and salivary indicators of autonomic dysfunction in autism spectrum disorder. Developmental Psychobiology,. doi:10.1002/dev.21051.
Ashwin, C., Wheelwright, S., & Baron-Cohen, S. (2006). Finding a face in the crowd: Testing the anger superiority effect in Asperger Syndrome. Brain and Cognition, 61, 78–95.
Aston-Jones, G., & Cohen, J. D. (2005). An Integrative theory of locus coeruleus-norepinephrine function: Adaptive gain and optimal performance. Annual Review of Neuroscience, 28, 403–450.
Aston-Jones, G., Iba, M., Clayton, E., Rajkowski, J., & Cohen, J. (2007). The locus coeruleus and regulation of behavioral flexibility and attention: Clinical implications. In G. A. Ordway, M. A. Schwartz, & A. Frazer (Eds.), Brain norepinephrine: Neurobiology and therapeutics (pp. 196–235). New York: Cambridge University Press.
Baldassi, S., Pei, F., Megna, N., Recupero, G., Viespoli, M., Igliozzi, R., et al. (2009). Search superiority in autism within, but not outside the crowding regime. Vision Research, 49, 2151–2156.
Baranek, G. T. (1999). Autism during infancy: A retrospective video analysis of sensory-motor and social behaviors at 9–12 months of age. Journal of Autism and Developmental Disorders, 29, 213–224.
Baron-Cohen, S., Wheelwright, S., Skinner, R., Martin, J., & Clubley, E. (2001). The Autism-Spectrum Quotient (AQ): Evidence from Asperger syndrome/high-functioning autism, males and females, scientists and mathematicians. Journal of Autism and Developmental Disorders, 31, 5–17.
Bayliss, A. P., & Kritikos, A. (2011). Brief report: Perceptual load and the autism spectrum in typically developed individuals. Journal of Autism and Developmental Disorders, 41, 1573–1578.
Beatty, J. (1982). Task-evoked pupillary responses, processing load, and the structure of processing resources. Psychological Bulletin, 91, 276–292.
Blaser, E., Eglington, L., & Kaldy, Z. (2012). Toddlers with ASD are better at visual search without trying harder: A pupillometric study. Poster presented at the Annual Meeting of the Vision Sciences Society, May 11-16, 2012, Naples, FL.
Blaser, E., Eglington, L., Carter, A. S., & Kaldy, Z. (2013). Pupillometry reveals a mechanism for the Autism Spectrum Disorder (ASD) advantage in visual tasks (submitted).
Bölte, S., Schlitt, S., Gapp, V., Hainz, D., Schirman, S., Poustka, F., et al. (2012). A close eye on the eagle-eyed visual acuity hypothesis of autism. Journal of Autism and Developmental Disorders, 42, 726–733.
Brock, J., Xu, J. Y., & Brooks, K. R. (2011). Individual differences in visual search: Relationship to autistic traits, discrimination thresholds, and speed of processing. Perception, 40, 739–742.
Bruckner, C., & Yoder, P. (2007). Restricted object use in young children with autism: Definition and construct validity. Autism, 11, 161–171.
Burack, J. A. (1994). Selective attention deficits in persons with autism: Preliminary evidence of an inefficient attentional lens. Journal of Abnormal Psychology, 103, 535–543.
Caron, M. J., Mottron, L. L., Berthiaume, C. C., & Dawson, M. M. (2006). Cognitive mechanisms, specificity and neural underpinnings of visuospatial peaks in autism. Brain, 129, 1789–1802.
Collignon, O., Charbonneau, G., Peters, F., Nassim, M., Lassonde, M., Lepore, F., et al. (2013). Reduced multisensory facilitation in persons with autism. Cortex, 49, 1704–1710.
Cook, J. (2012, November 29). The autism advantage. The New York Times Magazine. Retrieved from http://www.nytimes.com.
Dakin, S., & Frith, U. (2005). Vagaries of visual perception in autism. Neuron, 48, 497–507.
Darling, R. D., Alzghoul, L., Zhang, J., Khatri, N., Paul, I. A., Simpson, K. L., et al. (2011). Perinatal citalopram exposure selectively increases locus coeruleus circuit function in male rats. Journal of Neuroscience, 31, 16709–16715.
Dawson, G. (2008). Early behavioral intervention, brain plasticity, and the prevention of autism spectrum disorder. Development and Psychopathology, 20, 775–803.
Dawson, G., Osterling, J., Rinaldi, J., Carver, L., & McPartland, J. (2001). Brief report: recognition memory and stimulus-reward associations: indirect support for the role of ventromedial prefrontal dysfunction in autism. Journal of Autism and Developmental Disorders, 31, 337–341.
Duncan, J., & Humphreys, G. W. (1989). Visual search and stimulus similarity. Psychological Review, 96, 433–458.
Elsabbagh, M., & Johnson, M. H. (2007). Infancy and autism: progress, prospects, and challenges. Progress in Brain Research, 164, 355–383.
Elsabbagh, M., & Johnson, M. H. (2010). Getting answers from babies about autism. Trends In Cognitive Sciences, 14, 81–87.
Elsabbagh, M., Volein, A., Holmboe, K., Tucker, L., Csibra, G., Baron-Cohen, S., et al. (2009). Visual orienting in the early broader autism phenotype: disengagement and facilitation. Journal of Child Psychology and Psychiatry, 50, 637–642.
Falkmer, M., Stuart, G. W., Danielsson, H., Bram, S., Lönebrink, M., & Falkmer, T. (2011). Visual acuity in adults with Asperger’s syndrome: No evidence for “eagle-eyed” vision. Biological Psychiatry, 70, 812–816.
Franklin, A., Sowden, P., Notman, L., Gonzalez-Dixon, M., West, D., Alexander, I., et al. (2010). Reduced chromatic discrimination in children with autism spectrum disorders. Developmental Science, 13, 188–200.
Gillberg, C., Ehlers, S., Schaumann, H., & Jakobsson, G. (1990). Autism under age 3 years: A clinical study of 28 cases referred for autistic symptoms in infancy. Journal of Child Psychology and Psychiatry, 31, 921–934.
Harms, M. B., Martin, A., & Wallace, G. L. (2010). Facial emotion recognition in autism spectrum disorders: A review of behavioral and neuroimaging studies. Neuropsychology Review, 20, 290–322.
Hollander, E., Kaplan, A., Cartwright, C., & Reichman, D. (2000). Venlafaxine in children, adolescents, and young adults with autism spectrum disorders: An open retrospective clinical report. Journal of Child Neurology, 15, 132–135.
Iarocci, G., Burack, J. A., Shore, D. I., Mottron, L., & Enns, J. T. (2006). Global-local visual processing in high functioning children with autism: Structural vs. implicit task biases. Journal of Autism and Developmental Disorders, 36, 117–129.
Jackson, I., & Sirois, S. (2009). Infant cognition: going full factorial with pupil dilation. Developmental Science, 12, 670–679.
Jarrold, C., Gilchrist, I. D., & Bender, A. (2005). Embedded figures detection in autism and typical development: preliminary evidence of a double dissociation in relationships with visual search. Developmental Science, 8, 344–351.
Joseph, R. M., Keehn, B., Connolly, C., Wolfe, J. M., & Horowitz, T. S. (2009). Why is visual search superior in autism spectrum disorder? Developmental Science, 12, 1083–1096.
Julesz, B. (1981). Textons, the elements of texture perception, and their interactions. Nature, 290, 91–97.
Kahneman, D., & Beatty, J. (1966). Pupil diameter and load on memory. Science, 154, 1583–1585.
Kaldy, Z., Kraper, C., Carter, A. S., & Blaser, E. (2011). Toddlers with autism spectrum disorder are more successful at visual search than typically developing toddlers. Developmental Science, 14, 980–988.
Kawakubo, Y., Kasai, K., Okazaki, S., Hosokawa-Kakurai, M., Watanabe, K., Kuwabara, H., et al. (2007). Electrophysiological abnormalities of spatial attention in adults with autism during the gap overlap task. Clinical Neurophysiology, 118, 1464–1471.
Keehn, B., & Joseph, R. M. (2008). Impaired prioritization of novel onset stimuli in autism spectrum disorder. Journal of Child Psychology and Psychiatry, 49, 1296–1303.
Keehn, B., Müller, R. A., & Townsend, J. (2013). Atypical attentional networks and the emergence of autism. Neuroscience and Biobehavioral Reviews, 37, 164–183.
Koh, H. C., Milne, E., & Dobkins, K. (2010). Spatial contrast sensitivity in adolescents with autism spectrum disorders. Journal of Autism and Developmental Disorders, 40, 978–987.
Laeng, B., Ørbo, M., Holmlund, T., & Miozzo, M. (2011). Pupillary Stroop effects. Cognitive Processing, 12, 13–21.
Landry, R., & Bryson, S. E. (2004). Impaired disengagement of attention in young children with autism. Journal of Child Psychology and Psychiatry, 45, 1115–1122.
Liss, M., Saulnier, C., Fein, D., & Kinsbourne, M. (2006). Sensory and attention abnormalities in autistic spectrum disorders. Autism, 10, 155–172.
Mangelsdorf, S. C., Shapiro, J. R., & Marzolf, D. (1995). Developmental and temperamental differences in emotional regulation in infancy. Child Development, 66, 1817–1828.
Mehler, M. F., & Purpura, D. P. (2009). Autism, fever, epigenetics and the locus coeruleus. Brain Research Reviews, 59, 388–392.
Milne, E., Dunn, S. A., Freeth, M., & Rosas-Martinez, L. (2013). Visual search performance is predicted by the degree to which selective attention to features modulates the ERP between 350 and 600 ms. Neuropsychologia, 51, 1109–1118.
Minzenberg, M. J., Watrous, A. J., Yoon, J. H., Ursu, S., & Carter, C. S. (2008). Modafinil shifts human locus coeruleus to low-tonic, high-phasic activity during functional MRI. Science, 322, 1700–1702.
Mottron, L. (2011). Changing perceptions: The power of autism. Nature, 479, 33–35.
Mottron, L., Bouvet, L., Bonnel, A., Samson, F., Burack, J. A., Dawson, M., et al. (2013). Veridical mapping in the development of exceptional autistic abilities. Neuroscience and Biobehavioral Reviews, 37, 209–228.
Mottron, L., & Burack, J. A. (2001). Enhanced perceptual functioning in the development of autism. In J. A. Burack, T. Charman, N. Yirmiya, & P. R. Zelazo (Eds.), The development of autism: Perspectives from theory and research (pp. 131–148). Mahwah: Lawrence Erlbaum Associates Publishers.
Mottron, L., Dawson, M., Soulières, I., Hubert, B., & Burack, J. (2006). Enhanced perceptual functioning in autism: An update, and eight principles of autistic perception. Journal of Autism and Developmental Disorders, 36, 27–43.
Mottron, L., Mineau, S., Bernier, C. S., Berthiaume, C., Dawson, M., Lemay, M., et al. (2007). Lateral glances toward moving stimuli among young children with autism: Early regulation of locally oriented perception? Development and Psychopathology, 19, 23–36.
Mundy, P., Sigman, M., & Kasari, C. (1990). A longitudinal study of joint attention and language development in autistic children. Journal of Autism and Developmental Disorders, 20, 115–128.
Nassar, M. R., Rumsey, K. M., Wilson, R. C., Parikh, K., Heasly, B., & Gold, J. I. (2012). Rational regulation of learning dynamics by pupil-linked arousal systems. Nature Neuroscience, 15, 1040–1046.
O’Riordan, M. (2000). Superior modulation of activation levels of stimulus representations does not underlie superior discrimination in autism. Cognition, 77, 81–96.
O’Riordan, M. A. (2004). Superior visual search in adults with autism. Autism, 8, 229–248.
O’Riordan, M., & Plaisted, K. (2001). Enhanced discrimination in autism. The Quarterly Journal of Experimental Psychology A: Human Experimental Psychology, 54A, 961–979.
O’Riordan, M. A., Plaisted, K. C., Driver, J., & Baron-Cohen, S. (2001). Superior visual search in autism. Journal of Experimental Psychology: Human Perception and Performance, 27, 719–730.
Ozonoff, S., Macari, S., Young, G. S., Goldring, S., Thompson, M., & Rogers, S. J. (2008). Atypical object exploration at 12 months of age is associated with autism in a prospective sample. Autism, 12, 457–472.
Petersen, S. E., & Posner, M. I. (2012). The attention system of the human brain: 20 years after. Annual Review of Neuroscience, 35, 73–89.
Plaisted, K., O’Riordan, M., & Baron-Cohen, S. (1998). Enhanced visual search for a conjunctive target in autism: A research note. Journal of Child Psychology and Psychiatry, 39, 777–783.
Porter, G., Troscianko, T., & Gilchrist, I. D. (2007). Effort during visual search and counting: Insights from pupillometry. The Quarterly Journal of Experimental Psychology, 60, 211–229.
Posner, M. I., & Petersen, S. E. (1990). The attention system of the human brain. Annual Review of Neuroscience, 13, 25–42.
Remington, A., Swettenham, J., Campbell, R., & Coleman, M. (2009). Selective attention and perceptual load in autism spectrum disorder. Psychological Science, 20, 1388–1393.
Remington, A. M., Swettenham, J. G., & Lavie, N. (2012). Lightening the load: Perceptual load impairs visual detection in typical adults but not in autism. Journal of Abnormal Psychology, 121, 544–551.
Riby, D. M., Brown, P. H., Jones, N., & Hanley, M. (2012). Brief report: Faces cause less distraction in autism. Journal of Autism and Developmental Disorders, 42, 634–639.
Rogers, S. J., Hepburn, S. L., Stackhouse, T., & Wehner, E. (2003). Imitation performance in toddlers with autism and those with other developmental disorders. Journal of Child Psychology and Psychiatry, 44, 763–781.
Sara, S. J. (2009). The locus coeruleus and noradrenergic modulation of cognition. Nature Reviews Neuroscience, 10, 211–223.
Saslow, M. G. (1967). Effects of components of displacement step stimuli upon latency for saccadic eye movement. Journal of The Optical Society Of America, 57, 1024–1029.
Sasson, N., Elison, J., Turner-Brown, L., Dichter, G., & Bodfish, J. (2011). Brief report: Circumscribed attention in young children with autism. Journal of Autism and Developmental Disorders, 41, 242–247.
Sasson, N. J., Turner-Brown, L. M., Holtzclaw, T. N., Lam, K. S. L., & Bodfish, J. W. (2008). Children with autism demonstrate circumscribed attention during passive viewing of complex social and nonsocial picture arrays. Autism Research, 1, 31–42.
Shah, A. (1988). Visuospatial islets of ability and intellectual functioning in autism. Unpublished PhD thesis, University of London.
Shah, A., & Frith, U. (1983). An islet of ability in Autistic children. Journal of Child Psychology and Psychiatry, 4, 613–620.
Shah, A., & Frith, U. (1993). Why do autistic individuals show superior performance on the block design task? Journal of Child Psychology and Psychiatry, 34, 1351–1364.
Simmons, D. R., Robertson, A. E., McKay, L. S., Toal, E., McAleer, P., & Pollick, F. E. (2009). Vision in autism spectrum disorders. Vision Research, 49, 2705–2739.
Treisman, A. M., & Gelade, G. (1980). A feature-integration theory of attention. Cognitive Psychology, 12, 97–136.
Van der Geest, J. N., Kemner, C., Camfferman, G., Verbaten, M. N., & Van Engeland, H. (2001). Eye movements, visual attention, and autism: A saccadic reaction time study using the gap and overlap paradigm. Biological Psychiatry, 50, 614–619.
Wetherby, A. M., Woods, J., Allen, L., Cleary, J., Dickinson, H., & Lord, C. (2004). Early indicators of autism spectrum disorders in the second year of life. Journal of Autism and Developmental Disorders, 34, 473–493.
Wolfe, J. M. (1994). Guided Search 2.0: A revised model of visual search. Psychonomic Bulletin & Review, 1, 202–238.
Wolfe, J. M. (1998). What can 1,000,000 trials tell us about visual search? Psychological Science, 9, 33–39.
Wolfe, J. M., & Horowitz, T. S. (2004). What attributes guide the deployment of visual attention and how do they do it? Nature Reviews Neuroscience, 5, 1–7.
Zwaigenbaum, L., Bryson, S., Lord, C., Rogers, S., Carter, A., Carver, L., et al. (2009). Clinical assessment and management of toddlers with suspected autism spectrum disorder: Insights from studies of high-risk infants. Pediatrics, 123, 1383–1391.
Zwaigenbaum, L., Bryson, S., Rogers, T., Roberts, W., Brian, J., & Szatmari, P. (2005). Behavioral manifestations of autism in the first year of life. International Journal of Developmental Neuroscience, 23, 143–152.
Acknowledgments
This research was supported by National Institutes of Health Grant 2R15EY017985 awarded to EB and ZK and by the University of Massachusetts Presidential Science and Technology Award awarded to ZK, EB and ASC.
Author information
Authors and Affiliations
Corresponding author
Rights and permissions
About this article
Cite this article
Kaldy, Z., Giserman, I., Carter, A.S. et al. The Mechanisms Underlying the ASD Advantage in Visual Search. J Autism Dev Disord 46, 1513–1527 (2016). https://doi.org/10.1007/s10803-013-1957-x
Published:
Issue Date:
DOI: https://doi.org/10.1007/s10803-013-1957-x