Abstract
Evaluations with a uniform plant stand are fundamental for efficient selections in cassava (Manihot esculenta Crantz) breeding. However, it is difficult to correct data of missing plants. The overall objective of this study was to analyze yield losses in agronomic trials and to develop a function that satisfactorily adjusts plot yields affected by a varying number of missing plants. Eight clones were initially evaluated in different locations for five consecutive years. For every genotype, mean plot yields decreased as the number of missing plants increased. Average losses ranged from 10.6 to 78.8% by removing from one to eight plants, respectively. Yields per plant increased significantly when more than four plants were removed due to a compensatory growth effect. Graphic analyses showed that the power function was the best explaining the relationship between fresh root yield and number of harvested plants. A model that properly adjusted yield for all varieties but one is proposed. Hopefully, this formula to adjust yields will help to improve the quality of cassava trials worldwide.
Similar content being viewed by others
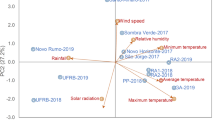
Avoid common mistakes on your manuscript.
Introduction
Uniform competition in cassava (Manihot esculenta Crantz), as for most crops, is fundamental for acceptable experimental errors in evaluation trials. However, it is not always feasible to achieve perfect plant stands in cassava trials and precision of results is frequently not satisfactory.
The morphological characteristics of cassava are highly variable. Plant height can vary from 1 to 4 m and plant type ranges from highly branching to non-branching erect types. Plant architecture influences the amount of planting material that a mother plant can produce. Erect, non-branching types generally produce larger amount of planting material and the harvest, storage and transport of stems is greatly facilitated (Ceballos and de la Cruz 2002; Alves 2001). The number of commercial stakes obtained from a single mother plant in a year ranges from 3 to 30, depending upon growth habit, climate, management, and soil conditions. This is considerably less than the propagation rate that can be achieved with other commercial crops (Leihner 2001).
When roots are harvested the previous season, the stems are also collected and stored, typically under the shade of a tree (Ceballos et al. 2007; Morante et al. 2005). Stems can only be stored for 1 to 2 months, depending on the environmental conditions. Several physiological and sanitary factors affect sprouting capacity which, combined with the low multiplication rate of planting material, results in frequent and chronic problems of variation in plant densities.
The effect of missing plants on plot yield may not be noticeable when there are one or two missing plants. The compensatory growth of neighboring plants usually helps to reduce differences in total plot yield. However, as the proportion of missing plants increases, the compensatory growth of the remaining plants is not enough to correct total plot yield (Gomez and De Datta 1972; James et al. 1973; Kamidi 1995; Mead 1968).
The covariate analysis can some times adjust cassava experimental plot yields when the plans are missing only for a short time before harvest time. However, when plants are missed throughout the growing season, competition effects and compensatory growth invalidate the linear covariance adjustment. Therefore, the relationship between plot yield and plot stand is no longer linear, and linear covariance analysis may result in unreliable yield estimates and failure to reduce experimental errors. The relationship between plant density and crop yield has received previous attention (Schmildt et al. 2001; Vencovsky and Cruz 1991; Verones et al. 1995; Willey and Heath 1969). Kamidi (1995) proposed an exponential model to correct plot stands in maize reinforcing the concept that the linear covariance analysis using plot stand as the covariate is no satisfactory, especially when the plants are missing long before maturity.
The objectives of this work were to estimate yield losses due to missing plants in experimental cassava trials and propose a model that can be used to correct yields based on ideal plant stands.
Materials and methods
Field evaluation trials
A set of agronomic evaluations, with eight different varieties, were conducted during five years at four contrasting environments in Colombia (Departments of Atlántico, Cauca, Meta and Valle del Cauca). For each variety, eight different treatments were applied by removing one, two, and up to eight ‘central’ plants of each plot, as well as a control treatment (no missing plant). The plants inside experimental plots were numbered as illustrated in Fig. 1, and were randomly removed from the treatment plot to achieve the specific treatment target 2 months after planting (before plant competition between neighboring plants started). Plots consisted of five rows of five plants, spaced 1 m apart within rows and 1 m between rows (standard plant density for cassava).
The number of experiments per variety was variable (Table 1). Some varieties were evaluated only 1 year, while others were evaluated for the 5 years this study lasted and at more than one environment. Eight cassava varieties were originally evaluated: CM 4919-1 and MTAI 8 are adapted to sub-humid environment; CM 4574-7, CM 6438-14 and CM 6740-7 are adapted to acid-soil savannas; CM 523-7, MCOL 1505 and SM 1058-13 are adapted to the mid-altitude valleys environment. These varieties differ in branching type and, therefore, competitive ability.
The design used was a randomized complete block design with three replications per experiment. Individual analyses of variances were performed for each experiment and combined for each variety within each environment and year. Graphic analyses were used to identify the best model to explain the relationship between fresh root yield and number of plants harvested. The information produced from the analysis of these trials was used to compare different models and select the best one based on its capacity to correct measured yields to the perfect plant stand.
Estimation of yield losses due to missing plants
Graphic analysis was initially performed to understand the relationship between plot yields of perfect plant stands (no missing plant) versus those for each treatment (different number of missing plants). This analysis showed that the power function best explained the relationship between fresh root yield and number of harvested plants. This function, in all cases, presented an R 2 value above those of the others functions analyzed (exponential, logarithmic and linear). The proposed model considers a power decline of yield associated with decreasing plant stand. The adjusted plot yield was, therefore, a function of both observed plot yield and plot stand, as follows:
where y a is the adjusted plot yield, y o the observed plot yield, N a is the ideal plot stand (in this case nine plants), N o is the number of harvested plants (in this experiment varying from one to nine depending on the assigned treatment), and α and β are unknown parameters. This model imposes the requirement that the adjusted yield should coincide with the observed yield when ideal and observed plot stands are equal. Additionally, a linear model was also fitted to the data, as follows:
where y a, y o, N a, and N o are the same parameters as indicated above.
The α and β values were estimated by a non-liner least squares iterative procedure. The SAS non-linear regression procedure based on the modified Gauss–Newton method was used to fit the proposed model. The models fit were assessed from the coefficients of determination (R 2) and magnitude of the residual values. Additionally, the invariances of the best models were tested. This test helped to define whether or not to fit with a common α and β for all cassava varieties and all environments (Boché and Lavalle 2004). Statistical tests of these hypotheses were performed on the basis of the extra sums of squares or the conditional error principle (Milliken and Debruin 1978).
Results
Analysis of variance for each experiment showed, as expected, significant differences among treatments for fresh root yield per plot (Table 1). The coefficient of variations (CV) ranged from 10.5 (Experiment 3) to 47.9% (Experiment 10). Experiments with CV above 30% (Experiments 10, 11 and 22) and two experiments with high root-rot incidence (Experiments 8 and 9) were eliminated from further analyses. The combined analyses of variance for each variety across years within environments showed highly significant differences among years and treatments (Table 2). The treatment-by-environment interactions were not significant, except for clone MTAI 8, which presented highly significant values. It is important to note that varieties CM 4574-7 and CM 6740-7, adapted to the acid-soil savannas (Meta), were also evaluated in the mid-altitude valleys environment (Valle del Cauca).
Figure 2 illustrates the relationship between yield per plant and number of missing plants. As expected, fresh root yield on a per plant basis remains relatively stable when few plants are missing. However, as the number of missing plants is ≥4, the yield per plant tends to increase considerably. For all varieties the mean plot yield decreased as the number of missing plant increased (Table 3). Average yield losses by removing one up to eight plants ranged from 10.6 to 78.8%, respectively.
The R 2 values were computed from the analysis of variance routine provided on the SAS listing. The power model was associated with a largest value of R 2 (0.9438) considerably better than the linear regression model (R 2 = 0.5973). Convergence of the power model was achieved in fewer than four iterations. Plots of the predicted yield ratio against the corresponding observed values indicated that the power model was appropriate. The fitted curve and the actual values are shown in Fig. 3. It can be observed that variability increases as the number of missing plants increases. In other words, as the number of plants increased the reliability of the adjustment was reduced.
Analysis of residuals for all analyses indicated little evidence to disprove the hypothesis that residuals were normally distributed with a mean equal to zero. The approximate F statistics developed by Milliken and Debruin (1978) were used to test the significance of the extra sums of squares due to common fits. Significant differences were detected between parameters for varieties and environment (P < 0.05). Table 4 shows the estimated parameter values individually for each variety, environment and combined data.
The fitted curves for all varieties are depicted in Fig. 4. Invariance analysis for most varieties did not show significant differences between their models indicating similar responses. Variety CM 4919-1, on the other hand, showed highly significant differences compared with the other varieties. According to the information generated, as expected, there was a variation in the response to missing plants for different varieties or the different environments where the trials were conducted. Nonetheless, a general model across varieties and environments was evaluated resulting in estimates for α = 0.727 and β = 0.805.
The general model was used to estimates adjusted yield to uniform full plot stands for each variety (Table 3). The analysis of variance (data not shown) indicated no significant difference after adjusted yield for all varieties, except for CM 4919-1, indicating a good fit of the proposed general model to adjust yield plot when there are missing plant in experimental plots, regardless of the environment were the trials are conducted, the varieties used or the number of missing plants.
Discussion
The results obtained in this work supported the expected effects of missing plant in field evaluations of cassava. The yield per plant increased along with the number of missing plants, mainly because the remaining plants around the missing one(s) were favored by less competition for limiting environmental factors such as light, water and nutrients (Fig. 2). The average yield when only one plant was harvested varied from 3.7 (CM 6740-7) to 10.2 kg (SM 1058-13), indicating large variation between varieties (Table 3).
The ultimate objective of this study was to develop a model capable of adjusting total plot yields (for treatments where one or more plants were missing) as close as possible to the values observed in the perfect plant stand of the same variety. The analysis of invariance, taking into account varieties and environments, showed similar responses to different groups. However, some models had significant differences indicting that for specific varieties and environment their α and β parameters were different.
The general model across environments and varieties (based on α = 0.727 and β = 0.805) was used to adjust total plot yields as presented in Table 3. It is recognized that, ideally, the correction for missing plants should be done individually for each variety and/or location. However, the information required to make such adjustment is usually missing beforehand and, consequently, such adjustment is seldom possible. The application of a more general model that can be applied by default in the analysis of different trials is highly desirable (Gomez and De Datta 1972), even if the precision in the adjustment is not perfect. The interest to develop a general model applicable to different cassava varieties and environmental conditions defined the nature of this study. Different set of environments with varying average yield potential and the use of varieties with contrasting plant architectures was purposely chosen, therefore, for this study.
There are few available options to reduce the experimental errors derived from missing plants. The most obvious strategy would be to maximize the possibility of obtaining perfect plant stands. In many crops it is feasible to overplant and then reduce the number of surviving plants down to the desired plant density. However, in the case of cassava, availability of planting material is a chronic limitation because of the low multiplication rate (Ceballos et al. 2007). This is particularly the case in recurrent selection schemes (Morante et al. 2005). Therefore, the occurrence of missing plants is unavoidable and approaches to adjust yields a necessity. The simplest correction would be a linear approach based on the yield per plant estimate: (total plot yield/number of harvested plants)*ideal plant stand. As demonstrated (Fig. 2), however, this approach would tend to overestimate corrected yields when the number of missing plants is high. Another linear correction could be based on the co-variance analysis. At the bottom of Table 3 the standard deviation of the corrected plot means for these two approaches is presented. In every case the application of the general model proposed in this study resulted in considerably smaller standard deviation values, indicating that the general model is better than other available methods.
The particular performance of cultivar CM 4919-1, a widely grown variety in the sub-humid environment of Colombia’s northern coast, was interesting because it failed to fit the general model proposed in this study. Plant height of CM 4919-1 was relatively low and it was the only clone that did not branch at all, showing a very distinctive, completely erect plant type. These features could explain the atypical behavior of this clone. Still the general model provided the best adjustment for total plot yields (Table 3).
Until now, cassava breeders have used two unsatisfactory approaches to overcome the problem of missing plants (re-planting a stem cutting to replace the missing plant or harvest plants in a border row). The general model proposed in this study can only be applied in trials where no such corrective measures have been used. Comparisons of coefficient of variation before and after adjusting the means would provide a fair estimate of the relative values of the method. The proposed general model is:
where each parameter is the same as described in the materials and method section.
References
Alves AAC (2001) Cassava botany and physiology. In: Hillocks RJ, Thresh JM, Bellotti AC (eds) Cassava: biology production and utilization. CABI Publishing, Wallingford, pp 67–89
Boché S, Lavalle A (2004) Comparación de modelos no lineales, una aplicación al crecimiento de frutos de carozo. Rev Soc Arg Gen 8(1). http://www.s-a-e.org.ar/revista-vol8.htlm
Ceballos H, De la Cruz GA (2002) Taxonomía y Morfología de la Planta. In: Ospina B, Ceballos H (eds) La Yuca en el Tercer Milenio. CIAT, Cali, pp 17–33
Ceballos H, Fregene M, Pérez JC, Morante N, Calle F (2007) Cassava genetic improvement. In: Kang MS, Priyadarshan PM (eds) Breeding major food staples. Blackwell Publishing, Ames, pp 365–391
Gomez KA, De Datta SK (1972) Missing hill in rice experimental plots. Agron J 64:163–164
James WC, Lawrence CH, Smith CS (1973) Yield losses due to missing plants in potato crops. Am Potato J 50(10):345–352
Kamidi RE (1995) Statistical adjustment of maize grain yield for sub-optimal plots stands. Exp Agric 31:299–306
Leihner D (2001) Agronomy and cropping systems. In: Hillocks RJ, Thresh JM, Bellotti AC (eds) Cassava: biology, production and utilization. CABI Publishing, Wallingford, pp 91–113
Mead R (1968) Measurement of competition between individuals plants in a population. J Ecol 56(1):35–45
Milliken GA, Debruin RL (1978) A procedure to test hypotheses for nonlinear models. Commun Stat Theory Methods 7:65–69
Morante N, Moreno X, Pérez JC, Calle F, Lenis JI, Ortega E, Jaramillo G, Ceballos H (2005) Precision of selection in early stages of cassava genetic improvement. J Root Crops 31:81–92
Schmildt ER, Cruz CD, Zanuncio JC, Pereira PRG, Ferrão RG (2001) Avaliação de métodos de correção do estande para estimar a produtividade em milho. Pesq Agrop Bras, Brasilia 36(8):1011–1018
Vencovsky R, Cruz CD (1991) Comparação de métodos de correção do rendimeinto de parcelas com estandes variados, I. Dados Simulados. Pesq Agropec Bras, Brasilia 26(5):647-657
Verones JA, Cruz CD, Correa LA, Scapim CA (1995) Comparação de métodos da ajuste do rendimiento de parcelas con estandes variados. Pesq Agropec Bras, Brasilia 30(2):169–174
Willey RW, Heath SB (1969) The quantitative relationship between corn populations and yield. Adv Agron 21:281–321
Author information
Authors and Affiliations
Corresponding author
Rights and permissions
About this article
Cite this article
Pérez, J.C., Ceballos, H., Ramírez, I.C. et al. Adjustment for missing plants in cassava evaluation trials. Euphytica 172, 59–65 (2010). https://doi.org/10.1007/s10681-009-0039-9
Received:
Accepted:
Published:
Issue Date:
DOI: https://doi.org/10.1007/s10681-009-0039-9