Abstract
Genotype × environment interaction (GEI) affects marketable fruit yield and average fruit weight of both hybrid and open-pollinated (OP) tomato genotypes. Cultivars vary significantly for marketable fruit yield, with hybrid cultivars having, on average, higher yield than OP cultivars. However, information is scanty on environmental factors affecting the differential response of tomato genotypes across environments. Hence, the aim of this research was to use factorial regression (FR) and partial least squares (PLS) regression, which incorporate external environmental and genotypic covariables directly into the model for interpreting GEI. In this research, data from an FAO multi-environment trial comprising 15 tomato genotypes (7 hybrid and 8 OP) evaluated in 18 locations of Latin America and the Caribbean were analyzed using FR and PLS. Environmental factors such as days to harvest, soil pH, mean temperature (MET), potassium available in the soil, and phosphorus fertilizer accounted for a sizeable portion of GEI for marketable fruit yield, whereas trimming, irrigation, soil organic matter, and nitrogen and phosphorus fertilizers were important environmental covariables for explaining GEI of average fruit weight. Locations with relatively high minimum and mean temperatures favored the marketable fruit yield of OP heat-tolerant lines CL 5915-223 and CL 5915-93. An OP cultivar (Catalina) and a hybrid (Apla) showed average marketable fruit yield across environments, while two hybrids (Sunny and Luxor) exhibited outstanding marketable fruit yield in high yielding locations (due to lower temperatures and higher pH) but a sharp yield loss in poor environments. Two stable hybrid genotypes in high yielding environments, Narita and BHN-39, also showed high and stable yield in average and low yielding environments.
Similar content being viewed by others
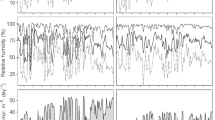
Avoid common mistakes on your manuscript.
Introduction
Tomato (Lycopersicon esculentum Mill.), which was domesticated in ancient Peru, has become the most popular and widely consumed vegetable in the world today, due to its flavor, nutritional value (high in vitamins C and A), short growth cycle, and relatively high yield. Although tomato is widely grown, the environment affects the performance of tomato genotypes substantially—e.g., in Latin America and the Caribbean (Ortiz and Izquierdo 1992), North America (Poysa et al. 1986), and Spain (Cuartero and Cubero 1982).
Open-pollinated (OP) and hybrid (H) cultivars are used by tomato farmers worldwide, depending on their access to inputs and markets. Cuartero and Cubero (1982) indicated that hybrids appeared to be more stable than their parents in multi-environment trials (METs) across four Spanish locations. Furthermore, stability analyses have been used to select stable, high yielding genotypes (Berry et al. 1988; Izquierdo et al. 1980; Ortiz 1991; Ortiz and Izquierdo 1994; Stofella et al. 1984) or cultivars with a small and stable blossom-end scar, a disorder in tomato fruit that reduces its marketability (Elkind et al. 1990). In some of the trials, unstable genotypes showed high yields in optimum environments, while low yielding genotypes were more stable across environments (Stofella et al. 1984). In most of the above research, each genotype’s yield stability was quantified using the traditional regression approach (Finlay and Wilkinson 1963; Eberhart and Russell 1966), which involves regressing the individual genotypes’ yield on the environmental means. Poysa et al. (1986) mentioned that many high yielding, useful tomato genotypes could be identified as unstable by regression analysis. However, the simple regression method does not allow incorporating other external covariables that may affect GEI and yield stability. Quantifying the environmental factors and climatic variables that affect tomato yield and GEI is of paramount importance for understanding the stability of genotypes across different environmental conditions.
In agriculture, METs are essential for selecting the most productive and stable cultivars to be used at different sites. Several statistical methods can be used to study GEI (Crossa 1990). Statistical models that incorporate a large number of external covariables into the analysis of MET have recently been employed for studying and explaining GEI (Vargas et al. 1998, 1999). Two of these models are the factorial regression model (FR) (Denis 1988; van Eeuwijk et al. 1996) and the partial least squares (PLS) regression method (Aastveit and Martens 1986; Talbot and Wheelwright 1989). The FR are ordinary linear models that allow the inclusion of external variables such as climatic data. When meteorological data or soil variables are available, they show high collinearity; because they are estimated very imprecisely, the interpretation of least squares regression coefficients is complicated. The PLS are bilinear models that offer a solution to the multicollinearity problem of external covariables.
Various authors have used FR and PLS for determining the most important environmental covariables influencing the GEI of grain yield in METs. A parsimonious description of agronomic treatments × environment interaction using FR and PLS was provided by Vargas et al. (2001) to investigate the factorial structure of the treatments and reduce the number of treatment terms in the interaction. Vargas et al. (1999) used a MET and an agronomic trial to compare results from AMMI, FR, and PLS for interpreting GEI in terms of external environmental and genotypic covariables. Reynolds et al. (2004) explained some of the physiological bases of GEI using FR and PLS in two historical wheat CIMMYT METs. They found that post-anthesis environmental conditions influenced GEI more than pre-anthesis environmental conditions. The analysis of GEI in winter wheat genotypes using FR with environmental covariates proved to be useful for identifying genotypes with sensitivity to certain environmental covariates (Brancout-Hulmel et al. 2000). Brancout-Hulmel et al. (2003) studied the effect of certain environmental variables on GEI of winter wheat using FR and the Additive Main effects and Multiplicative Interaction (AMMI). They concluded that FR is more powerful than AMMI because the sensitivity of different genotypes to environmental variables can be determined.
In some tropical growing locations, high temperature, flooding, and numerous disease and insect problems drastically reduce tomato yield (AVRDC 2001). Temperatures ranging between 27°C and 30°C during the day and 20°C at night affect fruit set in tomato (Rudick et al. 1977; Rylski 1979). Improved tomato lines with heat tolerance and multiple resistance to bacteria, fungi, and viruses, coupled with appropriate management practices, are needed to overcome such constraints of the hot-wet season. Developing inbred lines combining heat tolerance, multiple disease resistance, and good fruit quality has been difficult (AVRDC 2003). Furthermore, tomato research on genotypic stability and assessment of the GEI of economically important traits such as fruit yield and weight using environmental covariates have not been conducted. Therefore, studying the influence of climatic variables on the GEI of economically important tomato traits would provide valuable information to tomato breeders.
The Food and Agriculture Organization of the United Nations (FAO) organized a multilocational tomato trial for Latin America and Caribbean to assist national programs in the region in the systematic assessment of available OP and hybrid commercial cultivars. The objective of this research was to use FR and PLS in the FAO trial, consisting of 15 genotypes (8 OP and 7 hybrids) evaluated in 18 environments of Latin America and the Caribbean, for interpreting GEI of fruit yield and weight in terms of several environmental covariables. This will allow tomato breeders to group breeding materials and define their respective target populations of environments.
Materials and methods
Eight OP and 7 hybrid genotypes were planted in each of 18 locations in Latin America and the Caribbean. The genotypes (code numbers in brackets) were selected by breeders contributing to this multilocational trial based on their potential for becoming commercial cultivars in the region, irrespective of their breeding system or growth habit. The 8 OP genotypes were: Catalina [1], Dina RP [2], Licapal 21 [3], Truique [4]) Angela Gigante [10], CL 5915-223 [12], CL 5915-93 [13], and Flora Dade [15], which was widely grown in both America and Australia due to its firm fruit and shelf-life (Sumeghy 1983). The AVRDC CL genotypes [12] and [13] derive from the line CL5915-93D4-1-0-3, a valuable source of heat tolerance genes for tomato genetic improvement whose fruit set inheritance under high temperature could be accounted for by a simple additive and dominance effect model (Hanson et al. 2002). The seven tomato hybrids were: Apla [5], Narita [6], Contessa [7], Luxor [8], BHN-39 [9] Sunny [11], and NC EBR-2 [14]. The tomato cultivars included in this research showed determinate [4, 5, 6, 7, 8, 9, 11, 12, 13, 14], semi-determinate [1, 2, 15], or indeterminate growth [3, 10]. The Sp locus controls the growth type in tomato; indeterminate (Sp+) is dominant over determinate (Sp−) growth, which produces only a limited number of trusses and may be influenced by the environment (UPOV 2001). The determinate growth habit includes the semi-determinate types, which do not show their leaves or internodes between inflorescences. Similar to tomato of indeterminate growth habit, the shoots of semi-determinate types produce several flower clusters to the side of an apparent main stem, but occasionally the shoot ends in a flower cluster, as in the determinate growth habit.
Phenotypic data
Seeds of each of the 15 test genotypes were provided by private and public breeders, and distributed from the same lot to all cooperators involved in this METs. The field layout in each location was a randomized complete block design with four replications of each genotype. Plots consisted of 36 plants, 4 rows of nine plants each. The experimental unit for determining average fruit weight (g fruit−1) consisted of 5 plants per row, all from the middle 2 rows; following UPOV (2001) guidelines for recording data on at least 10 competitive plants used in tomato testing. The distance between rows was 1.5 m, with 0.5 m between plants. Local agronomic practices were used at each field location. Fields were fertilized with N–P–K (basal application and side-dressings; total amounts given in Table 1). Insects were controlled with pesticides, and furrow irrigation was applied as needed. Yield was evaluated for ripe fruit according to the maturity of each genotype. Tomato fruits were suitable for use in making paste or ketchup, or for selling fresh in the market, where consumers prefer larger sizes. Fruit with diameters of >48 mm were therefore considered marketable. Marketable fruit yield (t ha−1) was recorded on a per plot basis, and the same method and plot size were used across all locations. Soil conditions (soil type, percentage of organic matter, natural fertility, pH), meteorological data (temperature, day length, rainfall), and disease and pest management were recorded for each environment (Table 1).
Environmental data
The 18 environments in Latin America and the Caribbean were part of a regional tomato METs organized by FAO in 1991–1992 (Ortiz and Izquierdo 1994). Table 1 lists the locations, codes, and environmental covariables recorded by national tomato breeding program staff in each environment. These data were used in FR and PLS statistical analyses for explaining GEI for marketable fruit yield (t ha−1) and fruit weight (g). There were five climatic variables: maximum temperature (MXT), minimum temperature (MNT), mean temperature (MET) (all given in °C), rainfall (mm) (PRC), and degree days (DAY, base 10). Seven soil variables were recorded in field plots in the different countries where the trials were conducted: soil pH (PH), soil organic matter (OM, %), phosphorus (P, as P2O5 in ppm), potassium (K, as K20 in me/100 g), extra nitrogen (EX_N, kg ha−1), extra phosphorus (EX_P, kg ha−1), and extra potassium (EX_K, kg ha−1). The other four external variables were: trimming (TRM), drivings (DRI), irrigation (IRR), and days to harvest (DHA). The values of the climatic variables MXT, MNT, MET were averages of the entire cropping season, whereas PRC and DAY were accumulated from sowing day to harvesting day. The value of each environmental variable measured in each trial is given in Table 1.
The factorial regression model
Complete descriptions of the FR model are given in Denis (1988) and van Eeuwijk et al. (1996). The FR models the GEI directly using regressions on environmental (and/or genotypic) variables (Denis 1988; van Eeuwijk et al. 1996). FR models are ordinary linear models that aim to replace, in the GEI subspace, genotypic and environmental factors with a small number of genotypic (or environmental) covariables or genotypic sensitivities and environmental potentialities. FR models approximate GEI effects by the products of one or more (1) genotypic covariables (observed) × environmental potentialities (estimated), (2) genotypic sensitivities (estimated) × environmental covariables (observed), and (3) scale factor (estimated) × genotypic covariables (observed) × environmental covariables (observed).
For h = 1,..., H environmental covariables (centered) represented by \( z_{j1 } , \ldots ,z_{jH} , \) the linear model is \( \bar y_{ij} {\text{ = $\mu$ + $\tau $ }}_i {\text{ +$ \delta $ }}_j {\text{ + }}\sum\nolimits_{h{\text{ = 1}}}^H {{\text{$\varsigma $ }}_{ih} {\text{z}}_{jh} } {\text{ + $ \overline \varepsilon $ }}_{ij} \), H ≤ J − 1, where τ i and δ j denote the effects of genotypes and environments, respectively, and \({\text{ $\varsigma $ }}_{ih} \) represents a genotypic sensitivity (regression coefficient) with respect to the environmental covariable \( z_{jh} \). Constraints on the parameters are \( \sum\limits_i {{\text{ $\tau $ }}_i {\text{ = }}\sum\limits_j {{\text{ $ \delta $ }}_j {\text{ = }}\sum\limits_i {{\text{ $ \varsigma $ }}_{ih} {\text{ = 0}}} } } \). In matrix notation, the expectation is
where Z = [z jh ] is the J × H matrix of known environmental covariables, and ζ = [ζ ih ] is the I × H matrix of unknown differential genotypic sensitivities. The model should be fitted for all possible environmental covariables. The mean squares of the environmental covariable (i.e., MXT) × genotype were tested against the mean square error combined across all the environments. Only these significant covariables were considered as important for explaining total GEI variability.
When there is a high number of environmental (or genotypic) covariables that show high collinearity, the interpretation of the least squares regression coefficients is complicated because they are estimated very imprecisely. Therefore the stepwise procedure for choosing which covariables to include, implemented in release 8.1 of GENSTAT (2005), is useful for model construction. In this research GENSTAT release 8.1 was used for the FR analysis; the codes can be obtained from the second or third author.
Noise on the response variable also complicates the interpretation of FR parameters. Furthermore, since least squares estimation of the parameters in FR models is not unique when the number of covariables is larger than the number of observations, an alternative estimation method is needed. Partial least squares regression overcomes some of these problems and may be used as an alternative estimation method, as clearly described by Vargas et al. (1999).
Partial least squares
A full description of PLS can be found in Vargas et al. (1998, 1999). As in FR, PLS describes GEI in terms of differential sensitivity of genotypes to environmental covariables. However, these explanatory variables are hypothetical variables corresponding to linear combinations of the complete set of measured environmental variables, and there is no limit to the number of explanatory variables that can be used.
Multivariate Partial Least Squares (PLS) regression models (Aastveit and Martens 1986; Helland 1988) are a special type of bilinear model. When genotypic responses across environments (Y) are modeled using environmental covariables, the J × H matrix Z of H (h = 1,2,...,H) environmental covariables can be written in bilinear form as:
where the matrix T contains t 1 ⋯ t J J × 1 vectors called latent environmental covariables or Z-scores (indexed by environments), the matrix P has p 1...p H H × 1 vectors called Z-loadings (indexed by environmental variables), and E has the residuals. Similarly, the response variable matrix Y in bilinear form is
where the matrix Q contains q 1...q I I × 1 vectors called Y-loadings (indexed by genotypes) and F has the residuals. The relationship between Y and Z is transmitted through the latent variable T. The PLS algorithm performs separate (but simultaneous) principal component analysis of Z and of Y, which allows reducing the variables in each system to a smaller number of more interpretable latent variables. Helland (1988) showed that a reduced number of PLS latent variables give a low rank representation of the least squares estimates of the FR with environmental covariables because the expectation of Y′ is
(as in Eq. 1 of the FR), where T, Q, and Z are defined as before, and the vector W is H × 1 and contains the Z-loadings (or weights) of the environmental covariables; ζ contains the PLS approximation to the regression coefficients of the responses in Y to the environmental covariables in Z. The matrices T (with J coordinates for environments), Q (with I coordinates for genotypes), and W (with H coordinates for environmental covariables) can be represented in the PLS biplot such that projecting the jth environment (row) of T on the ith genotype (row) of Q [Y′ = (TQ′)′] approximates the G×E; and projecting the hth environmental covariable (row) of W on the ith genotype (row) of Q (QW′ = ζ) approximates the regression coefficient of the ith genotype on the hth environmental covariable (Vargas et al. 1999).
The PLS biplot
One advantage of the PLS is that results can be represented graphically in the form of biplots that give a general overview of the genotypes, environments, and environmental variables affecting GEI. Complete details and interpretation of the various kinds of biplots (including the PLS biplot) can be found in Vargas et al. (1999). The scores of the first two PLS factors for genotypes and environments are vectors in a space with starting points at the origin and end points determined by the scores. The distance between the end points of two genotype vectors (or environment vectors) indicates the amount of interaction between the genotypes (or environments). The cosine of the angle between two genotype (or environment) vectors approximates the correlation between genotypes (or environments). Acute angles indicate positive correlations, obtuse angles indicate negative correlations, and a right angle indicates no correlation. Environment and genotype vectors with the same direction have positive interaction, whereas vectors in opposite directions have negative interaction. Environmental variables in the same direction as the environments have a high value in those environments, whereas environmental covariables in opposite direction to the environments have low value in those environments. The PLS analyses and PLS biplots of this research were done in SAS (1999); the codes can be obtained from the second or third author.
Results and discussion
In a previous study, Ortiz and Izquierdo (1994) determined the yield stability of each genotype using the regression of the yield of individual genotypes on the environmental index, which was measured by the mean of all the genotypes grown in an environment. In that study, hybrid genotype Narita [6] and determinate OP Dina RP [2] were the most stable, whereas semi-determinate OP Flora Dade [15] showed an unstable marketable fruit yield.
The environment substantially affects the performance of tomato genotypes in the various countries of Latin America and the Caribbean, and the test cultivars varied significantly for both marketable fruit yield (Table 2) and average fruit weight (Table 3) in high and low yielding environments. On average hybrid genotypes (all with determinate growth) yielded more than OP cultivars, and some hybrids (e.g., Narita [6]) performed well even in very low yielding environments. The determinate OP cultivar Truique [4] was outstanding for average fruit weight across environments.
The mean marketable fruit yield and average fruit weight, and their corresponding GEI (residual after adjusting for the main effects of genotype and environments), for the 15 tomato cultivars tested are shown in Tables 2 and 3, respectively. GE interactions were significant in all the environments for both traits (Tables 4, 5). Two stable hybrid genotypes in high yielding environments (Narita [4] and BHN-39 [9]) also showed high and stable yield in average and low yielding environments. The determinate OP cultivar Catalina [1] and hybrid Apla [5] showed average marketable fruit yield across environments, while hybrids Sunny [11] and Luxor [8] exhibited outstanding marketable fruit yield in high yielding environments but a sharp yield loss in poor environments (due to higher temperatures and lower pH). Sunny was found to be a suitable cool-season (early-autumn transplanting) cultivar on the northern coast of New South Wales in Australia (Huett 1984), which may explain its poor performance in the heat-prone environments of Latin America and the Caribbean.
The results from the METs in Latin America and the Caribbean suggest that neither the heterogeneous composition of an OP cultivar nor the heterozygosity per se of a hybrid account for yield stability across environments in this region. As indicated by Ortiz and Izquierdo (1994), alleles that confer broader adaptation may likely be required to achieve tomato yield stability across environments. Hence, it is possible to select for yield stability in tomato genotypes, but they need to be grown in advanced tomato breeding trials for several seasons to identify high yielding and stable genotypes (Berry et al. 1988).
Factorial regression and partial least squares for marketable fruit yield
The FR model with a stepwise regression procedure for variable selection was used to determine the most informative subset of environmental covariables affecting marketable fruit yield. The subset of independent environmental covariables that explained 62% of total GEI included days to harvest (DHA×gen), soil pH (PH×gen), mean temperature (MET×gen), potassium (K×gen), extra phosphorus (EX_P×gen), and minimum temperature (MNT×gen) (Table 4). Both P and K are important for the crop; soft fruit and poor skin are affected by low K, whereas poor root growth and poor fruit development, which influence fruit weight, are associated with low P. Days to harvest (DHA) and soil pH (PH) together explained 34% of total GEI variability with only 28 degrees of freedom (from a total of 238 degrees of freedom). The application of nitrogen fertilizer (EX_N) explained a small portion of GEI variability. Tomatoes need N for vigorous growth, and stunted plants result from insufficient N fertilizer. Severe N stress can reduce tomato fruit yield by 60–70% (Scholberg et al. 2000). The remaining environmental covariables were statistically significant but did not explain much GEI variability for marketable fruit yield.
The PLS offers the possibility of including all factors affecting GEI: genotypes, environments, and their climatic components. The first PLS factor explained 27% of the GEI sum of squares, while the second PLS factor explained 11%. The PLS biplot is useful for understanding the causes of GEI. Figure 1 depicts the first two PLS factors with all 15 tomato genotypes evaluated in the 18 environments, plus 16 environmental covariables. The environmental covariables that most explained GEI in the FR analyses (DHA, PH, MET, MNT, EX_P, and K) tend to be located farther from the center of the PLS biplot, indicating that they caused large GEI for marketable fruit yield, as previously detected by the FR analysis.
Plot of the the first two partial least squares regression factors (factors 1 and 2) for marketable fruit yield for tomato 15 cultivars tested across 18 environments in Latin America and the Caribbean. Environment codes are given in Table 1 footnote and genotype codes are in Table 2 footnote. Environmental variables are: MXT: maximum temperature; MNT: minimum temperature; MET; mean temperature (all in °C); PRC: rainfall (mm); DAY: degree day; PH: soil pH; OM: organic matter; P: phosphorus; K: potassium; EX_N: extra nitrogen; EX_P: extra phosphorus; EX_K: extra potassium; TRM: trimming; DRI: drivings; IRR; irrigation; DHA: days to harvest
The PLS biplot for marketable fruit yield shows general GEI patterns with respect to environments, genotypes, and environmental covariables. Environments located on the right hand side of the PLS biplot (04, 06, 07, 11, 14, 15, 20, 27, 50, and 53) have relatively high values for environmental covariables located in the same direction (MET, MNT and DAY; Table 1), whereas sites located on the opposite side of the biplot (05, 21, 40, 41, 42, 43, 44, and 51) tend to have high soil pH (PH) and longer cropping seasons (DHA). As for genotypes, the first PLS axis clearly separates hybrid tomato genotypes (5, 6, 7, 8, 9, 11, and 14) (on the left) from OP genotypes (1, 2, 3, 4, 10, 12, and 13) (on the right), whereas the second PLS axis separates OP genotypes Catalina [1], Dina RP [2] (both showing semi-determinate growth), and Truique [4] (determinate growth) from Licapel 21 [3], Angela Gigante [10] (both indeterminate growth), and AVRDC CL lines [12, 13] (both showing determinate growth). These results indicate that, in terms of GEI, OP semi-determinate tomatoes Catalina [1], Dina RP [2], and determinate OP cultivar Truique [4] performed better in environments 04, 06, 07, 20, and 50, whereas OP Licapal 21 [3], Angela Gigante [10] (both indeterminate], and AVRDC CL lines [12, 13] performed better in environments 11, 14, 15, 27, and 53 (Table 2). The latter tend to have positive GEI in those sites and are thus favored by the relatively high degree day (DAY), and minimum [MNT] and mean [MET] temperatures prevailing in those environments. Because the first two PLS components accounted for only a portion of the GEI, some distortions in the PLS biplots are evident, such as the negative GEI value for Catalina [1] in sites 04 and 50, for Angela Gigante [10] in site 14, and for genotype CL 5915–93 [13] in sites 11 and 27. On the other hand, OP cultivars are not well adapted to environments with higher days to harvest (DHA) and soil pH (PH), such as environments 05, 21, 40, 41, 42, 43, 44, and 51 (Table 2) (i.e., they tend to have more negative GEI in those sites). However, the opposite is true for hybrid genotypes, which are more negatively affected by high temperatures than OP genotypes but are favored by soils with higher pH.
Although tomatoes grow well over a wide range of temperatures, fruit set is very sensitive to high temperatures, which decrease levels of auxin- and gibberellin-like substances, especially in floral buds and developing fruits (Kuo and Tsai 1984). This shortage of auxin and gibberellins could reduce fruit set in high temperatures (Sasaki et al. 2005). Also, flowers may produce oddly shaped fruit or fall off without setting any fruit at all. The METs results from Latin America and the Caribbean confirm that high mean temperature lead to low marketable fruit yield. Furthermore, watering transplants excessively under high temperature results in thin, leggy stems that lead to low yielding plants. Dinar and Rudich (1985) found that several physiological and biochemical processes (such as photosynthetic enzyme activity, membrane integrity, photophosphorylation, and electron transport in chloroplast, stomatal conductance to CO2 diffusion and photoassimilate translocation) may be affected by high temperatures. Proper fruit coloring is also affected by extreme temperatures; lycopene and carotenes are not synthesized at high temperatures, which precludes normal coloring in ripe fruits. Hence, heat tolerance is a major selection trait for tomato breeding programs targeting wet lowland climates in equatorial and tropical areas of the world (Giordano et al. 2005).
The PLS biplots show more specific GEI between genotypes and environments. Covariables MNT and MET are in the same direction as environments 04 (Estanzuela), 06 (Cogutepeque), 07 (San Andrés), 14 (Valle del Sábaco), 20 (San Cristobal), 27 (Palmira), and 50 (Belem), indicating these locations had relatively high minimum and mean temperatures (Table 1), which favored the marketable fruit yield of AVRDC OP heat-tolerant lines CL 5915-223 [12] and CL 5915-93 [13], located in the same direction. The reproductive processes in tomato are sensitive to high temperatures (Abdul-Baki 1991), and the number of pollen grains in heat tolerant genotypes is higher than in heat sensitive genotypes (Abdalla and Verkerk 1968; El Ahamdi and Stevens 1979; Peet and Batholemew 1996). It appears that proline accumulates in tomato leaf tissue at high temperatures, thereby causing its depletion in the reproductive tissue and seriously reducing pollen formation or viability (Kuo et al. 1986).
The amount of potassium in the soil in Cogutepeque (06) and Belém (50) was relatively high, which favored the positive GEI interaction of OP cultivar Triuque [4] in both locations. Soil organic matter (OM) content in Comayagua (11), San Antonio de Belén (15), and Centeno (53) was relatively high (Table 1); these environments are in the same direction in the biplot (Fig. 1), which favored the positive GEI of OP indeterminate cultivars Licapal 21 [3] and Angela Gigante [10] in these locations. Since the first two PLS factors do not explain all the GEI for marketable fruit yield, some distortions occurred, e.g., environment Constanza (21), which has relatively high OM content, is not in the same direction as OM in the PLS biplot.
Factorial regression and partial least squares for average fruit weight
The FR model with a stepwise regression procedure for variable selection found a subset of six independent environmental covariables (TRM, IRR, EX_P, EX_N, P, and OM) that explained 61% of the total GEI for average fruit weight (Table 5). Trimming (TRM) and irrigation (IRR) together described 29% of total GEI variability with only 28 degrees of freedom (from a total of 238 degrees of freedom). Four environmental covariables (MXT, MET, DRI, and PH) were not significant for explaining GEI of average fruit weight and thus not included in the FR analysis of variance in Table 5.
As previously mentioned, the FR with a stepwise selection procedure (employed by GENSTAT) selected six environmental covariables that explain most of the GEI. However, contrary to the PLS, the FR does not provide an overview of the general system comprising all genotypes, the environments, and the environmental covariates. The first PLS factor explained 26% of the GEI sum of squares, while the second PLS factor explained 13%. The PLS biplot of average fruit weight did not separate hybrid genotypes from OP genotypes. However, in terms of environments and their covariables, environments 05, 27, and 15 had relatively high trimming (TRM), P fertilizer (EX_P), and organic matter (OM) in the soil, as compared with the others, which tended to have higher values for the remaining covariables.
Specific trends can be visualized in Fig. 2. For example, indeterminate OP tomato genotype Licapal-21 [3] and AVRDC determinate growth selections [12, 13] had positive GEI with sites 06 and 50 (they are in the same direction) (Table 3), while OP semi-determinate genotype Catalina [1] showed positive GEI with environments 04, 43, and 53. Also, hybrid genotype BHN-39 [9] had positive average fruit weight in environments 04, 43, and 53. The average fruit weight of these genotypes in these environments is favored by relatively high MXTs during the growing cycle. However, high temperatures in tomato may reduce ripening time, thereby affecting fruit size. However, this interpretation of how temperature influences tomato fruit traits in certain environments should be taken with caution, given that fruit diameter of >48 mm was the threshold used for marketable weight in this research.
Plot of the first two partial least squares regression factors (factors 1 and 2) for average fruit weight for 15 tomato cultivars tested across 18 environments in Latin America and the Caribbean. Environment codes are given in Table 1 footnote and genotype codes are in Table 2 footnote. Environmental variables are: MXT: maximum temperature; MNT: minimum temperature; MET; mean temperature (all in °C); PRC: rainfall (mm); DAY: degree day; PH: soil pH; OM: organic matter; P: phosphorus; K: potassium; EX_N: extra nitrogen; EX_P: extra phosphorus; EX_K: extra potassium; TRM: trimming; DRI: drivings; IRR; irrigation; DHA: days to harvest
Chilean environments Chillán (42) and Curacaví (43) were irrigated (IRR) and show positive interaction with hybrid Contessa [7], which had high average fruit weight in these locations (Table 3). San Antonio de Belén (15) had the highest organic matter content, which favored the average fruit weight of OP semi-determinate cultivar Dina RP [2] and hybrids Apla [5], Narita [6], and NC EBR-2 [14]. Central American environments Baja Verapaz (05) and San Antonio de Belén (15) had the highest amounts of nitrogen (EX_N) and phosphorus (EX_P) fertilizer, which favored the average fruit weight of hybrids Luxor [8] and Apla [5], respectively. Temperature-related covariables such as MXTand MET were not important for explaining GEI of average fruit weight.
Conclusions
Factorial regression and PLS regression are useful tools for dissecting GEI of tomato METs when environments are defined by climatic and soil factors rather than by their production means. Analyses of the tomato METs included in this research show that, for marketable fruit yield, OP genotypes are favored by environments with higher temperatures during the growing cycle. On the other hand, hybrid genotypes are able to better exploit environments with higher soil pH. The GEI of tomato genotypes for fruit yield was influenced by temperature as well as days to harvest, soil pH, extra phosphorus, and amount of potassium in the soil. Concerning average fruit weight, OP and hybrid tomatoes showed similar sensitivity to environments with higher temperatures. For this trait GEI was most affected by trimming, irrigation, extra phosphorus and nitrogen, and organic matter.
The PLS biplot for marketable yield was able to cluster genotypes based on their breeding system (OP versus hybrids) along the first axis, and to further discriminate along the second axis among OP genotypes by growth habit or heat tolerance. However, the PLS biplot for average fruit weight did not separate the genotypes. The loadings of the variables included in each model can account for such a distinct result. While temperature (mean and minimum), soil PH, and length of cropping season were among the main loadings for marketable yield, cultural practices such as trimming, irrigation, or fertilizer use—the main loadings for average fruit weight—did not allow making distinctions among cultivars based on breeding system or growth habit. It seems that the average daily temperature, which was not important for explaining GEI of average fruit weight, plays an important role in tomato yields across the region, as shown by FR and PLS analyses for this trait. Nevertheless, the PLS biplots were useful for identifying adaptation patterns for both marketable fruit yield and average fruit weight of tomato genotypes included in the METs across Latin American and Caribbean locations. This could allow breeders to select such genotypes for further cultivar testing or as parental sources for local breeding programs.
It may be possible to gain more insight into tomato genetics for improving fruit weight and yield by adding molecular marker data associated with quantitative trait variation for both traits in the model for interpreting GEI. Molecular markers could further explain some of the gene × environment interaction variability and assist in breeding for low heritability traits such as fruit set under high temperature (Hanson et al. 2002). In such environments single plant selection in the F2 may not be effective; selection should be based on replicated family testing in the F3 and later generations. For example, Paterson et al. (1991) suggested that, for a low heritability trait such as soluble solids, the phenotype of F3 progeny could be predicted more accurately from the QTL genotype of the F3 parent than from the phenotype of the F2 individual. Futhermore, their results from trials in California showed that for traits with intermediate heritability (e.g., fruit pH), QTL genotype and observed phenotype were about equally effective at predicting progeny phenotype, whereas for a trait with high heritability (mass per fruit), knowing the QTL genotype of an individual added little, if any, predictive value to simply knowing the phenotype.
References
Aastveit H, Martens H (1986) Anova interactions interpreted by partial least squares regression. Biometrics 42:829–844
Abdalla AA, Verkerk K (1968) Growth, flowering and fruit set of the tomato at high temperature. Nether J Agr Sci 16:71–76
Abdul-Baki AA (1991) Tolerance of tomato cultivars and selected germplasm to heat stress. J Am Soc Horticult Sci 116:1113–1116
AVRDC (2001) AVRDC Report 2000. AVRDC, Shanhua, Taiwan
AVRDC (2003) AVRDC Report 2002. AVRDC, Shanhua, Taiwan
Berry SZ, Rafique Uddin M, Gould WA, Bisges AD, Dyer GD (1988) Stability in fruit yield, soluble solids and citric acid of eight machine-harvested processing tomato cultivars in northern Ohio. J Am Soc Horticult Sci 113:604–608
Brancourt-Hulmel M, Denis J-B, Lecomte C (2000) Determining environmental covariates which explain genotype environment interaction in winter wheat through probe genotypes and biadditive factorial regression. Theor Appl Genet 100:285–298
Brancourt-Hulmel M, Lecomte C (2003) Effect of environmental variates on genotype × environment interaction of winter wheat. Crop Sci 43:608–617
Crossa J (1990) Statistical analyses of multilocation trials. Adv Agron 44:55–85
Cuartero I, Cubero J (1982) Genotype-environment interaction in tomato. Theor Appl Genet 61:273–277
Denis J-B (1988) Two-way analysis using covariates. Statistics 19:123–132
Dinar M, Rudich J (1985) Effect of heat stress on assimilate partition in tomato. Ann Bot 56:239–249
Eberhart SA, Russell WA (1966) Stability parameters for comparing varieties. Crop Sci 6:36–40
El Ahamdi AB, Stevens MA, (1979) Reproductive responses of heat-tolerant tomatoes to high temperatures. J Am Soc Horticult Sci 104:686–691
Elkind Y, Ofra Bar-Oz Galper OB, Scott JW, Kedar N (1990) Genotype by environment interaction of tomato blossom-end scar size. Euphytica 50:91–95
Finlay KW, Wilkinson GN (1963) The analysis of adaptation in a plant breeding programme. Aust J Agricult Res 14:742–754
GENSTAT (2005) Release 8.1 (PC/Windows XP). Lawes Agricultural Trust, Rothamsted Experimental Station, UK
Giordano LB, Boiteux LS, da Silva JB, Carrijo OA (2005) Seleção de linhagens com tolerância ao calor em germoplasma de tomateiro coletado na região Norte do Brasil. Horticultura Brasileira 23:105–107
Hanson PM, Chen J, Kuo G (2002) Gene action and heritability of high-temperature fruit set in tomato line CL5915. HortScience 37:172–175
Helland IS (1988) On the structure of partial least squares. Commun Stat, Part B Simul Comput 17:581–607
Huett DO (1984) Field performance of tomato cultivars for cool-season production. Aust J Exp Agr Animal Husbandr 24:624–630
Izquierdo J, Masso CR, Villamil J (1980) Estabilidad en la producción de tomate para la industria. Investigaciones Agronómicas 1:47–51
Kuo CG, Chen HM, Ma LH (1986) Effect of high temperature on proline content in tomato floral buds and leaves. J Am Soc Horticult Sci 111:746–750
Kuo CG, Tsai CT (1984) Alternation by high temperature of auxin and gibberellin concentrations in the floral buds, flowers, and young fruit of tomato. HortScience 19:870–872
Ortiz R (1991) Una metodología de selección múltiple por productividad y estabilidad para cultivares de tomate. AgroCiencia (Chile) 7:135–142
Ortiz R, Izquierdo J (1992) Interacción genotipo por ambiente en el rendimiento comercial del tomate en América Latina y el Caribe. Turrialba 42:492–499
Ortiz R, Izquierdo J (1994) Yield stability differences among tomato genotypes grown in Latin America and the Caribbean. HortScience 29:1175–1177
Paterson AH, Damon S, Hewitt JD, Zamir D, Rabinowitch HD, Lincoln SE, Lander ES, Tanksley SD (1991) Mendelian factors underlying quantitative traits in tomato: comparison across species, generations, and environments. Genetics 127:181–197
Peet MM, Batholemew M (1996) Effect of night temperature on pollen characteristics, growth, and fruit set in tomato. J Am Soc Horticult Sci 121:514–519
Poysa VW, Garton R, Courtney WH, Metcalf JG, Muehmer J (1986) Genotype-environment interactions in processing tomatoes in Ontario. J Am Soc Horticult Sci 111:293–297
Reynolds MP, Trethowan R, Crossa J, Vargas M, Sayre KD (2004) Erratum to “Physiological factors associated with genotype by environment interaction in wheat”. Field Crop Res 85:253–274
Rudick J, Zamski E, Regev Y (1977) Genotypic variation for sensitivity to high temperature in the tomato: pollination and fruit set. Bot Gaz 138:448–452
Rylski I (1979) Fruit set and development of seeded and seedless tomato fruits under diverse regimes of temperature and pollination. J Am Soc Horticult Sci 36:195–205
SAS Institute Inc (1999) SAS/STAT®. User’s Guide. Version 8. SAS Institute Inc., Cary, North Carolina
Sasaki H, Yano T, Yamasaki A (2005) Reduction of high temperature inhibition in tomato fruit set by plant growth regulators. JARQ 39:135–138
Scholberg J, McNeal BL, Boote KJ, Jones JW, Locascio SJ, Olson SM (2000) Nitrogen stress effects on growth and nitrogen accumulation by field-grown tomato. Agron J 92:159–167
Stofella PJ, Bryan HH, Howe TH, Scott JW, Locascio SJ, Olson SM (1984) Stability differences among fresh market tomato genotypes. 1. Fruit yields. J Am Soc Horticult Sci 109:615–618
Sumeghy JB, Huett DO, McGlasson WB, Kavanagh EE, Nguyen VQ (1983) Evaluation of fresh market tomatoes of the determinate type irrigated by trickle and grown on raised beds covered with polyethylene mulch. Aust J Exp Agricult Animal Husbandr 23:325–330
Talbot M, Wheelwright AV (1989) The analysis of genotype × environment by partial least squares regression. Biuletyn Oceny Odmian, Zeszyt 21/22:19–25
UPOV (2001) Guidelines to conduct tests of distinctiveness, uniformity and stability – Tomato (Lycopersicon esculentum (L.) Karsten ex Farw.) UPOV, Geneva
Vargas M, Crossa J, Sayre K, Reynolds M, Ramirez M, Talbot M (1998) Interpreting genotype × environment interaction in wheat by partial least squares regression. Crop Sci 38:679–689
Vargas M, Crossa J, van Eeuwijk FA, Ramirez ME, Sayre K (1999) Using partial least squares, factorial regression and AMMI models for interpreting genotype × environment interaction. Crop Sci 39:955–967
Vargas M, Crossa J, van Eeuwijk FA, Sayre K, Reynolds MP (2001) Interpreting treatment × environment interaction in agronomy trials. Agron J 93:949–960
van Eeuwijk FA, Denis J-B, Kang MS (1996) Incorporating additional information on genotypes and environments in models for two-way genotype by environment tables. In: Kang S, Gauch HG (eds) Genotype-by-environment interaction. CRC Press, Boca Raton, Florida
Author information
Authors and Affiliations
Corresponding author
Rights and permissions
About this article
Cite this article
Ortiz, R., Crossa, J., Vargas, M. et al. Studying the effect of environmental variables on the genotype × environment interaction of tomato. Euphytica 153, 119–134 (2007). https://doi.org/10.1007/s10681-006-9248-7
Received:
Accepted:
Published:
Issue Date:
DOI: https://doi.org/10.1007/s10681-006-9248-7