Abstract
Arid urban green spaces provide numerous environmental benefits including carbon sequestration. This study assesses the carbon stock potential of urban tree plantations and turf grass landscapes in selected locations in Muscat, Oman. Urban trees and turf grasses were sampled via 30 × 30 m and 0.25 × 0.25 m quadrats, respectively. The carbon stocks were then determined via biomass models and a total organic carbon analyzer. In addition, the estimated stocks were quantified in monetary benefits according to US EPA approach. Following the measurement of 1768 trees, the study results showed that urban green spaces within Oman store approximately 11,100 ton/ha of CO2 eq. Also, the social benefit of the stored CO2 was estimated to be $ 621,100 (OMR 244,772). Roadside plantations revealed higher carbon sequestration potentials compared to plantations in public institutions, open spaces and public parks due to increases in roadside greenery with high biomass plantations. However, there is no statistical difference among the carbon stocks for the four land use types (p < 0.05). Turf grass carbon stocks were estimated to be 0.604 ± 0.09 kg C m−2. This is the first study in an arid urban area where comprehensive carbon stock has been conducted. Thus, sustainable urban greenery projects through planting of high biomass trees are essential as it may enhance carbon stock potentials in arid environments.
Similar content being viewed by others
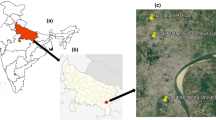
Explore related subjects
Discover the latest articles, news and stories from top researchers in related subjects.Avoid common mistakes on your manuscript.
1 Introduction
Urban vegetation is a beneficial component of urban design and provides many socioeconomic and biophysical benefits including provision of recreational services, aesthetic value and improvement of biodiversity (Pasher et al. 2014). Urban trees, shrubs and grasses also help in air quality improvement through absorption of gases, pollutants and particulate matter (with aerodynamic diameter of 2.5 and 10 micron meters), acting as wind breaks, creating shade, reducing noise and preventing runoff (Brack 2002; De Marco et al. 2018). Integrating vegetation (trees, shrubs, grasses) into urban areas in arid environments has become increasingly important in improving urban ecosystem resilience both to regulate urban microclimates and mitigate global climate change. Vegetation in urban areas may be an undervalued carbon sink through its sequestration of atmospheric CO2 via photosynthetic activity and storing excess carbon as biomass (Nowak and Crane 2002). CO2, which is the most abundant greenhouse gas (GHG), is stored within both aboveground biomass (AGB) and belowground biomass (BGB) through carbon sequestration. While the AGB refers to the stored carbon in biomass in stems, leaves or branches of vegetation, the BGB is the carbon stored in roots.
Forests (including urban forests) have received much attention in recent years for their potential roles as carbon sinks due to high carbon uptake by fast growing plants (Jo 2002; Liu and Li 2012; Nowak and Crane 2002). This natural process of capture and storage of CO2 contributes substantially climate change mitigation (Bayat et al. 2012). This process can be enhanced to reduce net emissions of atmospheric GHGs. Reducing Emissions from Deforestation and Forest Degradation (REDD+) program is widely utilized in developing countries within the purview of the United Nations Framework Convention on Climate Change’s (UNFCCC) mitigation measures to reduce carbon emissions from forest lands while achieving low carbon, sustainable growth and development (Gurung et al. 2015). Therefore, the initial actions toward implementing REDD+ at a country level requires measurement and understanding of carbon stocks dynamics. This is also necessary for the monitoring and development of future land management practices that will enhance carbon sinks (Hai et al. 2015).
Quantifying carbon sequestered by live trees requires the determination of their biomass. This may be done by harvesting trees of different sizes to determine their volume, subjecting them to different temperatures to determine their dry mass and density, and then finally estimating the biomass which is the product of density and the volume of the trees (Chave et al. 2005; Pearson et al. 2005; Sunaryathy et al. 2015). This is a very accurate method of estimating carbon stocks for individual trees, but it is destructive and cannot be used for a large number of trees of the same or different species due to their varying sizes or growth rates. Direct measurement of biomass is expensive, time-consuming and thus a difficult method of determining biomass that ultimately tends to release CO2 back to the atmosphere (Nowak et al. 2013; Yao et al. 2015).
Allometric biomass models are developed statistically by using measured diameter at breast height (DBH) as an independent variable, and estimating biomass as a dependent variable to form a regression model (Brown 1997; Wang 2006; Yoon et al. 2013). Biomass regression equations hold the advantage of reducing the destructive qualities of direct biomass measurement, as they can be used for different trees of existing historical archival DBH data and can be very useful in estimating temporal carbon stock dynamics (Nowak and Crane 2002). These advantages have led to the application of biomass models in several studies across Europe (e.g., Balderas and Lovett 2013; Grunzweig et al. 2003; Strohbach and Haase 2012), the USA (e.g., Myeong et al. 2006; Nowak et al. 2013; Phillips et al. 2016), Asia (e.g., Wang et al. 2005; Yao et al. 2015; Yoon et al. 2013) and Africa (e.g., Stoffberg et al. 2010). However, the disadvantages of biomass models are inaccuracies in measurements especially when they are used to estimate carbon stock of trees of different species (Amoatey et al. 2018). When it comes to urban vegetation, a lack of specific biomass equations for urban vegetation types and thus the dependence on biomass models derived from allometric equations derived from forest trees may pose challenges for the accurate estimation of carbon stocks in urban settings (Yoon et al. 2013).
The amount of carbon sequestered in trees depends on several factors including tree permanency (mortality rate), density and size (as proxied by DBH). Large urban trees with excellent growing and health conditions with DBH ≥ 77 cm can sequester up to 90 times the carbon of trees under similar conditions compared with trees with DBH of < 8 cm (Nowak and Crane 2002; Nowak et al. 2013; Stoffberg et al. 2010). Atmospheric carbon tends to be sequestered by young plants as they grow and accumulate biomass, whereas carbon is lost by aging trees that release carbon back to the atmosphere through respiration, tree cutting and decomposition (Hai et al. 2015; Nowak and Crane 2002). In managed urban plantations, this mixed stand of young and old trees is very common.
Additionally, urban planning has led to increase in turf grass landscaping in many urban areas. Turf grass improves environmental quality through mitigating flooding, minimizing soil erosion, reducing noise and providing recreational benefits (Selhorst and Lal 2013; Shchepeleva et al. 2016). Apart from these services, turf grass plays a substantial role in CO2 sequestration (Guertal 2012; Hamido et al. 2016; Kong et al. 2014; Ng et al. 2015; Shchepeleva et al. 2016). Although several species of turf grass exist, among the most common species used in urban landscapes are Axonopus compressus, Zoysia japonica and Cynodon dactylon (Kong et al. 2014; Odiwe et al. 2016). Most carbon stock studies in both forested and urban settings have focused mainly on trees (e.g., Escobedo et al. 2010; Gurung et al. 2015; Rahman et al. 2015; Strohbach and Haase 2012) with very limited studies on turf grass carbon stock dynamics(Penman et al. 2003). Consequently, we have limited understanding of the potential of turf grasses as carbon sinks. Another important issue with turf grass carbon stocks is the emission of other GHGs such as CH4 and N2O because of both biogenic respiration by turf grass and the application of fertilizers. In addition, CH4 and N2O have higher global warming potential than CO2, making it more challenging to use turf grasses as a CO2 sink (IPCC 2013; Weissert et al. 2016a, b). A study conducted by Kong et al. (2014) has shown that turf grass can store about 0.05–0.21 kg of carbon per every 1 m2 of turf landscape, while turf grass maintained by mowing and fertilization could also emit about 0.17–0.63 kg carbon dioxide equivalent (Ce) per 1 m2 per year to the atmosphere.
The arid nature of Muscat governorate has motivated the Government of Oman to embark on urban greening projects with the sole aim of improving environmental performances of the city. The trees and grass turfs were grown in Muscat to ensure provision of shades, promotion of urban biodiversity and to enhance aesthetic value of the city. However, the impact of these greeneries in mitigating local anthropogenic CO2 emissions through sequestrations has received less attention. This study seeks to analyze carbon stocks in urban green spaces in select locations in Muscat, Oman. This was achieved by employing measured DBH, and stem height (Hstem) data to estimate AGC and BGC stock pools of urban plantations (trees and palms) for different land use types such as parks, avenue plantations and open spaces. In addition, AGC stock of turf grass landscapes was assessed in these locations. Carbon stocks were determined through field measurements, the application of models and analytical procedures. The study will furthermore provide guidelines about urban greening and landscape projects and programs that could enhance carbon sequestration while reducing CO2 emissions. These guidelines will be useful in undertaking future large-scale carbon accounting at the city-scale, such as within Muscat, the focus of this study.
2 Materials and methods
2.1 Description of study area
The study area, the Muscat Governorate, is situated at 58° 24′ 09.20″ E and 58° 24′ 21.32″ N along the coastal Gulf of Sultanate of Oman with an altitude of 84.6 m (Fig. 1). The environmental conditions of the Muscat Governorate are characterized by arid and semiarid climates with annual precipitation ranging from 0 to 95 mm. Rainfall is very rare and only occurs in the month of December to January during winter season. The average monthly ambient temperature is 16.7 °C in January and 30.0 °C in June, while the maximum temperature could be as high as 25 °C in January and 40 °C in June (Directorate General of Meteorology 2017). The dominant species of trees and palms commonly found in roadside plantations (RP), public institutions (PI), open spaces (OS) and public parks (PP) in the Muscat Governorate include Nilotica sp. Ficus nitida, Prosopis cineraria, Azadirachta indica, F. altissima, Conocarpus lancifolius, Albizia saman, Peltophorum pterocarpum, F. religiosa, F. benghalensis, Phoenix sp and Conocarpus lancifolius. These plantations contain both native and exotic species and depend mainly on irrigation and fertilization systems as sources of water and nutrient supply. The plantations are managed exclusively by the Muscat Municipality.
2.2 Field measurements
Field data collection in Muscat was conducted from December 15, 2016 to January 30, 2017. During the field measurement, we sampled trees using 30 × 30 m sized quadrats within PPs, OSs, RPs and PIs, and a line transect procedure within RPs (at successive 100 m intervals). The DBH (at 1.3 m) for trees and palms and the basal diameter (D) (usually at 10 cm) for tree-like shrubs were determined with a DBH tape (Nero et al. 2017). To satisfy the input measurements for the biomass models used for palms, we determined Hstem of each palm in the study area with an electronic clinometer (Orozco-Aguilar et al. 2018). Since the health conditions of the trees, palms and typical shrubs were excellent, we applied a factor of 1 to the measured parameters according to Nowak and Crane (2002). Grass samples were taken from all the land use types except PI, as their turf grass management is similar to PP. For grasses (turf grass), a 0.25 m × 0.25 m subplot was randomly generated within the main plots of the line transects, and aboveground turf grass shoots were collected as described by Kong et al. (2014). The samples were collected separately in pre-labeled polyethylene bags (Diamond zipper bags polyethylene, Thailand).
2.3 Carbon stock and carbon dioxide equivalent estimations
We employed existing biomass models for estimation of biomass and biomass carbon. The measured DBH, Hstem and D data from the field survey were used to estimate the aboveground carbon (AGC) and belowground carbon (BGC) stocks with the models as shown in Table 1 (O’Donoghue and Shackleton 2013). Due to a wide diversity of planted vegetation in Muscat, all the biomass models in Table 1 were employed to estimate AGC and BGC stocks for trees, shrubs and palms using the measured DBH, D and Hstem as input parameters. We use the different biomass models to reduce the uncertainties of the estimation that would arise from using a single model for different vegetation types. The estimated biomass (kg) of the trees was converted by a factor of 0.5 to obtain the amount of carbon for each land use type (Nowak and Crane 2002). We estimate the equivalent carbon dioxide, CO2eq (tons), from the carbon stock by applying a conversion factor of 3.36 (molar ratio of carbon dioxide to carbon, 44/12) as shown in Eq. (1) (Stoffberg et al. 2010).
where Bm = biomass (kg), \(\frac{1}{2}\) = a factor of converting biomass to carbon, \(\frac{44}{{12}}\) = a conversion factor of carbon to equivalent CO2, and \(\frac{1}{1000}\) = factor of converting CO2 from kg to tons, respectively.
2.4 Laboratory analysis and turf grass carbon stock estimation
For turf grass biomass estimations, samples collected from the field were dried in an oven (Oven 300 Plus Series, UK) for about 105 °C for 48 h in order to determine the dry mass weight of (Mg) grass biomass. The sample was then cut into pieces and ground with a mixer grinder (Max-Ac300, Panasonic, Japan). The coarse powdered sample was then sieved with 106 μm mesh stainless steel (Endecotts Ltd, England) with the help of an Auto Shaker (Retsch, As 200, Germany). About 0.2 g of the sieved fine powdered grass sample was weighed with an electronic balance (Volar™ 3000, China), digested with 25% phosphoric acid and heated (about 100–120 °C) on a hot plate (Stuart Heat Stirrer, SB162, UK) for 30–35 min. The sample was then analyzed in a total organic carbon (TOC) analyzer (TOC-V, SHIMADZU, Japan) to determine the TOC concentration (Kong and Chu 2018). The instrument gives results in percentage concentration of organic carbon as determined by combustion–chemiluminescence method coupled with a chemiluminescence detector. This method involves complete oxidation of carbon present in the sample into CO2 with a copper catalyst at a temperature of 950 °C. The produced CO2 is detected with non-dispersion infrared (NDIR) analyzer (Shimadzu 2017). TOC concentration (%) obtained from each sample was used to estimate turf grass carbon stock (Tc) according to Eq. (2), where Mg (kg) is the oven dry mass of turf grass (Kong et al. 2014).
2.5 Statistical analysis
Finally, significant differences in mean total carbon stock (aboveground and belowground carbon stocks) for the four land use types were analyzed by one-way analysis of variance (ANOVA) with SPSS Statistics software (version 20).
3 Results
3.1 Arid urban vegetation analysis
Assessing species composition is essential for future planning and management of urban greenery. In this study, a total of 1768 trees (Table 2) were measured continuously during the field measurement campaign over fourth five days (45) days (December 15, 2016 to January 31, 2017). There were few differences in terms of floristic diversity for the four land use systems, but some species tended to dominate in particular land use types more than others. Azadirachta indica (85%) and Phoenix sp. (15%) were the most dominant species found in PI, while Prosopis cineraria accounts for about 90% in PP, especially in Al Qurum park, these were determined through visual observations and counting. In RP, Ficus nitida dominates in Al Azaiba and Al Sarooj road sides and accounts for about 75% of the species that were measured with the remaining percentages being Azadirachta indica, Peltophorum pterocarpum and Phoenix sp. However, OS composed of different species types with no dominance of a particular species.
3.2 DBH of measured trees
It was observed that most of the DBH classes were evenly distributed within all the four land use systems with PI and OS having most DBHs ranging from 21 to 60 cm (Fig. 2). However, DBH ranging from 81 to 120 cm was consistent among all the four land use systems, while trees of DBH > 121 cm were found mostly in RP (Fig. 2). Trees found in Al Azaiba and Al Sarooj avenues were larger and had maximum DBHs of 299 and 191 cm, respectively, with a few trees in Al Qurum Park having a DBH of approximately 200 cm (Table 2). The larger DBHs of some recorded trees were not from a single stem measurement but from the summation of individual stems within forked trees. Tree species such as F. nitida and F. religiosa found in Al Azaiba and Al Sarooj avenues had the largest DBHs followed by P. cineraria in Al Qurum park. The majority of the other species (A. indica, P. pterocarpum and Phoenix sp) abundant in all four land use systems had DBHs ranging from 20 to 60 cm (Fig. 2).
3.3 Stem height
Stem height (Hstem) was measured only for Phoenix sp. to correct underestimation or overestimation of carbon stocks, and it is a required input parameter for the biomass model for the Phoenix sp. Hstem class varies among the four land use systems, ranging from as low as 1–2.9 m to a maximum of > 10.9 m (Fig. 3). A range of 3–4.9 m was very abundant in PP (Al Sahawa Park) with a few 7.8–10.9 m individuals mostly found in OS. These distinct patterns in Hstem among the four systems may be due to different varieties among Phoenix sp, time of planting and the level of management activities.
3.4 Current carbon and CO2 equivalent stocks within the four land use types
We estimated how much carbon is currently sequestered by green spaces in Muscat Governorate based on four land management systems. Our results showed that carbon stocks vary greatly among the four land use systems in the Muscat Governorate. Carbon stocks ranged from 574 ton/ha for both the aboveground and belowground carbon in PI to a maximum of 1100 ton/ha in RP with a total CO2 equivalent amount of 11,100 ton/ha (Table 4).
Trees in the RP system store large amounts of aboveground carbon at 485 and 461 Mg/ha for Al Azaiba and Al Sarooj, respectively. These amounts are more than the aboveground carbon stored in Al Qurum (313 ton/ha) for PP, As-Seeb (237 ton/ha) for OS and SQU botanical garden (276 ton/ha) for PI (Table 3). The RP system had a small number of quadrates relative to the other land use types; therefore, the order of increasing carbon stock is RP > PP > OS > PI (Table 4). This order is in agreement with the larger but smaller number of DBH classes shown in Fig. 2.
Most urban studies have focused only on aboveground carbon stock. This study estimated belowground carbon to better understand its contribution to the total carbon pool. The overall BGC for the study was 308 ton/ha. RP (112 ton/ha) had the highest BGC compared to PP (72 ton/ha), OS (65 ton/ha) and PI (58 ton/ha) landscapes (Table 4). There were consistent trends in BGC among all the sampling locations, except Al Khould (6.6 ton/ha) and Al Sahawa (0.75 ton/ha) where BGC was very low. However, the highest BGC stocks (52 ton/ha) were recorded in Al Zaiba and Al Sarooj roads (Table 3).
3.5 Turf grass carbon stock potential
The turf grass carbon stock of the six locations in Muscat Governorate on three lands use systems (PP, OS and RP) is shown in Table 5. The main urban carbon sinks are trees, soils, turf grass and shrubs, yet most urban carbon stock and sequestration studies have concentrated mainly on trees and soils with very limited knowledge on carbon stocks of urban turf grasses which are also the main components of the urban landscape. Thus, the results of the study will shed light about the carbon sequestration potential of arid turf landscapes in urban environment.
The minimum and maximum organic carbon concentrations determined in the turf grasses in the study were 7.04–20.02%, respectively, with an average concentration of 10.65 ± 0.88% in Al Qurum Park and 14.57 ± 0.89% in Burj Al Sahawa (Table 5). However, there were similarities in the AGC stock of the turf grass in all the sampling sites except Al Sahawa Park which showed high carbon stocks (Table 5).
4 Discussion
The most common and dominant native species identified in this study included Prosopis cineraria, Azadirachta indica and Phoenix sp. These species may be important for sustenance of urban carbon stocks due to their ability to adapt to the local climate due to climate change. As reported by Lewis et al. (2016), urban carbon stocks can be further supported by native species, but factors such as growth rate, species types and management activities (pruning, litter removals, irrigation and fertilization) also play a critical role in enhancing carbon stock and sequestration.
The most important factor that affects aboveground carbon stock is the volume of the trees as proxied by their DBH (Nowak et al. 2013). According to Nowak et al. (2013) and Pearson et al. (2005), carbon sequestration and storage in urban areas can increase depending on the size of the DBH; thus, healthy trees of DBH > 77 cm can sequester and store about 90 times the aboveground carbon of similar trees with DBH < 8 cm. In general, trees in the Muscat Governorate had larger DBHs ranging from 61 to 299 cm out of the total measured DBH. This may be due to excellent management practices within the municipality and the presence of both fast growing and mature trees. Comparisons of DBH classes from other studies with those of this study have shown that the current study recorded maximum DBH classes for many of the tree species (Table 6).
Most of the large DBHs recorded under this study were not attributed to a single stem measurement but rather trees with several forked stems (ranging from 2 to 11) per tree. Thus, trees in Muscat may have higher biomass due to large mature trees with a large number of forked stems (Amoatey et al. 2018).
Considering tree height, which is an important parameter in carbon sequestration, the study did not investigate the individual date palm species, as our ultimate aim was to estimate the total carbon stock of all Phoenix sp. Dey et al. (2014) reported Hstem classes of Phoenix sp. to be 8.93–10 m and attributed the low heights to a lack of adequate soil nutrient for the palms. Similarly, Da Silva et al. (2015) measured Hstem of Euterpe precatoria ranging from 7.3–21.3 m (n = 56) in Amazon forest, Brazil. Goodman et al. (2013) similarly recorded n = 136 palms with Hstem ranging from 1.5–30.5 m in the Amazon. Most of the palms with high Hstem from the literature may not be suitable to compare with this study (Fig. 3) due to the fact that most palms in the literature are (1) growing in natural forests where there is adequate supply of nutrients and precipitation, and (2) of different species compared to Phoenix sp.
As reported by Nowak and Crane (2002), the carbon stocks of a particular area depend primarily on tree size and density, and thus, the amount of carbon increases with increase in total tree size and number of tree cover of a given standing basal area. This implies that trees with larger DBH may store about 1000 times more carbon than trees of smaller DBHs (Stoffberg et al. 2010). Thus, urban planning should consider planting high biomass trees to ensure maximum removal of anthropogenic CO2 (Yao et al. 2015).
To the best of the author’s knowledge, no previous study of a similar type has been conducted in four urban land use types (PP, OS, RP and PI) in an arid setting. Most studies estimating urban carbon stocks range from a few tens to a few millions of tons of stored carbon. Our estimates of 3000 ton/ha (Table 4) of carbon were higher than the amount reported by Lavista et al. (2016) in urban institutional vegetation in Darmaga, Indonesia, of 27.36 ton/ha and Dey et al. (2014) in Sylhet city with an estimation of 20.28/ha tons, Bangladesh. However, the total current estimates (3025.12 ton/ha) were lower compared to the amount reported by Strohbach and Haase (2012) of a selected urban forestry in Leipzig, Germany, of 316,000 ton/ha. However, these estimates are similar to the amount reported by Habtamu (2013) in nine urban parks in Addis Ababa, Ethiopia, with 5038 measured trees amounting to a total 3273 ton/ha of stored carbon compared to our estimates of 1768 trees.
The differences in above estimates may be due to variations in terms of geographical climatic conditions, age, DBH classes and tree density. The amount of carbon stock reported by Habtamu (2013) in tropical natural urban vegetation seems to be closer to this estimates, although they recorded a large number of trees relative to the current study. This implies that the amount of carbon stock within an urban green space of a particular tree species may be higher than that of a natural urban forest of a particular quadrate size. The trees under this study were old, large and fast growing with an average amount of 9.25 kg C/ tree compared to 5.3 kg C/tree in natural forest/urban forest. Regarding the age of urban trees, Tripathi et al. (2015) estimated only 444.31 tons of aboveground carbon from 2688 trees of a young urban forest in Delhi, India, relative to our estimates of 2717.05 ton/ha (Table 4) with 1768 trees. This has revealed that tree age may play an important role in carbon sequestration. Thus, the selected locations of the Muscat Governorate could currently store the total carbon stock of 11,102.19, CO2 eq ton/ha.
According to the US EPA (2017), the social benefit of carbon sequestration is $56 (22 OMR)/Mg of CO2. This implies that the estimates of 11,102.19, CO2 eq ton/ha storage of CO2 for the selected urban vegetation in Muscat could prevent damages valued at $621,722 (OMR 244,772). According to the land use types in Table 4, there are no statistical differences among the means of carbon stored at 95% confidence intervals (95% CI). This shows that there are no significant differences in carbon stocks in the four main land use types in Muscat governorate as the landscapes in the Muscat received similar urban greening managements. However, the current carbon storage trends of the four land use types might change based on major greening (thus, enhancing carbon sequestration) and infrastructure (i.e., releasing CO2 to atmosphere through removal of vegetation) projects that might occur in the future.
Turf grasses are important sinks for carbon in urban landscapes. The AGC carbon stocks measured in this study ranged from 0.239 ± 04 to 6.04 ± 0.09 kg C m−2 and were higher than the reported amount of 0.05–0.21 kg C m−2 by Kong et al. (2014) and 0.08–0.34 kg C m−2 by Shchepeleva et al. (2017). These great differences could be due to factors such as maturity and management practices of the turf grass. In the present study, turf grasses grown in the study locations received adequate irrigation, fertilization, pesticides applications and regular mowing. These management activities may lead to high growth rates of the turf grass thereby leading to high biomass. These factors may help explain why there we found higher turf grass carbon stocks in our study compared to Kong et al. (2014) and Shchepeleva et al. (2017), where most of the turf grasses receives minimal irrigation and fertilization as they depend mostly on natural precipitation and nutrients.
The turf grasses grown in Muscat certainly require the above mentioned management practices to grow due to unfavorable prevailing climatic factors. In this case, any factor that may limit the growth of turf grasses may lead to the intensification of management practices. However, efficient management practices should promote growth of turf grass cover and subsequently lead to high carbon stocks (Pouyat et al. 2009). Most of the urban soil and turf grass carbon stock studies found within the literature may have limited management practices due to their high decomposition rates of organic debris from the turf grasses and other plants. Moreover, the locations of this study may have regular irrigation with high soil moisture and nutrient supplies, thereby leading to an overall accumulation of carbon stock (Perie and Ouimet 2008). The AGC carbon stock for turf grasses may be affected by several factors, such as the intensity of management activities, organic carbon concentration, species composition and the ages of the turf grass. Here, there were some slight variations in the AGC stock of turf grass for all six locations. (Table 5).
Despite wide ranges of turf grass species in urban landscapes, the knowledge on carbon stock potential of the individual species presented here may contribute to carbon stock enhancement (Selhorst and Lal 2013). Turf grass may comprise of diverse grass species, which in turn may differ in their carbon stock potentials due to differences in growth pattern (e.g., spreading and prostate growth, growth rate and physiology) (Guertal 2012). We identified the three most abundant species of turf grasses in Muscat including Axonopus compressus, Paspalum vaginatum, Zoysia japonica and two other weed species, (Sesuvium portulacastrum and Wedelia sp) (Table 7) in this study.
The current results showed a range in AGC stock of the turf grass species from 0.24 ± 0.04 to 0.64 ± 0.07 kg C m−2 for the five species. The average amount of carbon stored by Axonopus compressus of 0.24 ± 0.04 kg C m−2 was significantly different from the estimated average amount of the same species reported by Kong et al. (2014) of 0.39 ± 0.04 kg C m−2 but similar to the value reported by Odiwe et al. (2016) of 0.32 ± 0.07 kg C m−2. However, the estimated amount of carbon stored by Zoysia japonica of 0.42 ± 0.09 kg C m−2 was greater than the amount determined by Kong et al. (2014) of the same species. These variations in carbon stocks among the turf grasses could be attributed to factors such as age, photosynthetic activities and the intensity of management practices involved. In the case of this study, the carbon storage of turf grass species may be enhanced by reduced mowing and litter removals. These will prevent excessive loss of AGB and improved soil organic nutrient supply through the decay of litter and could reduce excessive emissions of CO2 back to the environment from mowing activities.
Finally, the study has several limitations and uncertainties of carbon stocks estimates which were due to small sample size of the various locations. Most studies employ species-specific models for carbon stock estimations which were unavailable for this study as most of the species surveyed lack readily developed biomass models. Given that the study was unable to use species-specific models, we thus used generalized biomass models. This could lead to under- or over-estimations of the carbon stocks. Also, the study could not report the total turf grass carbon stocks but rather focused on small areas (0.25 m × 0.25 m). Therefore, future works need to employ satellites images and geographical information systems approach to better understand the carbon stock for the entire Muscat Governorate compared to the selected locations focused upon in this study.
5 Conclusion
Reducing the impact of climate change through urban greenery expansion may mitigate both local and global CO2 levels. Urban greenery’s natural process of capture and storage of CO2 has potential to contribute to climate change mitigation. Grasses are also potential sinks of carbon within urban landscapes that can sequester atmospheric CO2 within biomass.
The results indicated that AGC and BGC storage measured from 1768 trees of 11 selected locations based on field measurement within the four land use systems were 3000 ton/ha with an equivalent CO2 storage of 11,100 ton/ha. The maximum turf grass carbon stocks were estimated be 0.604 ± 0.09 kg C m−2. Planting of large growing tree species can enhance climate change mitigation in urban areas as they can sequester approximately 90 times the anthropogenic CO2 of smaller trees such as shrubs. Turf grass management activities should be minimized and conducted in an environment-friendly manner since intensive management practices can release considerable CO2 back into the urban environment. In comparison with previous studies, only one study in Al Foah area in United Arab Emirates with urban greenery and climate type similar to that of Muscat (Oman) has been conducted so far (Issa et al. 2019). The carbon stock estimates of that study were bias as it was focused only on AGC stocks specifically for palms. The carbon stock assessment under this was comprehensive as it encompasses different arid urban plant species, palms and turf grasses. In this current study, the amount of data generated from carbon stock assessments have been scoped to not only to provide a substantial body of knowledge but as a substrate for further global analytic work for future studies especially in arid urban areas.
Also, the results of this study may help persuade the government of Oman to maintain vegetation cover within the Muscat city and also to expand urban greenery projects for the sustainable city planning across the entire country. Currently, the government spends extensive resources for planting and maintaining vegetation in the Muscat Governorate, with the purpose of providing beautification and recreation in the city. However, the indirect environmental services of the vegetation in the city such as carbon sequestration have not been contemplated and studied. The study at large will gain wider attention to readers as it presents new findings on carbon storage potential of an arid urban area having hardly any precipitation and no natural vegetation, but managed plantations in contrast to the other tropical and temperate areas of the world.
References
Amoatey, P., Sulaiman, H., Kwarteng, A., & Al-Reasi, H. A. (2018). Above-ground carbon dynamics in different arid urban green spaces. Environmental Earth Sciences,77(12), 1–10.
Balderas, T. A., & Lovett, J. C. (2013). Using basal area to estimate aboveground carbon stocks in forests: La Primavera Biosphere’s Reserve Mexico. Forestry,86(2), 267–281.
Bayat, A. T., van Gils, H., & Weir, M. (2012). Carbon stock of European beech forest: A case at M. Pizzalto, Italy. APCBEE Procedia,1, 159–168.
Brack, C. L. (2002). Pollution mitigation and carbon sequestration by an urban forest. Environmental Pollution,116, S195–S200.
Brown, S. (1997). Estimating biomass and biomass change of tropical forests: A primer. FAO forestry paper-134.
Chave, J., Andalo, C., Brown, S., Cairns, M. A., Chambers, J. Q., Eamus, D., et al. (2005). Tree allometry and improved estimation of carbon stocks and balance in tropical forests. Oecologia,145(1), 87–99.
Da Silva, F., Suwa, R., Kajimoto, T., Ishizuka, M., Higuchi, N., & Kunert, N. (2015). Allometric equations for estimating biomass of Euterpe precatoria, the most abundant palm species in the Amazon. Forests,6(2), 450–463.
De Marco, A., Amoatey, P., Khaniabadi, Y. O., Sicard, P., & Hopke, P. K. (2018). Mortality and morbidity for cardiopulmonary diseases attributed to PM2.5 exposure in the metropolis of Rome, Italy. European Journal of Internal Medicine,57, 49–57.
Dey, A., Islam, M., & Masum, K. M. (2014). Above ground carbon stock through palm tree in the Homegarden of Sylhet city in Bangladesh. Journal of Forest and Environmental Science,30(3), 293–300.
Directorate General of Meteorology. (2017). Directorate General of Meteorology. https://www.met.gov.om/opencms/export/sites/default/dgman/en/home, Retrieved April 7, 2017.
Escobedo, F., Varela, S., Zhao, M., Wagner, J. E., & Zipperer, W. (2010). Analyzing the efficacy of subtropical urban forests in offsetting carbon emissions from cities. Environmental Science and Policy,13(5), 362–372.
Goodman, R. C., Phillips, O. L., del Castillo, T. D., Freitas, L., Cortese, S. T., Monteagudo, A., et al. (2013). Amazon palm biomass and allometry. Forest Ecology and Management,310, 994–1004.
Grunzweig, J. M., Lin, T., Rotenberg, E., Schwartz, A., & Yakir, D. (2003). Carbon sequestration in arid-land forest. Global Change Biology,9(5), 791–799.
Guertal, E. A. (2012). Carbon sequestration in turfed landscapes: A Review. In R. Lal & B. Augustin (Eds.), Carbon sequestration in urban ecosystems (pp. 197–213). Dordrecht: Springer.
Gurung, M. B., Bigsby, H., Cullen, R., & Manandhar, U. (2015). Estimation of carbon stock under different management regimes of tropical forest in the Terai Arc Landscape, Nepal. Forest Ecology and Management,356, 144–152.
Habtamu, M. (2013). Carbon stock estimation of urban forests in selected public city parks in Addis Ababa. Ethiopia: Addis Ababa University.
Hai, V. D., Do, T. V., Trieu, D. T., Sato, T., & Kozan, O. (2015). Carbon stocks in tropical evergreen broadleaf forests in Central Highland, Vietnam. International Forestry Review,17(1), 20–29.
Hamido, S. A., Guertal, E. A., & Wesley, W. C. (2016). Carbon sequestration under warm season turf grasses in home lawns. Journal of Geoscience and Environment Protection,04(09), 53–63.
IPCC. (2013). Climate Change 2013: The physical science basis. Contribution of working group I to the fifth assessment report of the Intergovernmental Panel on Climate Change (p. 1535). Cambridge University Press, Cambridge, New York, NY: Intergovernmental Panel on Climate Change.
Issa, S., Dahy, B., Saleous, N., & Ksiksi, T. (2019). Carbon stock assessment of date palm using remote sensing coupled with field-based measurements in Abu Dhabi (United Arab Emirates). International Journal of Remote Sensing,40, 7561–7580.
Jo, H. (2002). Impacts of urban greenspace on offsetting carbon emissions for middle Korea. Journal of Environmental Management,64(2), 115–126.
Kong, L., & Chu, L. M. (2018). Subtropical urban turfs: Carbon and nitrogen pools and the role of enzyme activity. Journal of Environmental Sciences,65, 18–28.
Kong, L., Shi, Z., & Chu, L. M. (2014). Carbon emission and sequestration of urban Turfgrass systems in Hong Kong. Science of The Total Environment,473–474, 132–138.
Lavista, L., Prasetyo, L. B., & Hermawan, R. (2016). Dynamics change of the above carbon stocks in Bogor Agricultural University, Darmaga campus. Procedia Environmental Sciences,33, 305–316.
Lewis, T., Smith, T. E., Hogg, B., Swift, S., Verstraten, L., Bryant, P., et al. (2016). Conversion of sub-tropical native vegetation to introduced conifer forest: Impacts on below-ground and above-ground carbon pools. Forest Ecology and Management,370, 65–75.
Liu, C., & Li, X. (2012). Carbon storage and sequestration by urban forests in Shenyang, China. Urban Forestry and Urban Greening,11(2), 121–128.
McPherson, E. G., Xiao, Q., & Aguaron, E. (2013). A new approach to quantify and map carbon stored, sequestered and emissions avoided by urban forests. Landscape and Urban Planning,120, 70–84.
Myeong, S., Nowak, D. J., & Duggin, M. J. (2006). A temporal analysis of urban forest carbon storage using remote sensing. Remote Sensing of Environment,101(2), 277–282.
Nero, B. F., Callo-Concha, D., Anning, A., & Denich, M. (2017). Urban Green spaces enhance climate change mitigation in cities of the global south: The case of Kumasi, Ghana. Procedia Engineering,198, 69–83.
Ng, B. J. L., Hutyra, L. R., Nguyen, H., Cobb, A. R., Kai, F. M., Harvey, C., et al. (2015). Carbon fluxes from an urban tropical grassland. Environmental Pollution,203, 227–234.
Nowak, D. J., & Crane, D. E. (2002). Carbon storage and sequestration by urban trees in the USA. Environmental Pollution,116, 381–389.
Nowak, D. J., Greenfield, E. J., Hoehn, R. E., & Lapoint, E. (2013). Carbon storage and sequestration by trees in urban and community areas of the United States. Environmental Pollution,178, 229–236.
O’Donoghue, A., & Shackleton, C. M. (2013). Current and potential carbon stocks of trees in urban parking lots in towns of the Eastern Cape, South Africa. Urban Forestry and Urban Greening,12, 443–449.
Odiwe, A. I., Olanrewaju, G. O., & Raimi, I. O. (2016). Carbon sequestration in selected grass species in a tropical lowland rainforest at Obafemi Awolowo University, Ile-Ife, Nigeria. Journal of Tropical Biology and Conservation (JTBC), 13, 1–12.
Orozco-Aguilar, L., Johnstone, D., Livesley, S. J., & Brack, C. (2018). The overlooked carbon loss due to decayed wood in urban trees. Urban Forestry and Urban Greening,29, 142–153.
Pasher, J., McGovern, M., Khoury, M., & Duffe, J. (2014). Assessing carbon storage and sequestration by Canada’s urban forests using high resolution earth observation data. Urban Forestry and Urban Greening,13(3), 484–494.
Pearson, T., Walker, S., & Brown, S. (2005). Sourcebook for land use, land-use change and forestry projects (pp. 1–64). Washington, DC: BioCarbon Fund and Winrock International.
Penman, J., Gytarsky, M., Hiraishi, T., Krug, T., Kruger, D., Pipatti, R., et al. (2003). Good practice guidance for land use, land-use change and forestry (pp. 1–590). Intergovernmental Panel on Climate Change, National Greenhouse Gas Inventories Programme (IPCC-NGGIP).
Perie, C., & Ouimet, R. (2008). Organic carbon, organic matter and bulk density relationships in boreal forest soils. Canadian Journal of Soil Science,88(3), 315–325.
Phillips, J., Duque, Á., Scott, C., Wayson, C., Galindo, G., Cabrera, E., et al. (2016). Live aboveground carbon stocks in natural forests of Colombia. Forest Ecology and Management,374, 119–128.
Pouyat, R. V., Yesilonis, I. D., & Golubiewski, N. E. (2009). A comparison of soil organic carbon stocks between residential turf grass and native soil. Urban Ecosystems,12(1), 45–62.
Rahman, M. M., Kabir, M. E., Akon, J. U., & Ando, A. S. M. (2015). High carbon stocks in roadside plantations under participatory management in Bangladesh. Global Ecology and Conservation,3, 412–423.
Selhorst, A., & Lal, R. (2013). Net carbon sequestration potential and emissions in home lawn turf grasses of the United States. Environmental Management,51(1), 198–208.
Shchepeleva, A. S., Vasenev, V. I., Mazirov, I. M., Vasenev, I. I., Prokhorov, I. S., & Gosse, D. D. (2016). Changes of soil organic carbon stocks and CO2 emissions at the early stages of urban turf grasses’ development. Urban Ecosystems,20, 309–321.
Shchepeleva, A. S., Vasenev, V. I., Mazirov, I. M., Vasenev, I. I., Prokhorov, I. S., & Gosse, D. D. (2017). Changes of soil organic carbon stocks and CO2 emissions at the early stages of urban turf grasses’ development. Urban Ecosystems,20(2), 309–321.
Shimadzu. (2017). TOC analyzers : Shimadzu scientific instruments. https://www.ssi.shimadzu.com/products/productgroup.cfm?subcatlink=tocanalyzers Retrieved April 7, 2017
Stoffberg, G. H., van Rooyen, M. W., van der Linde, M. J., & Groeneveld, H. T. (2010). Carbon sequestration estimates of indigenous street trees in the City of Tshwane, South Africa. Urban Forestry and Urban Greening,9(1), 9–14.
Strohbach, M. W., & Haase, D. (2012). Above-ground carbon storage by urban trees in Leipzig, Germany: Analysis of patterns in a European city. Landscape and Urban Planning,104(1), 95–104.
Sunaryathy, P. I., Kanniah, K. D., & Tan, K. P. (2015). Estimating aboveground biomass of oil palm trees by using the destructive method. World Journal of Agricultural Research,3(1), 17–19.
Tripathi, M., Joshi, H., et al. (2015). Carbon flow in Delhi urban forest ecosystems. Annals of Biological,6(8), 13–17.
US EPA. (2017). The Social cost of carbon|climate change|US EPA. https://www.epa.gov/climatechange/social-cost-carbon, Retrieved March 16, 2017
Wang, C. (2006). Biomass allometric equations for 10 co-occurring tree species in Chinese temperate forest. Forest Ecology and Management,222, 9–16.
Wang, Q., Adiku, S., Tenhunen, J., & Granier, A. (2005). On the relationship of NDVI with leaf area index in a deciduous forest site. Remote Sensing of Environment,94(2), 244–255.
Weissert, L. F., Salmond, J. A., & Schwendenmann, L. (2016a). Variability of soil organic carbon stocks and soil CO2 efflux across urban land use and soil cover types. Geoderma,271, 80–90.
Weissert, L. F., Salmond, J. A., Turnbull, J. C., & Schwendenmann, L. (2016b). Temporal variability in the sources and fluxes of CO2 in a residential area in an evergreen subtropical city. Atmospheric Environment,143, 164–176.
Yao, Z., Liu, J., Zhao, X., Long, D., & Wang, L. (2015). Spatial dynamics of aboveground carbon stock in urban green space: a case study of Xi’an, China. Journal of Arid Land,7(3), 350–360.
Yoon, T. K., Park, C.-W., Lee, S. J., Ko, S., Kim, K. N., Son, Y., et al. (2013). Allometric equations for estimating the aboveground volume of five common urban street tree species in Daegu, Korea. Urban Forestry and Urban Greening, 12(3), 344–434.
Acknowledgements
The authors are thankful to the Department of Soil, Water and Agricultural Engineering at Sultan Qaboos University for providing laboratory space for our analysis.
Funding
The authors also wish to express gratitude to the Department of Biology at Sultan Qaboos University for financial support through scholarship.
Author information
Authors and Affiliations
Corresponding author
Additional information
Publisher's Note
Springer Nature remains neutral with regard to jurisdictional claims in published maps and institutional affiliations.
Rights and permissions
About this article
Cite this article
Amoatey, P., Sulaiman, H. Quantifying carbon storage potential of urban plantations and landscapes in Muscat, Oman. Environ Dev Sustain 22, 7969–7984 (2020). https://doi.org/10.1007/s10668-019-00556-5
Received:
Accepted:
Published:
Issue Date:
DOI: https://doi.org/10.1007/s10668-019-00556-5