Abstract
Groundwater and surface water qualities are evaluated using geographic information system (GIS)-based geo-statistical and multivariable statistical methods. This research aims to investigate the water quality of Kızılırmak River, that remain within the provincial boundaries of Sivas, using geo-statistical and multivariable statistical methods, and to provide the water quality map of Kızılırmak River. In this regard, surface water samples from 28 surface water quality monitoring stations were analysed for wet and dry seasons. The hydro-chemical properties of surface water quality were determined, and the water quality index was evaluated for each station. Spherical, exponential and Gaussian models were determined as the best semi-variogram models according to the minimum root mean square error values and the cross-validation method. The final water surface quality map of Kızılırmak River was obtained by weighted superposition of the spatial distribution maps of the surface water quality parameters which were obtained by the geo-statistical method. The correlations between the surface water quality parameters were determined using multivariable statistical analysis methods such as correlation analysis and factor analysis (principal component analysis). The surface water quality in the study area was categorized as excellent, good, poor and very poor. The water quality of Kızılırmak River’s portion near Sivas city centre and in the South of the province did not meet the standards for drinking water purposes. This research provides the surface water quality map of the study area by use of GIS-based statistical methods.
Similar content being viewed by others
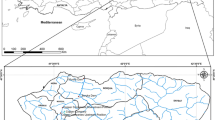
Explore related subjects
Discover the latest articles, news and stories from top researchers in related subjects.Avoid common mistakes on your manuscript.
1 Introduction
Rivers are important water resources that make a considerable contribution to the economic growth of a country and serve several purposes such as recreation, sports, fishing, irrigation, power generation and transportation (Mohamed et al. 2015). Rivers are highly susceptible to pollution as they incorporate several kinds of waste stemming from municipalities, industries and agricultural lands (Li et al. 2009). Agricultural pollution is expressed by the increase in the number of algae, which causes eutrophication at high nutrient levels and which cause serious damage to freshwater ecosystems; industrial pollution is indicated by biochemical oxygen demand (Leong et al. 2018). Considering the ever-increasing population, urbanization and industrialization, rivers have become indispensable disposal fields for various municipal and industrial wastes (Kumar et al. 2015) which results in the inevitable degradation of surface water quality. Surface water quality is under a persistent risk depending on the surface currents and anthropogenic discharges in the river basin (Liu et al. 2011). Prevention and control of river pollution hold particular importance as these are the main water resources for drinking, irrigation and industrial purposes in numerous areas throughout the world. In addition to the global issue of climate change-induced declining water resources, there is an ever-increasing urban water demand in most areas of the world (Haque et al. 2014).
The surface flow caused by rainwater creates mixing zones between land and water surfaces, and therefore faecal contamination, nutrients (N and P), basic ions and suspended solids cause pollution in a river (Howell 2018). As a means to prevent and control the declining water quality in rivers, reliable data as to the water quality should be gathered, changes in water quality should be analysed in a spatial scale, and water quality evaluation should be conducted for pollution monitoring and resource management (Wu et al. 2018). WQI is widely used in the evaluation of rivers’ water quality, and it has gained increasing importance in the management of water resources (Sutadian et al. 2016). The most effective way of defining water quality is to define the suitability of water resources for human consumption in terms of WQI. WQI allows the public to gain in-depth information as to the quality of water and enables modification of the policies formulated by various environmental monitoring agencies (Bharti and Katyal 2011; Akoteyon et al. 2011). WQI was initially developed by Horton (1965) in the USA using the most commonly used ten water quality variables involving dissolved oxygen (DO) pH, coliforms, specific conductivity, alkalinity and chloride. It has been commonly adopted and applied by European, African and Asian countries. In 1970, Brown’s group (Brown et al. 1970) developed a WQI which was similar to that of Horton (1965) and based on individual parameter weights (Tyagi et al. 2013).
Multivariable statistical analyses and geo-statistical techniques have been widely used to evaluate and characterize surface water quality (Belkhiri and Narany 2015). Multivariable statistical techniques are effective tools for evaluating human effects on water quality. Multivariable statistical techniques such as principal component analysis and factor analysis enable in-depth characterization of surface water through the interpretation of complex data matrices. These methods provide quick solutions for reliable management of water resources and pollution issues (Liu et al. 2011; Singh et al. 2004). Evaluation of water quality requires statistical analysis of multiple variables and the determination of the spatial distribution of contamination levels related to these variables. GIS and geo-statistical methods are powerful tools for spatial analysis (Yan et al. 2015; Vairavamoorthy et al. 2007) and estimation of the contaminant concentrations in locations that lack measurement data (Gharbia et al. 2016; Ella et al. 2001). The geo-statistical approach and the kriging methods hold various advantages regarding spatial correlation and the reliability of estimations (Büttner et al. 1998; Gharbia et al. 2016). In the geo-statistical analysis, if data exhibit a normal distribution, kriging technique is more likely to yield favourable results (Wu et al. 2011; Teikeu et al. 2016).
Surface water quality was evaluated using geo-statistical and multivariable statistical analysis methods in various studies. Zhao and Cui (2009) used multivariable statistical techniques such as clustering and factor analysis to analyse the source quality of River Luan (China). Wang et al. (2013) also used multivariable statistical techniques such as clustering analysis, principal component analysis and factor analysis to determine the temporal changes in the surface water quality of River Songuya near Harbin in 2005–2009 period using 15 water quality parameters. Marko et al. (2014) reported the spatial distribution of groundwater quality and estimated the chemical parameters of groundwaters using geo-statistical analysis method. Islam et al. (2018), Ben-Jemaa et al. (1994) and Teikeu et al. (2016) carried out analyses on groundwaters using geo-statistical and multivariable statistical techniques.
In this regard, the aim of the present research is to (1) evaluate the surface water quality of Kızılırmak River, and (2) provide the surface water quality mapping of Kızılırmak River within the provincial boundaries of Sivas, using geo-statistical and multivariable statistical methods.
2 Materials and methods
The basis of the method used in this study is the evaluation of surface water quality parameters through geo-statistical and multivariate statistical approaches. The flow chart of the method used in this study is shown in Fig. 1. Information about the method is explained in the following sections.
The analysis results (monthly average) of 28 surface water quality parameters [BOI, Ca, Cl, DO, Fe, K, HCO3, Mg, Mn, Na, NH4, NO2, NO3, pH, SO4, total dissolved solids (TDS), total hardness (TH), total phosphorus (TP)] of Kızılırmak River were obtained from the General Directorate of State Hydraulic Works (Ankara/Turkey) for the dry and wet seasons of the year 2015. A database representing the surface water quality was established in a GIS environment for the analyses, mapping procedures and evaluations that would be conducted in the further stages of the research.
The parameters for surface water quality were evaluated in accordance with Piper diagram and drinking water quality standards. WQI was calculated for all sampling stations, and the resulting WQI values were categorized as “excellent”, “good”, “poor” and “very poor”. Spatial distribution maps of the surface water quality parameters and WQI were prepared using the geo-statistical analysis module of GIS. Weighted-overlapping of the spatial distribution maps of surface water quality parameters was conducted to obtain the final surface water quality map of Kızılırmak River. Optimum semi-variogram models were determined for all parameters via geo-statistical analysis which was followed by statistical evaluations for the water quality parameters. In addition, the water quality of Kızılırmak River was evaluated using multivariable statistical methods such as correlation and factor analyses. During the research, AquaChem 2014.2 was used for the preparation of Piper diagram, ArcGIS 10.2 was used for the preparation of all geo-statistical analysis-based spatial distribution maps, and SPSS Statistics 22 was used for multivariable statistical analysis applications.
2.1 Study area
The study area consists of Kızılırmak, its tributaries and Kızılırmak River basin that remain within the provincial boundaries of Sivas (Fig. 2 ). The annual average discharge of Kızılırmak River is 39.42 m3/s. Kızılırmak is the longest river of Turkey that originates and empties into the sea inside its boundaries. It has a length of 1.151 km and discharges the waters of an area of 82.181 km2 into the Black Sea. Kızılırmak originates from the vicinity of Sivas-İmranlı, passes through the provincial lands of Kayseri, Nevşehir, Aksaray, Kırşehir, Ankara, Kırıkkale, Çankırı, Çorum, Sinop and Samsun and empties into the Black Sea from Bafta Plain. Kızılırmak Basin is largely located in the east of Central Anatolia region between 37°56′ and 41°44′ north latitudes and 32°48′–38°24′ east longitudes. A large proportion of Kızılırmak Basin consists of plains. The river is named after the coloured appearance of the clayey sediment at the basis of the riverbed in Kızıldağ region of Sivas province. The river’s water regime reaches its highest in April and drops down to the lowest level in July and February (Yüce and Ercan 2015).
2.2 Geology
Sivas Basin lays between the east of Erzincan and Kayseri in NE–SW direction with 250 km length and 50 km width. It is a sedimentary basin which incorporates metamorphic, magmatic and ultramafic rock formations. These sedimentary formations mainly consist of clastic, evaporite and carbonate rock formations which deposited at different geologic periods. Particularly, Hafik formations among evaporitic rock formations include industrial raw materials such as barites, halites (salt) and celestine. These units are widely exposed particularly among İmranlı, Zara and Hafik regions and pose serious agricultural and engineering problems due to their chemical composition. The basin also involves metallic mineral deposits such as chromium, iron and manganese (Poisson et al. 1996).
2.3 WQI calculation
WQI is defined as an evaluation method that shows the effect of water quality parameters on the general quality of water. Water quality and its suitability for drinking purposes can be examined through the determination of quality index. The drinking water standards that are recommended by the WHO are compiled in the evaluation of WQI (Sadat-Noori et al. 2014). A WQI evaluation aims to transform complex water quality data into a more understandable form. WQI is thereby regarded as a highly simple, efficient and helpful indicator of WQI water quality which is based on some specific parameters (Khwakaram et al. 2012).
This index is evaluated in five stages (Sadat-Noori et al. 2014; Hadithi 2012; Ambiga and Durai 2013):
First stage Assignment of a weighted value (wi) to each parameter for drinking water purposes depending on the relative importance of the parameter in terms of water quality. (The value 5 is assigned to the parameter with the highest relative importance, and the value 1 is assigned to the one with the lowest relative importance. For instance, 5 is assigned to NO3 parameter as it has the highest relative importance among others.)
Second stage Evaluation of relative weight by use of a weighted arithmetic index.
where Wi = relative weight, wi = the weight of each parameter, n = the number of parameters. Wi values calculated for each parameter are shown in Table 2.
Third stage Evaluation of quality rating scale (Qi) through a division of all concentration values by the drinking water standard concentration values specified by WHO (2017) for each of the chemical parameters in each water sample.
where Qi = quality rating scale, Ci = concentration of each chemical parameter in each water sample (mg/l), Si = drinking water standards specified by WHO (2017) for each chemical parameter (mg/l)
Fourth stage Evaluation of sub-index value for each chemical parameter.
where SIi = sub-index value of parameter i, Qi = quality rating scale based on the concentration of parameter i, and Wi = relative weight.
Fifth stage Evaluation of water quality index (WQI).
The sum of sub-indices of each water sample gives the WQI value.
2.4 Geo-statistical analysis
Geo-statistical interpolation techniques (such as kriging) use the statistical characteristics of the measured points (Esri 2001). The geo-statistical analysis establishes a relationship between the quality values in sampling locations and estimates the values in non-sampled areas. As a geo-statistical method, kriging method is a regression-based optimum interpolation method that uses the z values of data locations measured on the basis of spatial covariance values (Kavurmacı 2016). Kriging method provides strong and accurate interpolation. In other words, it is based on statistical models. Efficient use of kriging method requires an advanced level of statistical information. Calculation of an unknown value requires the use of all sample data. Kriging method is a multi-stage process which initially requires the detection of sampling points. An estimated surface model is then specified in accordance with these sampling points (Esri 2014).
In the case of normal data distribution, kriging method yields efficient results. Kriging process takes place in two stages. In the first stage, the spatial structure of data is calculated. In the second stage, an estimated surface is built. The kriging method uses spatial data relationships and the values of the sampling points adjacent to the estimated location to predict an unknown value for a given location (Sharma et al. 2015).
The main advantage of kriging method is its capability to reveal the interpolation-based estimation error for the spatial variable and determine the accuracy and reliability of spatial interpolation (Teikeu et al. 2016). To make a prediction for an unmeasured location, kriging method weights the measured values for adjacent locations, and thus, it provides a weighted sum which is expressed as follows: (Esri 2015).
where Z(Si) is the value measured at location i, λi is an unknown weight for a value measured at the location I, S0 = is the predicted location, N is the number of measured locations. The relationships between the estimated and measured data are determined using cross-validation statistics. In cross-validation, R2 is used as the performance criteria and it should converge to 1 (Kavurmacı 2016). The main characteristic of this method is the use of variograms in the estimation of spatial variations. In the evaluation of water quality, variables such as continuity of quality, range and direction of effect together constitute a function. Variogram γ(h) is a curve that indicates the change in water quality with distance and is expressed with the following equation (Kavurmacı and Üstün 2016).
Variogram should be determined using territorialized variables prior to kriging estimation. Experimental variogram [γ(h)] is defined as the separation distance of the mean semi-squared difference between Z(xi) and Z(xi + h), where γ(h) is the semi-variogram value, h is the lag distance, Z(xi) and Z(xi + h) are the value at point x and the value at point x + h, respectively (Belkhiri and Narany 2015).
RMSE criterion is used to make a comparison between different variogram models and determine the most best-fitted model, where the lowest RMSE value indicates the optimum model for the related data. RMSE criterion is defined with the following equation (Demer and Hepdeniz 2018).
The accuracy of estimations should be determined to define the difference between the measured and estimated values. For accurate estimation results, the RMSE value should be as low as possible and RMSSE (root mean square standardized error) should be close to 1 (Marko et al. 2014; Johnston et al. 2001). Geo-statistical analysis has been widely used to model the distribution of groundwater chemistry, and it is regarded as a combination of numerical techniques which use spatial attributes that are based on random models (Marko et al. 2014). Kriging weights are assigned to each parameter measured to ensure spatial auto-correlation between the measured and estimated locations, thus enabling the evaluation of the parameter for an unknown location (Kumar et al. 2011). The main advantage of ordinary kriging method over the other kriging methods is its simplicity and high estimation accuracy (Gorai and Kumar 2013). In recent years, semi-variogram models such as linear, exponential and spherical models have been widely used in related works (Varouchakis and Hristopulos 2013).
2.5 Multivariable statistical analysis
Multivariable statistical methods provide useful information related to the evaluation of water quality and surface water management. These methods minimize the size of large data sets, thus enabling their classification, modelling and interpretation (Massart and Kaufman 1983; Simeonov et al. 2003).
2.5.1 Correlation matrix
Correlation coefficients are used to establish a correlation between two variables, measure their statistical significance and to take into account the shared variability level between the individual pairs of water quality variables (Kurumbein and Graybill 1965; Gummadi et al. 2014). Correlation analysis measures the proximity between the specified dependent and independent variables. Correlation coefficients which are close to − 1 or + 1 are indicative of a linear correlation between x and y variables (Gummadi et al. 2014). This is also indicative of a high correlation between the two variables, thus indicating a good positive relationship between them. In cases where the correlation coefficient between two variables is zero, there is no correlation at the level of p < .05 between the two variables. If the condition r > .7 is satisfied, this indicates a strong correlation between the parameters, and r values between .5 and .7 indicate a moderate correlation (Manish et al. 2006).
2.5.2 Factor analysis
Factor analysis is an advanced tool used as a statistical method to evaluate the correlation between various parameters. This method was initially introduced by Krumbein (1957) to minimize the number of data required to define a few factors (Narmatha et al. 2011). The main purpose of factor analysis and principal components analysis is to reduce the dimensions of a multivariable data set. The main advantage of both techniques is their capability to preserve the existing information while generating new variables on the basis of linear combinations of the original variables (Mohamed et al. 2015). Factor analysis/principal components analysis has been widely used to analyse the hydro-chemical data set of different groundwater resources for sorting out the most important factors and reduce the amount of data with the least possible loss of information (Mustapha and Aris 2012; Belkhiri and Narany 2015). As a multivariable statistical technique, this method provides a general correlation between the measured chemicals (Varol and Davraz 2015). Principal components analysis reduces the correlations in a data set with multiple variables to a simple set of hidden factors (Islam et al. 2017). Factor analysis can be used to define a limited number of factors that describe the majority of the indices observed during water quality monitoring and evaluate water quality with combined factors (Yu et al. 2003).
In factor analysis, total variance is reduced to a single factor via principal component analysis and the factors are determined accordingly. Additionally, correlation matrices are built using R mode and the principal components are determined using factor eigenvalues. All factors with an eigenvalue higher than 1 (Kaiser 1960) are taken into consideration. Factor 1 has the highest eigenvalue and accounts for the biggest variation in the data set. Factor 2 has the second highest eigenvalue. Liu et al. (2003) classified factor loadings as strong (> .75), moderate (.50–.75) and weak (.30–.50) and used this classification for describing the correlation degree of each component (Varol and Davraz 2015). The factor analysis results applied in the present research were evaluated within the scope of this evaluation scale.
2.6 Piper diagram
Piper trilinear diagram (Piper 1944) provides a graphical illustration of major cations and anions and provides information about the hydro-chemical evolution and facies of water. This diagram enables the determination of the chemical reactions that take place in waters (Talabi 2012; Ebrahimi et al. 2016).
3 Results
3.1 Hydro-chemical properties of surface water
The pH values within the study area varied between 7.3–8.2 and 6.8–8.3 in wet and dry seasons, respectively (Table 1). All pH values within wet and dry seasons remain within the values recommended by the WHO (2017) for drinking water (Figs. 3n, 4n). The average of TDS concentrations is 892.57 mg/l and 1533.5 mg/l, respectively, for wet and dry seasons. TDS concentrations varied between 120–5320 mg/l and between 158–9741 mg/l, respectively, for wet and dry seasons (Table 1). In the wet season, 57.15% of the sampling points in the study area were in freshwater category (TDS < 1000 mg/l) and 42.85% were in brackish water category (TDS > 1000 mg/l). 57.15% and 53.57% of TDS values of Kızılırmak River near Sivas city centre and its tributaries exceeded the upper limit value of 1000 mg/l recommended by the WHO (2017) for drinking purposes, respectively, for wet and dry seasons (Figs. 3p, 4p).
HCO3 concentrations varied between 110.5–291.6 mg/l and 124.2–291.3 mg/l for wet and dry seasons, respectively (Table 1). In wet and dry seasons, 27 sampling points exceeded the upper limit (125 mg/l) of the WHO (2017) for HCO3 concentration and the stations with high HCO3 concentrations were largely located in the southwest of the study area (Figs. 3f, 4f). The average Cl concentrations were found as 154.83 mg/l and 400.29 mg/l, respectively, for wet and dry seasons. In the study area, Cl concentrations varied between 0.63 and 1114 mg/l in wet season, and between 0.96 and 3900 mg/l in dry season. 78.57% and 57.15% of the Cl values remained under the limit values recommended by the WHO (2017), respectively, for wet and dry seasons. Overall, high Cl values were observed in the Kızılırmak River and its tributaries located near Sivas city centre (Figs. 3c, 4c).
In wet season, NH4 varied between 0.007 and 4.72 mg/l, NO2 varied between 0.06 and 0.01 mg/l, and NO3 varied between 0.3 and 21.12 mg/l. In dry season, NH4 varied between 0.007 and 4.91 mg/l, NO2 varied between 0 and 0.11 mg/l, and NO3 varied between 0.8 and 38.5 mg/l (Table 1). In wet seasons, three sampling points and in dry season nine sampling points exceeded the upper limit (0.5 mg/l) of WHO (2017) for NH4 concentrations (Figs. 3k, 4k). In both seasons, all sampling points met the drinking water standards for NO2 and NO3 concentrations recommended by the WHO (2017) (Figs. 3l, m, 4l, m). Higher NH4, NO2 and NO3 concentrations detected in the surface waters in the south of Sivas city centre as compared to the north are ascribed to the sewage discharges coming from Sivas city centre. Particularly, domestic wastewater discharges and agricultural activities adversely affected the water quality of the Kızılırmak River near the south of Sivas city centre.
TH (total hardness) values in the study area varied between 114 and 1916 mg/l in a wet season and between 140 and 2491 mg/l in a dry season. In wet and dry seasons, respectively, 12 and 14 sampling points exceeded the limit value of mg/l recommended by the WHO (2017) for TH. In the wet season, 3.57% of the TH values in the study area were in medium–hard water category, 39.28% were in hard water category and 57.15% were in the very hard water category. In the dry season, 7.15% of the TH values were in the medium–hard category, 25% were in hard water category and 67.85% were in very hard water category (Anbazhagan and Nair 2004) (Figs. 3, 4q). The water quality of Kızılırmak River in the vicinity of Sivas city centre falls into “very hard water” category in terms of total hardness.
In terms of Na concentrations, 3 and 11 samples, respectively, in wet and dry seasons met the limit values recommended by the WHO (2017) (Figs. 3j, 4j). The standards for K values are met by all samples in both seasons (Figs. 3g, 4g). Ca concentrations varied between 31.42–490.2 mg/l and 40.93–931.16 mg/l in wet and dry seasons, respectively (Table 1). In wet and dry seasons, respectively, 14 and 18 sampling locations exceeded the upper limit (100 mg/l) for Ca concentrations (Figs. 2b, 3b). In both seasons, Fe and Mn concentrations remained under the limit values recommended by the WHO (2017) (Figs. 3e, i, 4e, i). Total P values exceeded the related standards in both seasons (Figs. 3r, 4r). SO4 values recommended by the WHO (2017) are met by 12 and 15 sampling locations in wet and dry seasons (Figs. 3o, 4o). The majority of the sampling points met the limit values for Mg concentrations in both seasons (Figs. 3h, 4h). In terms of cation and anion concentrations, the dominant ion in the study area was determined as SO4 and the ion with the lowest concentration was K, in both seasons. The descending order of ion concentrations is SO4, HCO3, Cl, Ca, Na, Mg, K in the wet season and SO4, Cl, Na, Ca, Mg, K in the dry season (Table 1; Figs. 3, 4).
3.2 WQI evaluation
According to WQI calculation method, a weight value (wi) is given primarily for the importance of each parameter in the evaluation of the quality of drinking water and the relative weight values (Wi) of each parameter are calculated (Table 2).
In this research, WQI values of groundwater samples varied between 36.30–392.75 and 52.84–705.12, respectively, for wet and dry seasons. The highest WQI values were observed at sampling station 7 in wet and dry seasons, and the lowest values were observed at sampling stations 3 and 9 (Table 3).
Table 4 shows the levels and definitions of water quality indices based on WQI values. WQI values were evaluated for all observation stations in the study area, and the calculated WQI values were evaluated in accordance with the water quality index levels and definitions (Sadat-Noori et al. 2014; Hadithi 2012; Khwakaram et al. 2012; Rupal et al. 2012) given in Table 4. Etim et al. (2013) found that the WQI values based on the water quality parameters at different sampling stations ranged from 3480 to 36.26 (excellent) for pipe-welded water, 38.52 to 48.67 (excellent) for well water, and 55.05 to 84.94 (well) for the stream. The WQI values obtained as a result of variations of physico-chemical parameters between different water samples provided the recommended standards (Etim et al. 2013). In the study performed by Goher et al. (2014), WQI values ranged between 43.68 and 65.48 for drinking water purpose. This study indicates that the water quality fluctuation could be classified from good to poor water for drinking water purposes. In our study, WQI-based water quality classification (Table 4) shows that in the wet season 17.85% of the water samples are in “excellent” category, 39.28% are in “good” category, 39.28% are in “poor” category, and 3.57% are in “not suitable for drinking” category. In the dry season, 46.42% of WQI values are in “good” category, 28.57% are in “poor” category, 21.42% are in “very poor” category, and 3.57% are in “not suitable for drinking” category. In both seasons, the regions remaining in “excellent water” category are mostly the located in the south and north of the Kızılırmak River. Particularly the part of the river in the south of Sivas city centre was in the “good water” category in the wet season, whereas it shifted to “poor water” category in the dry season (Table 4, Figs. 3s, 4s).
3.3 Geo-statistical evaluation
Gharbia et al. (2016) expressed that all parameters of groundwater quality have a strong spatial structure. Low RMSE values obtained for ten water quality parameters indicate that the model is well understood (Gharbia et al. 2016). In this work, ordinary kriging and semi-variogram models were applied to determine the spatial distribution of water quality on Kızılırmak River. RMSE values were determined to reveal the best-fitted semi-variogram model for all parameters used in the research. The optimum models were determined on the basis of the lowest RMSE values for all parameters. Gaussian was determined as the best-fitted model for BOD, Na, TDS, TH and WQI parameters, and the exponential model was specified as the best model for NH4 and SO4 parameters. A spherical model was determined as the best model for all remaining parameters. The best-fitted semi-variogram models for all parameters did not vary in wet and dry seasons (Table 5a, b). The RMSE values determined for the accuracy of estimation results varied between .010 and 1006.21 in the wet season and between .025 and 1778.29 in the dry season. RMSSE values, which are expected to converge to 1, were found to meet the related standard value in both seasons (Table 5a, b).
Values under 25% are indicative of high dependence, those between 25 and 75% indicate moderate dependency and those higher than 75% indicate low dependency (Nayanaka et al. 2010; Mehrjardi et al. 2008; Demer and Hepdeniz 2018). Marko et al. (2014) emphasized that other parameters except NO3 and temperature have strong spatial dependence, NO3 exhibits moderate spatial dependence, and temperature shows weak spatial dependence. Nugget/Sill ratio was used to determine the spatial dependence of surface water quality parameters for the Kızılırmak River. In the wet season, the spatial dependence (spatial correlation) between all parameters except NO2 and TDS was high and in the dry season the spatial dependence (spatial correlation) between all parameters except TDS was also high (Figs. 7, 8; Table 5a, b). Spatial dependence mainly depends on the factors such as existing aquifer geology, groundwater source, rainfall and infiltration processes as well as the groundwater topography that varies depending on the agricultural, residential and industrial areas (Bhuiyan et al. 2016).
Cross-validation technique was used to determine the correlations between the measured and estimated values for all parameters used in the research. The validity and accuracy of the variogram model can be tested using cross-validation (Kyriakidis 2004; Teikeu et al. 2016). Here, the aim is to evaluate how accurately the surface quality parameters are estimated in the non-sampled regions of the study area. In this method, a correlation coefficient (R2), which is supposed to converge to 1 for accurate estimation, is used for cross-validation (Kavurmacı 2016; Esri 2015). Accordingly, the distribution graphs were plotted for the measured and the estimated (by geo-statistical analysis–kriging method) values for all parameters and their R2 were evaluated for both seasons. The parameters with the highest R2 (.96) were determined as Mn, NO2 and total P in the wet season, and as Mn (.96) in the dry season. The parameters with the highest R2 values indicate that there is a high correlation between the measured and estimated values (Figs. 5, 6). The spatial distribution maps that show the estimated water quality of Kızılırmak River and its tributaries were prepared by use of the best-fitted variograms determined using the RMSE, RMSSE and R2 values on the basis of geo-statistical analysis and cross-validation-based statistical analyses, and these are shown in Figs. 3 and 4.
Figures 7 and 8 show the experimental semi-variogram (distribution points) around the omnidirectional semi-variogram model for all parameters used in the research. In the figures, the blue line shows the semi-variogram model, and the plus (+) sign shows the average of semi-variogram studies. A semi-variogram model shows the levels of the model at a given distance. The distance at which the model becomes linear is known as the range. The sample locations that are separated at distances which are shorter than the range are spatially auto-correlated, and more distant points are not auto-correlated. The value yielded by the semi-variogram model at the range (the value on y-axis) is termed as the threshold (Esri 2015). In this research, the range values belonging to the semi-variogram models built for wet and dry seasons for all parameters vary between 14.14 km and 209.50 (Table 5a, b). Such variations in the range can be ascribed to pollutant sources, agricultural activities and the factors which are effective on water quality (Islam et al. 2018). The geo-statistical results obtained in this study are in parallel with the geo-statistical results obtained in the literature studies.
3.4 Classification of surface water quality via Piper diagram
The analysis results of water samples that represent the surface water in the study area were shown in Piper diagram for both wet and dry seasons, and the hydro-chemical facies of the water samples were determined, accordingly. In the study area, the amount of alkali earth elements (Ca + Mg) in the surface water samples is higher than the amount of alkali elements (Na + K), and the total number of weak acid radicals (HCO3 + CO3) is lower than the total amount of strong acid radicals (SO4 + Cl). As indicated in the Piper diagram, the majority of water samples are in Ca–Mg–SO4–HCO3 water facies (Fig. 9a, b). High SO4 values observed in most parts of the study area mainly arise from the interaction of gypsum formations (due to the lithological structure of the area) with water in the study area. Also, the water samples being in Ca–Mg–SO4–HCO3 waters facies are indicative of the high water hardness and salinity in the study area.
3.5 Multivariable statistical evaluation
3.5.1 Correlation matrix evaluation
Liu et al. (2011) showed that there were positive correlations between TP, NH4-N, TN, TSS and negative correlations between DO, temperature, NH4-N and TN parameters. In this study, a correlation matrix was built both for wet and dry seasons using the anions, cations and other physical and chemical parameters that characterize the hydro-chemical composition of surface water (Table 6a, b). In the wet season, there is a moderate correlation between BOD and K values (r = .551; P < .05) and a negative correlation between the BOD and DO values (r = − .406; P < .05). Strong positive correlations were observed between Ca values and Cl, Na, NO3, SO4, TDS and TH values (r = .912, r = .858, r = .797, r = .958, r = .924 ve r = .979; P < .05). Low positive correlations were observed between Ca values and K and total P values (r = .421, r = .436; P < .05), and moderate positive correlations were observed between Ca values and Mg and NH4 (r = .622, r = .677; P < .05).
Strong positive correlations were found between Cl values and Na, NO3, SO4, TDS and TH values (r = .985, r = .932, r = .869, r = .958, r = .986, r = .949; P < .05), and low positive correlations were observed between Cl values and Mg and NH4 values (r = .475, r = .480; P < .05). Moderate positive correlations were observed between Cl values and total P values (r = .613; P < .05), low positive correlation was observed between DO values and NH4 values (r = .400; P < .05), and negative correlation was observed between DO values and K values (r = − .388; P < .05). No statistically significant difference was found between the sampling stations in terms of Fe and Mn values of surface water (P > .05). Low positive correlation was detected between K values and pH values (r = .455; P < .05), whereas moderate positive correlations were detected between K values and Mg and SO4 values (r = .661, r = .546; P < .05). Negative correlation was found between HCO3 values and pH values (r = − .416; P < .05). Likewise, strong positive correlations were found between Mg values and SO4 values, Na values and NO3, TDS and TS values, NO3 values and SO4, TDS, TH and total P values, SO4 values and TDS and TH values, and TDS values and TH values (r > .7).
In the dry season, no statistically significant difference was found between the sampling stations in terms of BOD, Fe, Mn and NO2 values of surface water samples (P > .05). Strong positive correlations were found between Ca values and SO4, Cl, Na, NH4, NO3, SO4, TDS, TH values; Cl values and Na, NO3, SO4, TDS, TH values; Mg values and total P values; Na values and NO3, SO4, TDS, TH values; NH4 values and SO4, TDS, TH values; NO3 values and SO4, TDS, TH values; SO4 values and TDS, TH values; and TDS values and TH values (r > .7) (Table 6b). In both seasons, the correlations between all parameters can vary depending on the chemical reactions between ions.
3.5.2 Factor analysis evaluation
Some researchers (Liu et al. 2011; Howladar et al. 2018, Gu et al. 2016; Simeonov et al. 2003) used factor analysis to evaluate the relationship between various parameters. Factor 1 accounted for 22.1% of the total variance and correlated with COD, BOD5, TON, TP and PO43−. This “organic” factor can be interpreted to represent effects from point sources such as municipal and industrial wastes. Factor 2 constitutes 19.8% of the total variance, and factor 2 correlated with mainly water-soluble N-types, NO2, NH4 and NO3, and secondary to PO43− or TP. This nutrient factor represents effects from non-point sources such as agricultural flow and atmospheric accumulation. Factor 3 is weighted on pH, DO and EC and represents the physical–chemical source of variability (Simeonov et al. 2003).
Factor 1 can be connected to un-controlled domestic discharges (Su et al. 2011; Gu et al. 2016). Factor 2 can be attributed to biochemical contamination (Zhou et al. 2007). Factor 3 can be caused by pollution from domestic discharges and from agriculture and surface flow (Gu et al. 2016).
The results of factor analysis and principal components analysis performed using 18 parameters at all surface water sampling stations on the Kızılırmak River are shown in Table 7. As a result of the performed analyses, three factors eigenvalues of which were higher than 1 were considered. These three factors describe 69.17% of total variance in the wet season, and 69.96% of total variance in the dry season. In wet season, the first factor describes 45.75% of total variance, and in this factor, Ca, Cl, Na, NO3, SO4, TDS and TH parameters are represented with positive strong correlations. Mg, NH4 and total P parameters were represented with positive moderate correlations, whereas pH parameter was represented with a negative moderate correlation. As indicated by the capability of the first factor, that incorporates the majority of water parameters, to describe total variance, this factor is capable of representing the water quality by itself. This factor indicates that the surface water samples in the study area are rich in Ca, Cl, Na, SO4, TDS and TH. This is mainly attributable to the fact that surface water is fed by groundwater which is in direct contact with soil and rock formations available in the study area’s geologic structure. In addition, this factor is indicative of high NO3 values in several surface water samples. Agricultural activities and un-controlled discharges from sewage have increased the effectiveness of NO3 on factor 1.
In the dry season, the first factor describes 49.27% of total variance, and in this factor, Ca, Cl, Na, NH4, NO3, SO4, TDS and TH parameters are represented with a positive strong correlation level; K and Mg parameters are represented with a positive moderate level, and DO and pH parameters are represented with a negative moderate correlation level. In the wet season, the second factor describes 13.78% of total variance where K parameter is represented with a positive strong correlation; and BOD, Mg and pH parameters are represented with a positive moderate correlation. The positive strong correlation level of K parameter is attributable to its being a K-rich mineral-based factor. In the dry season, the second factor describes 12.17% of total variance. In the dry season, there is no parameter with a strong positive correlation in this factor; and Mg, Mn and pH parameters are represented with positive moderate correlation; and HCO3 is represented with a negative moderate correlation. In the wet season, the third factor describes 9.62% of total variance, where DO and NH4 parameters are represented with a positive moderate correlation. In the dry season, the third factor describes 8.51% of total variance where total P is represented with a positive moderate correlation; and NO2 is represented with a negative moderate correlation. The results obtained from our study support the results obtained according to the literature studies.
3.6 Final surface water quality map
Weighted overlay method was used to build the final surface quality map for the study area. Sub-class units were established for each parameter considering the value intervals (Table 8) in terms of suitability for drinking purposes. Afterwards, considering the suitability of each parameter for drinking purposes, positive values varying between 1 and 4 were assigned to the sub-classes specified for each parameter. The spatial distribution maps, prepared for the water quality parameters of all sampling stations using geo-statistical analysis, were re-classified in accordance with the suitability intervals set for drinking water quality. Finally, the resulting thematic maps were subjected to weighted overlaying for each parameter using the weighed overlaying module of ArcGIS 10.2 software package.
The final surface water quality maps prepared using weighted overlaying for wet and dry seasons are shown in Fig. 10a, b. The water quality in the study area is categorized as excellent, good, poor and very poor. The regions with excellent water quality are the endpoints of the tributaries of Kızılırmak River. The water quality of the Kızılırmak River near the city centre of Sivas and in the south of the city was categorized as poor and very poor in wet and dry seasons, respectively. As the season shifts to dry from wet, the excellent water quality of Kızılırmak River did not change significantly, whereas the regions classified with the other quality levels (good and poor) were adversely affected.
4 Conclusions
Population growth and urbanization are threats to water resources. As a result of climate change, water resources decrease and the quality of existing water resources should be maintained. Due to population growth and urbanization, river water quality deteriorates and concerns over the reduction of river water quality arise. In this context, it has become mandatory to develop geo-statistical and multivariate statistical techniques for analysing, using and interpreting data sets of river quality management in order to overcome such concerns.
This research was carried out to evaluate the water quality of Kızılırmak River in Kızılırmak Basin within the provincial boundaries of Sivas using geo-statistical and multivariable statistical approaches. The spatial distribution maps were prepared in accordance with the best-fitted models for each parameter on the basis of the statistical values (RMSE, RMSSE, R2) obtained as a result of the geo-statistical analysis. A spherical model was determined as the best-fitted variogram for 66.66% of the surface water quality parameters. Piper diagram that reflects the hydro-chemical characteristics of the Kızılırmak River showed that the dominant water type is waters with Ca–Mg–SO4–HCO3 content. Correlation results showed that positive and negative correlations arise between ions depending on the dissolution, sedimentation and evaporation mechanisms within surface waters. In the wet season, the strongest positive correlation was detected between Cl values and TDS values (r = .986), while in the dry season the strongest positive correlation was detected between Cl values and Na values (r = .998). Factor analysis results show that the first factor has stronger positive loadings than the second and third factors as a characteristic of the surface waters in the study area.
The final map of the surface water quality, which was formed according to the weighted registration method, revealed the final surface water quality of the Kızılırmak River and its lateral branches. The spatial distribution maps of WQI calculated for wet and dry seasons and the final surface water quality maps clearly indicate the suitability of surface water quality of the Kızılırmak River for drinking purposes. The water quality at the endpoints of the tributaries of Kızılırmak River is in “excellent water” category. The portion of Kızılırmak River in the south of Sivas city centre is in “poor water” category in the wet season and in “very poor water” category in a dry season. Particularly, agricultural activities and urban wastewater discharges are considered to have an adverse effect on the water quality of the Kızılırmak River.
Consequently, this study shows that geo-statistical and multivariate statistical approaches can be useful in defining the sources of pollutants, easily understanding and interpreting the water quality data of complex structures, and determining the water quality based on spatial analysis. Geo-statistical and multivariate statistical approaches have shown that the development of monitoring strategies for the management of Kızılırmak river water quality and the analysis of GIS-based pollutant levels can generate useful information for experts and decision makers on the planning of river water resources.
References
Akoteyon, I. S., Omotayo, A. O., Soladoye, O., & Olaoye, H. O. (2011). Determination of water quality index and suitability of urban river for municipal water supply in Lagos-Nigeria. European Journal of Scientific Research,54, 263–271.
Ambiga, K., & Durai, R. A. (2013). Use of Geographical Information System and water quality index to assess groundwater quality in and around ranipet area, Vellore district, Tamilnadu. International Journal of Advanced Engineering Research and Studies/II/IV/July-Sept.2013/73-80.
Anbazhagan, S., & Nair, A. M. (2004). Geographic information system and groundwater quality mapping in Panvel Basin, Maharashtra, India. Environmental Geology,45, 753–761.
Belkhiri, L., & Narany, T. S. (2015). Using multivariate statistical analysis, geostatistical techniques and structural equation modeling to identify spatial variability of groundwater quality. Water Resources Management,29, 2073–2089.
Ben-Jemaa, F., Marino, M. A., & Loaiciga, H. A. (1994). Multivariate geostatistical design of ground-water monitoring networks. Journal of Water Resources Planning and Management,120, 505–522.
Bharti, N., & Katyal, D. (2011). Water quality indices used for surface water vulnerability assessment. International Journal of Environmental Science,2, 154–173.
Bhuiyan, M. A. H., Bodrud-Doza, M., Islam, A. T., Rakib, M. A., Rahman, M. S., & Ramanathan, A. L. (2016). Assessment of groundwater quality of Lakshimpur district of Bangladesh using water quality indices, geostatistical methods, and multivariate analysis. Environmental Earth Sciences,75, 1020.
Brown, R. M., McClelland, N. I., Deininger, R. A., & Tozer, R. G. (1970). Water quality index-do we dare? Water Sewage Works,117, 339–343.
Büttner, O., Becker, A., Kellner, S., et al. (1998). Geostatistical analysis of surface sediments in an acidic mining lake. Water Air Soil Pollution,108, 297–316.
Demer, S., & Hepdeniz, K. (2018). Mapping of groundwater quality in Isparta city center by using geostatistical methods with geographical information systems. Ömer Halisdemir University Journal of Engineering Sciences,7, 757–771.
Ebrahimi, M., Kazemi, H., Ehtashemi, M., & Rockaway, T. D. (2016). Assessment of groundwater quantity and quality and salt water intrusion in the Damghan basin. Iran. Chemie der Erde,76, 227–241.
Ella, V., Melvin, S., & Kanwar, R. (2001). Spatial analysis of NO3-N concentration in glacial till. Transactions of the ASAE,44, 317–327.
ESRI. (2001). Environmental Systems Research Institute, Using ArcGIS geostatistical analyst (300 p.), USA.
Esri. (2014). ArcMap 10.1 Spatial Analysis (213 p), Esri Information Systems Engineering and Training Limited Company, Ankara.
Esri. (2015). ArcMap 10.1 Help File. Online, http://resources.arcgis.com/en/help/main/10.1/. Accessed May 25, 2018.
Etim, E. E., Odoh, R., Itodo, A. U., Umoh, S. D., & Lawal, U. (2013). Water quality index for the assessment of water quality from different sources in the Niger Delta Region of Nigeria. Frontiers In Science,3(3), 89–95.
Gharbia, A. S., Gharbia, S. S., Abushbak, T., Wafi, H., Aish, A., Zelenakova, M., et al. (2016). Groundwater quality evaluation using GIS based geostatistical algorithms. Journal of Geoscience and Environment Protection,4, 89.
Goher, M. E., Hassan, A. M., Abdel-Moniem, I. A., Fahmy, A. H., & El-Sayed, S. M. (2014). Evaluation of surface water quality and heavy metal indices of Ismailia Canal, Nile River, Egypt. The Egyptian Journal of Aquatic Research,40(3), 225–233.
Gorai, A. K., & Kumar, S. (2013). Spatialdistribution analysis of groundwater quality index using GIS: A case study of Ranchi Municipal Corporation (RMC) area. GeoinfoGeostat Over,1, 2.
Gu, Q., Zhang, Y., Ma, L., Li, J., Wang, K., Zheng, K., et al. (2016). Assessment of reservoir water quality using multivariate statistical techniques: a case study of Qiandao Lake,China. Sustainability,8(3), 243.
Gummadi, S., Swarnalatha, G., Vishnuvardhan, Z., & Harika, D. (2014). Statistical analysis of the groundwater samples from bapatla mandal, guntur district, andhra pradesh, India. Journal of Environmental Science, Toxicology and Food Technology,8, 27–32.
Hadithi, M. A. (2012). Application of water quality index to assess suitability of groundwater quality for drinking purposes in Ratmao-Pathri Rao watershed, Haridwar District, India. American Journal of Scientific&Industrial Research,3, 395–402.
Haque, M. M., Rahman, A., Hagare, D., & Kibria, G. (2014). Probabilistic water demand forecasting using projected climatic data for Blue Mountains Water Supply System in Australia. Water Resources Management,28, 1959–1971.
Horton, R. K. (1965). An index number system for rating water quality. Journal of the Water Pollution Control Federation,37, 300–305.
Howell, E. T. (2018). Influences on water quality and abundance of cladophora, a shore-fouling green algae, over urban shoreline in Lake Ontario. Water,10, 1569.
Howladar, M. F., Rahman, M. M., Anas, F. S. A., & Shine, F. M. M. (2018). A chemical and multivariate statistical approach to assess the spatial variability of soil quality for environment around the Tamabil coal stockpile, Sylhet. Environmental Systems Research,7(1), 19.
Islam, M. A., Rahman, M. M., Bodrud-Doza, M., Muhib, M. I., Shammi, M., Zahid, A., et al. (2018). A study of groundwater irrigation water quality in south-central Bangladesh: a geo-statistical model approach using GIS and multivariate statistics. Acta Geochimica,37, 193–214.
Islam, M. A., Zahid, A., Rahman, M. M., Rahman, M. S., Shammi, M., et al. (2017). Investigation of groundwater quality and its suitability for drinking and agricultural use in the south central part of the coastal region in Bangladesh. Exposure and Health,9, 27–41.
Johnston, K., Hoef, J. M. V., Krivoruchko, K., & Lucas, N. (2001). ArcGIS® 9: Using ArcGIS® Geostatistical Analyst.
Kaiser, H. F. (1960). The application of electronic computers to factor analysis. Educational and Psychological Measurement,20, 141–151.
Kavurmacı, M. (2016). Evaluation of groundwater quality using a GIS-MCDA-based model: A case study in Aksaray, Turkey. Environmental Earth Sciences,75, 1–17.
Kavurmacı, M., & Üstün, A. K. (2016). Assessment of groundwater quality using DEA and AHP: a case study in the Sereflikochisar region in Turkey. Environmental Monitoring and Assessment,188, 1–13.
Khwakaram, A. I., Majid, S. N., & Hama, N. Y. (2012). Determination of water quality index (WQI) for Qalyasan stream in Sulaimanı City/Kurdistan region of Iraq. International Journal of Plant, Animal and Environmental Sciences,2, 148–157.
Krumbein, W. C. (1957). Comparison of percentage and ratio data in facies mapping. Journal of Sedimentary Petrology,27, 293–297.
Kumar, P. S., Jegathambal, P., & James, E. J. (2011). Multivariate and geostatistical analysis of groundwater quality in Palar river basin. Internatıonal Journal of Geology,4, 108–119.
Kumar, P., Kaushal, R. K., & Nigam, A. K. (2015). Assessment and management of ganga river water quality using multivariate statistical techniques in India. Asian Journal of Water, Environment and Pollution,12, 61–69.
Kurumbein, W. C., & Graybill, F. A. (1965). An introduction to statistical models in geology. New York: McGraw-Hill.
Kyriakidis, P. (2004). A geostatistical framework for area-to-point spatial interpolation. Geographical Analysis,36, 259–289.
Leong, S., Ismail, J., Denil, N., Sarbini, S., Wasli, W., & Debbie, A. (2018). Microbiological and Physicochemical Water Quality Assessments of River Water in an Industrial Region of the Northwest Coast of Borneo. Water,10(11), 1648.
Li, Y., Xu, L., & Li, S. (2009). Water quality analysis of the Songhua River Basin using multivariate techniques. Journal of Water Resource and Protection,1, 110.
Liu, C. W., Lin, K. H., & Kuo, Y. (2003). Application of factor analysis in the assessment of groundwater quality in a blackfoot disease area in Taiwan. Science of The Total Environment,313(1-3), 77–89.
Liu, W. C., Yu, H. L., & Chung, C. E. (2011). Assessment of water quality in a subtropical alpine lake using multivariate statistical techniques and geostatistical mapping: a case study. International Journal of Environmental Research and Public Health,8, 1126–1140.
Manish, K., Ramanathan, A., Rao, M. S., & Kumar, B. (2006). Identification and evaluation of hydrogeochemical processes in the groundwater environment of Delhi, India. Environmental Geology,50, 1025–1039.
Marko, K., Al-Amri, N. S., & Elfeki, A. M. (2014). Geostatistical analysis using GIS for mapping groundwater quality: case study in the recharge area of Wadi Usfan, western Saudi Arabia. Arabian Journal of Geosciences,7, 5239–5252.
Massart, D. L., & Kaufman, L. (1983). The interpretation of analytical chemical data by the use of cluster analysis. New York: Wiley.
Mehrjardi, R. T., Jahromi, M. Z., Mahmodi, S., & Heidari, A. (2008). Spatial distribution of groundwater quality with geostatistics. World Applied Sciences Journal,4, 9–17.
Mohamed, I., Othman, F., Ibrahim, A. I., Alaa-Eldin, M. E., & Yunus, R. M. (2015). Assessment of water quality parameters using multivariate analysis for Klang River basin, Malaysia. Environmental Monitoring and Assessment,187(1), 4182.
Mustapha, A., & Aris, A. Z. (2012). Multivariate statistical analysis and environmental modeling of heavy metals pollution by industries. Polish Journal of Environmental Studies,21, 1359–1367.
Narmatha, T., Jeyaseelan, A., Mohan, S. P., & Mohan, R. V. (2011). Integrating Multivariate Statistical Analysis with GIS for Groundwater in Pambar Sub-Basin, Tamil Nadu, India. International Journal of Geomatics and Geosciences,2, 392.
Nayanaka, V. G. D., Vitharana, W. A. U., & Mapa, R. B. (2010). Geostatistical analysis of soil properties to support spatial sampling in a paddy growing alfisol. Tropical Agricultural Research,22, 34–44.
Piper, A. M. (1944) A graphic procedure in the geochemical interpretation of water-analyses. Transactions, American Geophysical Union,25(6), 914.
Poisson, A., Guezou, J. C., Öztürk, A., İnan, S., Temiz, H., Gürsoy, H., et al. (1996). Tectonic setting and evolution of the Sivas Basin, Central Anatolia, Turkey. International Geology Review,38, 838–853.
Rupal, M., Tanushree, B., & Sukalyan, C. (2012). Quality characterization of groundwater using water quality index in Surat city, Gujarat, India. International Research Journal of Environment Sciences,1, 14–23.
Sadat-Noori, S. M., Ebrahimi, K., & Liaghat, A. M. (2014). Groundwater quality assessment using the water quality index and GIS in Saveh-Nobaran aquifer, Iran. Environmental Earth Sciences,71, 3827–3843.
Sharma, P. K., Vijay, R., & Punia, M. P. (2015). Geostatistical evaluation of groundwater quality distribution of Tonk district, Rajasthan. International Journal Of Geomatics And Geosciences,6, 1474–1485.
Simeonov, V., Stratis, J. A., Samara, C., Zachariadis, G., Voutsa, D., Anthemidis, A., et al. (2003). Assessment of the surface water quality in Northern Greece. Water Research,37, 4119–4124.
Singh, K. P., Malik, A., Mohan, D., & Sinha, S. (2004). Multivariate statistical techniques for the evaluation of spatial and temporal variations in water quality of Gomti river (India): A case study. Water Research,38, 3980–3992.
Su, S., Zhi, J., Lou, L., Huang, F., Chen, X., & Wu, J. (2011). Spatio-temporal patterns and source apportionment of pollution in Qiantang River (China) using neural-based modeling and multivariate statistical techniques. Physics and Chemistry of the Earth, Parts A/B/C, 36(9–11), 379–386.
Sutadian, A. D., Muttil, N., Yilmaz, A. G., & Perera, B. J. C. (2016). Development of river water quality indices—A review. Environmental Monitoring and Assessment,188, 58.
Teikeu, W. A., Meli’i, J. L., Nouck, P. N., Tabod, T. C., Nyam, F. E. A., & Aretouyap, Z. (2016). Assessment of groundwater quality in Yaoundé area, Cameroon, using geostatistical and statistical approaches. Environmental Earth Sciences,75, 21.
Tyagi, S., Sharma, B., Singh, P., & Dobhal, R. (2013). Water quality assessment in terms of water quality index. American Journal of Water Resources,1, 34–38.
Vairavamoorthy, K., Yan, J. M., Galgale, H. M., & Gorantiwar, S. D. (2007). IRA-WDS: A GIS-based risk analysis tool for water distribution systems. Environmental Modeling & Software,22, 951–965.
Varol, S., & Davraz, A. (2015). Evaluation of the Groundwater quality with WQI (Water Quality Index) and multivariate analysis: A case study of the Tefenni Plain (Burdur/Turkey). Environmental Earth Sciences,73, 1725–1744.
Varouchakis, E. A., & Hristopulos, D. T. (2013). Improvement of groundwater level prediction in sparsely gauged basins using physical laws and local geographic features as auxiliary variables. Advances in Water Resources,52, 34–49.
Wang, Y., Wang, P., Bai, Y., Tian, Z., Li, J., Shao, X., et al. (2013). Assessment of surface water quality via multivariate statistical techniques: a case study of the Songhua River Harbin region, China. Journal of Hydro-Environment Research,7, 30–40.
WHO. (2017). Guidelines for drinking water quality: training pack (4th ed.). Geneva, Switzerland: Incorporating The First Addendum.
Wu, Z., Wang, X., Chen, Y., Cai, Y., & Deng, J. (2018). Assessing river water quality using water quality index in Lake Taihu Basin, China. Science of the Total Environment,612, 914–922.
Wu, C., Wu, J., Luo, Y., Zhang, H., Teng, Y., & DeGloria, S. D. (2011). Spatial interpolation of severely skewed data with several peak values by the approach integrating kriging and triangular irregular network interpolation. Environmental Earth Sciences,63, 1093–1103.
Yan, C. A., Zhang, W., Zhang, Z., Liu, Y., Deng, C., & Nie, N. (2015). Assessment of water quality and identification of polluted risky regions based on field observations & GIS in the honghe river watershed, China. PLoS ONE,10, 1–13.
Yu, S., Shang, J., Zhao, J., & Guo, H. (2003). Factor analysis and dynamics of water quality of the Songhua River, Northeast China. Water Air and Soil Pollution,144, 159–169.
Yüce, M. İ., & Ercan, B. (2015). Determination of rainfall-flow relationship in Kızılırmak Basin. 4. In Water Structures Symposium, 410–418, 19–20 November, Antalya.
Zhao, Z. W., & Cui, F. Y. (2009). Multivariate statistical analysis for the surface water quality of the Luan River, China. Journal of Zhejiang University-SCIENCE A,10, 142–148.
Zhou, F., Huang, G. H., Guo, H., Zhang, W., & Hao, Z. (2007). Spatio-temporal patterns and source apportionment of coastal water pollution in eastern Hong Kong. Water Research,41, 3429–3439.
Acknowledgements
The data used in this study have been provided within the scope of the project supported by Sivas Cumhuriyet University (Turkey) Scientific Research Projects unit (Project Number: MF-002). I would like to thank the staff of the General Directorate of State Hydraulic Works (Ankara/Turkey), who helped us with water quality data.
Author information
Authors and Affiliations
Corresponding author
Additional information
Publisher's Note
Springer Nature remains neutral with regard to jurisdictional claims in published maps and institutional affiliations.
Rights and permissions
About this article
Cite this article
Karakuş, C.B. Evaluation of water quality of Kızılırmak River (Sivas/Turkey) using geo-statistical and multivariable statistical approaches. Environ Dev Sustain 22, 4735–4769 (2020). https://doi.org/10.1007/s10668-019-00472-8
Received:
Accepted:
Published:
Issue Date:
DOI: https://doi.org/10.1007/s10668-019-00472-8